The notes when study the Coursera class by Mr. Andrew Ng "Neural Networks & Deep Learning", section 3.2 "Neural Network Representation". Share it with you and hope it helps!
------------------
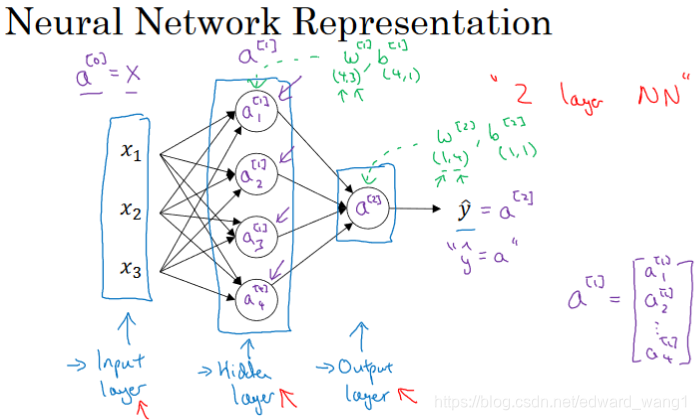
Figure-1 shows names of different parts of Neural Network.
- It has Input layer, hidden layer and output layer
- The term hidden layer refers to the fact that in a training set the true values for these nodes in the middle are not observed.
- An alternative notation for the input x is
where a stands for activation
- The hidden layer generates some set of activations called
. The first unit generates value
, the second unit generates value
and so on. So here
- The output layer generates value
which is just a real number. And
- One funny thing is this neural network here is called a 2-layer NN in convention. The reason is we don't count input layer.
- The hidden layer will have parameters associated with it:
and
- The output layer will have parameters associated with it:
and
<end>