在处理深度学习图片分类问题时,训练素材的数量是一个很大的问题,除了疯狂的采集图片,通过对图片预处理的方式,可以提高图片数量,降低训练干扰因素,提高识别确认度。
读取图片,转换为实数后方便后边对图片进行转换处理。
image_raw_data = tf.gfile.FastGFile(temp_path, 'rb').read()
img_data = tf.image.decode_jpeg(image_raw_data)
# 数据类型转换为实数方便程序对图像进行处理
img_data = tf.image.convert_image_dtype(img_data, dtype=tf.float32)
img_data = tf.image.convert_image_dtype(img_data, dtype=tf.uint8)
思路:通过对图片进行翻转、放大、亮度调节、对比度调节、色相调节、饱和度调节、灰度图片,进行训练素材的扩展。减少色差光线等因素对训练结果的影响。
# 左右翻转
flipped = tf.image.flip_left_right(img_data)
#上下翻转
flipped = tf.image.flip_up_down(img_data)
#调整图像的大小 双线性插值法
resized = tf.image.resize_images(img_data, [720, 1280], method=0)
resized = np.asarray(resized.eval(), dtype='uint8')
adjusted = tf.image.resize_image_with_crop_or_pad(resized,616 , 980)
# 在[-max_delta, max_delta)的范围随机调整图片的亮度。
adjusted = tf.image.random_brightness(img_data, max_delta=0.2)
# 在[lower, upper]的范围随机调整图的对比度。
adjusted = tf.image.random_contrast(img_data, 0.5, 1)
# 在[-max_delta, max_delta]的范围随机调整图片的色相。max_delta的取值在[0, 0.5]之间。
adjusted = tf.image.random_hue(img_data, 0.1)
# 在[lower, upper]的范围随机调整图的饱和度。
adjusted = tf.image.random_saturation(img_data, 0.0, 2.0)
#灰度图片
adjusted = tf.image.rgb_to_grayscale(img_data)
处理后图片为:
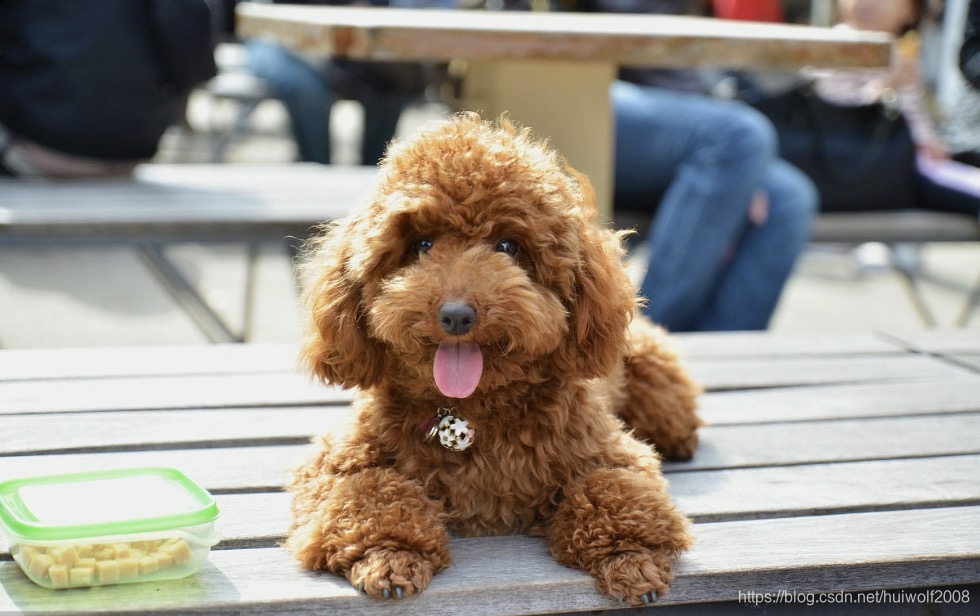
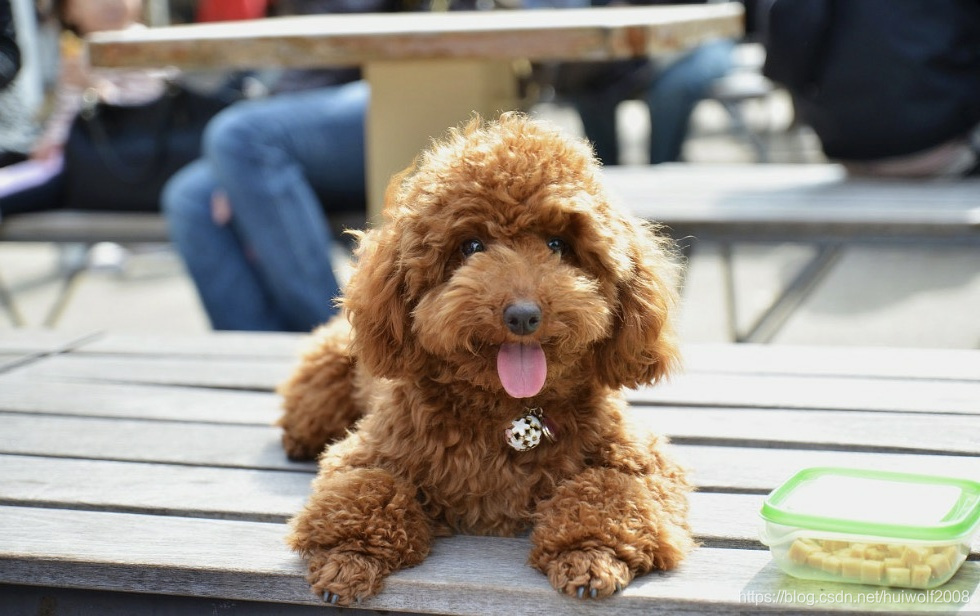
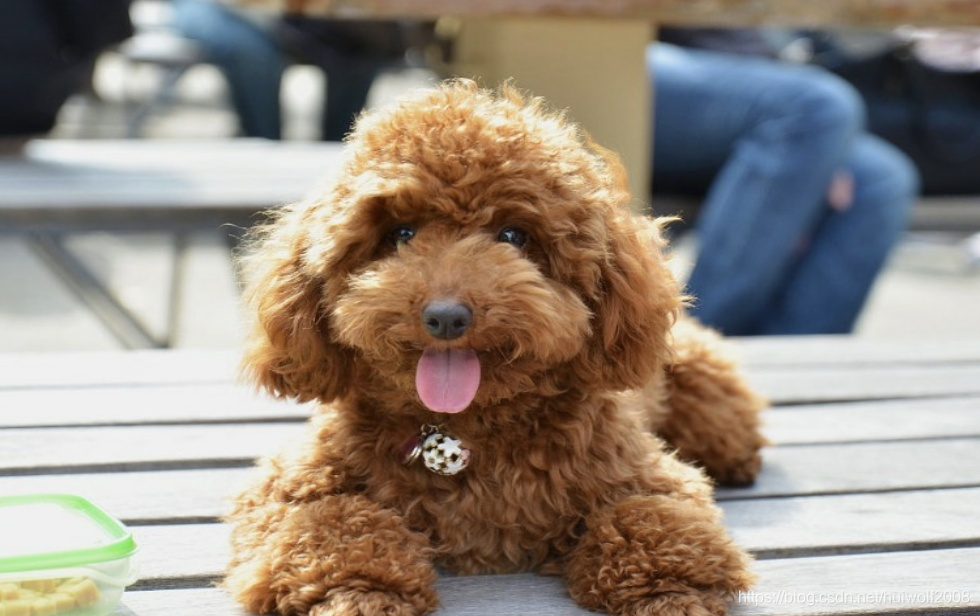
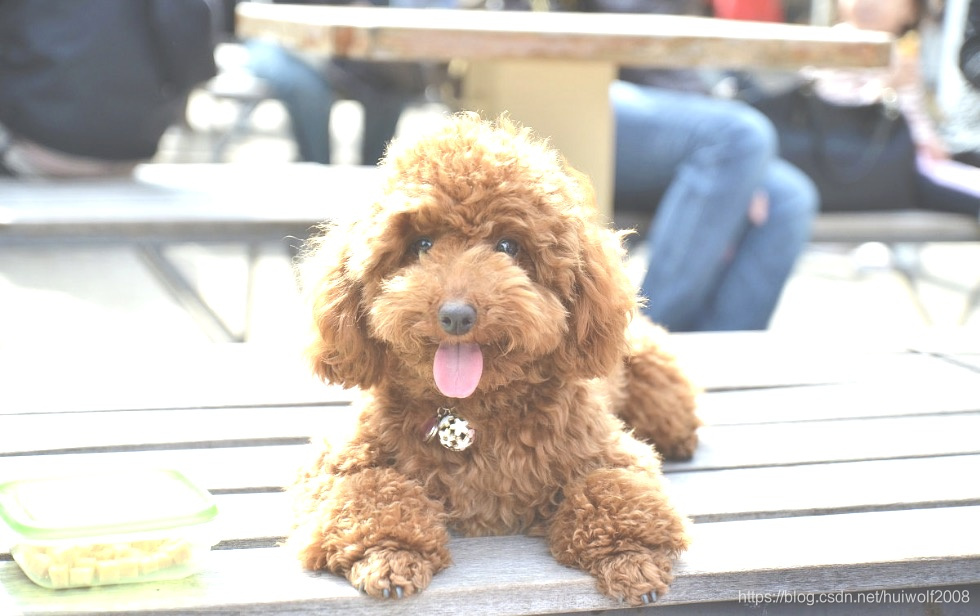
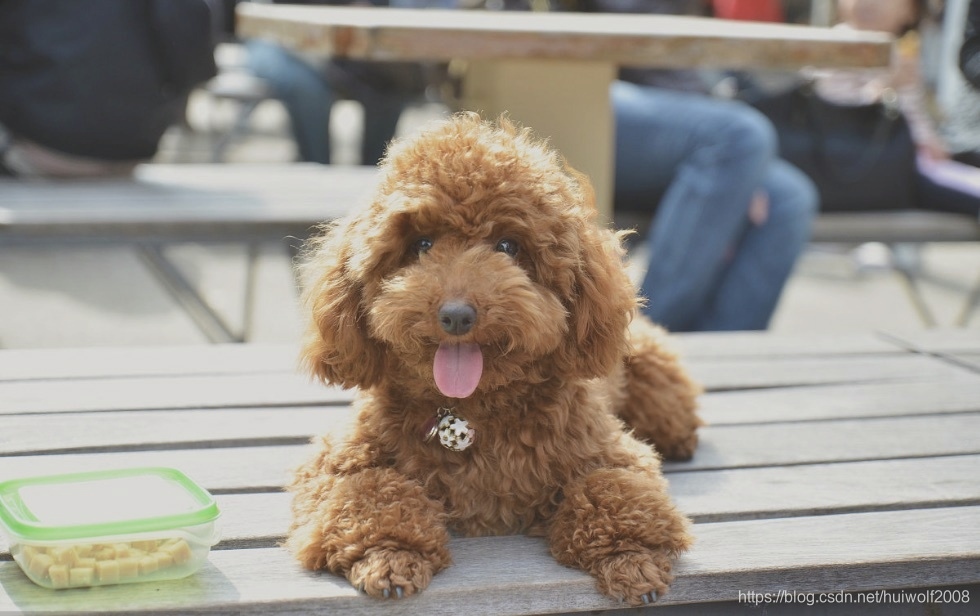
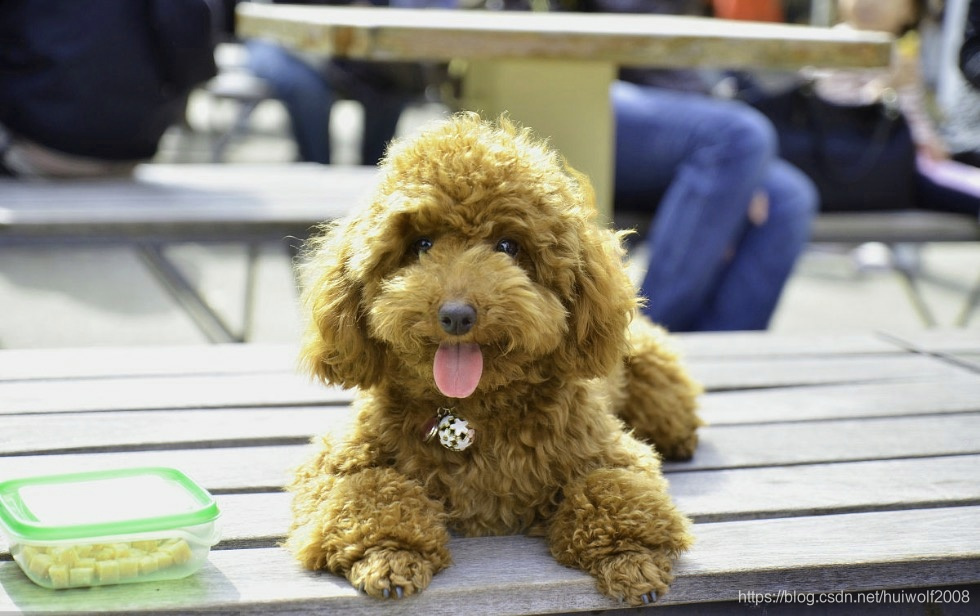
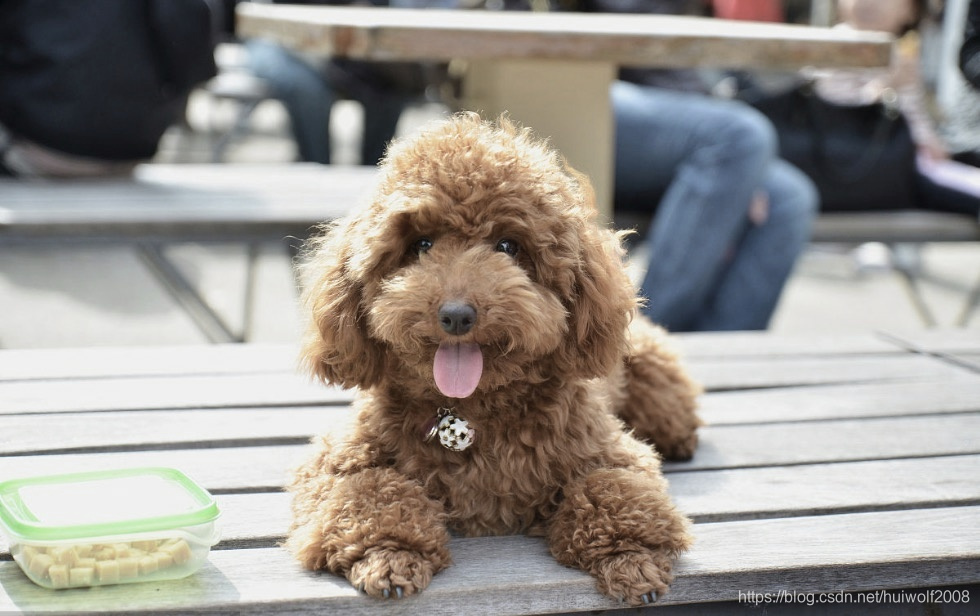
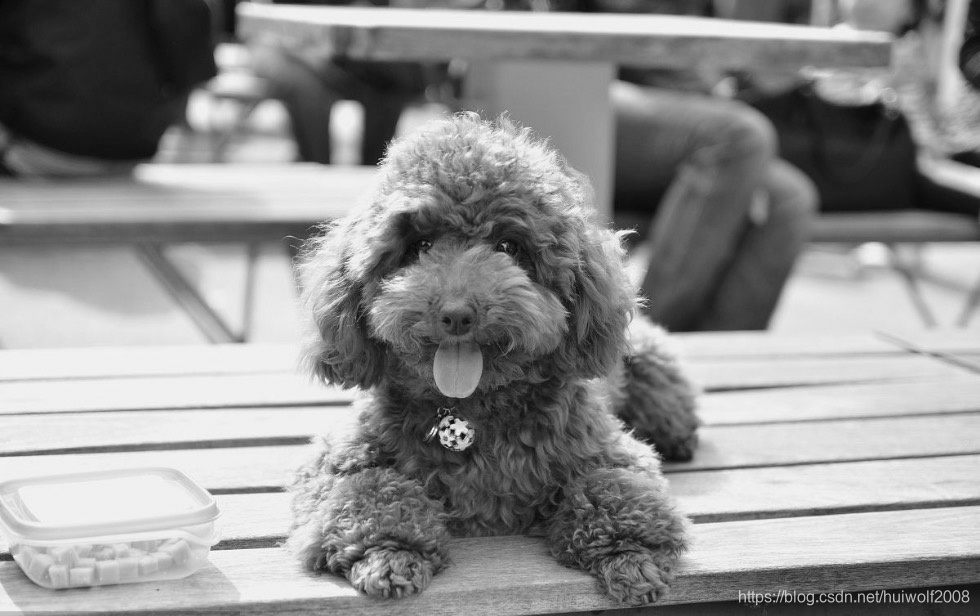
贴上完整代码如下,使用时可根据实际应用情况,调节各图片处理参数值,以达到理想效果。
import os
import filetype
import numpy as np
import tensorflow as tf
"""
python3 pic_processing.py --pic_path=photo/ --save_path=photo_result/ --base_num=100
"""
tf.app.flags.DEFINE_string(
'pic_path', '', 'The picture path.')
tf.app.flags.DEFINE_string(
'save_path', '', 'save picture path.')
tf.app.flags.DEFINE_integer(
'base_num', 0, 'Number of filename.')
FLAGS = tf.app.flags.FLAGS
#创建文件编号
def createFileNum(base_num):
i=[base_num]
def create():
i[0] = i[0]+1
return i[0]
return create
#保存文件
def save_pic(filename,img_data):
filename = FLAGS.save_path+str(filename) + '.jpg'
encoded_image = tf.image.encode_jpeg(img_data)
with tf.gfile.GFile(filename, 'wb') as f:
f.write(encoded_image.eval())
def main(__):
print("FLAGS.pic_path", FLAGS.pic_path)
fileName = createFileNum(FLAGS.base_num)
if not FLAGS.pic_path:
raise ValueError('You must supply the pic resource --pic_path')
return
if not FLAGS.save_path:
raise ValueError('You must supply the new file save path --save_path')
return
picfiles = os.listdir(FLAGS.pic_path)
with tf.Session() as sess:
i = 0
for pic in picfiles:
temp_path = os.path.join(FLAGS.pic_path,pic)
if not os.path.isdir(temp_path):
kind = filetype.guess(temp_path)
if not kind is None:
i += 1
print("( %d/%d ) %s" % (i, len(picfiles), temp_path))
# 使用start_queue_runners之后,才会开始填充队列
image_raw_data = tf.gfile.FastGFile(temp_path, 'rb').read()
img_data = tf.image.decode_jpeg(image_raw_data)
# 数据类型转换为实数方便程序对图像进行处理
img_data = tf.image.convert_image_dtype(img_data, dtype=tf.float32)
img_data = tf.image.convert_image_dtype(img_data, dtype=tf.uint8)
save_pic(fileName(),img_data)
# 左右翻转
flipped = tf.image.flip_left_right(img_data)
save_pic(fileName(), flipped)
#上下翻转
# flipped = tf.image.flip_up_down(img_data)
# save_pic(fileName(), flipped)
#调整图像的大小 双线性插值法
resized = tf.image.resize_images(img_data, [720, 1280], method=0)
resized = np.asarray(resized.eval(), dtype='uint8')
adjusted = tf.image.resize_image_with_crop_or_pad(resized,616 , 980)
save_pic(fileName(), adjusted)
# 在[-max_delta, max_delta)的范围随机调整图片的亮度。
adjusted = tf.image.random_brightness(img_data, max_delta=0.5)
save_pic(fileName(), adjusted)
# 在[lower, upper]的范围随机调整图的对比度。
adjusted = tf.image.random_contrast(img_data, 0.5, 1)
save_pic(fileName(), adjusted)
# 在[-max_delta, max_delta]的范围随机调整图片的色相。max_delta的取值在[0, 0.5]之间。
adjusted = tf.image.random_hue(img_data, 0.1)
save_pic(fileName(), adjusted)
# 在[lower, upper]的范围随机调整图的饱和度。
adjusted = tf.image.random_saturation(img_data, 0.0, 2.0)
save_pic(fileName(), adjusted)
#灰度图片
adjusted = tf.image.rgb_to_grayscale(img_data)
save_pic(fileName(), adjusted)
if __name__ == '__main__':
tf.app.run()
说明:该脚本对外提供了参数设置,运行时也加入了进度提示。使用时执行命令:
python3 pic_processing.py --pic_path=原始图片目录 --save_path=输出图片目录 --base_num=100文件编号
问题:该脚本为单线程,如果在服务器上处理,可改为多线程模式提高效率。
多线程处理时可采用tensorflow多线程方式,把处理和保存文件的操作放入各个线程去处理。
import os
import filetype
import numpy as np
import tensorflow as tf
import threading
tf.app.flags.DEFINE_string(
'pic_path', '', 'The picture path.')
tf.app.flags.DEFINE_string(
'save_path', '', 'save picture path.')
tf.app.flags.DEFINE_integer(
'base_num', 0, 'Number of filename.')
tf.app.flags.DEFINE_integer(
'thread_num', 10, 'Number of thread,A multiplier of 10.')
FLAGS = tf.app.flags.FLAGS
#创建文件编号
def createFileNum(base_num):
i=[base_num]
def create():
i[0] = i[0]+1
return i[0]
return create
#保存文件
def save_pic(filename,img_data,sess):
filename = FLAGS.save_path+filename + '.jpg'
encoded_image = tf.image.encode_jpeg(img_data)
with tf.gfile.GFile(filename, 'wb') as f:
f.write(encoded_image.eval(session=sess))
#多线程
class myThread(threading.Thread):
def __init__(self,name,filepath,filenum):
threading.Thread.__init__(self)
self.name = name
self.filepath = filepath
self.filenum = filenum
def run(self):
self.graph = tf.Graph() # 为每个类(实例)单独创建一个graph
self.sess = tf.Session(graph=self.graph) # 创建新的sess
with self.sess.as_default():
with self.graph.as_default():
image_raw_data = tf.gfile.FastGFile(self.filepath, 'rb').read()
img_data = tf.image.decode_jpeg(image_raw_data)
# 数据类型转换为实数方便程序对图像进行处理
img_data = tf.image.convert_image_dtype(img_data, dtype=tf.float32)
img_data = tf.image.convert_image_dtype(img_data, dtype=tf.uint8)
save_pic(str(self.filenum)+"_1", img_data, self.sess)
# 左右翻转
# flipped = tf.image.flip_left_right(img_data)
# save_pic(FLAGS.save_path + str(fileName()) + '.jpg', flipped)
# 调整图像的大小 双线性插值法
resized = tf.image.resize_images(img_data, [792, 1408], method=0)
resized = np.asarray(resized.eval(), dtype='uint8')
img_data = tf.image.resize_image_with_crop_or_pad(resized, 720, 1280)
save_pic(str(self.filenum)+"_2", img_data, self.sess)
# 在[-max_delta, max_delta)的范围随机调整图片的亮度。
img_data = tf.image.random_brightness(img_data, max_delta=0.1)
save_pic(str(self.filenum)+"_3", img_data, self.sess)
# 在[lower, upper]的范围随机调整图的对比度。
img_data = tf.image.random_contrast(img_data, 0.5, 1)
save_pic(str(self.filenum)+"_5", img_data, self.sess)
# 在[-max_delta, max_delta]的范围随机调整图片的色相。max_delta的取值在[0, 0.5]之间。
img_data = tf.image.random_hue(img_data, 0.3)
save_pic(str(self.filenum)+"_7", img_data, self.sess)
# 在[lower, upper]的范围随机调整图的饱和度。
img_data = tf.image.random_saturation(img_data, 0.0, 2.0)
save_pic(str(self.filenum)+"_8", img_data, self.sess)
# 灰度图片
img_data = tf.image.rgb_to_grayscale(img_data)
save_pic(str(self.filenum)+"_0", img_data, self.sess)
def main(__):
print("FLAGS.pic_path", FLAGS.pic_path)
fileName = createFileNum(FLAGS.base_num)
if not FLAGS.pic_path:
raise ValueError('You must supply the pic resource --pic_path')
return
if not FLAGS.save_path:
raise ValueError('You must supply the new file save path --save_path')
return
picfiles = os.listdir(FLAGS.pic_path)
with tf.Session() as sess:
index = 0
tf.local_variables_initializer().run()
threads = tf.train.start_queue_runners()
for pic in picfiles:
image_path = os.path.join(FLAGS.pic_path,pic)
index += 1
if not os.path.isdir(image_path):
kind = filetype.guess(image_path)
if not kind is None:
print("( %d/%d ) %s" % (index, len(picfiles), image_path))
# 使用start_queue_runners之后,才会开始填充队列
fileNum = fileName()
threads.append(myThread(index, image_path, fileNum))
if index%FLAGS.thread_num == 0 or index == len(picfiles):
for t in threads:
t.start()
t.join()
threads.clear()
if __name__ == '__main__':
tf.app.run()