刚刚刷了一下Qwen的Repo,发现又有新模型了。
喜欢开源是吧,就爱这样的你~~
这次是数学推理的过程奖励模型,共有3个,分别是,Qwen2.5-Math-7B-PRM800K、Qwen2.5-Math-PRM-7B和Qwen2.5-Math-PRM-72B。
其中,Qwen2.5-Math-7B-PRM800K是利用开源数据集PRM800K在 Qwen2.5-Math-7B-Instruct 进行微调得到的模型,Qwen2.5-Math-PRM-7B和Qwen2.5-Math-PRM-72B是利用自己数据训练的模型。
效果不用说了,在ProcessBench中表现出更强的错误识别性能,远超之前开源的PRM模型。
HF Model: https://huggingface.co/Qwen/Qwen2.5-Math-7B-PRM800K https://huggingface.co/Qwen/Qwen2.5-Math-PRM-7B https://huggingface.co/Qwen/Qwen2.5-Math-PRM-72B
在进行PRM使用的时候请注意:
-
建议使用双换行符(“\n\n”)来分隔解决方案中的各个步骤。
-
在每一步之后,插入一个特殊的标记“ <extra_0> ”。对于奖励计算,是通过提取该令牌被分类为正的概率分数,从而得到介于 0 和 1 之间的奖励值。
快速使用:
import torch from transformers import AutoModel, AutoTokenizer import torch.nn.functional as F def make_step_rewards(logits, token_masks): probabilities = F.softmax(logits, dim=-1) probabilities = probabilities * token_masks.unsqueeze(-1) # bs, seq_len, num_labels all_scores_res = [] for i in range(probabilities.size(0)): sample = probabilities[i] # seq_len, num_labels positive_probs = sample[sample != 0].view(-1, 2)[:, 1] # valid_tokens, num_labels non_zero_elements_list = positive_probs.cpu().tolist() all_scores_res.append(non_zero_elements_list) return all_scores_res model_name = "Qwen/Qwen2.5-Math-PRM-72B" device = "auto" tokenizer = AutoTokenizer.from_pretrained(model_name, trust_remote_code=True) model = AutoModel.from_pretrained( model_name, device_map=device, torch_dtype=torch.bfloat16, trust_remote_code=True, ).eval() data = { "system": "Please reason step by step, and put your final answer within \boxed{}.", "query": "Sue lives in a fun neighborhood. One weekend, the neighbors decided to play a prank on Sue. On Friday morning, the neighbors placed 18 pink plastic flamingos out on Sue's front yard. On Saturday morning, the neighbors took back one third of the flamingos, painted them white, and put these newly painted white flamingos back out on Sue's front yard. Then, on Sunday morning, they added another 18 pink plastic flamingos to the collection. At noon on Sunday, how many more pink plastic flamingos were out than white plastic flamingos?", "response": [ "To find out how many more pink plastic flamingos were out than white plastic flamingos at noon on Sunday, we can break down the problem into steps. First, on Friday, the neighbors start with 18 pink plastic flamingos.", "On Saturday, they take back one third of the flamingos. Since there were 18 flamingos, (1/3 \times 18 = 6) flamingos are taken back. So, they have (18 - 6 = 12) flamingos left in their possession. Then, they paint these 6 flamingos white and put them back out on Sue's front yard. Now, Sue has the original 12 pink flamingos plus the 6 new white ones. Thus, by the end of Saturday, Sue has (12 + 6 = 18) pink flamingos and 6 white flamingos.", "On Sunday, the neighbors add another 18 pink plastic flamingos to Sue's front yard. By the end of Sunday morning, Sue has (18 + 18 = 36) pink flamingos and still 6 white flamingos.", "To find the difference, subtract the number of white flamingos from the number of pink flamingos: (36 - 6 = 30). Therefore, at noon on Sunday, there were 30 more pink plastic flamingos out than white plastic flamingos. The answer is (\boxed{30})." ] } messages = [ {"role": "system", "content": data['system']}, {"role": "user", "content": data['query']}, {"role": "assistant", "content": "<extra_0>".join(data['response']) + "<extra_0>"}, ] conversation_str = tokenizer.apply_chat_template( messages, tokenize=False, add_generation_prompt=False ) input_ids = tokenizer.encode( conversation_str, return_tensors="pt", ).to(model.device) outputs = model(input_ids=input_ids) step_sep_id = tokenizer.encode("<extra_0>")[0] token_masks = (input_ids == step_sep_id) step_reward = make_step_rewards(outputs[0], token_masks) print(step_reward) # [[0.9921875, 0.0047607421875, 0.32421875, 0.8203125]]
AI大模型学习路线
如果你对AI大模型入门感兴趣,那么你需要的话可以点击这里大模型重磅福利:入门进阶全套104G学习资源包免费分享!
扫描下方csdn官方合作二维码获取哦!
这是一份大模型从零基础到进阶的学习路线大纲全览,小伙伴们记得点个收藏!
第一阶段: 从大模型系统设计入手,讲解大模型的主要方法;
第二阶段: 在通过大模型提示词工程从Prompts角度入手更好发挥模型的作用;
第三阶段: 大模型平台应用开发借助阿里云PAI平台构建电商领域虚拟试衣系统;
第四阶段: 大模型知识库应用开发以LangChain框架为例,构建物流行业咨询智能问答系统;
第五阶段: 大模型微调开发借助以大健康、新零售、新媒体领域构建适合当前领域大模型;
第六阶段: 以SD多模态大模型为主,搭建了文生图小程序案例;
第七阶段: 以大模型平台应用与开发为主,通过星火大模型,文心大模型等成熟大模型构建大模型行业应用。
100套AI大模型商业化落地方案
大模型全套视频教程
200本大模型PDF书籍
👉学会后的收获:👈
• 基于大模型全栈工程实现(前端、后端、产品经理、设计、数据分析等),通过这门课可获得不同能力;
• 能够利用大模型解决相关实际项目需求: 大数据时代,越来越多的企业和机构需要处理海量数据,利用大模型技术可以更好地处理这些数据,提高数据分析和决策的准确性。因此,掌握大模型应用开发技能,可以让程序员更好地应对实际项目需求;
• 基于大模型和企业数据AI应用开发,实现大模型理论、掌握GPU算力、硬件、LangChain开发框架和项目实战技能, 学会Fine-tuning垂直训练大模型(数据准备、数据蒸馏、大模型部署)一站式掌握;
• 能够完成时下热门大模型垂直领域模型训练能力,提高程序员的编码能力: 大模型应用开发需要掌握机器学习算法、深度学习框架等技术,这些技术的掌握可以提高程序员的编码能力和分析能力,让程序员更加熟练地编写高质量的代码。
LLM面试题合集
大模型产品经理资源合集
大模型项目实战合集
👉获取方式:
😝有需要的小伙伴,可以保存图片到wx扫描二v码免费领取【保证100%免费】🆓
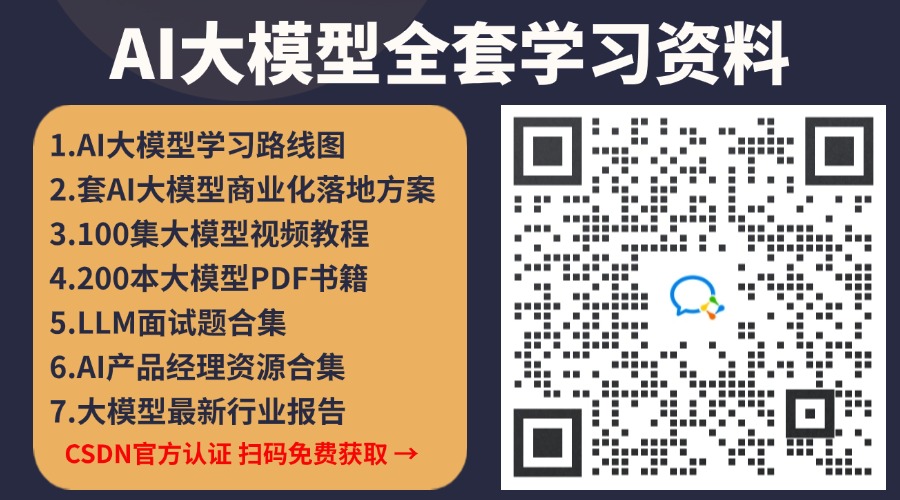