★★★ 本文源自AlStudio社区精品项目,【点击此处】查看更多精品内容 >>>
基于PaddleSeg的钢筋长度超限监控
1. 项目介绍
钢铁厂在生产钢筋的过程中,会存在部分钢筋长度超限的问题,如果不进行处理,容易造成机械臂损伤。因此,需要通过质检流程,筛选出存在长度超限问题的钢筋批次,并进行预警。传统的处理方式是人工核查,一方面增加了人工成本,拉低了生产效率,另一方面也要求工人师傅对业务比较熟练,能够准确地判断钢筋长度是否超限,存在一定的误判率。在AI时代,利用深度学习技术,可以实现端到端全自动的钢筋长度超限监控,从而降低人工成本,提高生产效率。整体技术方案可以归纳为如下步骤:
- 在钢筋一侧安装摄像头,拍摄图像;
- 利用图像分割技术提取钢筋掩膜;
- 根据摄像头位置和角度确定长度界限;
- 最后根据该长度界限和钢筋分割范围的几何关系判断本批次钢筋是否超限。
1.1 痛点问题
钢筋长度超限监控问题,传统质检方式需要专人介入,自动化程度低,且存在一定的误判率,影响钢筋生产的效率和质量。该业务场景背景复杂、钢筋数量、形状、超限程度不一,导致利用模型进行图像分割难度较大。而钢筋超限会导致机器损伤,对于超限情况需要及时响应,避免损伤发生,因此对模型精度与速度要求非常高。
1.2 解决方案
钢筋超限监控问题可以转换为图像分割后的几何判断问题,而为了实现图像分割,我们使用提供了全流程分割方案的PaddleSeg
开发套件,只需简单地修改配置文件,就可以进行模型训练,获得高精度的分割效果。进一步地,我们挑选使用精度和速度平衡的PP-LiteSeg
,实现高精度的同时,满足工业部署的要求。
1.3 模型简介
轻量级语义分割模型 PP-LiteSeg
,包含以下几个关键性模块改进:
- FLD(Flexible and Lightweight Decoder),是一种灵活且轻量级的解码器,用于降低传统解码器模块的计算负载;
- UAFM(Unified Attention Fusion Module),是一个统一注意力融合模块,采用空域与通道注意力生成用于特征融合的权值;
- SPPM(Simple Pyramid Pooling Module),是一个简单的金字塔池化模块,以极低的计算消耗进行全局上下文信息聚合。
相比其他方案,PP-LiteSeg
取得了精度与速度之间的平衡,其结构如下:
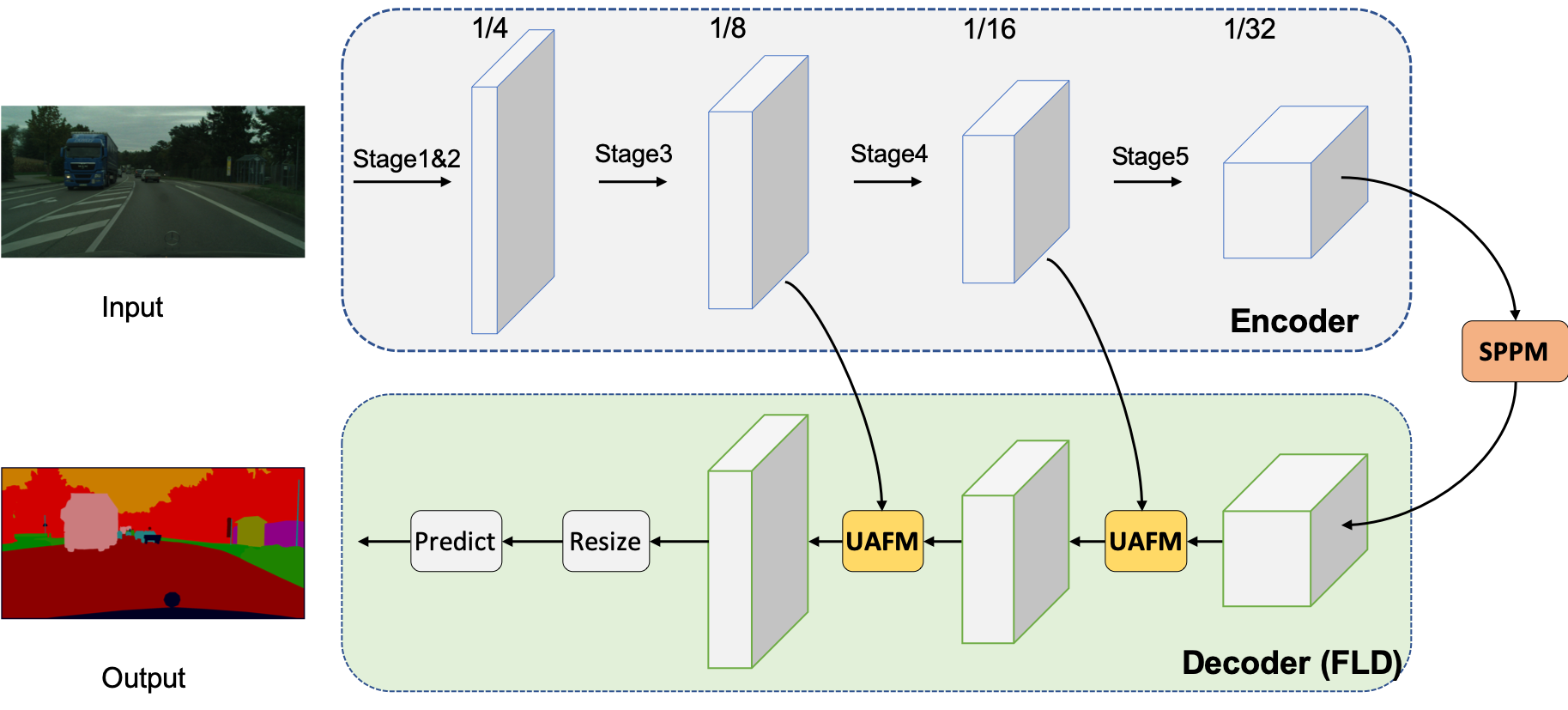
2. 安装环境
- Python >= 3.6
- PaddlePaddle >= 2.1
- PaddleSeg
# 将 PaddleSeg git clone 到本地
%cd /home/aistudio/work
# !git clone https://github.com/PaddlePaddle/PaddleSeg
# 由于github国内访问较慢,因此,也可以使用gitee
# !git clone https://gitee.com/PaddlePaddle/PaddleSeg
# checkout到v2.6
# !git checkout release/2.6
!git clone --branch release/2.6 --depth 1 https://gitee.com/PaddlePaddle/PaddleSeg.git # 设置depth为1,可以免去下载无用的历史版本记录,加快clone速度
# 切换到PaddleSeg目录
%cd /home/aistudio/work/PaddleSeg
# 安装项目所需的依赖
# !pip install -U pip wheel
!pip install -r requirements.txt
3. 处理数据
# 查看当前挂载的数据集目录,并解压。
%cd /home/aistudio/data/
!tar xf data196420/dataset.tar.xz
# 切换到工作目录
%cd /home/aistudio/work/PaddleSeg
/home/aistudio/work/PaddleSeg
可借助PaddleSeg
中tools
目录下的脚本labelme2seg.py
,将labelme
格式标注转换成paddleseg
支持的格式。
# 运行`labelme2seg.py`
!python /home/aistudio/work/PaddleSeg/tools/labelme2seg.py ~/data/dataset
使用PaddleSeg
提供的脚本(split_dataset_list.py
)将数据集划分为训练集、验证集和测试集。
!python tools/split_dataset_list.py ~/data/dataset . annotations --split 0.7 0.15 0.15
4. 模型训练
此处我们选择轻量级语义分割模型PP-LiteSeg
模型,对钢筋进行分割。具体介绍可参考PP-LiteSeg
的README说明文件。为了在自定义数据集上使用PP-LiteSeg
模型,需要对PaddleSeg
提供的默认配置文件(PaddleSeg/configs/pp_liteseg/pp_liteseg_stdc1_cityscapes_1024x512_scale0.5_160k.yml
)进行轻微修改。
如下所示,添加自定义数据集路径、类别数等信息。
batch_size: 4 # total: 4*4
iters: 2000
optimizer:
type: sgd
momentum: 0.9
weight_decay: 5.0e-4
lr_scheduler:
type: PolynomialDecay
end_lr: 0
power: 0.9
warmup_iters: 100
warmup_start_lr: 1.0e-5
learning_rate: 0.005
loss:
types:
- type: OhemCrossEntropyLoss
min_kept: 130000 # batch_size * 1024 * 512 // 16
- type: OhemCrossEntropyLoss
min_kept: 130000
- type: OhemCrossEntropyLoss
min_kept: 130000
coef: [1, 1, 1]
train_dataset:
type: Dataset
dataset_root: /home/aistudio/data/dataset
train_path: /home/aistudio/data/dataset/train.txt
num_classes: 2
transforms:
- type: ResizeStepScaling
min_scale_factor: 0.125
max_scale_factor: 1.5
scale_step_size: 0.125
- type: RandomPaddingCrop
crop_size: [1024, 512]
- type: RandomHorizontalFlip
- type: RandomDistort
brightness_range: 0.5
contrast_range: 0.5
saturation_range: 0.5
- type: Normalize
mode: train
val_dataset:
type: Dataset
dataset_root: /home/aistudio/data/dataset
val_path: /home/aistudio/data/dataset/val.txt
num_classes: 2
transforms:
- type: Normalize
mode: val
test_config:
aug_eval: True
scales: 0.5
model:
type: PPLiteSeg
backbone:
type: STDC1
pretrained: https://bj.bcebos.com/paddleseg/dygraph/PP_STDCNet1.tar.gz
arm_out_chs: [32, 64, 128]
seg_head_inter_chs: [32, 64, 64]
!python3 train.py --config /home/aistudio/work/pp_liteseg_stdc1.yml \
--use_vdl \
--save_dir output/mask_iron \
--save_interval 500 \
--log_iters 100 \
--num_workers 8 \
--do_eval \
--keep_checkpoint_max 10
/home/aistudio/work/PaddleSeg/paddleseg/models/losses/rmi_loss.py:78: DeprecationWarning: invalid escape sequence \i
"""
2023-03-06 11:12:52 [INFO]
------------Environment Information-------------
platform: Linux-4.15.0-140-generic-x86_64-with-debian-stretch-sid
Python: 3.7.4 (default, Aug 13 2019, 20:35:49) [GCC 7.3.0]
Paddle compiled with cuda: True
NVCC: Build cuda_11.2.r11.2/compiler.29618528_0
cudnn: 8.2
GPUs used: 1
CUDA_VISIBLE_DEVICES: None
GPU: ['GPU 0: Tesla V100-SXM2-32GB']
GCC: gcc (Ubuntu 7.5.0-3ubuntu1~16.04) 7.5.0
PaddleSeg: 2.6.0
PaddlePaddle: 2.4.0
OpenCV: 4.6.0
------------------------------------------------
2023-03-06 11:12:53 [INFO]
---------------Config Information---------------
batch_size: 4
iters: 2000
loss:
coef:
- 1
- 1
- 1
types:
- ignore_index: 255
min_kept: 130000
type: OhemCrossEntropyLoss
- ignore_index: 255
min_kept: 130000
type: OhemCrossEntropyLoss
- ignore_index: 255
min_kept: 130000
type: OhemCrossEntropyLoss
lr_scheduler:
end_lr: 0
learning_rate: 0.005
power: 0.9
type: PolynomialDecay
warmup_iters: 100
warmup_start_lr: 1.0e-05
model:
arm_out_chs:
- 32
- 64
- 128
backbone:
pretrained: https://bj.bcebos.com/paddleseg/dygraph/PP_STDCNet1.tar.gz
type: STDC1
seg_head_inter_chs:
- 32
- 64
- 64
type: PPLiteSeg
optimizer:
momentum: 0.9
type: sgd
weight_decay: 0.0005
test_config:
aug_eval: true
scales: 0.5
train_dataset:
dataset_root: /home/aistudio/data/dataset
mode: train
num_classes: 2
train_path: /home/aistudio/data/dataset/train.txt
transforms:
- max_scale_factor: 1.5
min_scale_factor: 0.125
scale_step_size: 0.125
type: ResizeStepScaling
- crop_size:
- 1024
- 512
type: RandomPaddingCrop
- type: RandomHorizontalFlip
- brightness_range: 0.5
contrast_range: 0.5
saturation_range: 0.5
type: RandomDistort
- type: Normalize
type: Dataset
val_dataset:
dataset_root: /home/aistudio/data/dataset
mode: val
num_classes: 2
transforms:
- type: Normalize
type: Dataset
val_path: /home/aistudio/data/dataset/val.txt
------------------------------------------------
W0306 11:12:53.040948 2444 gpu_resources.cc:61] Please NOTE: device: 0, GPU Compute Capability: 7.0, Driver API Version: 11.2, Runtime API Version: 11.2
W0306 11:12:53.041000 2444 gpu_resources.cc:91] device: 0, cuDNN Version: 8.2.
2023-03-06 11:12:54 [INFO] Loading pretrained model from https://bj.bcebos.com/paddleseg/dygraph/PP_STDCNet1.tar.gz
Connecting to https://bj.bcebos.com/paddleseg/dygraph/PP_STDCNet1.tar.gz
Downloading PP_STDCNet1.tar.gz
[==================================================] 100.00%
Uncompress PP_STDCNet1.tar.gz
[==================================================] 100.00%
2023-03-06 11:13:00 [INFO] There are 145/145 variables loaded into STDCNet.
Corrupt JPEG data: 2 extraneous bytes before marker 0xd9
Corrupt JPEG data: 2 extraneous bytes before marker 0xd9
/opt/conda/envs/python35-paddle120-env/lib/python3.7/site-packages/paddle/nn/layer/norm.py:712: UserWarning: When training, we now always track global mean and variance.
"When training, we now always track global mean and variance."
/opt/conda/envs/python35-paddle120-env/lib/python3.7/site-packages/paddle/fluid/dygraph/math_op_patch.py:277: UserWarning: The dtype of left and right variables are not the same, left dtype is paddle.float32, but right dtype is paddle.int64, the right dtype will convert to paddle.float32
.format(lhs_dtype, rhs_dtype, lhs_dtype))
Corrupt JPEG data: 2 extraneous bytes before marker 0xd9
Corrupt JPEG data: 2 extraneous bytes before marker 0xd9
Corrupt JPEG data: 2 extraneous bytes before marker 0xd9
Corrupt JPEG data: 2 extraneous bytes before marker 0xd9
2023-03-06 11:13:25 [INFO] [TRAIN] epoch: 3, iter: 100/2000, loss: 2.1926, lr: 0.004950, batch_cost: 0.2491, reader_cost: 0.06910, ips: 16.0550 samples/sec | ETA 00:07:53
Corrupt JPEG data: 2 extraneous bytes before marker 0xd9
Corrupt JPEG data: 2 extraneous bytes before marker 0xd9
Corrupt JPEG data: 2 extraneous bytes before marker 0xd9
Corrupt JPEG data: 2 extraneous bytes before marker 0xd9
Corrupt JPEG data: 2 extraneous bytes before marker 0xd9
2023-03-06 11:13:49 [INFO] [TRAIN] epoch: 6, iter: 200/2000, loss: 1.8833, lr: 0.004765, batch_cost: 0.2382, reader_cost: 0.08182, ips: 16.7906 samples/sec | ETA 00:07:08
Corrupt JPEG data: 2 extraneous bytes before marker 0xd9
Corrupt JPEG data: 2 extraneous bytes before marker 0xd9
Corrupt JPEG data: 2 extraneous bytes before marker 0xd9
Corrupt JPEG data: 2 extraneous bytes before marker 0xd9
Corrupt JPEG data: 2 extraneous bytes before marker 0xd9
2023-03-06 11:14:11 [INFO] [TRAIN] epoch: 9, iter: 300/2000, loss: 1.7562, lr: 0.004526, batch_cost: 0.2207, reader_cost: 0.06289, ips: 18.1201 samples/sec | ETA 00:06:15
Corrupt JPEG data: 2 extraneous bytes before marker 0xd9
Corrupt JPEG data: 2 extraneous bytes before marker 0xd9
Corrupt JPEG data: 2 extraneous bytes before marker 0xd9
Corrupt JPEG data: 2 extraneous bytes before marker 0xd9
Corrupt JPEG data: 2 extraneous bytes before marker 0xd9
Corrupt JPEG data: 2 extraneous bytes before marker 0xd9
2023-03-06 11:14:34 [INFO] [TRAIN] epoch: 11, iter: 400/2000, loss: 1.5520, lr: 0.004286, batch_cost: 0.2253, reader_cost: 0.03780, ips: 17.7563 samples/sec | ETA 00:06:00
Corrupt JPEG data: 2 extraneous bytes before marker 0xd9
Corrupt JPEG data: 2 extraneous bytes before marker 0xd9
Corrupt JPEG data: 2 extraneous bytes before marker 0xd9
Corrupt JPEG data: 2 extraneous bytes before marker 0xd9
2023-03-06 11:14:58 [INFO] [TRAIN] epoch: 14, iter: 500/2000, loss: 1.6580, lr: 0.004044, batch_cost: 0.2409, reader_cost: 0.06312, ips: 16.6059 samples/sec | ETA 00:06:01
2023-03-06 11:14:58 [INFO] Start evaluating (total_samples: 32, total_iters: 32)...
3/32 [=>............................] - ETA: 14s - batch_cost: 0.5002 - reader cost: 0.4586Corrupt JPEG data: 2 extraneous bytes before marker 0xd9
32/32 [==============================] - 7s 231ms/step - batch_cost: 0.2309 - reader cost: 0.2074
2023-03-06 11:15:05 [INFO] [EVAL] #Images: 32 mIoU: 0.9828 Acc: 0.9936 Kappa: 0.9826 Dice: 0.9913
2023-03-06 11:15:05 [INFO] [EVAL] Class IoU:
[0.9915 0.9742]
2023-03-06 11:15:05 [INFO] [EVAL] Class Precision:
[0.9957 0.987 ]
2023-03-06 11:15:05 [INFO] [EVAL] Class Recall:
[0.9958 0.9868]
2023-03-06 11:15:05 [INFO] [EVAL] The model with the best validation mIoU (0.9828) was saved at iter 500.
Corrupt JPEG data: 2 extraneous bytes before marker 0xd9
Corrupt JPEG data: 2 extraneous bytes before marker 0xd9
Corrupt JPEG data: 2 extraneous bytes before marker 0xd9
Corrupt JPEG data: 2 extraneous bytes before marker 0xd9
Corrupt JPEG data: 2 extraneous bytes before marker 0xd9
Corrupt JPEG data: 2 extraneous bytes before marker 0xd9
Corrupt JPEG data: 2 extraneous bytes before marker 0xd9
2023-03-06 11:15:27 [INFO] [TRAIN] epoch: 17, iter: 600/2000, loss: 1.1448, lr: 0.003801, batch_cost: 0.2184, reader_cost: 0.05941, ips: 18.3150 samples/sec | ETA 00:05:05
Corrupt JPEG data: 2 extraneous bytes before marker 0xd9
Corrupt JPEG data: 2 extraneous bytes before marker 0xd9
Corrupt JPEG data: 2 extraneous bytes before marker 0xd9
Corrupt JPEG data: 2 extraneous bytes before marker 0xd9
Corrupt JPEG data: 2 extraneous bytes before marker 0xd9
2023-03-06 11:15:49 [INFO] [TRAIN] epoch: 19, iter: 700/2000, loss: 1.0914, lr: 0.003556, batch_cost: 0.2136, reader_cost: 0.04413, ips: 18.7289 samples/sec | ETA 00:04:37
Corrupt JPEG data: 2 extraneous bytes before marker 0xd9
Corrupt JPEG data: 2 extraneous bytes before marker 0xd9
Corrupt JPEG data: 2 extraneous bytes before marker 0xd9
Corrupt JPEG data: 2 extraneous bytes before marker 0xd9
Corrupt JPEG data: 2 extraneous bytes before marker 0xd9
Corrupt JPEG data: 2 extraneous bytes before marker 0xd9
2023-03-06 11:16:13 [INFO] [TRAIN] epoch: 22, iter: 800/2000, loss: 1.3022, lr: 0.003309, batch_cost: 0.2464, reader_cost: 0.06541, ips: 16.2332 samples/sec | ETA 00:04:55
Corrupt JPEG data: 2 extraneous bytes before marker 0xd9
Corrupt JPEG data: 2 extraneous bytes before marker 0xd9
Corrupt JPEG data: 2 extraneous bytes before marker 0xd9
Corrupt JPEG data: 2 extraneous bytes before marker 0xd9
Corrupt JPEG data: 2 extraneous bytes before marker 0xd9
Corrupt JPEG data: 2 extraneous bytes before marker 0xd9
2023-03-06 11:16:36 [INFO] [TRAIN] epoch: 25, iter: 900/2000, loss: 1.0730, lr: 0.003060, batch_cost: 0.2270, reader_cost: 0.06602, ips: 17.6230 samples/sec | ETA 00:04:09
Corrupt JPEG data: 2 extraneous bytes before marker 0xd9
Corrupt JPEG data: 2 extraneous bytes before marker 0xd9
Corrupt JPEG data: 2 extraneous bytes before marker 0xd9
Corrupt JPEG data: 2 extraneous bytes before marker 0xd9
2023-03-06 11:16:59 [INFO] [TRAIN] epoch: 28, iter: 1000/2000, loss: 1.3344, lr: 0.002809, batch_cost: 0.2290, reader_cost: 0.05981, ips: 17.4704 samples/sec | ETA 00:03:48
2023-03-06 11:16:59 [INFO] Start evaluating (total_samples: 32, total_iters: 32)...
3/32 [=>............................] - ETA: 18s - batch_cost: 0.6236 - reader cost: 0.5855Corrupt JPEG data: 2 extraneous bytes before marker 0xd9
32/32 [==============================] - 8s 243ms/step - batch_cost: 0.2428 - reader cost: 0.2198
2023-03-06 11:17:07 [INFO] [EVAL] #Images: 32 mIoU: 0.9767 Acc: 0.9912 Kappa: 0.9763 Dice: 0.9882
2023-03-06 11:17:07 [INFO] [EVAL] Class IoU:
[0.9883 0.965 ]
2023-03-06 11:17:07 [INFO] [EVAL] Class Precision:
[0.9969 0.9739]
2023-03-06 11:17:07 [INFO] [EVAL] Class Recall:
[0.9913 0.9906]
2023-03-06 11:17:07 [INFO] [EVAL] The model with the best validation mIoU (0.9828) was saved at iter 500.
Corrupt JPEG data: 2 extraneous bytes before marker 0xd9
Corrupt JPEG data: 2 extraneous bytes before marker 0xd9
Corrupt JPEG data: 2 extraneous bytes before marker 0xd9
Corrupt JPEG data: 2 extraneous bytes before marker 0xd9
Corrupt JPEG data: 2 extraneous bytes before marker 0xd9
Corrupt JPEG data: 2 extraneous bytes before marker 0xd9
2023-03-06 11:17:27 [INFO] [TRAIN] epoch: 30, iter: 1100/2000, loss: 1.1206, lr: 0.002555, batch_cost: 0.2058, reader_cost: 0.04158, ips: 19.4394 samples/sec | ETA 00:03:05
Corrupt JPEG data: 2 extraneous bytes before marker 0xd9
Corrupt JPEG data: 2 extraneous bytes before marker 0xd9
Corrupt JPEG data: 2 extraneous bytes before marker 0xd9
Corrupt JPEG data: 2 extraneous bytes before marker 0xd9
Corrupt JPEG data: 2 extraneous bytes before marker 0xd9
2023-03-06 11:17:51 [INFO] [TRAIN] epoch: 33, iter: 1200/2000, loss: 0.9999, lr: 0.002298, batch_cost: 0.2337, reader_cost: 0.06408, ips: 17.1125 samples/sec | ETA 00:03:06
Corrupt JPEG data: 2 extraneous bytes before marker 0xd9
Corrupt JPEG data: 2 extraneous bytes before marker 0xd9
Corrupt JPEG data: 2 extraneous bytes before marker 0xd9
Corrupt JPEG data: 2 extraneous bytes before marker 0xd9
2023-03-06 11:18:14 [INFO] [TRAIN] epoch: 36, iter: 1300/2000, loss: 0.8688, lr: 0.002038, batch_cost: 0.2311, reader_cost: 0.06141, ips: 17.3074 samples/sec | ETA 00:02:41
Corrupt JPEG data: 2 extraneous bytes before marker 0xd9
Corrupt JPEG data: 2 extraneous bytes before marker 0xd9
Corrupt JPEG data: 2 extraneous bytes before marker 0xd9
Corrupt JPEG data: 2 extraneous bytes before marker 0xd9
Corrupt JPEG data: 2 extraneous bytes before marker 0xd9
Corrupt JPEG data: 2 extraneous bytes before marker 0xd9
2023-03-06 11:18:35 [INFO] [TRAIN] epoch: 38, iter: 1400/2000, loss: 0.8655, lr: 0.001775, batch_cost: 0.2123, reader_cost: 0.04386, ips: 18.8383 samples/sec | ETA 00:02:07
Corrupt JPEG data: 2 extraneous bytes before marker 0xd9
Corrupt JPEG data: 2 extraneous bytes before marker 0xd9
Corrupt JPEG data: 2 extraneous bytes before marker 0xd9
Corrupt JPEG data: 2 extraneous bytes before marker 0xd9
Corrupt JPEG data: 2 extraneous bytes before marker 0xd9
Corrupt JPEG data: 2 extraneous bytes before marker 0xd9
2023-03-06 11:18:59 [INFO] [TRAIN] epoch: 41, iter: 1500/2000, loss: 0.8779, lr: 0.001506, batch_cost: 0.2428, reader_cost: 0.07047, ips: 16.4764 samples/sec | ETA 00:02:01
2023-03-06 11:18:59 [INFO] Start evaluating (total_samples: 32, total_iters: 32)...
3/32 [=>............................] - ETA: 17s - batch_cost: 0.6126 - reader cost: 0.5622Corrupt JPEG data: 2 extraneous bytes before marker 0xd9
32/32 [==============================] - 8s 243ms/step - batch_cost: 0.2428 - reader cost: 0.2171
2023-03-06 11:19:07 [INFO] [EVAL] #Images: 32 mIoU: 0.9858 Acc: 0.9947 Kappa: 0.9857 Dice: 0.9928
2023-03-06 11:19:07 [INFO] [EVAL] Class IoU:
[0.993 0.9787]
2023-03-06 11:19:07 [INFO] [EVAL] Class Precision:
[0.9969 0.9878]
2023-03-06 11:19:07 [INFO] [EVAL] Class Recall:
[0.996 0.9906]
2023-03-06 11:19:08 [INFO] [EVAL] The model with the best validation mIoU (0.9858) was saved at iter 1500.
Corrupt JPEG data: 2 extraneous bytes before marker 0xd9
Corrupt JPEG data: 2 extraneous bytes before marker 0xd9
Corrupt JPEG data: 2 extraneous bytes before marker 0xd9
Corrupt JPEG data: 2 extraneous bytes before marker 0xd9
Corrupt JPEG data: 2 extraneous bytes before marker 0xd9
2023-03-06 11:19:31 [INFO] [TRAIN] epoch: 44, iter: 1600/2000, loss: 0.7487, lr: 0.001233, batch_cost: 0.2284, reader_cost: 0.06946, ips: 17.5115 samples/sec | ETA 00:01:31
Corrupt JPEG data: 2 extraneous bytes before marker 0xd9
Corrupt JPEG data: 2 extraneous bytes before marker 0xd9
Corrupt JPEG data: 2 extraneous bytes before marker 0xd9
Corrupt JPEG data: 2 extraneous bytes before marker 0xd9
Corrupt JPEG data: 2 extraneous bytes before marker 0xd9
2023-03-06 11:19:52 [INFO] [TRAIN] epoch: 46, iter: 1700/2000, loss: 0.7271, lr: 0.000952, batch_cost: 0.2108, reader_cost: 0.04096, ips: 18.9710 samples/sec | ETA 00:01:03
Corrupt JPEG data: 2 extraneous bytes before marker 0xd9
Corrupt JPEG data: 2 extraneous bytes before marker 0xd9
Corrupt JPEG data: 2 extraneous bytes before marker 0xd9
Corrupt JPEG data: 2 extraneous bytes before marker 0xd9
Corrupt JPEG data: 2 extraneous bytes before marker 0xd9
Corrupt JPEG data: 2 extraneous bytes before marker 0xd9
2023-03-06 11:20:16 [INFO] [TRAIN] epoch: 49, iter: 1800/2000, loss: 0.7356, lr: 0.000662, batch_cost: 0.2409, reader_cost: 0.06832, ips: 16.6025 samples/sec | ETA 00:00:48
Corrupt JPEG data: 2 extraneous bytes before marker 0xd9
Corrupt JPEG data: 2 extraneous bytes before marker 0xd9
Corrupt JPEG data: 2 extraneous bytes before marker 0xd9
Corrupt JPEG data: 2 extraneous bytes before marker 0xd9
Corrupt JPEG data: 2 extraneous bytes before marker 0xd9
2023-03-06 11:20:39 [INFO] [TRAIN] epoch: 52, iter: 1900/2000, loss: 0.6789, lr: 0.000356, batch_cost: 0.2301, reader_cost: 0.05793, ips: 17.3814 samples/sec | ETA 00:00:23
Corrupt JPEG data: 2 extraneous bytes before marker 0xd9
Corrupt JPEG data: 2 extraneous bytes before marker 0xd9
Corrupt JPEG data: 2 extraneous bytes before marker 0xd9
Corrupt JPEG data: 2 extraneous bytes before marker 0xd9
2023-03-06 11:21:02 [INFO] [TRAIN] epoch: 55, iter: 2000/2000, loss: 0.6566, lr: 0.000006, batch_cost: 0.2289, reader_cost: 0.06389, ips: 17.4774 samples/sec | ETA 00:00:00
2023-03-06 11:21:02 [INFO] Start evaluating (total_samples: 32, total_iters: 32)...
3/32 [=>............................] - ETA: 16s - batch_cost: 0.5517 - reader cost: 0.5198Corrupt JPEG data: 2 extraneous bytes before marker 0xd9
32/32 [==============================] - 8s 238ms/step - batch_cost: 0.2374 - reader cost: 0.2141
2023-03-06 11:21:09 [INFO] [EVAL] #Images: 32 mIoU: 0.9847 Acc: 0.9943 Kappa: 0.9846 Dice: 0.9923
2023-03-06 11:21:09 [INFO] [EVAL] Class IoU:
[0.9924 0.9771]
2023-03-06 11:21:09 [INFO] [EVAL] Class Precision:
[0.9967 0.9869]
2023-03-06 11:21:09 [INFO] [EVAL] Class Recall:
[0.9957 0.9899]
2023-03-06 11:21:10 [INFO] [EVAL] The model with the best validation mIoU (0.9858) was saved at iter 1500.
<class 'paddle.nn.layer.conv.Conv2D'>'s flops has been counted
<class 'paddle.nn.layer.norm.BatchNorm2D'>'s flops has been counted
<class 'paddle.nn.layer.activation.ReLU'>'s flops has been counted
<class 'paddle.nn.layer.pooling.AvgPool2D'>'s flops has been counted
<class 'paddle.nn.layer.pooling.AdaptiveAvgPool2D'>'s flops has been counted
Total Flops: 11465470848 Total Params: 8039122
4.1 训练避坑指南
one_hot_kernel
相关的报错,需要注意类别是否正确设置,需要考虑背景类,即num_classes: 实际类 + 1
。
Error: /paddle/paddle/phi/kernels/gpu/one_hot_kernel.cu:38 Assertion `p_in_data[idx] >= 0 && p_in_data[idx] < depth` failed. Illegal index value, Input(input) value should be greater than or equal to 0, and less than depth [1], but received [1].
Error: /paddle/paddle/phi/kernels/gpu/one_hot_kernel.cu:38 Assertion `p_in_data[idx] >= 0 && p_in_data[idx] < depth` failed. Illegal index value, Input(input) value should be greater than or equal to 0, and less than depth [1], but received [1].
Error: /paddle/paddle/phi/kernels/gpu/one_hot_kernel.cu:38 Assertion `p_in_data[idx] >= 0 && p_in_data[idx] < depth` failed. Illegal index value, Input(input) value should be greater than or equal to 0, and less than depth [1], but received [1].
one_hot_kernel
另一种报错
Input(input) value should be greater than or equal to 0, and less than depth [5], but received [-1].
Error: /paddle/paddle/phi/kernels/gpu/one_hot_kernel.cu:38 Assertion `p_in_data[idx] >= 0 && p_in_data[idx] < depth` failed. Illegal index value, Input(input) value should be greater than or equal to 0, and less than depth [5], but received [-1].
Error: /paddle/paddle/phi/kernels/gpu/one_hot_kernel.cu:38 Assertion `p_in_data[idx] >= 0 && p_in_data[idx] < depth` failed. Illegal index value, Input(input) value should be greater than or equal to 0, and less than depth [5], but received [-1].
Error: /paddle/paddle/phi/kernels/gpu/one_hot_kernel.cu:38 Assertion `p_in_data[idx] >= 0 && p_in_data[idx] < depth` failed. Illegal index value,
此时需要注意mask中标签是否超过[0, num_classes + 1]
的范围。
4.2 数据模型可视化
使用AI Studio
自带的基于VisualDL
的可视化功能,可以很方便地实现数据可视化。
点击启动VisualDL
服务,即可在AI Studio
上启动VisualDL
服务,并开始追踪训练进展情况。
5. 模型评估
!python val.py \
--config /home/aistudio/work/pp_liteseg_stdc1.yml \
--model_path output/mask_iron/best_model/model.pdparams
2023-03-06 11:22:01 [INFO]
---------------Config Information---------------
batch_size: 4
iters: 2000
loss:
coef:
- 1
- 1
- 1
types:
- min_kept: 130000
type: OhemCrossEntropyLoss
- min_kept: 130000
type: OhemCrossEntropyLoss
- min_kept: 130000
type: OhemCrossEntropyLoss
lr_scheduler:
end_lr: 0
learning_rate: 0.005
power: 0.9
type: PolynomialDecay
warmup_iters: 100
warmup_start_lr: 1.0e-05
model:
arm_out_chs:
- 32
- 64
- 128
backbone:
pretrained: https://bj.bcebos.com/paddleseg/dygraph/PP_STDCNet1.tar.gz
type: STDC1
seg_head_inter_chs:
- 32
- 64
- 64
type: PPLiteSeg
optimizer:
momentum: 0.9
type: sgd
weight_decay: 0.0005
test_config:
aug_eval: true
scales: 0.5
train_dataset:
dataset_root: /home/aistudio/data/dataset
mode: train
num_classes: 2
train_path: /home/aistudio/data/dataset/train.txt
transforms:
- max_scale_factor: 1.5
min_scale_factor: 0.125
scale_step_size: 0.125
type: ResizeStepScaling
- crop_size:
- 1024
- 512
type: RandomPaddingCrop
- type: RandomHorizontalFlip
- brightness_range: 0.5
contrast_range: 0.5
saturation_range: 0.5
type: RandomDistort
- type: Normalize
type: Dataset
val_dataset:
dataset_root: /home/aistudio/data/dataset
mode: val
num_classes: 2
transforms:
- type: Normalize
type: Dataset
val_path: /home/aistudio/data/dataset/val.txt
------------------------------------------------
W0306 11:22:01.376241 7367 gpu_resources.cc:61] Please NOTE: device: 0, GPU Compute Capability: 7.0, Driver API Version: 11.2, Runtime API Version: 11.2
W0306 11:22:01.376297 7367 gpu_resources.cc:91] device: 0, cuDNN Version: 8.2.
2023-03-06 11:22:02 [INFO] Loading pretrained model from https://bj.bcebos.com/paddleseg/dygraph/PP_STDCNet1.tar.gz
2023-03-06 11:22:03 [INFO] There are 145/145 variables loaded into STDCNet.
2023-03-06 11:22:03 [INFO] Loading pretrained model from output/mask_iron/best_model/model.pdparams
2023-03-06 11:22:03 [INFO] There are 247/247 variables loaded into PPLiteSeg.
2023-03-06 11:22:03 [INFO] Loaded trained params of model successfully
2023-03-06 11:22:03 [INFO] Start evaluating (total_samples: 32, total_iters: 32)...
Corrupt JPEG data: 2 extraneous bytes before marker 0xd9
32/32 [==============================] - 6s 196ms/step - batch_cost: 0.1953 - reader cost: 0.1395
2023-03-06 11:22:09 [INFO] [EVAL] #Images: 32 mIoU: 0.9858 Acc: 0.9947 Kappa: 0.9857 Dice: 0.9928
2023-03-06 11:22:09 [INFO] [EVAL] Class IoU:
[0.993 0.9787]
2023-03-06 11:22:09 [INFO] [EVAL] Class Precision:
[0.9969 0.9878]
2023-03-06 11:22:09 [INFO] [EVAL] Class Recall:
[0.996 0.9906]
由评估输出可见,模型性能 mIoU: 0.9858 Acc: 0.9947。能够满足实际工业场景需求。
# 也可进行多尺度翻转评估
!python val.py \
--config /home/aistudio/work/pp_liteseg_stdc1.yml \
--model_path output/mask_iron/best_model/model.pdparams \
--aug_eval \
--scales 0.75 1.0 1.25 \
--flip_horizontal
2023-03-06 11:22:43 [INFO]
---------------Config Information---------------
batch_size: 4
iters: 2000
loss:
coef:
- 1
- 1
- 1
types:
- min_kept: 130000
type: OhemCrossEntropyLoss
- min_kept: 130000
type: OhemCrossEntropyLoss
- min_kept: 130000
type: OhemCrossEntropyLoss
lr_scheduler:
end_lr: 0
learning_rate: 0.005
power: 0.9
type: PolynomialDecay
warmup_iters: 100
warmup_start_lr: 1.0e-05
model:
arm_out_chs:
- 32
- 64
- 128
backbone:
pretrained: https://bj.bcebos.com/paddleseg/dygraph/PP_STDCNet1.tar.gz
type: STDC1
seg_head_inter_chs:
- 32
- 64
- 64
type: PPLiteSeg
optimizer:
momentum: 0.9
type: sgd
weight_decay: 0.0005
test_config:
aug_eval: true
scales: 0.5
train_dataset:
dataset_root: /home/aistudio/data/dataset
mode: train
num_classes: 2
train_path: /home/aistudio/data/dataset/train.txt
transforms:
- max_scale_factor: 1.5
min_scale_factor: 0.125
scale_step_size: 0.125
type: ResizeStepScaling
- crop_size:
- 1024
- 512
type: RandomPaddingCrop
- type: RandomHorizontalFlip
- brightness_range: 0.5
contrast_range: 0.5
saturation_range: 0.5
type: RandomDistort
- type: Normalize
type: Dataset
val_dataset:
dataset_root: /home/aistudio/data/dataset
mode: val
num_classes: 2
transforms:
- type: Normalize
type: Dataset
val_path: /home/aistudio/data/dataset/val.txt
------------------------------------------------
W0306 11:22:43.975018 7513 gpu_resources.cc:61] Please NOTE: device: 0, GPU Compute Capability: 7.0, Driver API Version: 11.2, Runtime API Version: 11.2
W0306 11:22:43.975075 7513 gpu_resources.cc:91] device: 0, cuDNN Version: 8.2.
2023-03-06 11:22:45 [INFO] Loading pretrained model from https://bj.bcebos.com/paddleseg/dygraph/PP_STDCNet1.tar.gz
2023-03-06 11:22:45 [INFO] There are 145/145 variables loaded into STDCNet.
2023-03-06 11:22:45 [INFO] Loading pretrained model from output/mask_iron/best_model/model.pdparams
2023-03-06 11:22:45 [INFO] There are 247/247 variables loaded into PPLiteSeg.
2023-03-06 11:22:45 [INFO] Loaded trained params of model successfully
2023-03-06 11:22:45 [INFO] Start evaluating (total_samples: 32, total_iters: 32)...
Corrupt JPEG data: 2 extraneous bytes before marker 0xd9
32/32 [==============================] - 6s 199ms/step - batch_cost: 0.1990 - reader cost: 0.0707
2023-03-06 11:22:52 [INFO] [EVAL] #Images: 32 mIoU: 0.9877 Acc: 0.9954 Kappa: 0.9876 Dice: 0.9938
2023-03-06 11:22:52 [INFO] [EVAL] Class IoU:
[0.9939 0.9815]
2023-03-06 11:22:52 [INFO] [EVAL] Class Precision:
[0.997 0.9904]
2023-03-06 11:22:52 [INFO] [EVAL] Class Recall:
[0.9969 0.9909]
# 还可进行滑窗评估
!python val.py \
--config /home/aistudio/work/pp_liteseg_stdc1.yml \
--model_path output/mask_iron/best_model/model.pdparams \
--is_slide \
--crop_size 256 256 \
--stride 128 128
2023-03-06 11:23:01 [INFO]
---------------Config Information---------------
batch_size: 4
iters: 2000
loss:
coef:
- 1
- 1
- 1
types:
- min_kept: 130000
type: OhemCrossEntropyLoss
- min_kept: 130000
type: OhemCrossEntropyLoss
- min_kept: 130000
type: OhemCrossEntropyLoss
lr_scheduler:
end_lr: 0
learning_rate: 0.005
power: 0.9
type: PolynomialDecay
warmup_iters: 100
warmup_start_lr: 1.0e-05
model:
arm_out_chs:
- 32
- 64
- 128
backbone:
pretrained: https://bj.bcebos.com/paddleseg/dygraph/PP_STDCNet1.tar.gz
type: STDC1
seg_head_inter_chs:
- 32
- 64
- 64
type: PPLiteSeg
optimizer:
momentum: 0.9
type: sgd
weight_decay: 0.0005
test_config:
aug_eval: true
scales: 0.5
train_dataset:
dataset_root: /home/aistudio/data/dataset
mode: train
num_classes: 2
train_path: /home/aistudio/data/dataset/train.txt
transforms:
- max_scale_factor: 1.5
min_scale_factor: 0.125
scale_step_size: 0.125
type: ResizeStepScaling
- crop_size:
- 1024
- 512
type: RandomPaddingCrop
- type: RandomHorizontalFlip
- brightness_range: 0.5
contrast_range: 0.5
saturation_range: 0.5
type: RandomDistort
- type: Normalize
type: Dataset
val_dataset:
dataset_root: /home/aistudio/data/dataset
mode: val
num_classes: 2
transforms:
- type: Normalize
type: Dataset
val_path: /home/aistudio/data/dataset/val.txt
------------------------------------------------
W0306 11:23:01.181236 7584 gpu_resources.cc:61] Please NOTE: device: 0, GPU Compute Capability: 7.0, Driver API Version: 11.2, Runtime API Version: 11.2
W0306 11:23:01.181291 7584 gpu_resources.cc:91] device: 0, cuDNN Version: 8.2.
2023-03-06 11:23:02 [INFO] Loading pretrained model from https://bj.bcebos.com/paddleseg/dygraph/PP_STDCNet1.tar.gz
2023-03-06 11:23:02 [INFO] There are 145/145 variables loaded into STDCNet.
2023-03-06 11:23:02 [INFO] Loading pretrained model from output/mask_iron/best_model/model.pdparams
2023-03-06 11:23:02 [INFO] There are 247/247 variables loaded into PPLiteSeg.
2023-03-06 11:23:02 [INFO] Loaded trained params of model successfully
2023-03-06 11:23:02 [INFO] Start evaluating (total_samples: 32, total_iters: 32)...
Corrupt JPEG data: 2 extraneous bytes before marker 0xd9
32/32 [==============================] - 17s 533ms/step - batch_cost: 0.5324 - reader cost: 0.0147
2023-03-06 11:23:19 [INFO] [EVAL] #Images: 32 mIoU: 0.9862 Acc: 0.9948 Kappa: 0.9861 Dice: 0.9930
2023-03-06 11:23:19 [INFO] [EVAL] Class IoU:
[0.9932 0.9792]
2023-03-06 11:23:19 [INFO] [EVAL] Class Precision:
[0.9975 0.9868]
2023-03-06 11:23:19 [INFO] [EVAL] Class Recall:
[0.9957 0.9922]
6. 模型预测
使用predict.py
可用来查看具体样本的切割样本效果。
!python predict.py \
--config /home/aistudio/work/pp_liteseg_stdc1.yml \
--model_path output/mask_iron/best_model/model.pdparams \
--image_path /home/aistudio/data/dataset/ec539f77-7061-4106-9914-8d66f450234d.jpg \
--save_dir output/result
# !python predict.py \
# --config /home/aistudio/work/pp_liteseg.yml \
# --model_path output/mask_iron/best_model/model.pdparams \
# --image_path /home/aistudio/data/dataset/20220705-153804.jpg \
# --save_dir output/result
2023-03-06 11:25:10 [INFO]
---------------Config Information---------------
batch_size: 4
iters: 2000
loss:
coef:
- 1
- 1
- 1
types:
- min_kept: 130000
type: OhemCrossEntropyLoss
- min_kept: 130000
type: OhemCrossEntropyLoss
- min_kept: 130000
type: OhemCrossEntropyLoss
lr_scheduler:
end_lr: 0
learning_rate: 0.005
power: 0.9
type: PolynomialDecay
warmup_iters: 100
warmup_start_lr: 1.0e-05
model:
arm_out_chs:
- 32
- 64
- 128
backbone:
pretrained: https://bj.bcebos.com/paddleseg/dygraph/PP_STDCNet1.tar.gz
type: STDC1
seg_head_inter_chs:
- 32
- 64
- 64
type: PPLiteSeg
optimizer:
momentum: 0.9
type: sgd
weight_decay: 0.0005
test_config:
aug_eval: true
scales: 0.5
train_dataset:
dataset_root: /home/aistudio/data/dataset
mode: train
num_classes: 2
train_path: /home/aistudio/data/dataset/train.txt
transforms:
- max_scale_factor: 1.5
min_scale_factor: 0.125
scale_step_size: 0.125
type: ResizeStepScaling
- crop_size:
- 1024
- 512
type: RandomPaddingCrop
- type: RandomHorizontalFlip
- brightness_range: 0.5
contrast_range: 0.5
saturation_range: 0.5
type: RandomDistort
- type: Normalize
type: Dataset
val_dataset:
dataset_root: /home/aistudio/data/dataset
mode: val
num_classes: 2
transforms:
- type: Normalize
type: Dataset
val_path: /home/aistudio/data/dataset/val.txt
------------------------------------------------
W0306 11:25:10.929594 7985 gpu_resources.cc:61] Please NOTE: device: 0, GPU Compute Capability: 7.0, Driver API Version: 11.2, Runtime API Version: 11.2
W0306 11:25:10.929652 7985 gpu_resources.cc:91] device: 0, cuDNN Version: 8.2.
2023-03-06 11:25:12 [INFO] Loading pretrained model from https://bj.bcebos.com/paddleseg/dygraph/PP_STDCNet1.tar.gz
2023-03-06 11:25:12 [INFO] There are 145/145 variables loaded into STDCNet.
2023-03-06 11:25:12 [INFO] Number of predict images = 1
2023-03-06 11:25:12 [INFO] Loading pretrained model from output/mask_iron/best_model/model.pdparams
2023-03-06 11:25:12 [INFO] There are 247/247 variables loaded into PPLiteSeg.
2023-03-06 11:25:12 [INFO] Start to predict...
1/1 [==============================] - 1s 1s/step
预测的结果如下所示
import matplotlib.pyplot as plt
import cv2
im = cv2.imread("/home/aistudio/work/PaddleSeg/output/result/pseudo_color_prediction/ec539f77-7061-4106-9914-8d66f450234d.png")
# cv2.imshow("result", im)
plt.imshow(cv2.cvtColor(im, cv2.COLOR_BGR2RGB))
plt.figure()
im = cv2.imread("/home/aistudio/work/PaddleSeg/output/result/added_prediction/ec539f77-7061-4106-9914-8d66f450234d.jpg")
plt.imshow(cv2.cvtColor(im, cv2.COLOR_BGR2RGB))
<matplotlib.image.AxesImage at 0x7f4aad9cf050>
接下来,利用预测的结果,并采用最大联通域处理后,判断钢筋是否超限,(此脚本的路径为 work/postprocessing.py
)
import cv2
def largestcomponent(img_path, threshold=None):
"""
Filter the input image_path with threshold, only component that have area larger than threshold will be kept.
Arg:
img_path: path to a binary img
threshold: connected componet with area larger than this value will be kept
"""
binary = cv2.imread(img_path, cv2.IMREAD_GRAYSCALE)
binary[binary == binary.min()] = 0
binary[binary == binary.max()] = 255
assert (
binary.max() == 255 and binary.min() == 0
), "The input need to be a binary image, but the maxval in image is {} and the minval in image is {}".format(
binary.max(), binary.min()
)
if threshold is None:
threshold = binary.shape[0] * binary.shape[1] * 0.01
contours, hierarchy = cv2.findContours(
binary, cv2.RETR_TREE, cv2.CHAIN_APPROX_NONE
)
for k in range(len(contours)):
if cv2.contourArea(contours[k]) < threshold:
cv2.fillPoly(binary, [contours[k]], 0)
cv2.imwrite(img_path.split(".")[0] + "_postprocessed.png", binary)
# 此处,我们可以对比最大联通域处理前后的差别,可以发现滤除了小的联通区域
prediction = "/home/aistudio/work/PaddleSeg/output/result/pseudo_color_prediction/ec539f77-7061-4106-9914-8d66f450234d.png"
# prediction = "/home/aistudio/work/PaddleSeg/output/result/pseudo_color_prediction/20220705-153804.png"
largestcomponent(prediction)
before_image = cv2.imread(prediction)
after_image = cv2.imread(prediction.replace(".png", "_postprocessed.png"))
plt.subplot(1, 2, 1)
plt.imshow(cv2.cvtColor(before_image, cv2.COLOR_BGR2RGB))
plt.subplot(1, 2, 2)
plt.imshow(cv2.cvtColor(after_image, cv2.COLOR_BGR2RGB))
plt.show()
# 判断钢筋是否超限
def excesslimit(image_path, direction="right", position=0.6):
"""
Automatically tells if the steel bar excess manually set position.
Arg:
img_path: path to a binary img
direction: which part of the img is the focused area for detecting bar excession.
position: the ratio of the position of the line to the width of the image.
Return:
excess: whether there is steel wheel excess the limit line.
excess_potion: the portion of the excess steel bar to the whole bar.
"""
excess_portion = 0.0
binary = cv2.imread(image_path, cv2.IMREAD_GRAYSCALE)
binary[binary == binary.min()] = 0
binary[binary == binary.max()] = 255
assert (
binary.max() == 255 and binary.min() == 0
), "The input need to be a binary image, but the maxval in image is {} and the minval in image is {}".format(
binary.max(), binary.min()
)
assert (
direction == "left" or direction == "right"
), "The direction indicates the side of image that iron excess, it should be 'right' or 'left', but we got {}".format(
direction
)
assert (
position > 0 and position < 1
), "The position indicates the relative position to set the line, it should bigger than 0 and smaller than 1, but we got {}".format(
position
)
img_pos = int(binary.shape[1] * position)
if direction == "right":
if binary[:, img_pos:].sum() > 0:
excess_portion = binary[:, img_pos:].sum() / binary.sum()
binary[:, img_pos : img_pos + 3] = 255
else:
if binary[:, :img_pos].sum() > 0:
excess_portion = binary[:, :img_pos].sum() / binary.sum()
binary[:, img_pos - 3 : img_pos] = 255
print(
"The iron is {}excessed in {}, and the excess portion is {}".format(
["", "not "][excess_portion == 0], image_path, excess_portion
)
)
# cv2.imwrite(image_path.split(".")[0] + "_fullpostprocessed.png", binary)
cv2.imwrite(image_path.replace("_postprocessed.png", "_fullpostprocessed.png"), binary)
return excess_portion > 0, excess_portion
# 对预测结果批量判断是否超限
import os
import glob
output_dir = "/home/aistudio/work/PaddleSeg/output"
pseudo_color_result = os.path.join(output_dir, 'result/pseudo_color_prediction')
os.system(f"rm {pseudo_color_result}/*_*postprocessed.*")
for img_path in glob.glob(os.path.join(pseudo_color_result, "*.png")):
largestcomponent(img_path)
postproc_img_path = img_path.replace(".png", "_postprocessed.png")
excesslimit(postproc_img_path, "left", 0.3)
The iron is excessed in /home/aistudio/work/PaddleSeg/output/result/pseudo_color_prediction/ec539f77-7061-4106-9914-8d66f450234d_postprocessed.png, and the excess portion is 0.040420855682695565
/opt/conda/envs/python35-paddle120-env/lib/python3.7/site-packages/ipykernel_launcher.py:51: DeprecationWarning: In future, it will be an error for 'np.bool_' scalars to be interpreted as an index
#可视化后处理结果
im = cv2.imread("/home/aistudio/work/PaddleSeg/output/result/pseudo_color_prediction/ec539f77-7061-4106-9914-8d66f450234d_fullpostprocessed.png")
# cv2.imshow("result", im)
plt.imshow(im)
<matplotlib.image.AxesImage at 0x7f4aad9064d0>
7. 模型推理与部署
7.1 使用 Paddle Inference 推理
导出训练好的模型为paddle inference
模型
!export CUDA_VISIBLE_DEVICES=0 # Set a usable GPU.
# If on windows, Run the following command
# set CUDA_VISIBLE_DEVICES=0
!python export.py \
--config /home/aistudio/work/pp_liteseg_stdc1.yml \
--model_path output/mask_iron/best_model/model.pdparams \
--save_dir output/inference
W0306 12:13:14.298084 14841 gpu_resources.cc:61] Please NOTE: device: 0, GPU Compute Capability: 7.0, Driver API Version: 11.2, Runtime API Version: 11.2
W0306 12:13:14.302328 14841 gpu_resources.cc:91] device: 0, cuDNN Version: 8.2.
2023-03-06 12:13:15 [INFO] Loading pretrained model from https://bj.bcebos.com/paddleseg/dygraph/PP_STDCNet1.tar.gz
2023-03-06 12:13:16 [INFO] There are 145/145 variables loaded into STDCNet.
2023-03-06 12:13:16 [INFO] Loaded trained params of model successfully.
2023-03-06 12:13:19 [INFO] Model is saved in output/inference.
使用推理模型预测
!python deploy/python/infer.py \
--config output/inference/deploy.yaml \
--save_dir output/infer_result \
--image_path /home/aistudio/data/dataset/bcd33bcd-d48c-4409-940d-51301c8a7697.jpg
2023-03-06 12:14:42 [INFO] Use GPU
W0306 12:14:44.105751 15073 analysis_predictor.cc:1391] The one-time configuration of analysis predictor failed, which may be due to native predictor called first and its configurations taken effect.
[1m[35m--- Running analysis [ir_graph_build_pass][0m
[1m[35m--- Running analysis [ir_graph_clean_pass][0m
[1m[35m--- Running analysis [ir_analysis_pass][0m
[32m--- Running IR pass [is_test_pass][0m
[32m--- Running IR pass [simplify_with_basic_ops_pass][0m
[32m--- Running IR pass [conv_bn_fuse_pass][0m
I0306 12:14:44.260706 15073 fuse_pass_base.cc:59] --- detected 39 subgraphs
[32m--- Running IR pass [conv_eltwiseadd_bn_fuse_pass][0m
I0306 12:14:44.283650 15073 fuse_pass_base.cc:59] --- detected 4 subgraphs
[32m--- Running IR pass [embedding_eltwise_layernorm_fuse_pass][0m
[32m--- Running IR pass [multihead_matmul_fuse_pass_v2][0m
[32m--- Running IR pass [fused_multi_transformer_encoder_pass][0m
[32m--- Running IR pass [fused_multi_transformer_decoder_pass][0m
[32m--- Running IR pass [fused_multi_transformer_encoder_fuse_qkv_pass][0m
[32m--- Running IR pass [fused_multi_transformer_decoder_fuse_qkv_pass][0m
[32m--- Running IR pass [multi_devices_fused_multi_transformer_encoder_fuse_qkv_pass][0m
[32m--- Running IR pass [multi_devices_fused_multi_transformer_decoder_fuse_qkv_pass][0m
[32m--- Running IR pass [fuse_multi_transformer_layer_pass][0m
[32m--- Running IR pass [gpu_cpu_squeeze2_matmul_fuse_pass][0m
[32m--- Running IR pass [gpu_cpu_reshape2_matmul_fuse_pass][0m
[32m--- Running IR pass [gpu_cpu_flatten2_matmul_fuse_pass][0m
[32m--- Running IR pass [gpu_cpu_map_matmul_v2_to_mul_pass][0m
[32m--- Running IR pass [gpu_cpu_map_matmul_v2_to_matmul_pass][0m
[32m--- Running IR pass [matmul_scale_fuse_pass][0m
[32m--- Running IR pass [multihead_matmul_fuse_pass_v3][0m
[32m--- Running IR pass [gpu_cpu_map_matmul_to_mul_pass][0m
[32m--- Running IR pass [fc_fuse_pass][0m
[32m--- Running IR pass [fc_elementwise_layernorm_fuse_pass][0m
[32m--- Running IR pass [conv_elementwise_add_act_fuse_pass][0m
[32m--- Running IR pass [conv_elementwise_add2_act_fuse_pass][0m
[32m--- Running IR pass [conv_elementwise_add_fuse_pass][0m
[32m--- Running IR pass [transpose_flatten_concat_fuse_pass][0m
[32m--- Running IR pass [constant_folding_pass][0m
[32m--- Running IR pass [runtime_context_cache_pass][0m
[1m[35m--- Running analysis [ir_params_sync_among_devices_pass][0m
I0306 12:14:44.666788 15073 ir_params_sync_among_devices_pass.cc:89] Sync params from CPU to GPU
[1m[35m--- Running analysis [adjust_cudnn_workspace_size_pass][0m
[1m[35m--- Running analysis [inference_op_replace_pass][0m
[1m[35m--- Running analysis [memory_optimize_pass][0m
I0306 12:14:44.693254 15073 memory_optimize_pass.cc:219] Cluster name : shape_1.tmp_0_slice_0 size: 8
I0306 12:14:44.693290 15073 memory_optimize_pass.cc:219] Cluster name : shape_0.tmp_0_slice_0 size: 8
I0306 12:14:44.693295 15073 memory_optimize_pass.cc:219] Cluster name : x size: 12
I0306 12:14:44.693297 15073 memory_optimize_pass.cc:219] Cluster name : max_1.tmp_0 size: 4
I0306 12:14:44.693307 15073 memory_optimize_pass.cc:219] Cluster name : batch_norm_21.tmp_2 size: 2048
I0306 12:14:44.693315 15073 memory_optimize_pass.cc:219] Cluster name : batch_norm_21.tmp_0 size: 2048
I0306 12:14:44.693318 15073 memory_optimize_pass.cc:219] Cluster name : relu_28.tmp_0 size: 8192
I0306 12:14:44.693325 15073 memory_optimize_pass.cc:219] Cluster name : pool2d_5.tmp_0 size: 65536
I0306 12:14:44.693327 15073 memory_optimize_pass.cc:219] Cluster name : concat_5.tmp_0 size: 4096
I0306 12:14:44.693341 15073 memory_optimize_pass.cc:219] Cluster name : concat_1.tmp_0 size: 1024
I0306 12:14:44.693343 15073 memory_optimize_pass.cc:219] Cluster name : concat_3.tmp_0 size: 2048
[1m[35m--- Running analysis [ir_graph_to_program_pass][0m
I0306 12:14:44.716624 15073 analysis_predictor.cc:1314] ======= optimize end =======
I0306 12:14:44.718086 15073 naive_executor.cc:110] --- skip [feed], feed -> x
I0306 12:14:44.719072 15073 naive_executor.cc:110] --- skip [argmax_0.tmp_0], fetch -> fetch
W0306 12:14:44.962486 15073 gpu_resources.cc:61] Please NOTE: device: 0, GPU Compute Capability: 7.0, Driver API Version: 11.2, Runtime API Version: 11.2
W0306 12:14:44.966229 15073 gpu_resources.cc:91] device: 0, cuDNN Version: 8.2.
2023-03-06 12:14:46 [INFO] Finish
img_path = "/home/aistudio/work/PaddleSeg/output/infer_result/bcd33bcd-d48c-4409-940d-51301c8a7697.png"
im = cv2.imread(img_path, cv2.IMREAD_UNCHANGED)
plt.imshow(cv2.cvtColor(im, cv2.COLOR_BGR2RGB))
<matplotlib.image.AxesImage at 0x7f4ab61111d0>
根据模型输出,判断钢筋是否超限
img_path = "/home/aistudio/work/PaddleSeg/output/infer_result/bcd33bcd-d48c-4409-940d-51301c8a7697.png"
largestcomponent(img_path)
postproc_img_path = img_path.replace(".png", "_postprocessed.png")
excesslimit(postproc_img_path, "right", 0.5)
The iron is excessed in /home/aistudio/work/PaddleSeg/output/infer_result/bcd33bcd-d48c-4409-940d-51301c8a7697_postprocessed.png, and the excess portion is 0.1875316840529423
/opt/conda/envs/python35-paddle120-env/lib/python3.7/site-packages/ipykernel_launcher.py:51: DeprecationWarning: In future, it will be an error for 'np.bool_' scalars to be interpreted as an index
(True, 0.1875316840529423)
# 可视化判断结果
img_path = "/home/aistudio/work/PaddleSeg/output/infer_result/bcd33bcd-d48c-4409-940d-51301c8a7697_fullpostprocessed.png"
im = cv2.imread(img_path)
plt.imshow(im)
<matplotlib.image.AxesImage at 0x7f4aff724490>
7.2 使用 FastDeploy 部署
FastDeploy是一款全场景、易用灵活、极致高效的AI推理部署工具。提供📦开箱即用的云边端部署体验, 支持超过 🔥150+ Text, Vision, Speech和跨模态模型,并实现🔚端到端的推理性能优化。包括图像分类、物体检测、图像分割、人脸检测、人脸识别、关键点检测、抠图、OCR、NLP、TTS等任务,满足开发者多场景、多硬件、多平台的产业部署需求。
# 安装 fastdeploy
!pip install fastdeploy-gpu-python -f https://www.paddlepaddle.org.cn/whl/fastdeploy.html
import fastdeploy as fd
model_file = "/home/aistudio/work/PaddleSeg/output/inference/model.pdmodel"
params_file = "/home/aistudio/work/PaddleSeg/output/inference/model.pdiparams"
infer_cfg_file = "/home/aistudio/work/PaddleSeg/output/inference/deploy.yaml"
# 模型推理的配置信息
option = fd.RuntimeOption()
model = fd.vision.segmentation.PaddleSegModel(model_file, params_file, infer_cfg_file, option)
[INFO] fastdeploy/vision/common/processors/transform.cc(93)::FuseNormalizeHWC2CHW Normalize and HWC2CHW are fused to NormalizeAndPermute in preprocessing pipeline.
[INFO] fastdeploy/vision/common/processors/transform.cc(159)::FuseNormalizeColorConvert BGR2RGB and NormalizeAndPermute are fused to NormalizeAndPermute with swap_rb=1
[INFO] fastdeploy/runtime/backends/openvino/ov_backend.cc(226)::InitFromPaddle Compile OpenVINO model on device_name:CPU.
[INFO] fastdeploy/runtime/runtime.cc(322)::CreateOpenVINOBackend Runtime initialized with Backend::OPENVINO in Device::CPU.
# 预测结果
import cv2
img_path = "/home/aistudio/data/dataset/8f7fcf0a-a3ea-41f2-9e67-4cbaa61238a4.jpg"
im = cv2.imread(img_path)
result = model.predict(im)
print(result)
SegmentationResult Image masks 10 rows x 10 cols:
[0, 0, 0, 0, 0, 0, 0, 0, 0, 0, .....]
[0, 0, 0, 0, 0, 0, 0, 0, 0, 0, .....]
[0, 0, 0, 0, 0, 0, 0, 0, 0, 0, .....]
[0, 0, 0, 0, 0, 0, 0, 0, 0, 0, .....]
[0, 0, 0, 0, 0, 0, 0, 0, 0, 0, .....]
[0, 0, 0, 0, 0, 0, 0, 0, 0, 0, .....]
[0, 0, 0, 0, 0, 0, 0, 0, 0, 0, .....]
[0, 0, 0, 0, 0, 0, 0, 0, 0, 0, .....]
[0, 0, 0, 0, 0, 0, 0, 0, 0, 0, .....]
[0, 0, 0, 0, 0, 0, 0, 0, 0, 0, .....]
...........
result shape is: [1080 1920]
# 使用fastdeploy提供的可视化函数进行可视化
import matplotlib.pyplot as plt
vis_im = fd.vision.visualize.vis_segmentation(im, result, 0.5)
plt.imshow(cv2.cvtColor(vis_im, cv2.COLOR_BGR2RGB))
<matplotlib.image.AxesImage at 0x7f49223e7150>
# 判断钢筋是否超限
# 为了便于演示,兼容上面的判断接口。这处将结果导出为mask图片。
import numpy as np
mask = np.reshape(result.label_map, result.shape)
mask = np.uint8(mask)
mask_path = "/home/aistudio/work/PaddleSeg/output/infer_result/mask.png"
cv2.imwrite(mask_path, mask)
# print(mask_path)
largestcomponent(mask_path)
post_img_path = mask_path.replace(".png", "_postprocessed.png")
# print(post_img_path)
excesslimit(post_img_path, "right", 0.7)
The iron is not excessed in /home/aistudio/work/PaddleSeg/output/infer_result/mask_postprocessed.png, and the excess portion is 0.0
v2.cvtColor(vis_im, cv2.COLOR_BGR2RGB))
<matplotlib.image.AxesImage at 0x7f49223e7150>
[外链图片转存中...(img-SadVrJ5V-1678806404026)]
```python
# 判断钢筋是否超限
# 为了便于演示,兼容上面的判断接口。这处将结果导出为mask图片。
import numpy as np
mask = np.reshape(result.label_map, result.shape)
mask = np.uint8(mask)
mask_path = "/home/aistudio/work/PaddleSeg/output/infer_result/mask.png"
cv2.imwrite(mask_path, mask)
# print(mask_path)
largestcomponent(mask_path)
post_img_path = mask_path.replace(".png", "_postprocessed.png")
# print(post_img_path)
excesslimit(post_img_path, "right", 0.7)
The iron is not excessed in /home/aistudio/work/PaddleSeg/output/infer_result/mask_postprocessed.png, and the excess portion is 0.0
(False, 0.0)
# 可视化判断结果
im_path = "/home/aistudio/work/PaddleSeg/output/infer_result/mask_fullpostprocessed.png"
im = cv2.imread(im_path)
plt.imshow(im)
<matplotlib.image.AxesImage at 0x7f49213344d0>
8. 总结
本项目演示了在工业实际场景下,使用PaddleSeg
开发套件,进行语义分割模型训练,并使用FastDeploy
进行部署应用,解决钢筋长度超限的自动监控问题。结果证明,本技术方案切实可行,可实现端到端全自动的钢筋长度超限监控,为企业生产降本增效。希望本应用范例可以给各行业从业人员和开发者带来有益的启发。
加入交流群,一起学习吧
更多关于分割的知识请移步PaddleSeg,如有帮助,欢迎Star⭐支持~
github地址:https://github.com/PaddlePaddle/PaddleSeg
欢迎加入PaddleSeg微信群,交流更多前沿技术。
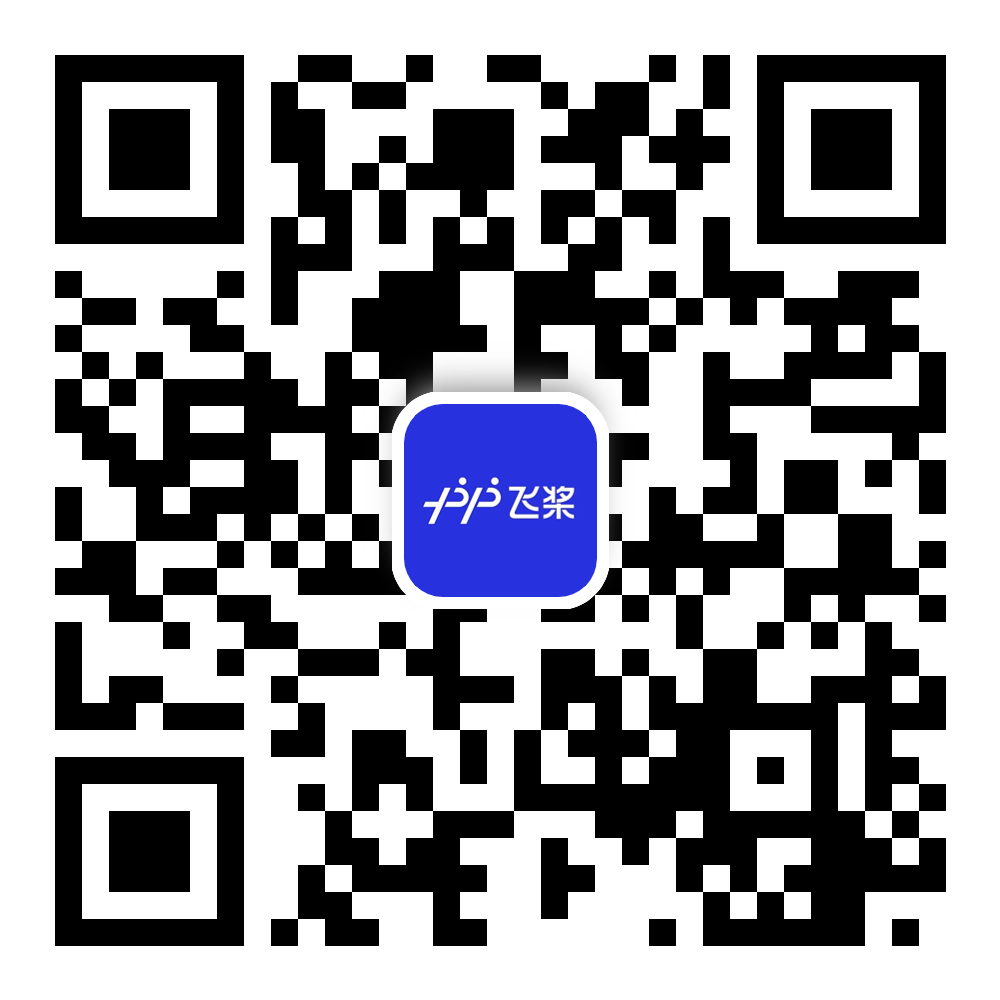