import numpy as np,matplotlib.pyplot as plt,copy plt.rcParams['font.sans-serif']=['SimHei'] plt.rcParams['axes.unicode_minus']=False #1.数据预处理 import sklearn.datasets as dts def data_process(): data_cancer=dts.load_breast_cancer() x=data_cancer.data[:,:-1] y=data_cancer.target #特征缩放(标准化) x=(x-np.mean(x,axis=0))/np.std(x,axis=0,ddof=1) #截距拼接 m,n=x.shape x=np.c_[np.ones((m,1)),x] y=np.c_[y] #洗牌 np.random.seed(5) order=np.random.permutation(m) x=x[order] y=y[order] # 分割训练集和测试集 6:4 num = int(m * 0.6) train_x, test_x = np.split(x, [num]) train_y, test_y = np.split(y, [num]) return train_x, test_x, train_y, test_y #线性模型 def lh(x,theta): z=x.dot(theta) return z # 实现Sigmoid函数 def sigmoid(z): h=1/(1+np.exp(-z)) return h # 实现逻辑回归的代价函数 交叉熵+正则化 def loss_func(h,y,lamda,thetaR): m=len(h) R=lamda/(2*m)*np.sum(thetaR**2) J=-1/m*np.sum(y*np.log(h)+(1-y)*np.log(1-h))+R return J # 实现梯度下降函数 def grad_decent(x,h,y,lamda,thetaR): e=h-y m=len(h) dt=1/m*x.T.dot(e)+lamda/m*thetaR return dt # 实现逻辑回归模型精度函数 def acc_func(h,y): acc=np.mean(y==(h>=0.5)) return acc # 通过梯度下降训练逻辑回归模型 def train_mode(x,y,lamda=0.1,alpha=0.7,iters=100): m,n=x.shape theta=np.zeros((n,1)) loss_list=[] for i in range(iters): z=lh(x,theta) h=sigmoid(z) thetaR=copy.copy(theta) thetaR[0]=0 loss=loss_func(h,y,lamda,thetaR) loss_list.append(loss) dt=grad_decent(x,h,y,lamda,thetaR) theta=theta-alpha*dt return loss_list,theta train_x, test_x, train_y, test_y=data_process() loss_list01,theta=train_mode(train_x,train_y) print('\迭代过程中的损失值:',loss_list01) plt.plot(loss_list01,c='r') plt.show() # 用所得模型对测试集的数据进行预测,并计算准确率 test_z = lh(test_x,theta) test_h = sigmoid(test_z) print('测试精度:',acc_func(test_h,test_y)) # 使用训练集的X1,X2两组特征画出0-1分布散点图及分割线 m,n = train_x.shape for i in range(m): if train_y[i] == 0: plt.plot(train_x[i,1],train_x[i,2],'ob') elif train_y[i] == 1: plt.plot(train_x[i,1],train_x[i,2],'*r') x1_min=np.min(train_x[:,1],axis=0) x1_max=np.max(train_x[:,1],axis=0) x2_min=(-theta[0]-theta[1]*x1_min)/theta[2] x2_max=(-theta[0]-theta[1]*x1_max)/theta[2] plt.plot([x1_min,x1_max],[x2_min,x2_max],'m') plt.xlabel('x1') plt.ylabel('x2') plt.show()
逻辑回归正则化(# 使用训练集的X1,X2两组特征画出0-1分布散点图及分割线m,n = train_x.shape)
于 2022-06-06 10:59:59 首次发布
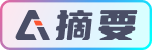