多层感知机
ReLu的存在,是为了调节X平方或者高次方前边的系数,组合起来更贴近你想要的那条曲线用的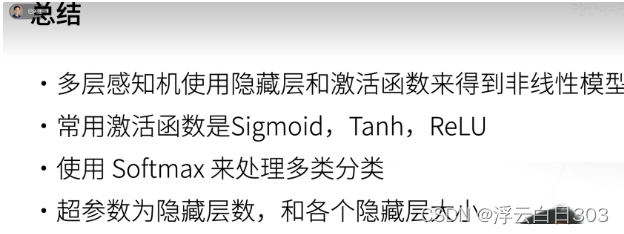
多层感知机的简洁实现
##多层感知机的从零实现
import torch
from torch import nn
from d2l import torch as d2l
batch =256
train,test = d2l.load_data_fashion_mnist(batch)
#单隐藏层感知机
hide = 256
input = 784
output = 10
w1= nn.Parameter(torch.randn(input,hide,requires_grad=True))
b1 = nn.Parameter(torch.zeros(hide,requires_grad=True))
w2 = nn.Parameter(torch.randn(