第十二章深度学习
import numpy as np
import matplotlib.pyplot as plt
from matplotlib.font_manager import FontProperties
fonts=FontProperties(fname="/Library/Fonts/华文细黑.ttf",size=14)
import seaborn as sns
from skimage.io import imread
from skimage.color import rgb2gray
from sklearn.model_selection import train_test_split
from sklearn.preprocessing import OneHotEncoder
from sklearn import metrics
import tensorflow as tf
import tensorflow.compat.v1 as tf
tf.disable_eager_execution()
import os
path_img=os.path.join(os.getcwd(),"data","chap12","莱娜.tiff")
im=imread(path_img)
imggray=rgb2gray(im)
plt.figure(figsize=(6,6))
plt.imshow(imggray,cmap=plt.cm.gray)
plt.axis("off")
plt.show()
print(imggray.shape)
imggray=tf.convert_to_tensor(imggray,dtype=np.float32)
im_height,im_width=imggray.shape
input_im=tf.reshape(imggray,[1,im_height,im_width,1],name="image")
ker=tf.constant([[-1,-1,-1],[-1,8,-1],[-1,-1,-1]],dtype=tf.float32,name="ker")
kernel=tf.reshape(ker,[3,3,1,1],name="kernel")
res=tf.squeeze(tf.nn.conv2d(input_im,kernel,strides=[1,1,1,1],padding="SAME"))
with tf.Session() as sess:
conv_im1=sess.run(res)
print("卷积后图像的大小",conv_im1.shape)
plt.figure(figsize=(6,6))
plt.imshow(conv_im1,cmap=plt.cm.gray)
plt.axis("off")
plt.show()
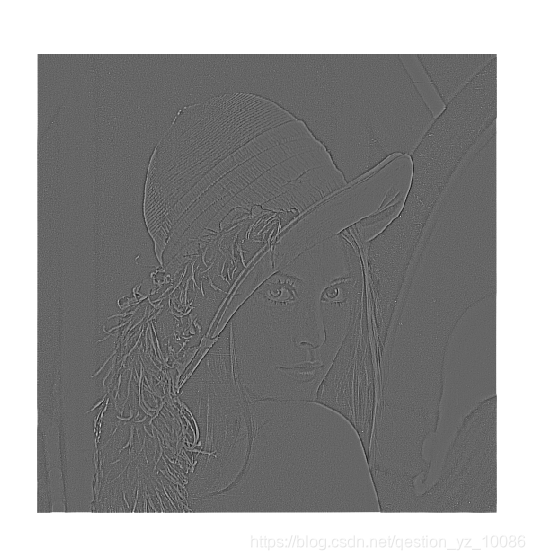
import numpy as np
import matplotlib.pyplot as plt
from matplotlib.font_manager import FontProperties
fonts=FontProperties(fname="/Library/Fonts/华文细黑.ttf",size=14)
import seaborn as sns
from skimage.io import imread
from skimage.color import rgb2gray
from sklearn.model_selection import train_test_split
from sklearn.preprocessing import OneHotEncoder
from sklearn import metrics
import tensorflow as tf
import tensorflow.compat.v1 as tf
tf.disable_eager_execution()
import os
path_img=os.path.join(os.getcwd(),"data","chap12","莱娜.tiff")
im=imread(path_img)
imggray=rgb2gray(im)
plt.figure(figsize=(6,6))
plt.imshow(imggray,cmap=plt.cm.gray)
plt.axis("off")
plt.show()
print(imggray.shape)
imggray=tf.convert_to_tensor(imggray,dtype=np.float32)
im_height,im_width=imggray.shape
input_im=tf.reshape(imggray,[1,im_height,im_width,1],name="image")
ker=tf.constant([[-1,-1,-1],[-1,8,-1],[-1,-1,-1]],dtype=tf.float32,name="ker")
kernel=tf.reshape(ker,[3,3,1,1],name="kernel")
res=tf.squeeze(tf.nn.conv2d(input_im,kernel,strides=[1,1,1,1],padding="SAME"))
with tf.Session() as sess:
conv_im1=sess.run(res)
print("卷积后图像的大小",conv_im1.shape)
plt.figure(figsize=(6,6))
plt.imshow(conv_im1,cmap=plt.cm.gray)
plt.axis("off")
plt.show()
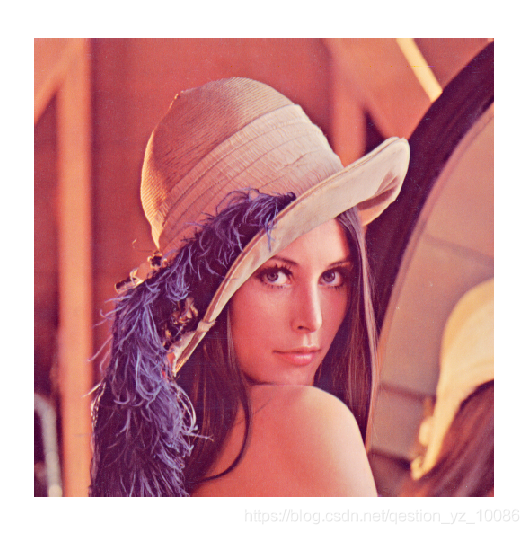
import numpy as np
import matplotlib.pyplot as plt
from matplotlib.font_manager import FontProperties
fonts=FontProperties(fname="/Library/Fonts/华文细黑.ttf",size=14)
import seaborn as sns
from skimage.io import imread
from skimage.color import rgb2gray
from sklearn.model_selection import train_test_split
from sklearn.preprocessing import OneHotEncoder
from sklearn import metrics
import tensorflow as tf
import tensorflow.compat.v1 as tf
tf.disable_eager_execution()
import os
path_img=os.path.join(os.getcwd(),"data","chap12","莱娜.tiff")
im=imread(path_img)
imggray=rgb2gray(im)
plt.figure(figsize=(6,6))
plt.imshow(imggray,cmap=plt.cm.gray)
plt.axis("off")
plt.show()
print(imggray.shape)
imggray=tf.convert_to_tensor(imggray,dtype=np.float32)
im_height,im_width=imggray.shape
input_im=tf.reshape(imggray,[1,im_height,im_width,1],name="image")
ker=tf.constant([[-1,-1,-1],[-1,8,-1],[-1,-1,-1]],dtype=tf.float32,name="ker")
kernel=tf.reshape(ker,[3,3,1,1],name="kernel")
res=tf.squeeze(tf.nn.conv2d(input_im,kernel,strides=[1,1,1,1],padding="SAME"))
with tf.Session() as sess:
conv_im1=sess.run(res)
print("卷积后图像的大小",conv_im1.shape)
plt.figure(figsize=(6,6))
plt.imshow(conv_im1,cmap=plt.cm.gray)
plt.axis("off")
plt.show()
im=imread(path_img)
plt.figure(figsize=(6,6))
plt.imshow(im)
plt.axis("off")
plt.show()
im=tf.convert_to_tensor(im,dtype=np.float32)
im_height,im_width,im_channels=im.shape
input_im=tf.reshape(im,[1,im_height,im_width,im_channels])
ker=tf.constant([[[[-1,-1,-1],[-1,8,-1],[-1,-1,-1]],
[[-1,-1,-1],[-1,8,-1],[-1,-1,-1]],
[[-1,-1,-1],[-1,8,-1],[-1,-1,-1]],
[[-1,-1,-1],[-1,8,-1],[-1,-1,-1]],
[[-1,-1,-1],[-1,8,-1],[-1,-1,-1]],
[[-1,-1,-1],[-1,8,-1],[-1,-1,-1]]]],dtype=tf.float32,name="ker")
print(ker)
kernel=tf.reshape(ker,[3,3,3,2],name="kernel")
res=tf.nn.conv2d(input_im,kernel,strides=[1,2,2,1],padding="VALID")
with tf.Session() as sess:
conv_im2=sess.run(res)
print("卷积后输出结果大小:",conv_im2.shape)
plt.figure(figsize=(8,4))
plt.subplot(1,2,1)
plt.imshow(conv_im2[0][:,:,0],cmap=plt.cm.gray)
plt.axis("off")
plt.subplot(1,2,2)
plt.imshow(conv_im2[0][:,:,1],cmap=plt.cm.gray)
plt.axis("off")
plt.show()
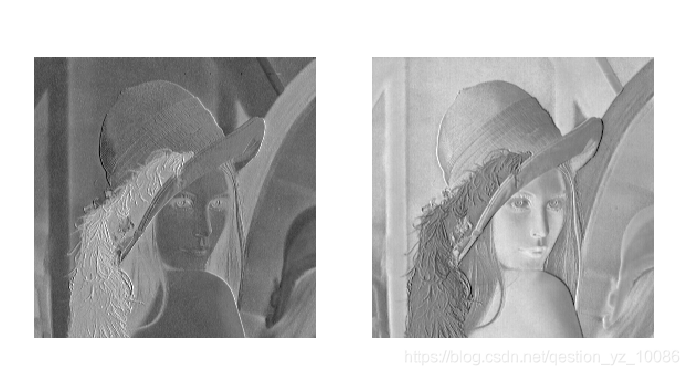
import numpy as np
import matplotlib.pyplot as plt
from matplotlib.font_manager import FontProperties
fonts=FontProperties(fname="/Library/Fonts/华文细黑.ttf",size=14)
import seaborn as sns
from skimage.io import imread
from skimage.color import rgb2gray
from sklearn.model_selection import train_test_split
from sklearn.preprocessing import OneHotEncoder
from sklearn import metrics
import tensorflow as tf
import tensorflow.compat.v1 as tf
tf.disable_eager_execution()
import os
path_img=os.path.join(os.getcwd(),"data","chap12","莱娜.tiff")
im=imread(path_img)
imggray=rgb2gray(im)
plt.figure(figsize=(6,6))
plt.imshow(imggray,cmap=plt.cm.gray)
plt.axis("off")
plt.show()
print(imggray.shape)
imggray=tf.convert_to_tensor(imggray,dtype=np.float32)
im_height,im_width=imggray.shape
input_im=tf.reshape(imggray,[1,im_height,im_width,1],name="image")
ker=tf.constant([[-1,-1,-1],[-1,8,-1],[-1,-1,-1]],dtype=tf.float32,name="ker")
kernel=tf.reshape(ker,[3,3,1,1],name="kernel")
res=tf.squeeze(tf.nn.conv2d(input_im,kernel,strides=[1,1,1,1],padding="SAME"))
with tf.Session() as sess:
conv_im1=sess.run(res)
print("卷积后图像的大小",conv_im1.shape)
plt.figure(figsize=(6,6))
plt.imshow(conv_im1,cmap=plt.cm.gray)
plt.axis("off")
plt.show()
im=imread(path_img)
plt.figure(figsize=(6,6))
plt.imshow(im)
plt.axis("off")
plt.show()
im=tf.convert_to_tensor(im,dtype=np.float32)
im_height,im_width,im_channels=im.shape
input_im=tf.reshape(im,[1,im_height,im_width,im_channels])
ker=tf.constant([[[[-1,-1,-1],[-1,8,-1],[-1,-1,-1]],
[[-1,-1,-1],[-1,8,-1],[-1,-1,-1]],
[[-1,-1,-1],[-1,8,-1],[-1,-1,-1]],
[[-1,-1,-1],[-1,8,-1],[-1,-1,-1]],
[[-1,-1,-1],[-1,8,-1],[-1,-1,-1]],
[[-1,-1,-1],[-1,8,-1],[-1,-1,-1]]]],dtype=tf.float32,name="ker")
print(ker)
kernel=tf.reshape(ker,[3,3,3,2],name="kernel")
res=tf.nn.conv2d(input_im,kernel,strides=[1,2,2,1],padding="VALID")
with tf.Session() as sess:
conv_im2=sess.run(res)
print("卷积后输出结果大小:",conv_im2.shape)
plt.figure(figsize=(8,4))
plt.subplot(1,2,1)
plt.imshow(conv_im2[0][:,:,0],cmap=plt.cm.gray)
plt.axis("off")
plt.subplot(1,2,2)
plt.imshow(conv_im2[0][:,:,1],cmap=plt.cm.gray)
plt.axis("off")
plt.show()
'''池化'''
max_pool=tf.nn.max_pool(conv_im2,ksize=[1,2,2,1],strides=[1,1,1,1],padding="VALID")
with tf.Session() as sess:
max_pool_val=sess.run(max_pool)
print("池化后输出结果大小:",max_pool_val.shape)
plt.figure(figsize=(8,4))
plt.subplot(1,2,1)
plt.imshow(max_pool_val[0][:,:,0],cmap=plt.cm.gray)
plt.axis("off")
plt.subplot(1,2,2)
plt.imshow(max_pool_val[0][:,:,1],cmap=plt.cm.gray)
plt.axis("off")
plt.show()
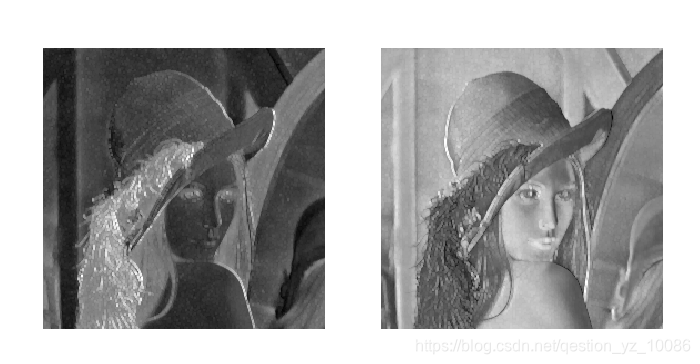
import numpy as np
import matplotlib.pyplot as plt
from matplotlib.font_manager import FontProperties
fonts=FontProperties(fname="/Library/Fonts/华文细黑.ttf",size=14)
import seaborn as sns
from skimage.io import imread
from skimage.color import rgb2gray
from sklearn.model_selection import train_test_split
from sklearn.preprocessing import OneHotEncoder
from sklearn import metrics
import tensorflow as tf
import tensorflow.compat.v1 as tf
tf.disable_eager_execution()
import os
path_img=os.path.join(os.getcwd(),"data","chap12","莱娜.tiff")
im=imread(path_img)
imggray=rgb2gray(im)
plt.figure(figsize=(6,6))
plt.imshow(imggray,cmap=plt.cm.gray)
plt.axis("off")
plt.show()
print(imggray.shape)
imggray=tf.convert_to_tensor(imggray,dtype=np.float32)
im_height,im_width=imggray.shape
input_im=tf.reshape(imggray,[1,im_height,im_width,1],name="image")
ker=tf.constant([[-1,-1,-1],[-1,8,-1],[-1,-1,-1]],dtype=tf.float32,name="ker")
kernel=tf.reshape(ker,[3,3,1,1],name="kernel")
res=tf.squeeze(tf.nn.conv2d(input_im,kernel,strides=[1,1,1,1],padding="SAME"))
with tf.Session() as sess:
conv_im1=sess.run(res)
print("卷积后图像的大小",conv_im1.shape)
plt.figure(figsize=(6,6))
plt.imshow(conv_im1,cmap=plt.cm.gray)
plt.axis("off")
plt.show()
im=imread(path_img)
plt.figure(figsize=(6,6))
plt.imshow(im)
plt.axis("off")
plt.show()
im=tf.convert_to_tensor(im,dtype=np.float32)
im_height,im_width,im_channels=im.shape
input_im=tf.reshape(im,[1,im_height,im_width,im_channels])
ker=tf.constant([[[[-1,-1,-1],[-1,8,-1],[-1,-1,-1]],
[[-1,-1,-1],[-1,8,-1],[-1,-1,-1]],
[[-1,-1,-1],[-1,8,-1],[-1,-1,-1]],
[[-1,-1,-1],[-1,8,-1],[-1,-1,-1]],
[[-1,-1,-1],[-1,8,-1],[-1,-1,-1]],
[[-1,-1,-1],[-1,8,-1],[-1,-1,-1]]]],dtype=tf.float32,name="ker")
print(ker)
kernel=tf.reshape(ker,[3,3,3,2],name="kernel")
res=tf.nn.conv2d(input_im,kernel,strides=[1,2,2,1],padding="VALID")
with tf.Session() as sess:
conv_im2=sess.run(res)
print("卷积后输出结果大小:",conv_im2.shape)
plt.figure(figsize=(8,4))
plt.subplot(1,2,1)
plt.imshow(conv_im2[0][:,:,0],cmap=plt.cm.gray)
plt.axis("off")
plt.subplot(1,2,2)
plt.imshow(conv_im2[0][:,:,1],cmap=plt.cm.gray)
plt.axis("off")
plt.show()
'''池化'''
max_pool=tf.nn.max_pool(conv_im2,ksize=[1,2,2,1],strides=[1,1,1,1],padding="VALID")
with tf.Session() as sess:
max_pool_val=sess.run(max_pool)
print("池化后输出结果大小:",max_pool_val.shape)
plt.figure(figsize=(8,4))
plt.subplot(1,2,1)
plt.imshow(max_pool_val[0][:,:,0],cmap=plt.cm.gray)
plt.axis("off")
plt.subplot(1,2,2)
plt.imshow(max_pool_val[0][:,:,1],cmap=plt.cm.gray)
plt.axis("off")
plt.show()
avg_pool=tf.nn.avg_pool(conv_im2,ksize=[1,3,3,1],strides=[1,2,2,1],padding="VALID")
with tf.Session() as sess:
avg_pool_val=sess.run(avg_pool)
print("池化后输出的结果大小:",avg_pool_val.shape)
plt.figure(figsize=(8,4))
plt.subplot(1,2,1)
plt.imshow(avg_pool_val[0][:,:,0],cmap=plt.cm.gray)
plt.axis("off")
plt.subplot(1,2,2)
plt.imshow(avg_pool_val[0][:,:,1],cmap=plt.cm.gray)
plt.axis("off")
plt.show()
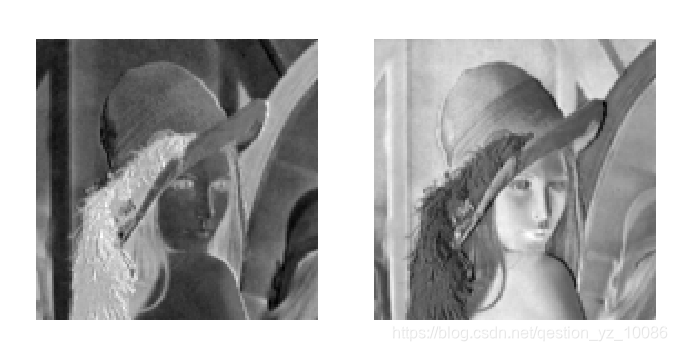