代码及分析
#避免不必要的警告
import warnings
warnings.filterwarnings("ignore")
#初步导包
import numpy as np
import matplotlib.pyplot as plt
import matplotlib.cm as cm
%matplotlib inline
#将绘图嵌入jupyter notebook
#从keras库导入mnist数据集
from keras.datasets import mnist
#对数据进行训练集和测试集的分类
(X_train,y_train),(X_test,y_test)=mnist.load_data()
#打印样本信息
print('训练集中共有{}个样本'.format(len(X_train)))
print('测试集中共有{}个样本'.format(len(X_test)))
print(X_train.shape)
print(y_train.shape)
print(X_test.shape)
print(y_test.shape)
训练集中共有60000个样本
测试集中共有10000个样本
(60000, 28, 28)
(60000,)
(10000, 28, 28)
(10000,)
plt.rcParams['font.sans-serif']=['SimHei']#字体
plt.rcParams['axes.unicode_minus']=False#负号
#打印出图片及其对应的真实的标签(黑白款式)
for i in range(16):
plt.style.use({'figure.figsize':(12,12)})
plt.subplot(1,4,i%4+1)
plt.imshow(X_train[i],cmap='gray')
title='The true label:{}'.format(str(y_train[i]))
plt.title(title)
plt.xticks([])
plt.yticks([])
plt.axis('off')
if i%4 == 3:
plt.show()
#计算机识别图像是以像素点的形式,每个像素由0-255灰度构成,绘制其热力图(黑白款)
def visualize_input(img,ax):
ax.imshow(img,cmap='gray')
width,height=img.shape
thresh=img.max()/2.5
for x in range(width):
for y in range(height):
ax.annotate(str(round(img[x][y],2)),xy=(y,x),
horizontalalignment='center',
verticalalignment='center',
color='white' if img[x][y]<thresh else 'black')
#打印索引值为53对应的数字图像
i=53
fig=plt.figure(figsize=(10,10))
ax=fig.add_subplot(111)
visualize_input(X_train[i],ax)
.
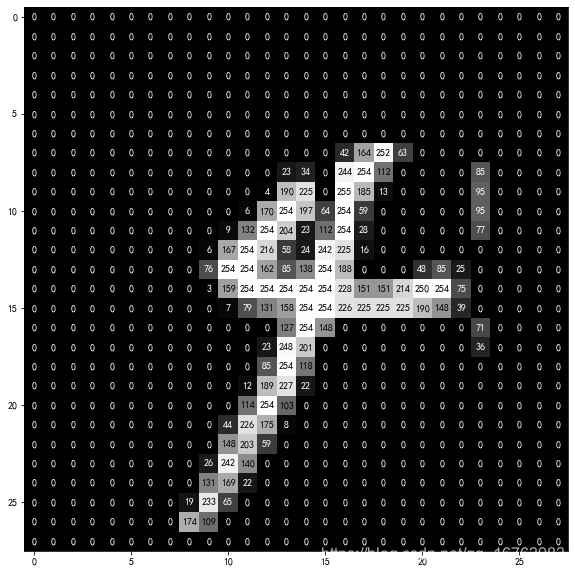
#预处理的归一化处理,将0-255化为0-1的数值
X_train=X_train.astype('float32')/255
X_test=X_test.astype('float32')/255
#打印训练集中某个元素
print(X_train[0])
[[0. 0. 0. 0. 0. 0.
0. 0. 0. 0. 0. 0.
0. 0. 0. 0. 0. 0.
0. 0. 0. 0. 0. 0.
0. 0. 0. 0. ]
[0. 0. 0. 0. 0. 0.
0. 0. 0. 0. 0. 0.
0. 0. 0. 0. 0. 0.
0. 0. 0. 0. 0. 0.
0. 0. 0. 0. ]
[0. 0. 0. 0. 0. 0.
0. 0. 0. 0. 0. 0.
0. 0. 0. 0. 0. 0.
0. 0. 0. 0. 0. 0.
0. 0. 0. 0. ]
[0. 0. 0. 0. 0. 0.
0. 0. 0. 0. 0. 0.
0. 0. 0. 0. 0. 0.
0. 0. 0. 0. 0. 0.
0. 0. 0. 0. ]
[0. 0. 0. 0. 0. 0.
0. 0. 0. 0. 0. 0.
0. 0. 0. 0. 0. 0.
0. 0. 0. 0. 0. 0.
0. 0. 0. 0. ]
[0. 0. 0. 0. 0. 0.
0. 0. 0. 0. 0. 0.
0.01176471 0.07058824 0.07058824 0.07058824 0.49411765 0.53333336
0.6862745 0.10196079 0.6509804 1. 0.96862745 0.49803922
0. 0. 0. 0. ]
[0. 0. 0. 0. 0. 0.
0. 0. 0.11764706 0.14117648 0.36862746 0.6039216
0.6666667 0.99215686 0.99215686 0.99215686 0.99215686 0.99215686
0.88235295 0.6745098 0.99215686 0.9490196 0.7647059 0.2509804
0. 0. 0. 0. ]
[0. 0. 0. 0. 0. 0.
0. 0.19215687 0.93333334 0.99215686 0.99215686 0.99215686
0.99215686 0.99215686 0.99215686 0.99215686 0.99215686 0.9843137
0.3647059 0.32156864 0.32156864 0.21960784 0.15294118 0.
0. 0. 0. 0. ]
[0. 0. 0. 0. 0. 0.
0. 0.07058824 0.85882354 0.99215686 0.99215686 0.99215686
0.99215686 0.99215686 0.7764706 0.7137255 0.96862745 0.94509804
0. 0. 0. 0. 0. 0.
0. 0. 0. 0. ]
[0. 0. 0. 0. 0. 0.
0. 0. 0.3137255 0.6117647 0.41960785 0.99215686
0.99215686 0.8039216 0.04313726 0. 0.16862746 0.6039216
0. 0. 0. 0. 0. 0.
0. 0. 0. 0. ]
[0. 0. 0. 0. 0. 0.
0. 0. 0. 0.05490196 0.00392157 0.6039216
0.99215686 0.3529412 0. 0. 0. 0.
0. 0. 0. 0. 0. 0.
0. 0. 0. 0. ]
[0. 0. 0. 0. 0. 0.
0. 0. 0. 0. 0. 0.54509807
0.99215686 0.74509805 0.00784314 0. 0. 0.
0. 0. 0. 0. 0. 0.
0. 0. 0. 0. ]
[0. 0. 0. 0. 0. 0.
0. 0. 0. 0. 0. 0.04313726
0.74509805 0.99215686 0.27450982 0. 0. 0.
0. 0. 0. 0. 0. 0.
0. 0. 0. 0. ]
[0. 0. 0. 0. 0. 0.
0. 0. 0. 0. 0. 0.
0.13725491 0.94509804 0.88235295 0.627451 0.42352942 0.00392157
0. 0. 0. 0. 0. 0.
0. 0. 0. 0. ]
[0. 0. 0. 0. 0. 0.
0. 0. 0. 0. 0. 0.
0. 0.31764707 0.9411765 0.99215686 0.99215686 0.46666667
0.09803922 0. 0. 0. 0. 0.
0. 0. 0. 0. ]
[0. 0. 0. 0. 0. 0.
0. 0. 0. 0. 0. 0.
0. 0. 0.1764706 0.7294118 0.99215686 0.99215686
0.5882353 0.10588235 0. 0. 0. 0.
0. 0. 0. 0. ]
[0. 0. 0. 0. 0. 0.
0. 0. 0. 0. 0. 0.
0. 0. 0. 0.0627451 0.3647059 0.9882353
0.99215686 0.73333335 0. 0. 0. 0.
0. 0. 0. 0. ]
[0. 0. 0. 0. 0. 0.
0. 0. 0. 0. 0. 0.
0. 0. 0. 0. 0. 0.9764706
0.99215686 0.9764706 0.2509804 0. 0. 0.
0. 0. 0. 0. ]
[0. 0. 0. 0. 0. 0.
0. 0. 0. 0. 0. 0.
0. 0. 0.18039216 0.50980395 0.7176471 0.99215686
0.99215686 0.8117647 0.00784314 0. 0. 0.
0. 0. 0. 0. ]
[0. 0. 0. 0. 0. 0.
0. 0. 0. 0. 0. 0.
0.15294118 0.5803922 0.8980392 0.99215686 0.99215686 0.99215686
0.98039216 0.7137255 0. 0. 0. 0.
0. 0. 0. 0. ]
[0. 0. 0. 0. 0. 0.
0. 0. 0. 0. 0.09411765 0.44705883
0.8666667 0.99215686 0.99215686 0.99215686 0.99215686 0.7882353
0.30588236 0. 0. 0. 0. 0.
0. 0. 0. 0. ]
[0. 0. 0. 0. 0. 0.
0. 0. 0.09019608 0.25882354 0.8352941 0.99215686
0.99215686 0.99215686 0.99215686 0.7764706 0.31764707 0.00784314
0. 0. 0. 0. 0. 0.
0. 0. 0. 0. ]
[0. 0. 0. 0. 0. 0.
0.07058824 0.67058825 0.85882354 0.99215686 0.99215686 0.99215686
0.99215686 0.7647059 0.3137255 0.03529412 0. 0.
0. 0. 0. 0. 0. 0.
0. 0. 0. 0. ]
[0. 0. 0. 0. 0.21568628 0.6745098
0.8862745 0.99215686 0.99215686 0.99215686 0.99215686 0.95686275
0.52156866 0.04313726 0. 0. 0. 0.
0. 0. 0. 0. 0. 0.
0. 0. 0. 0. ]
[0. 0. 0. 0. 0.53333336 0.99215686
0.99215686 0.99215686 0.83137256 0.5294118 0.5176471 0.0627451
0. 0. 0. 0. 0. 0.
0. 0. 0. 0. 0. 0.
0. 0. 0. 0. ]
[0. 0. 0. 0. 0. 0.
0. 0. 0. 0. 0. 0.
0. 0. 0. 0. 0. 0.
0. 0. 0. 0. 0. 0.
0. 0. 0. 0. ]
[0. 0. 0. 0. 0. 0.
0. 0. 0. 0. 0. 0.
0. 0. 0. 0. 0. 0.
0. 0. 0. 0. 0. 0.
0. 0. 0. 0. ]
[0. 0. 0. 0. 0. 0.
0. 0. 0. 0. 0. 0.
0. 0. 0. 0. 0. 0.
0. 0. 0. 0. 0. 0.
0. 0. 0. 0. ]]
#预处理的标签独热向量编码
#编码只是一个序号,没有大小,需要转化为独热向量编码
from keras.utils import np_utils
y_train=np_utils.to_categorical(y_train,10)
y_test=np_utils.to_categorical(y_test,10)
y_train[:10]
array([[0., 0., 0., 0., 0., 1., 0., 0., 0., 0.],
[1., 0., 0., 0., 0., 0., 0., 0., 0., 0.],
[0., 0., 0., 0., 1., 0., 0., 0., 0., 0.],
[0., 1., 0., 0., 0., 0., 0., 0., 0., 0.],
[0., 0., 0., 0., 0., 0., 0., 0., 0., 1.],
[0., 0., 1., 0., 0., 0., 0., 0., 0., 0.],
[0., 1., 0., 0., 0., 0., 0., 0., 0., 0.],
[0., 0., 0., 1., 0., 0., 0., 0., 0., 0.],
[0., 1., 0., 0., 0., 0., 0., 0., 0., 0.],
[0., 0., 0., 0., 1., 0., 0., 0., 0., 0.]], dtype=float32)
from keras.models import Sequential
from keras.layers import Dense,Dropout,Flatten
model=Sequential()
#将28*28拉伸为784的一维长向量(详见后文思考)
model.add(Flatten(input_shape=(28,28)))
#搭建三层神经网络模型
model.add(Dense(512,activation='relu'))
model.add(Dropout(0.2))
model.add(Dense(512,activation='relu'))
model.add(Dropout(0.2))
model.add(Dense(512,activation='relu'))
model.add(Dropout(0.2))
model.add(Dense(10,activation='softmax'))
#在每一层随机掐死20%数据,防止过拟合
model.summary()
Model: "sequential_11"
_________________________________________________________________
Layer (type) Output Shape Param #
=================================================================
flatten_11 (Flatten) (None, 784) 0
_________________________________________________________________
dense_34 (Dense) (None, 512) 401920
_________________________________________________________________
dropout_24 (Dropout) (None, 512) 0
_________________________________________________________________
dense_35 (Dense) (None, 512) 262656
_________________________________________________________________
dropout_25 (Dropout) (None, 512) 0
_________________________________________________________________
dense_36 (Dense) (None, 512) 262656
_________________________________________________________________
dropout_26 (Dropout) (None, 512) 0
_________________________________________________________________
dense_37 (Dense) (None, 10) 5130
=================================================================
Total params: 932,362
Trainable params: 932,362
Non-trainable params: 0
_________________________________________________________________
model.compile(loss='categorical_crossentropy',optimizer='rmsprop',metrics=['accuracy'])
model.evaluate(X_test,y_test,verbose=1)
#未经过训练的精度在10%左右即从10个数中随机选择的概率大小
10000/10000 [==============================] - 1s 62us/step
[2.3300374851226806, 0.0908999964594841]
#果然只有9.09%
#将准确度最高的值存入hdf5文件中
from keras.callbacks import ModelCheckpoint
checkpointer=ModelCheckpoint(filepath='mnist.model.best.hdf5',verbose=1,save_best_only=True)
#将训练集进行划分4:1其中20%用于进行验证,对训练数据进行16轮训练
hist=model.fit(X_train,y_train,batch_size=128,epochs=16,validation_split=0.2,callbacks=[checkpointer],verbose=1,shuffle=True)
Train on 48000 samples, validate on 12000 samples
Epoch 1/16
48000/48000 [==============================] - 7s 149us/step - loss: 0.2951 - accuracy: 0.9085 - val_loss: 0.1216 - val_accuracy: 0.9638
Epoch 00001: val_loss improved from inf to 0.12163, saving model to mnist.model.best.hdf5
Epoch 2/16
48000/48000 [==============================] - 6s 126us/step - loss: 0.1240 - accuracy: 0.9632 - val_loss: 0.1069 - val_accuracy: 0.9706
Epoch 00002: val_loss improved from 0.12163 to 0.10688, saving model to mnist.model.best.hdf5
Epoch 3/16
48000/48000 [==============================] - 6s 118us/step - loss: 0.0920 - accuracy: 0.9721 - val_loss: 0.0980 - val_accuracy: 0.9735
Epoch 00003: val_loss improved from 0.10688 to 0.09795, saving model to mnist.model.best.hdf5
Epoch 4/16
48000/48000 [==============================] - 6s 117us/step - loss: 0.0729 - accuracy: 0.9795 - val_loss: 0.1035 - val_accuracy: 0.9753
Epoch 00004: val_loss did not improve from 0.09795
Epoch 5/16
48000/48000 [==============================] - 6s 119us/step - loss: 0.0657 - accuracy: 0.9814 - val_loss: 0.1062 - val_accuracy: 0.9758
Epoch 00005: val_loss did not improve from 0.09795
Epoch 6/16
48000/48000 [==============================] - 6s 120us/step - loss: 0.0552 - accuracy: 0.9844 - val_loss: 0.1136 - val_accuracy: 0.9779
Epoch 00006: val_loss did not improve from 0.09795
Epoch 7/16
48000/48000 [==============================] - 6s 127us/step - loss: 0.0527 - accuracy: 0.9856 - val_loss: 0.1142 - val_accuracy: 0.9787
Epoch 00007: val_loss did not improve from 0.09795
Epoch 8/16
48000/48000 [==============================] - 6s 120us/step - loss: 0.0460 - accuracy: 0.9877 - val_loss: 0.1206 - val_accuracy: 0.9780
Epoch 00008: val_loss did not improve from 0.09795
Epoch 9/16
48000/48000 [==============================] - 6s 121us/step - loss: 0.0463 - accuracy: 0.9878 - val_loss: 0.1515 - val_accuracy: 0.9736
Epoch 00009: val_loss did not improve from 0.09795
Epoch 10/16
48000/48000 [==============================] - 6s 120us/step - loss: 0.0444 - accuracy: 0.9889 - val_loss: 0.1232 - val_accuracy: 0.9778
Epoch 00010: val_loss did not improve from 0.09795
Epoch 11/16
48000/48000 [==============================] - 6s 117us/step - loss: 0.0412 - accuracy: 0.9892 - val_loss: 0.1682 - val_accuracy: 0.9801
Epoch 00011: val_loss did not improve from 0.09795
Epoch 12/16
48000/48000 [==============================] - 6s 118us/step - loss: 0.0403 - accuracy: 0.9908 - val_loss: 0.1517 - val_accuracy: 0.9811
Epoch 00012: val_loss did not improve from 0.09795
Epoch 13/16
48000/48000 [==============================] - 6s 117us/step - loss: 0.0368 - accuracy: 0.9904 - val_loss: 0.1541 - val_accuracy: 0.9787
Epoch 00013: val_loss did not improve from 0.09795
Epoch 14/16
48000/48000 [==============================] - 6s 121us/step - loss: 0.0396 - accuracy: 0.9904 - val_loss: 0.1681 - val_accuracy: 0.9766
Epoch 00014: val_loss did not improve from 0.09795
Epoch 15/16
48000/48000 [==============================] - 6s 119us/step - loss: 0.0374 - accuracy: 0.9913 - val_loss: 0.1767 - val_accuracy: 0.9799
Epoch 00015: val_loss did not improve from 0.09795
Epoch 16/16
48000/48000 [==============================] - 6s 117us/step - loss: 0.0371 - accuracy: 0.9924 - val_loss: 0.2129 - val_accuracy: 0.9808
Epoch 00016: val_loss did not improve from 0.09795
#从最高的精度文件加载模型
model.load_weights('mnist.model.best.hdf5')
#给模型喂测试集数据
model.evaluate(X_test,y_test,verbose=0)
[0.08776235084307846, 0.9739999771118164]
#绘制出损失值和精确度随着训练加深而变化的图像
def plot_history(network_history):
plt.figure()
plt.xlabel('Epochs')
plt.ylabel('Loss')
plt.plot(network_history.history['loss'])
plt.plot(network_history.history['val_loss'])
plt.legend(['Training','Validation'],loc='lower right')
plt.rcParams['figure.figsize'] = (8.0, 4.0)
plt.show()
plt.figure()
plt.xlabel('Epochs')
plt.ylabel('Accuracy')
plt.plot(network_history.history['accuracy'])
plt.plot(network_history.history['val_accuracy'])
plt.legend(['Training','Validation'],loc='lower right')
plt.rcParams['figure.figsize'] = (8.0, 4.0)
plt.show()
plot_history(hist)
.
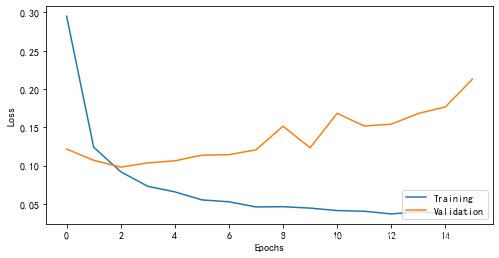
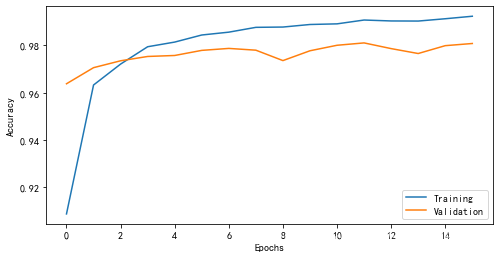
损失值loss(或者说cost也行)和accuracy都随着迭代次数增加逐渐收敛于一个稳定值记录精度最大值,使其读入模型是一个关键点。嗯,没错!
#对索引值为8的数据预测
i=8
plt.imshow(X_test[i])
img_test=X_test[i].reshape(-1,28,28)
prediction=model.predict(img_test)[0]
title='The true label:{}\nThe predicted label:{}'.format(np.argmax(y_test[i]),np.argmax(prediction))
plt.title(title)
plt.rcParams['figure.figsize'] = (8.0, 4.0)
plt.show()
plt.bar(range(10),prediction)
plt.title('The possibility of prediction')
plt.xticks([0,1,2,3,4,5,6,7,8,9])
plt.show()
.
.
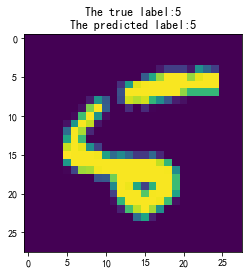
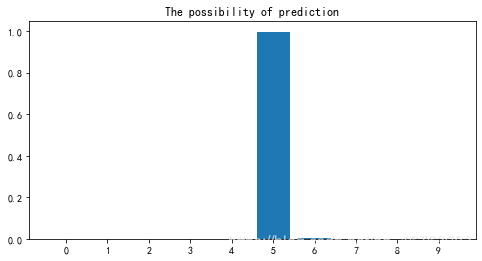
#对索引值为1264的数据进行预测
i=1264
plt.imshow(X_test[i])
img_test=X_test[i].reshape(-1,28,28)
prediction=model.predict(img_test)[0]
title='The true label:{}\nThe predicted label:{}'.format(np.argmax(y_test[i]),np.argmax(prediction))
plt.title(title)
plt.rcParams['figure.figsize'] = (8.0, 4.0)
plt.show()
plt.bar(range(10),prediction)
plt.title('The possibility of prediction')
plt.xticks([0,1,2,3,4,5,6,7,8,9])
plt.show()
.
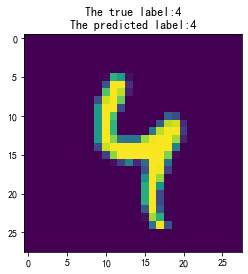
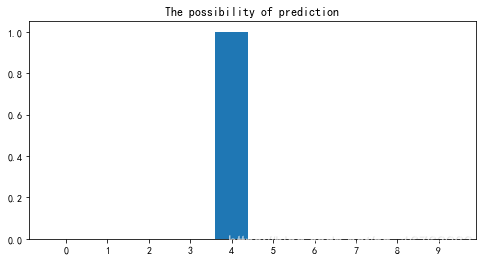
#用tensorflow中的tensorboard对模型进行可视化处理
import tensorflow as tf
writer=tf.summary.FileWriter('./log/', tf.get_default_graph())
writer.close()
#在cmd中输入如下代码可以通过网址 启动web服务器
tensorboard --logdir=./log
可观的思考
全连接神经网络 将28x28的向量展开为784位长向量多层感知机 每一层的神经元都与另一层神经元相连 密集层dense
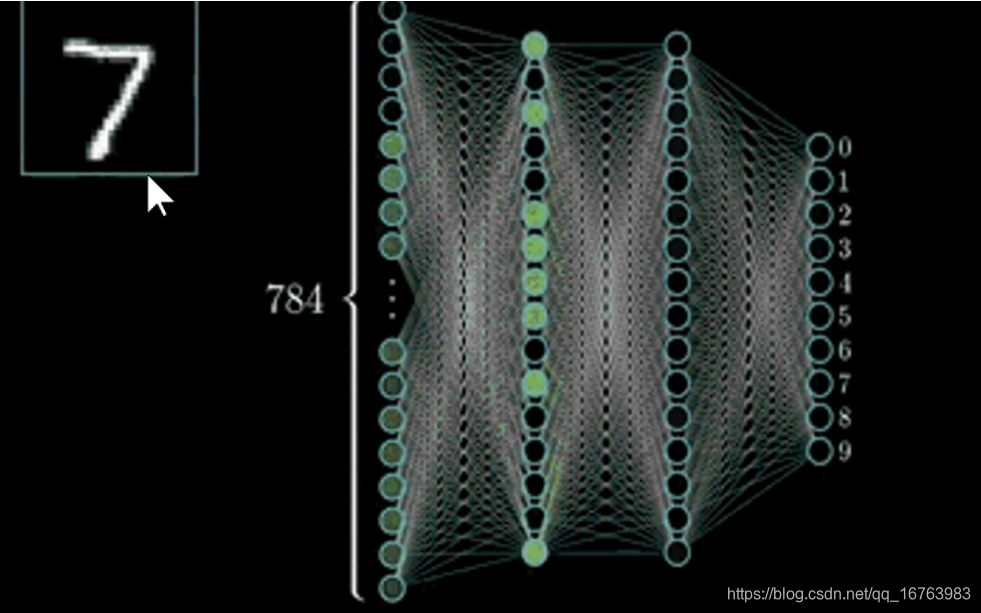
识别原理
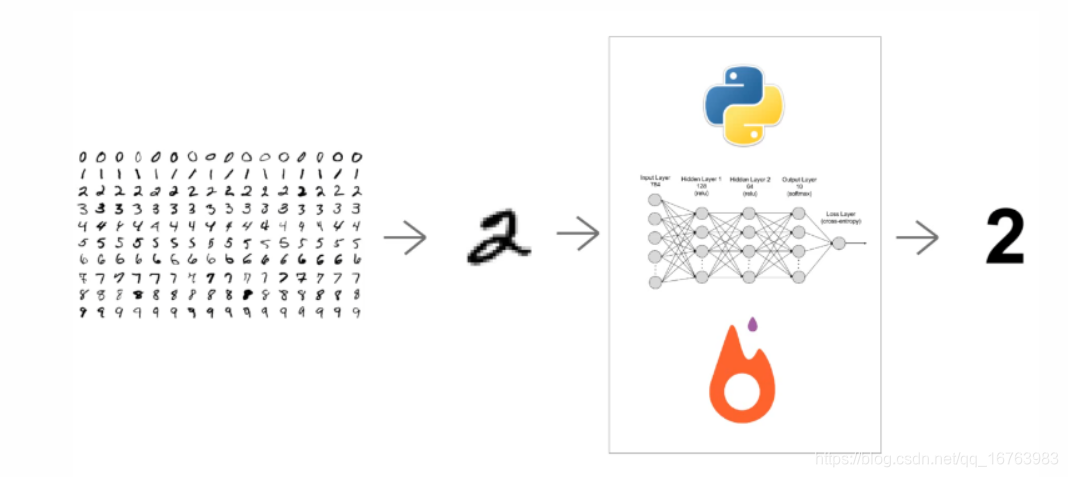
用t-SNE对数据进行非线性降维在三维空间中距离远的在二维空间中远,在三维空间中近的在二维空间近!下图为第50次迭代过程:
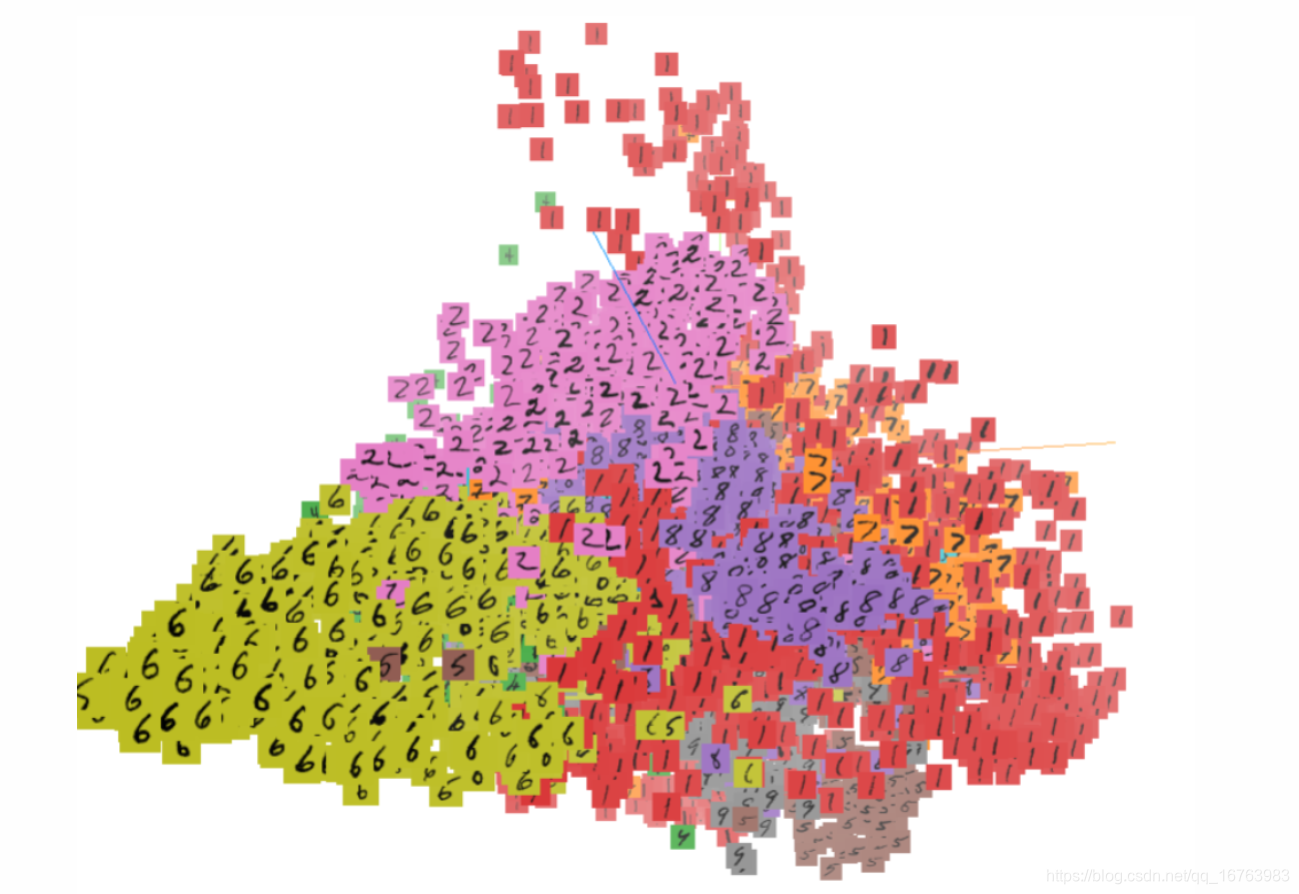
下图为第100次迭代过程:
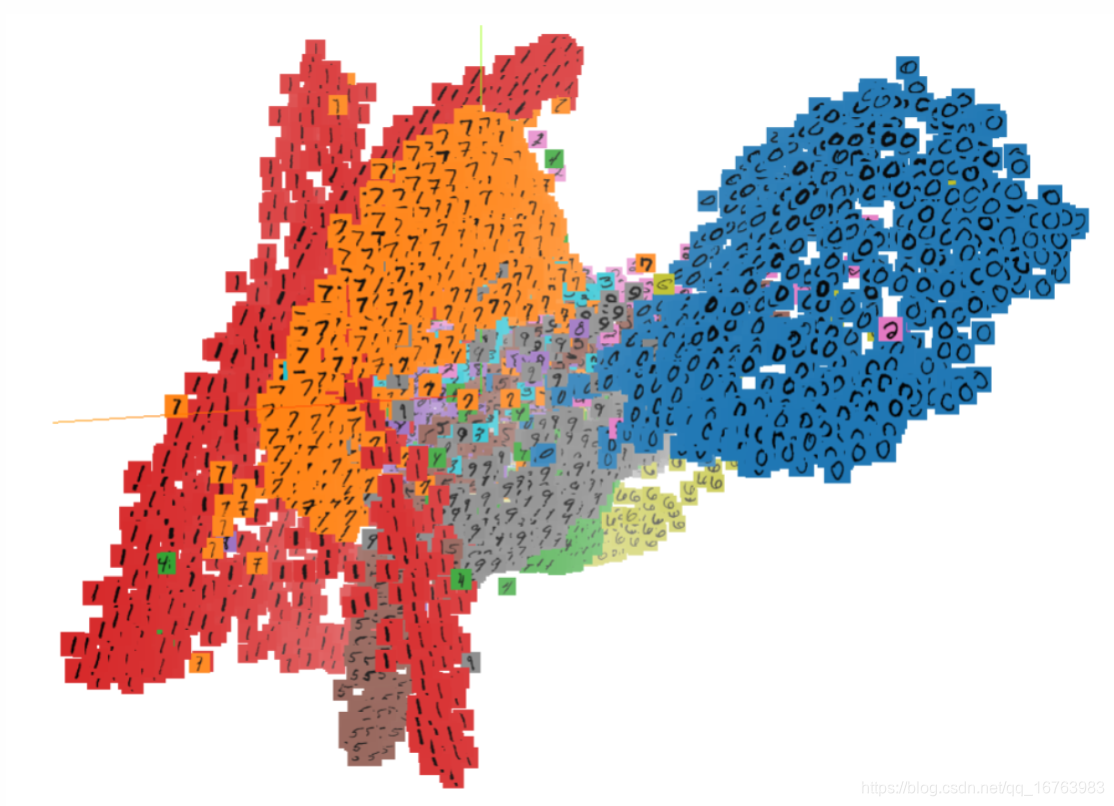
下图为二维空间中进行300余次的迭代过程:
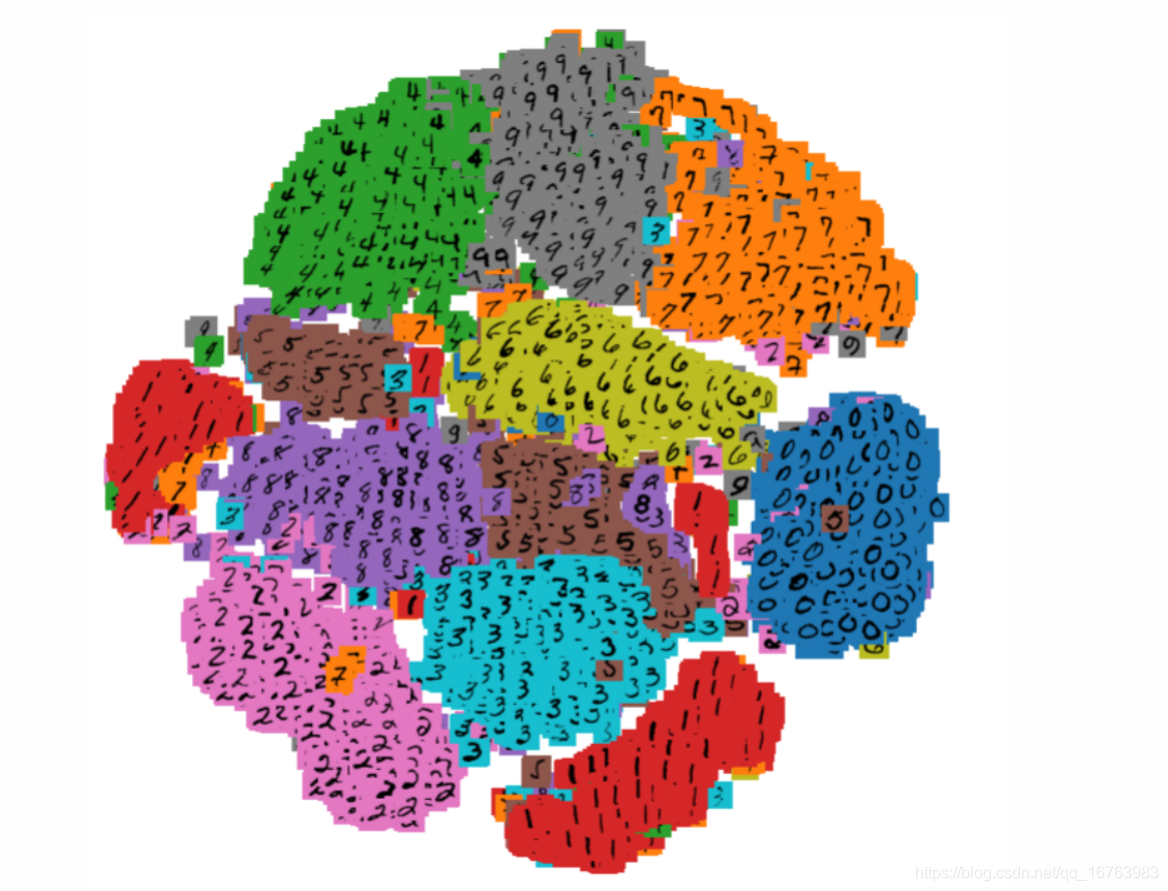
观察可以得到:4 9 7相似度较高 1 7容易混淆…(太多结论,就不多说啦)
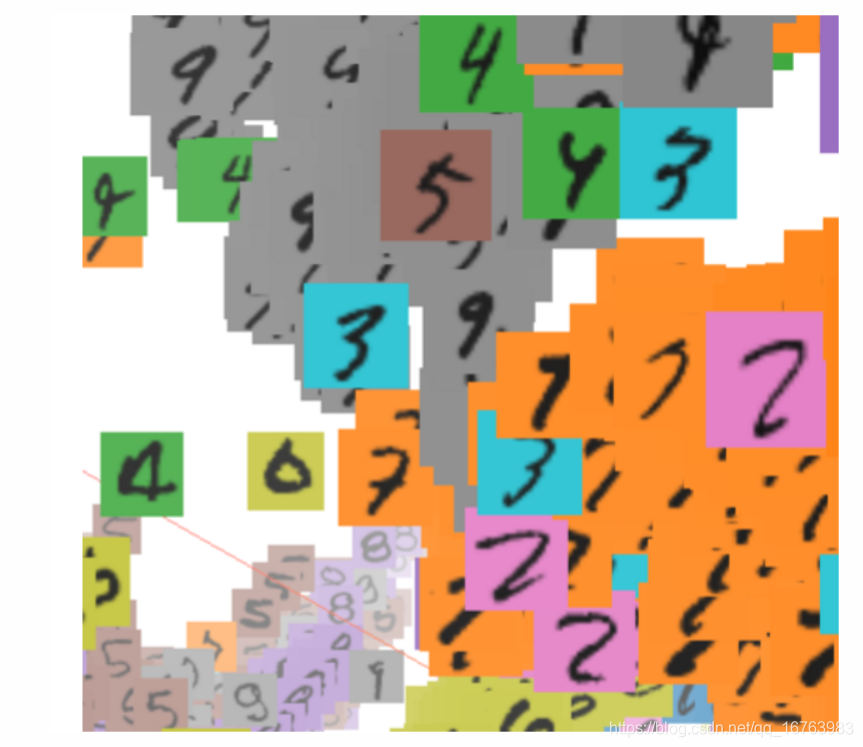
放大图像可以看到在写法上很相似的3 5 7 4及其容易混淆!
TIPS
训练集:高考前的练习册train
验证集:高考前的自主模拟卷validation_split
测试集:高考正式考卷test
数据集:存储具体图片像素的
标签集:存储图片对应标号的
索引:图片对应的一个序号(感觉乱得无关紧要)
关于tensorflow keras的讨论
优点:用了tensorflow keras框架,很方便地导入mnist数据集,并使用keras高度封装的模型进行训。适合入门使用,不需要动手写模型代码的细节,方便对数据进行处理
缺点:框架和模型是静态的,没有较好的可调性和动态性。可以用pytorch对这个过程进行训练,但是在代码上难度更大,需要亲手写很多代码细节所以下一步就是学习通过pytorch来进行模型的训练和预测(不调用keras)…(待续…)
以上图片来自Google高维可视化开源网页工具Embedding Projector
:http://projector.tensorflow.org/
---------------------------------------------------------------------------------------------------- 2020.1.20