http://www.aiskyeye.com/
2018年已经办过一年了。2019年在ICCV上办。
We encourage the participants to use the provided training data for each task, but also allow them to use additional training data. The use of additional training data must be indicated in the "method description" when uploading results to the server.
We emphasize that any form of annotation or use of the VisDrone testing sets for either supervised or unsupervised training is strictly forbidden. The participants are required to explicitly specify any an all external data used for training in the "method description" in submission. In addition, the participants are NOT allowed to train a model in one task using the training or validation sets in other tasks.
we have divided the test set into two splits, including test-challenge and test-dev. Test-dev一天交3次,test-challenge一共交3次。
不明白为什么download里test-dev是不能下载的。。。
Vision Meets Drones: A Challenge
Abstract
our benchmark has more than 2:5 million annotated instances in 179; 264 images/video frames.
Introduction
现状:缺少大的数据集。
Altogether we carefully annotated more than 2:5 million bounding boxes of object instances from these categories. Moreover, some important attributes including visibility of scenes, object category and occlusion, are provided for better data usage.
感觉还是挺大的,也没什么别的数据集可以利用了。
3.2 Task 1: Object Detection in Images
The VisDrone2018 provides a dataset of 10; 209 images for this task, with 6; 471 images used for training, 548 for validation and 3; 190 for testing.
For truncation ratio, it is used to indicate the degree of object parts appears outside a frame. If an object is not fully captured within a frame, we annotate the bounding box across the frame boundary and estimate the truncation ratio based on the region outside the image. It is worth mentioning that a target is skipped during evaluation if its truncation ratio is larger than 50%.
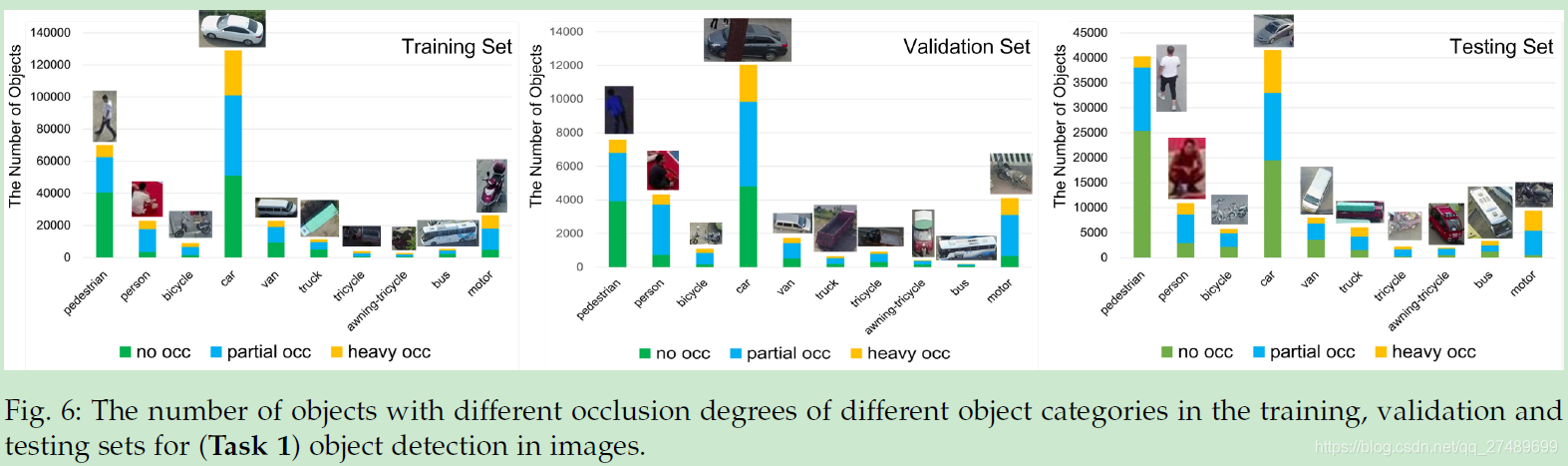
Three degrees of occlusions: no occlusion (occlusion ratio 0%), partial occlusion (occlusion ratio 1% 50%), and heavy occlusion (occlusion ratio > 50%).
2018task1winner
选择retina net,移除后面两层,只用P3,4,5
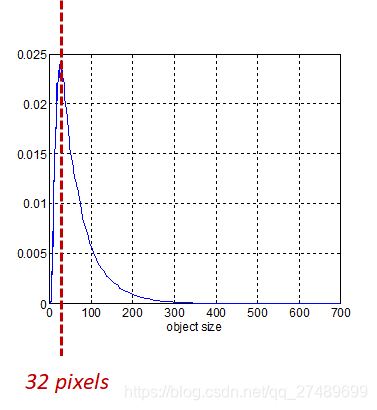
Cascade R-CNN: Delving into High Quality Object Detection
Single-Shot Bidirectional Pyramid Networks for High-Quality Object Detection
数据观察
task1
bbox观察
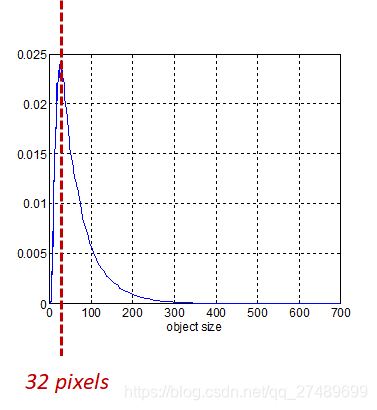
<p2 | p2 | p3 | p4 | p5 | p6 | >p6 | |
train | 48.3% | 30.6% | 16.2% | 4.4% | 0.5% | 0.01% | 0% |
val | 55.3% | 30.8% | 11.4% | 2.4% | 0.1% | 0.007% | 0% |
train_val | 49.0% | 30.6% | 15.7% | 4.1% | 0.5% | 0.01% | 0% |
<p2 | p2 | p3 | p4 | p5 | p6 | >p6 | |
train | 28.2% | 34.6% | 24.5% | 10.5% | 2.0% | 0.1% | 0.002% |
val | 33.0% | 37.6% | 21.8% | 6.9% | 0.7% | 0.03% | 0.005% |
train_val | 28.7% | 34.9% | 24.2% | 10.2% | 1.9% | 0.1% | 0.002% |
<p2 | p2 | p3 | p4 | p5 | p6 | >p6 | |
train | 6.2% | 22.0% | 34.6% | 24.5% | 10.6% | 2.0% | 0.1% |
val | 7.1% | 26.0% | 37.6% | 21.8% | 6.9% | 0.7% | 0.03% |
train_val | 6.3% | 22.4% | 34.9% | 24.2% | 10.2% | 1.9% | 0.1% |
类别分布
0 ignored | 1 pedestrain | 2 people | 3 bicycle | 4 car | 5 van | 6 truck | 7 tricycly | 8 awning-tricycle | 9 bus | 10 motor | 11 others | |
train | 2.49% | 22.44% | 7.65% | 2.96% | 40.97% | 7.06% | 3.64% | 1.36% | 0.92% | 1.68% | 8.39% | 0.43% |
val | 3.43% | 22.02% | 12.76% | 3.24% | 35.01% | 4.92% | 1.87% | 2.60% | 1.32% | 0.62% | 12.16% | 0.08% |
trian_val | 2.59% | 22.40% | 8.17% | 2.99% | 40.37% | 6.84% | 3.46% | 1.49% | 0.96% | 1.57% | 8.77% | 0.40% |
0 ignored | 1 pedestrain | 2 people | 3 bicycle | 4 car | 5 van | 6 truck | 7 tricycly | 8 awning-tricycle | 9 bus | 10 motor | 11 others | |
train | 3.89% | 6.42% | 1.82% | 1.45% | 54.11% | 11.66% | 9.46% | 1.39% | 1.07% | 4.69% | 3.35% | 0.69% |
val | 8.23% | 7.44% | 3.67% | 1.42% | 54.88% | 7.68% | 5.80% | 2.38% | 1.29% | 1.82% | 5.23% | 0.15% |
trian_val | 4.18% | 6.49% | 1.94% | 1.45% | 54.16% | 11.39% | 9.22% | 1.46% | 1.09% | 4.50% | 3.47% | 0.65% |
图片尺寸分布
480×360 | 960×540 | 1344×756 | 1360×765 | 1389×1042 | 1398×1048 | 1400×788 | 1400×1050 | 1916×1078 | 1920×1080 | 2000×1500 | all | |
train | 1 | 250 | 1 | 743 | 1 | 30 | 1299 | 2498 | 537 | 339 | 772 | 6462 |
val | 0 | 121 | 0 | 408 | 0 | 0 | 0 | 0 | 0 | 19 | 0 | 548 |
test | 0 | 64 | 0 | 150 | 0 | 0 | 712 | 491 | 127 | 36 | 0 | 1580 |
train 平均图像大小:1575738.0529247911