从最基础的RNN开始
这里一个方框是一个cell,公式是
对应手动实现代码是
注意这里是dot点积,还需要注意各个维度的含义
n_x:输入向量的维度
m:batch_size
T_x:时间序列长度
这边是(n_x,m,T_x)到了pytorch模型那边不一样
def rnn_cell_forward(xt, a_prev, parameters):
a_next=np.tanh(np.dot(parameters["Wax"],xt)+np.dot(parameters["Waa"],a_prev)+parameters["ba"])
yt_pred=softmax(np.dot(parameters["Wya"],a_next)+parameters["by"])
cache=(a_next,a_prev,xt,parameters)
return a_next,yt_pred,cache
def rnn_forward(x,a0,parameters):
cacahes=[]
n_x,m,T_x=x.shape
n_y,n_a=parameters["Wya"].shape
a=np.zeros((n_a,m,T_x))
y_pred=np.zeros((n_y,m,T_x))
a_next=a0
for t in range(T_x):
a_next,yt_pred,cache=rnn_cell_forward(x[:,:,t],a_next,parameters)
a[:,:,t]=a_next
y_pred[:,:,t]=yt_pred
caches.append(cache)
cacahes=(caches,x)
return a,y_pred,caches
对应的pytorch代码是
x shape (T_x,m,n_x) 如果batch_first=True 那么变成(m,T_x,n_x)
这边的nn.RNN直接完成的是上面整个图而不是单个细胞
在调用rnn_layer时,会把每个输出y和最新的隐藏层状态都记录下来 最后的h是最后时刻的hidden_state
import torch
from torch import nn
vocab_size=10000
num_hiddens=10
rnn_layer = nn.RNN(input_size=vocab_size,hidden_size=num_hiddens,batch_first=True)
num_steps = 4
batch_size = 5
state = None # 初始隐藏层状态可以不定义
X = torch.rand(batch_size,num_steps,vocab_size)
# Y shape (batch, time_step, output_size)
# state_new shape (n_layers, batch, hidden_size)
Y, state_new = rnn_layer(X, state)
print(Y.shape,state_new.shape)
Y的shape是torch.Size([1, 4, 10]) state_new的shape是 torch.Size([1, 5, 10])
题外补充nn.RNN
nn.RNN(input_size, hidden_size, num_layers=1, nonlinearity=tanh, bias=True, batch_first=False, dropout=0, bidirectional=False)
输入X :shape (T_x,m,n_x)
输出Y和state_new :
Y shape (batch, time_step, output_size)
state_new shape (n_layers, batch, hidden_size)
LSTM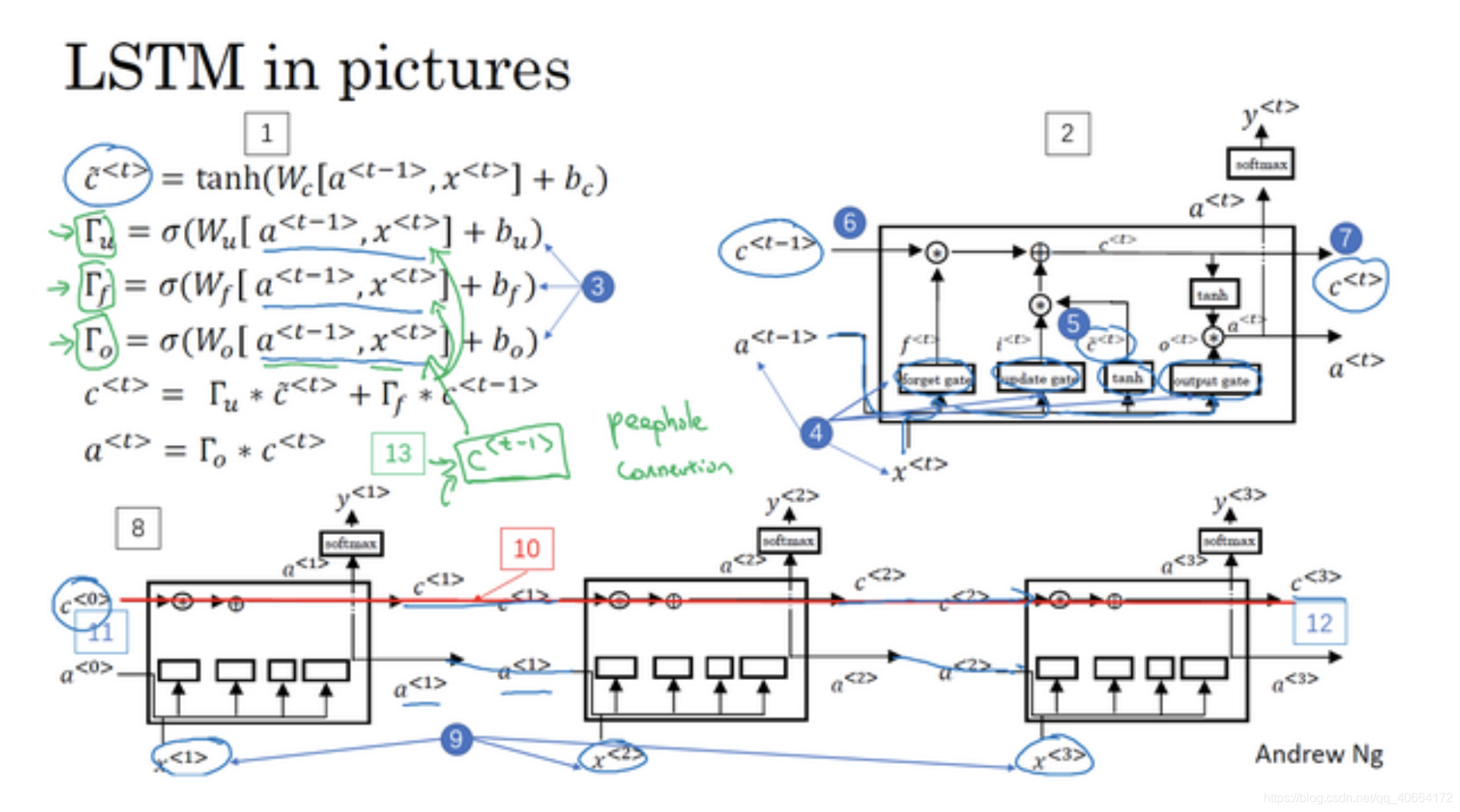
LSTM就是细胞内部结构更加复杂,多了3个门,而且隐藏层状态a不等于c
下面举个具体例子加深对各参数维度理解
每次输入64张 28*28的图片 每个时间步对一行28个像素点进行计算,一共28个时间步
单拿出来r_out, (h_n, h_c) = self.rnn(x, None)
每次会把当前的x一行和隐藏层状态传给下一个细胞进行forward
class RNN(nn.Module):
def __init__(self):
super(RNN, self).__init__()
self.rnn = nn.LSTM( # if use nn.RNN(), it hardly learns
input_size=INPUT_SIZE, #每个时间点input28个像素点
hidden_size=128, # rnn hidden unit
num_layers=1, # number of rnn layer
batch_first=True, # input & output will has batch size as 1s dimension. e.g. (batch, time_step, input_size) 如果是false(time_step, batch, input_size)
)
self.out = nn.Linear(128, 10) #隐藏层n_a和n_y原本都是64,现在我们希望y输出10个维度
def forward(self, x):
# x shape (batch, time_step, input_size)
# r_out shape (batch, time_step, output_size)
# h_n shape (n_layers, batch, hidden_size)
# h_c shape (n_layers, batch, hidden_size)
r_out, (h_n, h_c) = self.rnn(x, None) # None represents zero initial hidden state
# choose r_out at the last time step
out = self.out(r_out[:, -1, :])
return out
再举新闻文本分类的例子
假设句子长度固定为32,多退少补,词向量长度为300
这里面会有两张表 词表(每个词对应一个下标)和embedding表(10000✖️300 将词转换为词向量)
将每句话中的每个词查到下标之后(1✖️32)进行embeding(300✖️32)
梯度修剪(防止梯度爆炸)
def clip(gradients, maxValue):
'''
Clips the gradients' values between minimum and maximum.
Arguments:
gradients -- a dictionary containing the gradients "dWaa", "dWax", "dWya", "db", "dby"
maxValue -- everything above this number is set to this number, and everything less than -maxValue is set to -maxValue
Returns:
gradients -- a dictionary with the clipped gradients.
'''
dWaa, dWax, dWya, db, dby = gradients['dWaa'], gradients['dWax'], gradients['dWya'], gradients['db'], gradients['dby']
### START CODE HERE ###
# clip to mitigate exploding gradients, loop over [dWax, dWaa, dWya, db, dby]. (≈2 lines)
for gradient in [dWax, dWaa, dWya, db, dby]:
np.clip(gradient,-maxValue , maxValue, out=gradient)
### END CODE HERE ###
gradients = {"dWaa": dWaa, "dWax": dWax, "dWya": dWya, "db": db, "dby": dby}
return gradients
采样
采样就是利用训练好的参数生成新的数据
def sample(parameters, char_to_ix, seed):
"""
Sample a sequence of characters according to a sequence of probability distributions output of the RNN
Arguments:
parameters -- python dictionary containing the parameters Waa, Wax, Wya, by, and b.
char_to_ix -- python dictionary mapping each character to an index.
seed -- used for grading purposes. Do not worry about it.
Returns:
indices -- a list of length n containing the indices of the sampled characters.
"""
# Retrieve parameters and relevant shapes from "parameters" dictionary
Waa, Wax, Wya, by, b = parameters['Waa'], parameters['Wax'], parameters['Wya'], parameters['by'], parameters['b']
vocab_size = by.shape[0]
n_a = Waa.shape[1]
### START CODE HERE ###
# Step 1: Create the one-hot vector x for the first character (initializing the sequence generation). (≈1 line)
x = np.zeros((vocab_size,1))
# Step 1': Initialize a_prev as zeros (≈1 line)
a_prev = np.zeros((n_a,1))
# Create an empty list of indices, this is the list which will contain the list of indices of the characters to generate (≈1 line)
indices = []
# Idx is a flag to detect a newline character, we initialize it to -1
idx = -1
# Loop over time-steps t. At each time-step, sample a character from a probability distribution and append
# its index to "indices". We'll stop if we reach 50 characters (which should be very unlikely with a well
# trained model), which helps debugging and prevents entering an infinite loop.
counter = 0
newline_character = char_to_ix['\n']
while (idx != newline_character and counter != 50):
# Step 2: Forward propagate x using the equations (1), (2) and (3)
a = np.tanh(np.dot(Wax,x)+np.dot(Waa,a_prev)+b)
z = np.dot(Wya,a)+by
y = softmax(z)
# for grading purposes
np.random.seed(counter+seed)
# Step 3: Sample the index of a character within the vocabulary from the probability distribution y
idx = np.random.choice(range(len(y)),p=y.ravel())
# Append the index to "indices"
indices.append(idx)
# Step 4: Overwrite the input character as the one corresponding to the sampled index.
x = np.zeros((vocab_size,1))
x[idx] = 1
# Update "a_prev" to be "a"
a_prev = a
# for grading purposes
seed += 1
counter +=1
### END CODE HERE ###
if (counter == 50):
indices.append(char_to_ix['\n'])
return indices
训练一次参数的过程
这边用Y是X的下一时刻,用于rnn_forward的时候和y_hat计算loss
def optimize(X, Y, a_prev, parameters, learning_rate = 0.01):
"""
Execute one step of the optimization to train the model.
Arguments:
X -- list of integers, where each integer is a number that maps to a character in the vocabulary.
Y -- list of integers, exactly the same as X but shifted one index to the left.
a_prev -- previous hidden state.
parameters -- python dictionary containing:
Wax -- Weight matrix multiplying the input, numpy array of shape (n_a, n_x)
Waa -- Weight matrix multiplying the hidden state, numpy array of shape (n_a, n_a)
Wya -- Weight matrix relating the hidden-state to the output, numpy array of shape (n_y, n_a)
b -- Bias, numpy array of shape (n_a, 1)
by -- Bias relating the hidden-state to the output, numpy array of shape (n_y, 1)
learning_rate -- learning rate for the model.
Returns:
loss -- value of the loss function (cross-entropy)
gradients -- python dictionary containing:
dWax -- Gradients of input-to-hidden weights, of shape (n_a, n_x)
dWaa -- Gradients of hidden-to-hidden weights, of shape (n_a, n_a)
dWya -- Gradients of hidden-to-output weights, of shape (n_y, n_a)
db -- Gradients of bias vector, of shape (n_a, 1)
dby -- Gradients of output bias vector, of shape (n_y, 1)
a[len(X)-1] -- the last hidden state, of shape (n_a, 1)
"""
### START CODE HERE ###
# Forward propagate through time (≈1 line)
loss, cache = rnn_forward(X,Y,a_prev,parameters)
# Backpropagate through time (≈1 line)
gradients, a = rnn_backward(X,Y,parameters,cache)
# Clip your gradients between -5 (min) and 5 (max) (≈1 line)
gradients = clip(gradients,5)
# Update parameters (≈1 line)
parameters = update_parameters(parameters,gradients,learning_rate)
### END CODE HERE ###
return loss, gradients, a[len(X)-1]
整个模型
每2000次用当前的parameters进行一次采样 生成新的词
def model(data, ix_to_char, char_to_ix, num_iterations = 35000, n_a = 50, dino_names = 7, vocab_size = 27):
"""
Trains the model and generates dinosaur names.
Arguments:
data -- text corpus
ix_to_char -- dictionary that maps the index to a character
char_to_ix -- dictionary that maps a character to an index
num_iterations -- number of iterations to train the model for
n_a -- number of units of the RNN cell
dino_names -- number of dinosaur names you want to sample at each iteration.
vocab_size -- number of unique characters found in the text, size of the vocabulary
Returns:
parameters -- learned parameters
"""
# Retrieve n_x and n_y from vocab_size
n_x, n_y = vocab_size, vocab_size
# Initialize parameters
parameters = initialize_parameters(n_a, n_x, n_y)
# Initialize loss (this is required because we want to smooth our loss, don't worry about it)
loss = get_initial_loss(vocab_size, dino_names)
# Build list of all dinosaur names (training examples).
with open("dinos.txt") as f:
examples = f.readlines()
examples = [x.lower().strip() for x in examples]
# Shuffle list of all dinosaur names
shuffle(examples)
# Initialize the hidden state of your LSTM
a_prev = np.zeros((n_a, 1))
# Optimization loop
for j in range(num_iterations):
### START CODE HERE ###
# Use the hint above to define one training example (X,Y) (≈ 2 lines)
index = j%len(examples)
X = [None] + [char_to_ix[ch] for ch in examples[index]]
Y = X[1:] + [char_to_ix["\n"]]
# Perform one optimization step: Forward-prop -> Backward-prop -> Clip -> Update parameters
# Choose a learning rate of 0.01
curr_loss, gradients, a_prev = optimize(X,Y,a_prev,parameters,learning_rate=0.01)
### END CODE HERE ###
# Use a latency trick to keep the loss smooth. It happens here to accelerate the training.
loss = smooth(loss, curr_loss)
# Every 2000 Iteration, generate "n" characters thanks to sample() to check if the model is learning properly
if j % 2000 == 0:
print('Iteration: %d, Loss: %f' % (j, loss) + '\n')
# The number of dinosaur names to print
seed = 0
for name in range(dino_names):
# Sample indices and print them
sampled_indices = sample(parameters, char_to_ix, seed)
print_sample(sampled_indices, ix_to_char)
seed += 1 # To get the same result for grading purposed, increment the seed by one.
print('\n')
return parameters