P
S
P
N
e
t
−
M
o
d
e
l
(
p
y
t
o
r
c
h
版
本
)
PSPNet-Model(pytorch版本)
PSPNet−Model(pytorch版本)
训练、验证代码逻辑
import torch
from torch import nn
from torch.nn import functional as F
import extractors
import warnings
warnings.filterwarnings("ignore")
class PSPModule(nn.Module):
def __init__(self, features, out_features=1024, sizes=(1, 2, 3, 6)):
super().__init__()
self.stages = []
self.stages = nn.ModuleList([self._make_stage(features, size) for size in sizes])
self.bottleneck = nn.Conv2d(features * (len(sizes) + 1), out_features, kernel_size=1)
self.relu = nn.ReLU()
def _make_stage(self, features, size):
prior = nn.AdaptiveAvgPool2d(output_size=(size, size))
conv = nn.Conv2d(features, features, kernel_size=1, bias=False)
return nn.Sequential(prior, conv)
def forward(self, feats):
h, w = feats.size(2), feats.size(3)
print(feats.size())
priors = [F.upsample(input=stage(feats), size=(h, w), mode='bilinear') for stage in self.stages] + [feats]
bottle = self.bottleneck(torch.cat(priors, 1))
return self.relu(bottle)
class PSPUpsample(nn.Module):
def __init__(self, in_channels, out_channels):
super().__init__()
self.conv = nn.Sequential(
nn.Conv2d(in_channels, out_channels, 3, padding=1),
nn.BatchNorm2d(out_channels),
nn.PReLU()
)
def forward(self, x):
h, w = 2 * x.size(2), 2 * x.size(3)
p = F.upsample(input=x, size=(h, w), mode='bilinear')
return self.conv(p)
class PSPNet(nn.Module):
def __init__(self, n_classes=18, sizes=(1, 2, 3, 6), psp_size=2048, deep_features_size=256, backend='resnet34',
pretrained=False):
super().__init__()
self.feats = getattr(extractors, backend)(pretrained)
self.psp = PSPModule(psp_size, 1024, sizes)
self.drop_1 = nn.Dropout2d(p=0.3)
self.up_1 = PSPUpsample(1024, 256)
self.up_2 = PSPUpsample(256, 64)
self.up_3 = PSPUpsample(64, 64)
self.drop_2 = nn.Dropout2d(p=0.15)
self.final = nn.Sequential(
nn.Conv2d(64, n_classes, kernel_size=1),
nn.LogSoftmax()
)
self.classifier = nn.Sequential(
nn.Linear(deep_features_size, 256),
nn.ReLU(),
nn.Linear(256, n_classes)
)
def forward(self, x):
print('x:', x.size())
f, class_f = self.feats(x);print('f:', f.size());print('class_f:', class_f.size());
p = self.psp(f);print('p:', p.size())
p = self.drop_1(p);print('p:', p.size())
p = self.up_1(p);print('p:', p.size())
p = self.drop_2(p);print('p:', p.size())
p = self.up_2(p);print('p:', p.size())
p = self.drop_2(p);print('p:', p.size())
p = self.up_3(p);print('p:', p.size())
p = self.drop_2(p);print('p:', p.size())
auxiliary = F.adaptive_max_pool2d(input=class_f, output_size=(1, 1)).view(-1, class_f.size(1));print('auxiliary:', auxiliary.size())
res1 = self.final(p);print('res1:', res1.size())
res2 = self.classifier(auxiliary);print('res2:', res2.size())
return res1 , res2
rgb = torch.randn(1, 3, 512, 512)
net = PSPNet(psp_size=512,n_classes=8,deep_features_size=256)
out, out_cls = net(rgb)
print('---out--'*5)
print(out.shape)
print('--out_cls---'*5)
print(out_cls)
print('-----'*5)
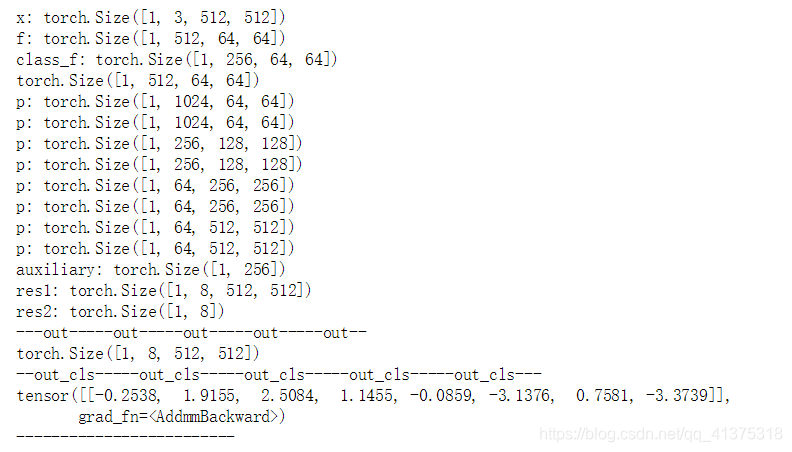