参考:
《MIND: Modality independent neighbourhood descriptor for multi-modal deformable registration》论文
MIND(模态独立邻域描述符)是一种图像配准算法,旨在提取每个像素/体素周围局部区域的结构信息。
一、原理介绍
1、公式介绍
MIND表达式如下:


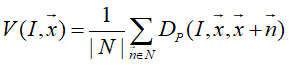
Dp(I,x,x+r)指以x点为中心的Patch块和以x+r为中心的Patch块的距离,即两个Patch块之差的平方。
V(I,x)是指图像中x点的6邻域搜索空间内各点的Dp(I,x,x+r)相加再平均。(这个邻域搜索空间后面介绍)
MIND(I,x,r)是感兴趣点x的邻域搜索空间内这n个点的Dp(I,x,x+r)除以该点的V(I,x),再负对数。
邻域搜索空间neigh Search Space:分为三种
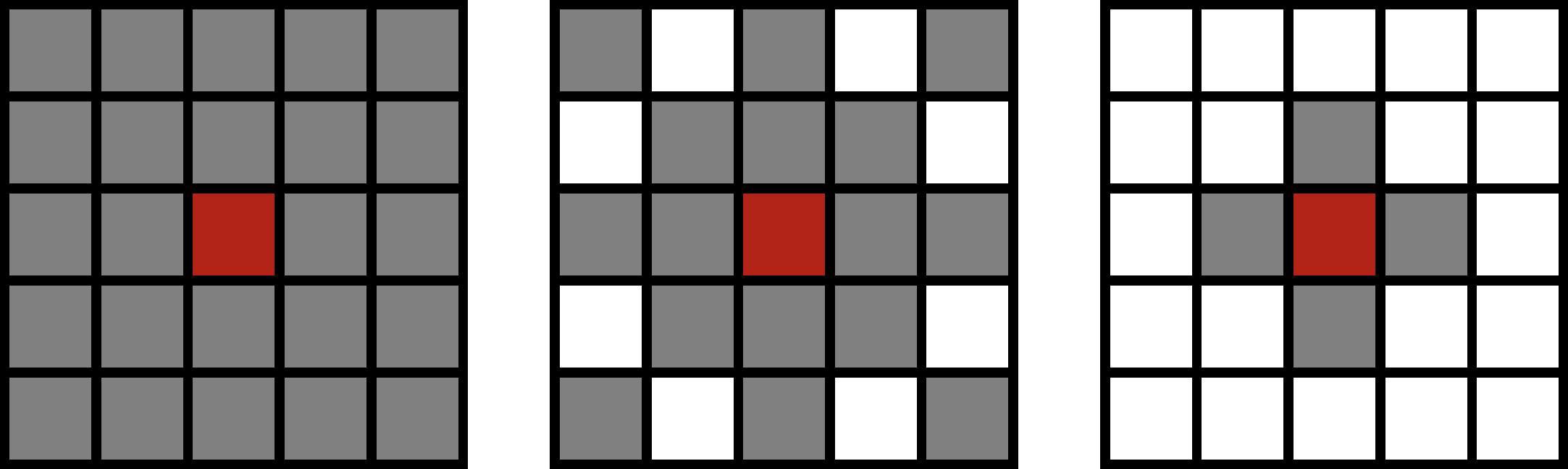
紧密型采样(全采样) 稀疏性采样(45°采样) 6邻域采样
*红色小块为感兴趣点,即需要求MIND特征的像素点。
如果设置一个5*5的一个neigh,紧密型采样的邻域搜索空间包括除红色像素以外的所有灰色像素点;稀疏型采样同理如中图所示;6邻域采样应该是文章针对三维图像来讲的,对于二维的图像,应该也可以说是4邻域。
Patch:3*3的patch是指一个3*3的像素小块
2、图示说明
从一个5*5的像素块开始理解:
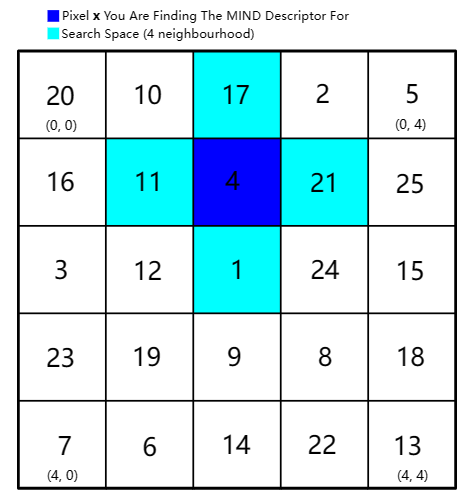
*蓝色像素4的地方是感兴趣点,即正要计算MIND特征的像素点,
*靛青色像素是设置的4邻域搜索空间
开始计算之前,先设置参数patch(3*3),neigh(3*3,4邻域采样)
(1)4邻域搜索空间内各点的Dp(I,x,x+r)
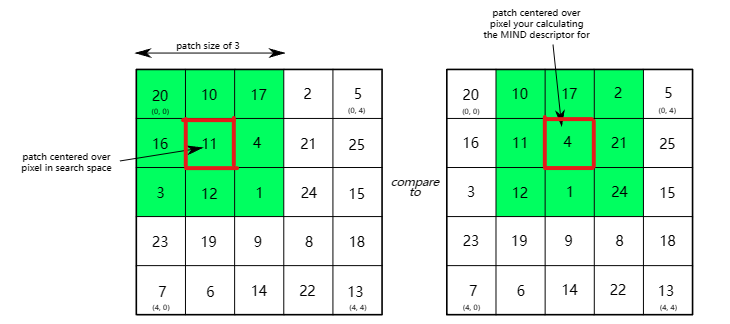
向量x=(1,2),向量x+r={(1,1),(0,2),(1,3),(2,2)}
同理求完四个点的Dp(I,x,x+r)如下图所示:
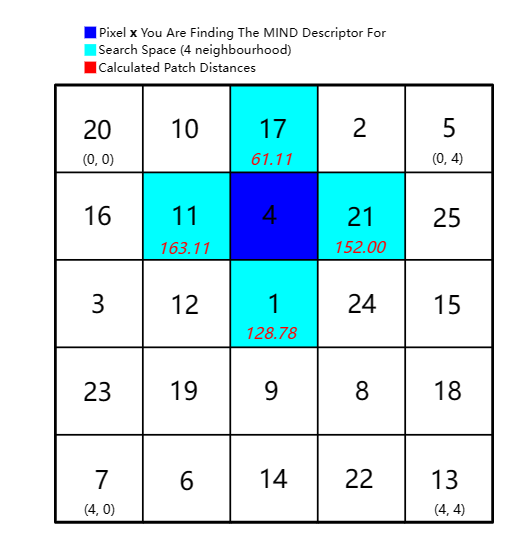
(2)求V(I,x)
V(I,x)在2维图像中需要求4邻域搜索空间的4个点的Dp(I,x,x+r)的平均
到这里只求得了一个像素点(1,2)的V(I,x),对于整个5*5的像素块或者256*256的图像,我们就需要求出这个像素块或者图像的V(I,x)的一个矩阵。
array([[ 15.33, 41.97, 68.19, 87.61, 60.97],
[ 47.06, 93.78, 126.25, 137.28, 90.56],
[ 93.72, 141.75, 157.06, 129.78, 81.75],
[109.42, 147.36, 152.08, 99.03, 61.08],
[ 77.69, 95.56, 94.03, 49.36, 31.5 ]])
(3)计算MIND特征

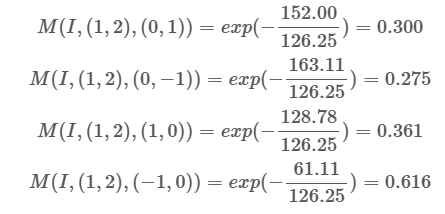
那么MIND(I,(1,2))=[0.300,0.275,0.361,0.616]
同样到这里,我们只获得了点(1,2)的MIND特征,而对于这个5*5的像素块,我们还要获得其余24个点的MIND特征。
最终得到的这个5*5的像素块的MIND特征如下:
mind_descriptors =
array([
[[0.199, 0.404, 0.258, 0.884],
[0.142, 0.554, 0.381, 0.611],
[0.354, 0.301, 0.408, 0.421],
[0.505, 0.446, 0.265, 0.307],
[0.955, 0.374, 0.205, 0.25 ]],
[[0.367, 0.615, 0.126, 0.643],
[0.176, 0.604, 0.266, 0.649],
[0.3 , 0.275, 0.361, 0.616],
[0.395, 0.33 , 0.328, 0.428],
[0.878, 0.244, 0.248, 0.344]],
[[0.43 , 0.845, 0.142, 0.354],
[0.301, 0.572, 0.255, 0.416],
[0.326, 0.339, 0.377, 0.44 ],
[0.419, 0.257, 0.552, 0.308],
[0.765, 0.251, 0.445, 0.214]],
[[0.49 , 0.887, 0.224, 0.188],
[0.376, 0.589, 0.308, 0.269],
[0.349, 0.388, 0.371, 0.365],
[0.383, 0.199, 0.525, 0.46 ],
[0.621, 0.211, 0.413, 0.339]],
[[0.488, 0.948, 0.325, 0.121],
[0.518, 0.558, 0.39 , 0.162],
[0.432, 0.512, 0.412, 0.201],
[0.575, 0.202, 0.575, 0.274],
[0.528, 0.42 , 0.459, 0.18 ]]])
如果想要比较一个5*5和像素块A和一个5*5的像素块B的结构相似性,那么就要先获得A和B的形状为(5,5,4)的MIND特征矩阵。然后将每个像素点的对应的MIND矩阵加和作为该像素点的MIND特征,最后就又会得到A的5*5的MIND特征图和B的MIND特征图。论文中的MIND特征图展示如下:
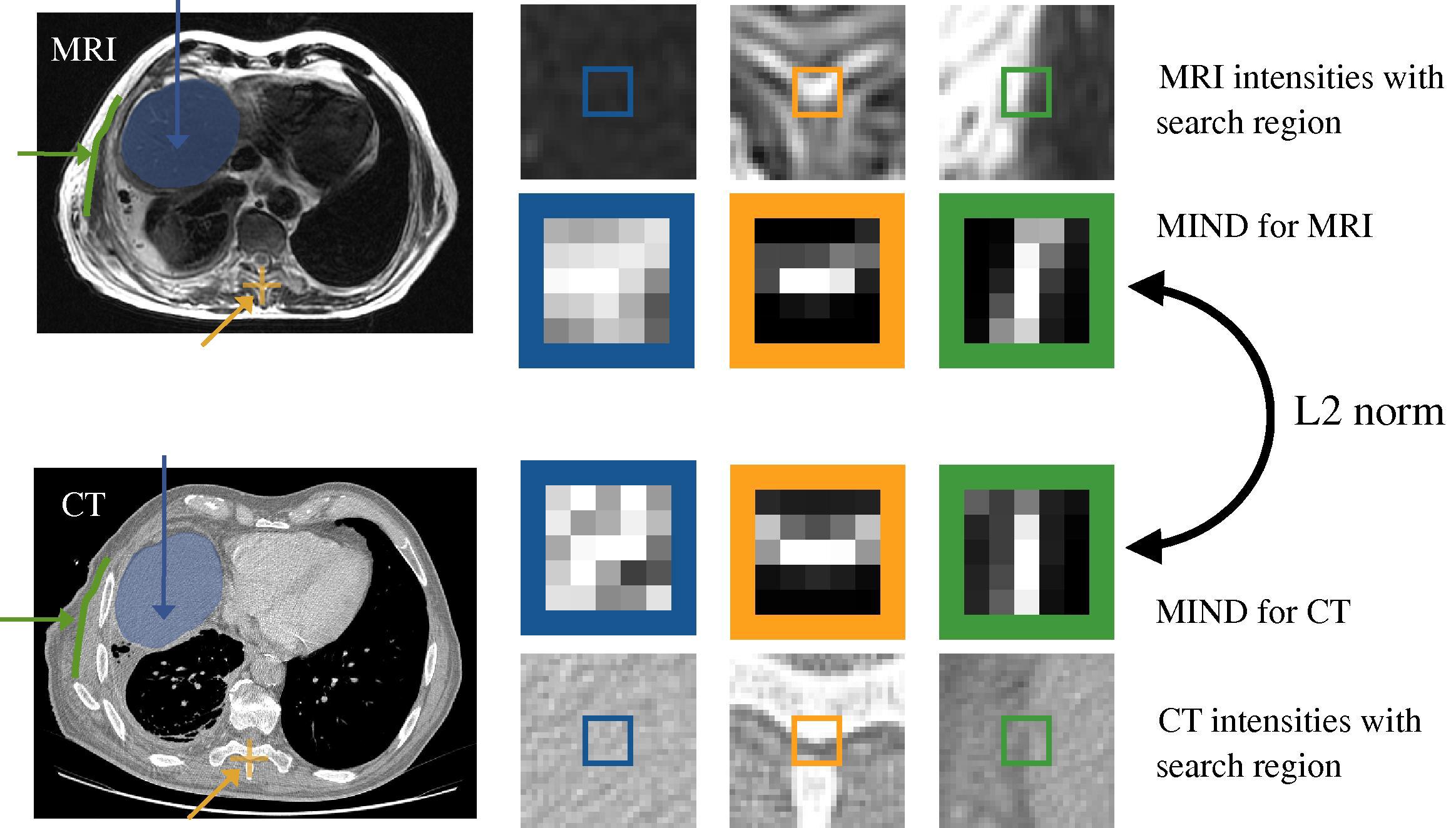
二、Python实现代码Pytorch(2D版)
import torch
import numpy as np
import torchvision
import SimpleITK as sitk
import torch.nn.functional as F
import matplotlib.pyplot as plt
def gaussian_kernel(sigma, sz):
xpos_vec = np.arange(sz)
ypos_vec = np.arange(sz)
output = np.ones([1, 1,sz, sz], dtype=np.single)
midpos = sz // 2
for xpos in xpos_vec:
for ypos in ypos_vec:
output[:,:,xpos,ypos] = np.exp(-((xpos-midpos)**2 + (ypos-midpos)**2) / (2 * sigma**2)) / (2 * np.pi * sigma**2)
return output
def torch_image_translate(input_, tx, ty, interpolation='nearest'):
# got these parameters from solving the equations for pixel translations
# on https://www.tensorflow.org/api_docs/python/tf/contrib/image/transform
translation_matrix = torch.zeros([1, 3, 3], dtype=torch.float)
translation_matrix[:, 0, 0] = 1.0
translation_matrix[:, 1, 1] = 1.0
translation_matrix[:, 0, 2] = -2*tx/(input_.size()[2]-1)
translation_matrix[:, 1, 2] = -2*ty/(input_.size()[3]-1)
translation_matrix[:, 2, 2] = 1.0
grid = F.affine_grid(translation_matrix[:, 0:2, :], input_.size()).to(input_.device)
wrp = F.grid_sample(input_, grid, mode=interpolation)
return wrp
def Dp(image, xshift, yshift, sigma, patch_size):
shift_image = torch_image_translate(image, xshift, yshift, interpolation='nearest')#将image在x、y方向移动I`(a)
diff = torch.sub(image, shift_image)#计算差分图I-I`(a)
diff_square = torch.mul(diff, diff)#(I-I`(a))^2
res = torch.conv2d(diff_square, weight =torch.from_numpy(gaussian_kernel(sigma, patch_size)), stride=1, padding=3)#C*(I-I`(a))^2
return res
def MIND(image, patch_size, neigh_size, sigma, eps,image_size0,image_size1, name='MIND'):
# compute the Modality independent neighbourhood descriptor (MIND) of input image.
# suppose the neighbor size is R, patch size is P.
# input image is 384 x 256 x input_c_dim
# output MIND is (384-P-R+2) x (256-P-R+2) x R*R
reduce_size = int((patch_size + neigh_size - 2) / 2)#卷积后减少的size
# estimate the local variance of each pixel within the input image.
Vimg = torch.add(Dp(image, -1, 0, sigma, patch_size), Dp(image, 1, 0, sigma, patch_size))
Vimg = torch.add(Vimg, Dp(image, 0, -1, sigma, patch_size))
Vimg = torch.add(Vimg, Dp(image, 0, 1, sigma, patch_size))#sum(Dp)
Vimg = torch.div(Vimg,4) + torch.mul(torch.ones_like(Vimg), eps)#防除零
# estimate the (R*R)-length MIND feature by shifting the input image by R*R times.
xshift_vec = np.arange( -(neigh_size//2), neigh_size - (neigh_size//2))#邻域计算
yshift_vec = np.arange(-(neigh_size // 2), neigh_size - (neigh_size // 2))#邻域计算
iter_pos = 0
for xshift in xshift_vec:
for yshift in yshift_vec:
if (xshift,yshift) == (0,0):
continue
MIND_tmp = torch.exp(torch.mul(torch.div(Dp(image, xshift, yshift, sigma, patch_size), Vimg), -1))#exp(-D(I)/V(I))
tmp = MIND_tmp[:, :, reduce_size:(image_size0 - reduce_size), reduce_size:(image_size1 - reduce_size)]
if iter_pos == 0:
output = tmp
else:
output = torch.cat([output,tmp], 1)
iter_pos = iter_pos + 1
# normalization.
input_max, input_indexes = torch.max(output, dim=1)
output = torch.div(output,input_max)
return output
def abs_criterion(in_, target):
return torch.mean(torch.abs(in_ - target))
if __name__ == '__main__':
patch_size=7
neigh_size=9
sigma=2.0
eps=1e-5
image_size0=512
image_size1=512
ct_path = r'F:\dataset\coarseReg\00001_SRO1_ct_axial_drr.nii.gz'
mr_path = r'F:\dataset\coarseReg\00001_SRO1_mr_axial_drr.nii.gz'
ct_sitk = sitk.ReadImage(ct_path,sitk.sitkFloat32)
mr_sitk = sitk.ReadImage(mr_path,sitk.sitkFloat32)
ct_axial_drr = sitk.GetArrayFromImage(ct_sitk)[np.newaxis ,np.newaxis,:,:]
mr_axial_drr = sitk.GetArrayFromImage(mr_sitk)[np.newaxis ,np.newaxis,:,: ]
plt.imshow(ct_axial_drr.squeeze())
plt.show()
ct_axial_drr = torch.from_numpy(ct_axial_drr)
mr_axial_drr = torch.from_numpy(mr_axial_drr)
A_MIND = MIND(ct_axial_drr, patch_size, neigh_size, sigma, eps,image_size0,image_size1, name='realA_MIND')
B_MIND = MIND(mr_axial_drr, patch_size, neigh_size, sigma, eps,image_size0,image_size1, name='realA_MIND')
g_loss_MIND = abs_criterion(A_MIND, B_MIND)
print('g_loss_MIND', g_loss_MIND)
# tf = np.load( r"C:\Users\shcho\Desktop\validation\tf.npy" )
# tc = np.load( r"C:\Users\shcho\Desktop\validation\tc.npy" )
# plt.imshow(tf)
# plt.show()
# plt.imshow(tc)
# plt.show()
# print(tf.shape, tc.shape)
#
# # print(tf.transpose((2,0,1)).shape, tc.shape)
# t = tf.transpose((2,0,1)) -tc
#
# # t = tf -tc
#
# print(np.max(t))
可以通过上面代码提取整张图像的MIND特征,且该函数可以被用于基于CycleGAN网络处理MRtoCT图像的损失函数并得到很好的结果,如论文《Unsupervised MR-to-CT Synthesis using Structure Constrained CycleGAN》所述,同理可用于相似的CycleGAN网络模型处理医学图像的任务中来约束A,B域的结构信息。