Incorporating Convolution Designs into Visual Transformers
**动机:**然而,纯变压器架构通常需要大量的训练数据或额外的监督,以获得与卷积神经网络(CNNs)相当的性能。为了克服这些限制,我们分析了直接从NLP中借用变压器架构时的潜在缺陷。在此基础上,==我们提出了一种新的卷积增强图像变压器(CeiT),==它结合了cnn在提取低水平特征、增强局部性和变压器在建立远程依赖关系方面的优势。对原变压器进行了三个修改:
1)我们设计了一个图像到令牌(I2T)模块,而不是直接的标记化,该模块从生成的低级特征中提取补丁;
2)每个编码器块中的前馈网络被替换为局部增强的前馈(LeFF)层,促进空间维度中相邻标记之间的相关性;
3)在利用多层次表示的变压器顶部附加了一个分层类标记注意(LCA)。
引言:
回顾卷积,其主要特征是**平移不变性和局部性**[22,31]。平移不变性与权值共享机制有关,该机制可以捕获视觉任务[23]中的几何和拓扑信息。对于局部性,在视觉任务[11,26,9]中,一个常见的假设是相邻的像素往往是相关的。然而,纯变压器架构并没有充分利用这些先前在图像中存在的偏差。首先,ViT对大小为16×16或32×32的原始输入图像中的补丁进行直接标记化。很难提取出在图像中形成一些基本结构的低级特征(如角落和边缘)。其次,自我注意模块集中于建立令牌之间的长期依赖关系,而忽略了空间维度中的局部性。
为了解决这些问题,我们设计了一种卷积增强图像变压器(CeiT),结合cnn在提取低级特征方面的优势、增强局部性以及变压器在关联远程依赖方面的优势。与普通ViT相比,做了三个修改。为了解决第一个问题,
我们设计了一个图像到标记(I2T)模块,而不是从原始输入图像中进行直接的标记化,该模块从生成的低级特征中提取补丁,其中补丁的尺寸更小,然后被平化为一系列标记。由于设计良好的结构,I2T模块不会引入更多的计算成本。
为了解决第二个问题,每个编码器块中的前馈网络被替换为局部增强的前馈(LeFF)层,该层促进了空间维度中相邻令牌之间的相关性。
为了利用自我注意的能力,在变压器的顶部附加了一个分层的类标记注意(LCA),利用多层表示来改进最终的表示。综上所述,我们的贡献如下:
- 我们设计了一种新的视觉变压器架构,即卷积增强图像变压器(CeiT)。它结合了卷积神经网络在提取低水平特征方面的优势,加强了局部性,以及变压器在建立远程依赖关系方面的优势。
- 在ImageNet和7个下游任务上的实验结果表明,与之前的ransformer 相比,CeiT的有效性和泛化能力学生和最先进的网络神经网络,不需要大量的培训数据和额外的CNN教师。例如,使用与ResNet- 50相似的模型大小,CeiT-S在ImageNet上获得了82.0%的前1位精度。当细化到384×384的分辨率时,结果提高到83.3%。
- CeiT模型比纯变压器模型具有更好的收敛性,训练迭代次数少3次×,显著降低了训练成本
3. Methodology
我们的CeiT是基于ViT而设计的。首先,我们将在第3.1节中简要概述了ViT的基本组件。接下来,我们将介绍三个包含卷积设计和有利于视觉变压器的修改,包括3.2节中的图像到令牌(I2T)模块、3.3节中的本地增强的FeedForwad(LeFF)模块和3.4节中的分层类令牌注意(LCA)模块。最后,我们在第3.5节中分析了这些所建议的模块的计算复杂度。
3.1.我们首先重新审视ViT中的基本组件,包括标记化、编码器块、多头自注意(MSA)层和前馈网络(FFN)层。
Tokenization.
标准的变压器[37]接收一系列的令牌嵌入作为输入。为了处理2D图像,ViT将图像 x ∈ R H × W × 3 x∈R_{H×W×3} x∈RH×W×3重构为扁平的2D补丁序列 x p ∈ R N × ( P 2 ⋅ 3 ) x_p∈R^{N×(P^2·3)} xp∈RN×(P2⋅3),其中(H,W)为原始图像的分辨率,3为RGB图像的通道数,(P,P)为每个图像补丁的分辨率, N = H W / P 2 N = HW/P^2 N=HW/P2为得到的补丁数,也作为变压器的有效输入序列长度。这些补丁被扁平并映射到大小为C的潜在嵌入,然后在序列中添加一个额外的类标记作为图像表示,从而输入大小为 x t ∈ R ( N + 1 ) × C x_t∈R^{(N+1)×C} xt∈R(N+1)×C的序列.
在实践中,ViT将补丁大小为16×16或32×32分割每个图像。但是,对具有大补丁的输入图像的直接标记化可能有两个限制: 1)很难捕获图像中的低级信息(如边和角);2)大内核的参数化过多,往往难以优化,因此需要更多的训练样本或训练迭代。
编码器块。ViT是由一系列堆叠的编码器组成的。每个编码器都有MSA和FFN的两个子层。在每个子层周围使用一个残差连接[12],然后是层归一化(LN)[1]。每个编码器的输出为:
与cnn在每个阶段开始时进行降采样不同,在不同的编码器块中,标记的长度没有减少。有效的感受域不能有效地扩展,这可能会影响视觉变压器的优化效率。
MSA.
就是标准的注意力机制!
FFN.
FFN执行点级操作,并分别应用于每个令牌。它由两个线性变换组成,中间有非线性激活:
而σ(·)是ViT中GELU [13]的非线性激活。
作为MSA模块的补充,FFN模块对每个令牌进行维数展开/降简和非线性变换,从而提高了令牌的表示能力。然而,在视觉中很重要的标记之间的空间关系并没有被考虑。这导致原始的ViT需要大量的训练数据来学习这些归纳偏差。
3.2.具有低水平特征的图像到令牌
为了解决上述标记化中的问题,我们提出了一个简单而有效的模块,名为图像-标记(I2T),该模块从特征映射中提取补丁,而不是从原始输入图像中提取补丁。如图2所示,I2T模块是一个由卷积层和最大池化层组成。消融研究还表明,在卷积层之后的批层有利于训练过程。它的可记号为:
其中,x‘∈R H S×WS×D,S为原始输入图像的步幅,D为富集通道数。然后将学习到的特征映射提取为空间维度上的斑块序列。为了保持生成的令牌数量与ViT保持一致,我们将补丁的分辨率缩小为 ( P / S , P / S ) (P/S,P/S) (P/S,P/S)。在实践中,我们设置了S = 4。
I2T充分利用了cnn在提取低级特征方面的优势,通过缩小补丁大小,降低了嵌入的训练难度。这也不同于ViT中提出的变压器类型变压器,使用常规ResNet-50从最后两个阶段提取高级特征。我们的I2T要轻得多。
3.3. Locally-Enhanced Feed-Forward Network
为了结合cnn提取局部信息的优势和变压器建立随机依赖关系的能力,我们提出了一个局部增强的前馈网络(LeFF)层。在每个编码器块中,我们保持MSA模块不变,以保持捕获令牌之间的全局相似性的能力。相反,原来的前馈网络层被LeFF所取代。其结构如图3所示。
其结构如图3所示。一个LeFF模块执行以下步骤。
首先,给定由前一个MSA模块生成的标记
x
h
t
∈
R
(
N
+
1
)
×
C
x_h^t∈R^{(N+1)×C}
xht∈R(N+1)×C,我们将它们分成补丁标记
x
h
p
∈
R
N
×
C
x_h^p∈R^{N×C}
xhp∈RN×C和相应的类标记
x
h
c
∈
R
C
x_h^c∈R^C
xhc∈RC。采用线性投影将补丁标记的嵌入扩展到更高维的
x
l
1
p
∈
R
N
×
(
e
×
C
)
x_{l1 }^p∈R^{N×(e×C})
xl1p∈RN×(e×C),其中e是扩展率。
其次,根据相对于原始图像的位置,将补丁标记恢复到空间维度上的 x p s ∈ R √ N × √ N × ( e × C ) x^s_ p∈R^{√N×√N×(e×C)} xps∈R√N×√N×(e×C)的“图像”。
第三,我们对这些恢复的补丁标记的核大小为k进行深度卷积,增强与相邻的 k 2 − 1 k^2−1 k2−1标记的表示相关性,得到 x p d ∈ R √ N × √ N × ( e × C ) x^d_p∈R^{√N×√N×(e×C)} xpd∈R√N×√N×(e×C)。
第四,这些贴片标记被扁平为 x p f ∈ R N × ( e × C ) x^f_p∈R^{N×(e×C)} xpf∈RN×(e×C)序列。最后,用 x p l 2 ∈ R N × C x^{l_2 }_p∈R^{N×C} xpl2∈RN×C将补丁标记投影到初始维度,并与类标记连接,得到 x t + 1 h ∈ R ( N + 1 ) × C x^h_ {t +1}∈R^{(N+1)×C} xt+1h∈R(N+1)×C。在每一个线性投影和深度卷积之后,添加一个批数和一个GELU。这些程序可记录如下:
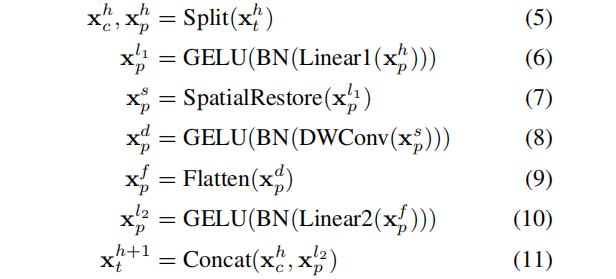
3.4.分层分类标记注意(Layer-wise Class-Token Attention)
在cnn中,随着网络的加深,特征图的感受野增加。在ViT中也发现了类似的观察结果,其“注意距离”随着深度的增加而增加。因此,特征表示在不同的层上将是不同的。为了集成跨不同层的信息,我们设计了一个分层的类标记注意(LCA)模块。与标准的ViT以最后一个l层的类令牌
x
c
(
L
)
x^{ (L)}_ c
xc(L)作为最终的表示不同,LCA关注不同层的类令牌。
如图4所示,LCA得到一个类标记序列作为输入,可以表示为 X c = [ x ( 1 ) c 、 ⋅ ⋅ ⋅ 、 x ( l ) c 、 ⋅ ⋅ ⋅ 、 x ( L ) c ] , X_c = [x_{(1)}^c、···、x_{(l)}^c、···、x_{(L)}^c ], Xc=[x(1)c、⋅⋅⋅、x(l)c、⋅⋅⋅、x(L)c],其中l表示图层的深度。LCA遵循标准的变压器块,其中包含一个MSA和一个FFN层。与最初的MSA计算任意两个标记(O(n 2))之间相似性的相似性不同,LCA==只计算最后一层中的类标记与其他层中的其他类标记(O (n))之间的相关性,==其中n表示标记的数量。对应的 x ( L ) c x_{(L)}^c x(L)c值通过注意与他人聚合。然后将聚合的值发送到一个FFN层中,从而得到最终的表示形式.
import math
import torch
import torch.nn as nn
import torch.nn.functional as F
from functools import partial
from timm.models.layers import DropPath, to_2tuple, trunc_normal_
from timm.models.registry import register_model
from timm.models.vision_transformer import default_cfgs, _cfg
__all__ = [
'ceit_tiny_patch16_224', 'ceit_small_patch16_224', 'ceit_base_patch16_224',
'ceit_tiny_patch16_384', 'ceit_small_patch16_384',
]
#Image-to-Tokens with Low-level Features
class Image2Tokens(nn.Module):
def __init__(self, in_chans=3, out_chans=64, kernel_size=7, stride=2):
super(Image2Tokens, self).__init__()
self.conv = nn.Conv2d(in_chans, out_chans, kernel_size=kernel_size, stride=stride,
padding=kernel_size // 2, bias=False)
self.bn = nn.BatchNorm2d(out_chans)
self.maxpool = nn.MaxPool2d(kernel_size=3, stride=2, padding=1)
def forward(self, x):
x = self.conv(x)
x = self.bn(x)
x = self.maxpool(x)
return x
class Mlp(nn.Module):
def __init__(self, in_features, hidden_features=None, out_features=None, act_layer=nn.GELU, drop=0.):
super().__init__()
out_features = out_features or in_features
hidden_features = hidden_features or in_features
self.fc1 = nn.Linear(in_features, hidden_features)
self.act = act_layer()
self.fc2 = nn.Linear(hidden_features, out_features)
self.drop = nn.Dropout(drop)
def forward(self, x):
x = self.fc1(x)
x = self.act(x)
x = self.drop(x)
x = self.fc2(x)
x = self.drop(x)
return x
class LocallyEnhancedFeedForward(nn.Module):
def __init__(self, in_features, hidden_features=None, out_features=None, act_layer=nn.GELU, drop=0.,
kernel_size=3, with_bn=True):
super().__init__()
out_features = out_features or in_features
hidden_features = hidden_features or in_features
# pointwise
self.conv1 = nn.Conv2d(in_features, hidden_features, kernel_size=1, stride=1, padding=0)
# depthwise
self.conv2 = nn.Conv2d(
hidden_features, hidden_features, kernel_size=kernel_size, stride=1,
padding=(kernel_size - 1) // 2, groups=hidden_features
)
# pointwise
self.conv3 = nn.Conv2d(hidden_features, out_features, kernel_size=1, stride=1, padding=0)
self.act = act_layer()
# self.drop = nn.Dropout(drop)
self.with_bn = with_bn
if self.with_bn:
self.bn1 = nn.BatchNorm2d(hidden_features)
self.bn2 = nn.BatchNorm2d(hidden_features)
self.bn3 = nn.BatchNorm2d(out_features)
def forward(self, x):
b, n, k = x.size()#输入是一个序列
cls_token, tokens = torch.split(x, [1, n - 1], dim=1)#第一维度是类别token
x = tokens.reshape(b, int(math.sqrt(n - 1)), int(math.sqrt(n - 1)), k).permute(0, 3, 1, 2)#将tokenreshape到图像形状
#无论是不是使用bn,最后都是对图像token进行处理
if self.with_bn:
x = self.conv1(x)
x = self.bn1(x)
x = self.act(x)
x = self.conv2(x)
x = self.bn2(x)
x = self.act(x)
x = self.conv3(x)
x = self.bn3(x)
else:
x = self.conv1(x)
x = self.act(x)
x = self.conv2(x)
x = self.act(x)
x = self.conv3(x)
#再次将token变为序列,与类别token拼接
tokens = x.flatten(2).permute(0, 2, 1)
out = torch.cat((cls_token, tokens), dim=1)
return out
class Attention(nn.Module):
def __init__(self, dim, num_heads=8, qkv_bias=False, qk_scale=None, attn_drop=0., proj_drop=0.):
super().__init__()
self.num_heads = num_heads
head_dim = dim // num_heads
# NOTE scale factor was wrong in my original version, can set manually to be compat with prev weights
self.scale = qk_scale or head_dim ** -0.5
self.qkv = nn.Linear(dim, dim * 3, bias=qkv_bias)
self.attn_drop = nn.Dropout(attn_drop)
self.proj = nn.Linear(dim, dim)
self.proj_drop = nn.Dropout(proj_drop)
self.attention_map = None
def forward(self, x):
B, N, C = x.shape
qkv = self.qkv(x).reshape(B, N, 3, self.num_heads, C // self.num_heads).permute(2, 0, 3, 1, 4)
q, k, v = qkv[0], qkv[1], qkv[2] # make torchscript happy (cannot use tensor as tuple)
attn = (q @ k.transpose(-2, -1)) * self.scale
attn = attn.softmax(dim=-1)
# self.attention_map = attn
attn = self.attn_drop(attn)
x = (attn @ v).transpose(1, 2).reshape(B, N, C)
x = self.proj(x)
x = self.proj_drop(x)
return x
class AttentionLCA(Attention):
def __init__(self, dim, num_heads=8, qkv_bias=False, qk_scale=None, attn_drop=0., proj_drop=0.):
super(AttentionLCA, self).__init__(dim, num_heads, qkv_bias, qk_scale, attn_drop, proj_drop)
self.dim = dim
self.qkv_bias = qkv_bias
def forward(self, x):
q_weight = self.qkv.weight[:self.dim, :]
q_bias = None if not self.qkv_bias else self.qkv.bias[:self.dim]
kv_weight = self.qkv.weight[self.dim:, :]
kv_bias = None if not self.qkv_bias else self.qkv.bias[self.dim:]
B, N, C = x.shape
_, last_token = torch.split(x, [N-1, 1], dim=1)
q = F.linear(last_token, q_weight, q_bias)\
.reshape(B, 1, self.num_heads, C // self.num_heads).permute(0, 2, 1, 3)
kv = F.linear(x, kv_weight, kv_bias)\
.reshape(B, N, 2, self.num_heads, C // self.num_heads).permute(2, 0, 3, 1, 4)
k, v = kv[0], kv[1]
attn = (q @ k.transpose(-2, -1)) * self.scale
attn = attn.softmax(dim=-1)
# self.attention_map = attn
attn = self.attn_drop(attn)
x = (attn @ v).transpose(1, 2).reshape(B, 1, C)
x = self.proj(x)
x = self.proj_drop(x)
return x
class Block(nn.Module):
def __init__(self, dim, num_heads, mlp_ratio=4., qkv_bias=False, qk_scale=None, drop=0., attn_drop=0.,
drop_path=0., act_layer=nn.GELU, norm_layer=nn.LayerNorm, kernel_size=3, with_bn=True,
feedforward_type='leff'):
super().__init__()
# NOTE: drop path for stochastic depth, we shall see if this is better than dropout here
self.drop_path = DropPath(drop_path) if drop_path > 0. else nn.Identity()
self.norm2 = norm_layer(dim)
mlp_hidden_dim = int(dim * mlp_ratio)
self.norm1 = norm_layer(dim)
self.feedforward_type = feedforward_type
if feedforward_type == 'leff':
self.attn = Attention(
dim, num_heads=num_heads, qkv_bias=qkv_bias, qk_scale=qk_scale, attn_drop=attn_drop, proj_drop=drop)
self.leff = LocallyEnhancedFeedForward(
in_features=dim, hidden_features=mlp_hidden_dim, act_layer=act_layer, drop=drop,
kernel_size=kernel_size, with_bn=with_bn,
)
else: # LCA
self.attn = AttentionLCA(
dim, num_heads=num_heads, qkv_bias=qkv_bias, qk_scale=qk_scale, attn_drop=attn_drop, proj_drop=drop)
self.feedforward = Mlp(
in_features=dim, hidden_features=mlp_hidden_dim, act_layer=act_layer, drop=drop
)
def forward(self, x):
if self.feedforward_type == 'leff':
x = x + self.drop_path(self.attn(self.norm1(x)))
x = x + self.drop_path(self.leff(self.norm2(x)))
return x, x[:, 0]
else: # LCA
_, last_token = torch.split(x, [x.size(1)-1, 1], dim=1)
x = last_token + self.drop_path(self.attn(self.norm1(x)))
x = x + self.drop_path(self.feedforward(self.norm2(x)))
return x
class HybridEmbed(nn.Module):
""" CNN Feature Map Embedding
Extract feature map from CNN, flatten, project to embedding dim.
"""
def __init__(self, backbone, img_size=224, patch_size=16, feature_size=None, in_chans=3, embed_dim=768):
super().__init__()
assert isinstance(backbone, nn.Module)
img_size = to_2tuple(img_size)
self.img_size = img_size
self.backbone = backbone
if feature_size is None:
with torch.no_grad():
# FIXME this is hacky, but most reliable way of determining the exact dim of the output feature
# map for all networks, the feature metadata has reliable channel and stride info, but using
# stride to calc feature dim requires info about padding of each stage that isn't captured.
training = backbone.training
if training:
backbone.eval()
o = self.backbone(torch.zeros(1, in_chans, img_size[0], img_size[1]))
if isinstance(o, (list, tuple)):
o = o[-1] # last feature if backbone outputs list/tuple of features
feature_size = o.shape[-2:]
feature_dim = o.shape[1]
backbone.train(training)
else:
feature_size = to_2tuple(feature_size)
feature_dim = self.backbone.feature_info.channels()[-1]
print('feature_size is {}, feature_dim is {}, patch_size is {}'.format(
feature_size, feature_dim, patch_size
))
self.num_patches = (feature_size[0] // patch_size) * (feature_size[1] // patch_size)
self.proj = nn.Conv2d(feature_dim, embed_dim, kernel_size=patch_size, stride=patch_size)
def forward(self, x):
x = self.backbone(x)
if isinstance(x, (list, tuple)):
x = x[-1] # last feature if backbone outputs list/tuple of features
x = self.proj(x).flatten(2).transpose(1, 2)
return x
class CeIT(nn.Module):
def __init__(self,
img_size=224,
patch_size=16,
in_chans=3,
num_classes=1000,
embed_dim=768,
depth=12,
num_heads=12,
mlp_ratio=4.,
qkv_bias=False,
qk_scale=None,
drop_rate=0.,
attn_drop_rate=0.,
drop_path_rate=0.,
hybrid_backbone=None,
norm_layer=nn.LayerNorm,
leff_local_size=3,
leff_with_bn=True):
"""
args:
- img_size (:obj:`int`): input image size
- patch_size (:obj:`int`): patch size
- in_chans (:obj:`int`): input channels
- num_classes (:obj:`int`): number of classes
- embed_dim (:obj:`int`): embedding dimensions for tokens
- depth (:obj:`int`): depth of encoder
- num_heads (:obj:`int`): number of heads in multi-head self-attention
- mlp_ratio (:obj:`float`): expand ratio in feedforward
- qkv_bias (:obj:`bool`): whether to add bias for mlp of qkv
- qk_scale (:obj:`float`): scale ratio for qk, default is head_dim ** -0.5
- drop_rate (:obj:`float`): dropout rate in feedforward module after linear operation
and projection drop rate in attention
- attn_drop_rate (:obj:`float`): dropout rate for attention
- drop_path_rate (:obj:`float`): drop_path rate after attention
- hybrid_backbone (:obj:`nn.Module`): backbone e.g. resnet
- norm_layer (:obj:`nn.Module`): normalization type
- leff_local_size (:obj:`int`): kernel size in LocallyEnhancedFeedForward
- leff_with_bn (:obj:`bool`): whether add bn in LocallyEnhancedFeedForward
"""
super().__init__()
self.num_classes = num_classes
self.num_features = self.embed_dim = embed_dim # num_features for consistency with other models
self.i2t = HybridEmbed(
hybrid_backbone, img_size=img_size, patch_size=patch_size, in_chans=in_chans, embed_dim=embed_dim)
num_patches = self.i2t.num_patches
self.cls_token = nn.Parameter(torch.zeros(1, 1, embed_dim))
self.pos_embed = nn.Parameter(torch.zeros(1, num_patches + 1, embed_dim))
self.pos_drop = nn.Dropout(p=drop_rate)
dpr = [x.item() for x in torch.linspace(0, drop_path_rate, depth)] # stochastic depth decay rule
self.blocks = nn.ModuleList([
Block(
dim=embed_dim, num_heads=num_heads, mlp_ratio=mlp_ratio, qkv_bias=qkv_bias, qk_scale=qk_scale,
drop=drop_rate, attn_drop=attn_drop_rate, drop_path=dpr[i], norm_layer=norm_layer,
kernel_size=leff_local_size, with_bn=leff_with_bn)
for i in range(depth)])
# without droppath
self.lca = Block(
dim=embed_dim, num_heads=num_heads, mlp_ratio=mlp_ratio, qkv_bias=qkv_bias, qk_scale=qk_scale,
drop=drop_rate, attn_drop=attn_drop_rate, drop_path=0., norm_layer=norm_layer,
feedforward_type = 'lca'
)
self.pos_layer_embed = nn.Parameter(torch.zeros(1, depth, embed_dim))
self.norm = norm_layer(embed_dim)
# NOTE as per official impl, we could have a pre-logits representation dense layer + tanh here
# self.repr = nn.Linear(embed_dim, representation_size)
# self.repr_act = nn.Tanh()
# Classifier head
self.head = nn.Linear(embed_dim, num_classes) if num_classes > 0 else nn.Identity()
trunc_normal_(self.pos_embed, std=.02)
trunc_normal_(self.cls_token, std=.02)
self.apply(self._init_weights)
def _init_weights(self, m):
if isinstance(m, nn.Linear):
trunc_normal_(m.weight, std=.02)
if isinstance(m, nn.Linear) and m.bias is not None:
nn.init.constant_(m.bias, 0)
elif isinstance(m, nn.LayerNorm):
nn.init.constant_(m.bias, 0)
nn.init.constant_(m.weight, 1.0)
@torch.jit.ignore
def no_weight_decay(self):
return {'pos_embed', 'cls_token'}
def get_classifier(self):
return self.head
def reset_classifier(self, num_classes, global_pool=''):
self.num_classes = num_classes
self.head = nn.Linear(self.embed_dim, num_classes) if num_classes > 0 else nn.Identity()
def forward_features(self, x):
B = x.shape[0]
x = self.i2t(x)
cls_tokens = self.cls_token.expand(B, -1, -1) # stole cls_tokens impl from Phil Wang, thanks
x = torch.cat((cls_tokens, x), dim=1)
x = x + self.pos_embed
x = self.pos_drop(x)
cls_token_list = []
for blk in self.blocks:
x, curr_cls_token = blk(x)
cls_token_list.append(curr_cls_token)
all_cls_token = torch.stack(cls_token_list, dim=1) # B*D*K
all_cls_token = all_cls_token + self.pos_layer_embed
# attention over cls tokens
last_cls_token = self.lca(all_cls_token)
last_cls_token = self.norm(last_cls_token)
return last_cls_token.view(B, -1)
def forward(self, x):
x = self.forward_features(x)
x = self.head(x)
return x
@register_model
def ceit_tiny_patch16_224(pretrained=False, **kwargs):
"""
convolutional + pooling stem
local enhanced feedforward
attention over cls_tokens
"""
i2t = Image2Tokens()
model = CeIT(
hybrid_backbone=i2t,
patch_size=4, embed_dim=192, depth=12, num_heads=3, mlp_ratio=4, qkv_bias=True,
norm_layer=partial(nn.LayerNorm, eps=1e-6), **kwargs)
model.default_cfg = _cfg()
return model
@register_model
def ceit_small_patch16_224(pretrained=False, **kwargs):
"""
convolutional + pooling stem
local enhanced feedforward
attention over cls_tokens
"""
i2t = Image2Tokens()
model = CeIT(
hybrid_backbone=i2t,
patch_size=4, embed_dim=384, depth=12, num_heads=6, mlp_ratio=4, qkv_bias=True,
norm_layer=partial(nn.LayerNorm, eps=1e-6), **kwargs)
model.default_cfg = _cfg()
return model
@register_model
def ceit_base_patch16_224(pretrained=False, **kwargs):
"""
convolutional + pooling stem
local enhanced feedforward
attention over cls_tokens
"""
i2t = Image2Tokens()
model = CeIT(
hybrid_backbone=i2t,
patch_size=4, embed_dim=768, depth=12, num_heads=12, mlp_ratio=4, qkv_bias=True,
norm_layer=partial(nn.LayerNorm, eps=1e-6), **kwargs)
model.default_cfg = _cfg()
return model
@register_model
def ceit_tiny_patch16_384(pretrained=False, **kwargs):
"""
convolutional + pooling stem
local enhanced feedforward
attention over cls_tokens
"""
i2t = Image2Tokens()
model = CeIT(
hybrid_backbone=i2t, img_size=384,
patch_size=4, embed_dim=192, depth=12, num_heads=3, mlp_ratio=4, qkv_bias=True,
norm_layer=partial(nn.LayerNorm, eps=1e-6), **kwargs)
model.default_cfg = _cfg()
return model
@register_model
def ceit_small_patch16_384(pretrained=False, **kwargs):
"""
convolutional + pooling stem
local enhanced feedforward
attention over cls_tokens
"""
i2t = Image2Tokens()
model = CeIT(
hybrid_backbone=i2t, img_size=384,
patch_size=4, embed_dim=384, depth=12, num_heads=6, mlp_ratio=4, qkv_bias=True,
norm_layer=partial(nn.LayerNorm, eps=1e-6), **kwargs)
model.default_cfg = _cfg()
return model