1. 前提了解
- 首先我们清理要注意到异常数据,缺失数据,对于缺失数据我们可以用什么来替换,对于异常数据我们可以用什么来进行填充,考虑占比多少需不需要删除掉。
- 其次我们要注意到缺失数据,对于缺失数据,我的策略有两种,其一:查看数据是否是正常情况,比如泰坦尼克号中,有些数据是没有的但是他是正常情况,其二:设定阀值来选择是否保留这一列,这个设定阀值的大小看你对列数据的理解,确定哪个值是合适的,而不是直接删除。
- 我们如何查看异常数据,我们可以遍历每一列的唯一值来查看数据是否有异常,从唯一值我们可以很清晰的看出数据爬取过程中出现的差错。这篇文章使用了这个代码进行遍历,如下所示:
-
# 遍历所有列并打印唯一值 for column in data.columns: unique_values = data[column].unique() print(f"Column: {column}") print(f"Unique values ({len(unique_values)}): {unique_values}") print("\n")
2. 数据清理
1. 查看源数据
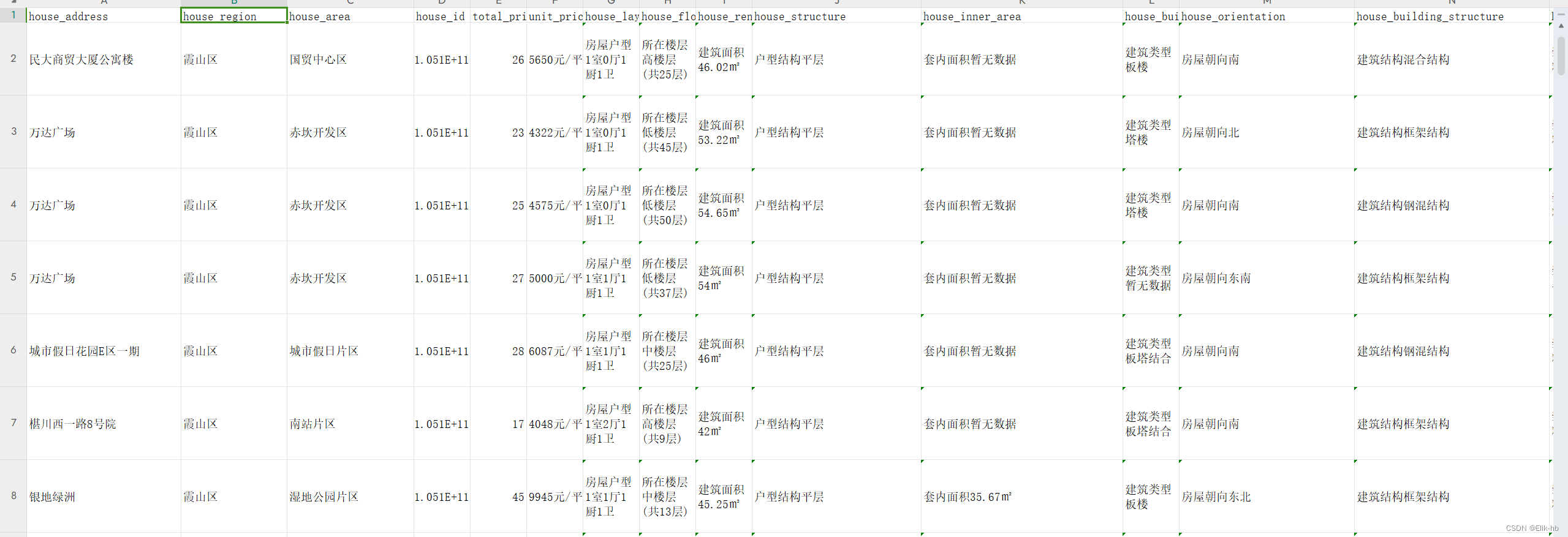
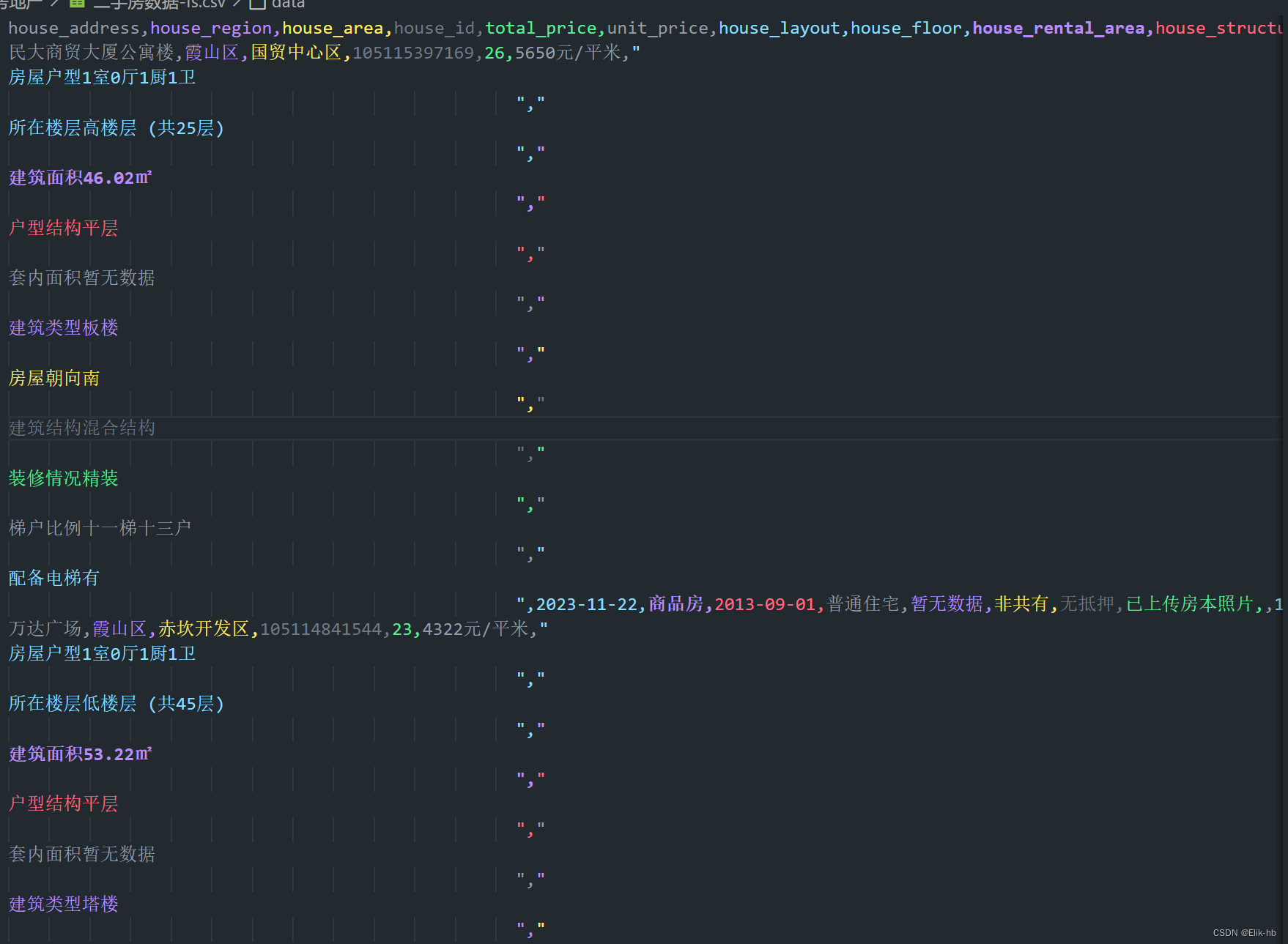
从图1和图2我们可以看到,文本数据有很多的空格和符号,所以我们进行去除和分割
2. 文本数据进行处理
import pandas as pd
# 读取CSV文件
data = pd.read_csv('二手房数据-fs.csv', encoding='utf-8')
# 去除额外空格和符号
data = data.applymap(lambda x: x.strip() if isinstance(x, str) else x)
# 分割文本数据
data = data.applymap(lambda x: x.split(',')[0] if isinstance(x, str) else x)
data.to_csv('二手房数据-文本处理.csv', index=False, encoding='utf-8')
运行后,查看保存的处理后的数据
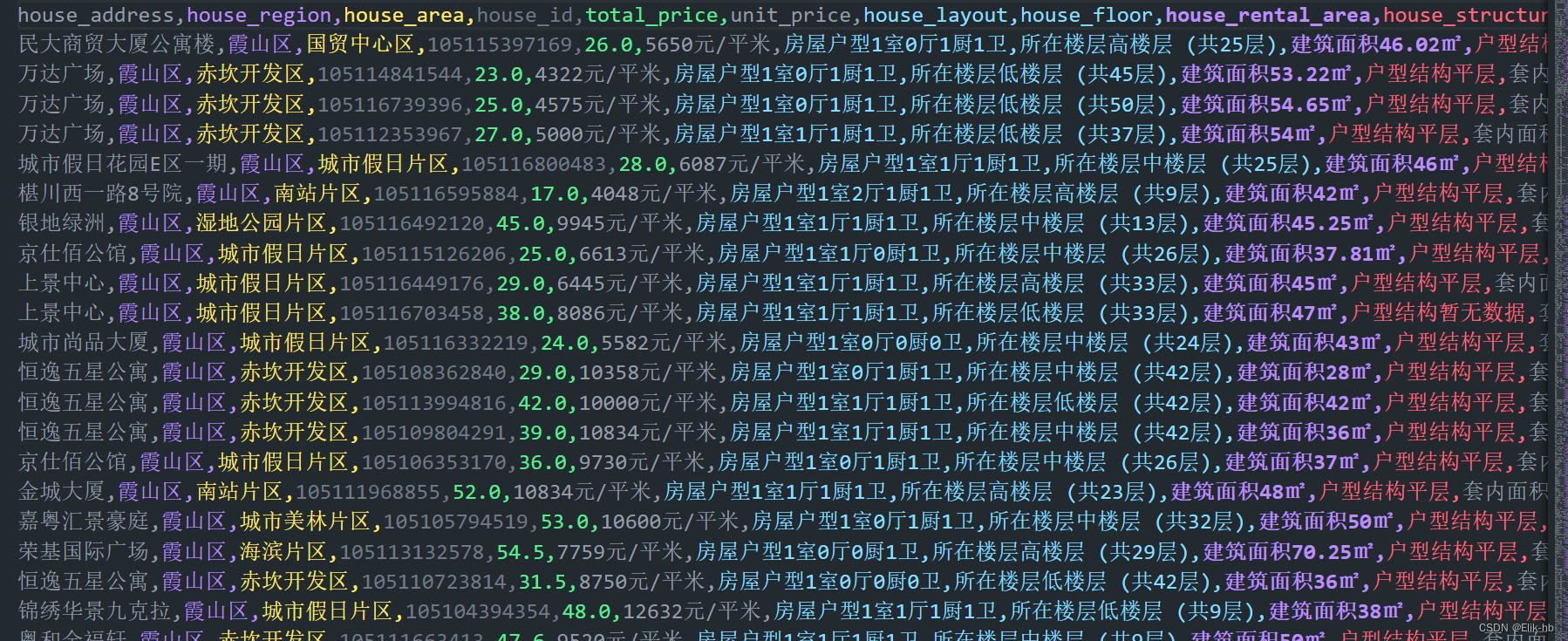
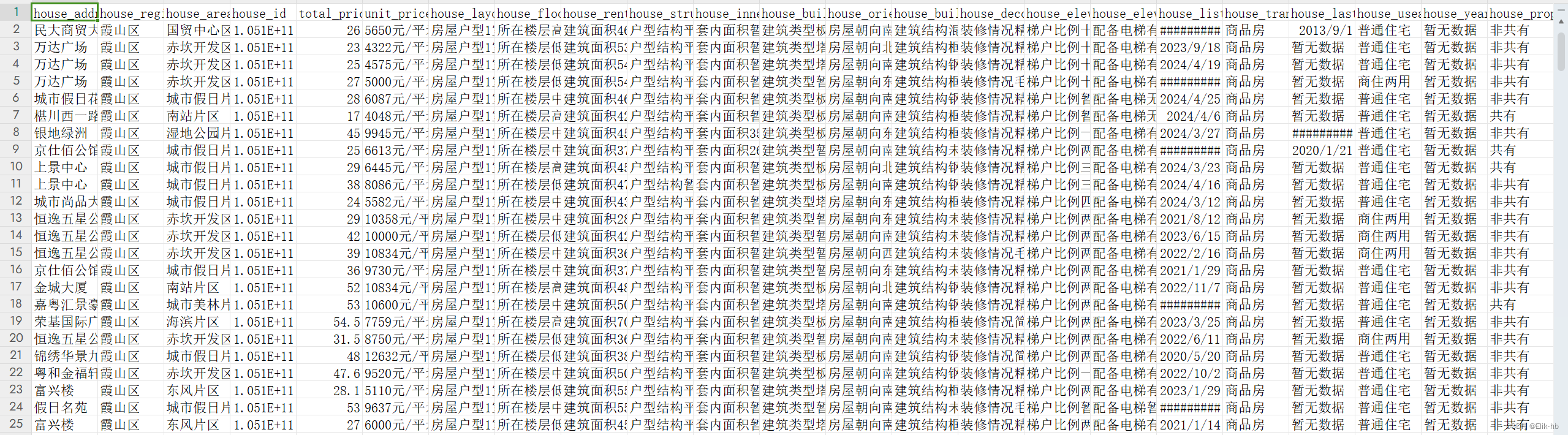
从图3和图4我们可以看到,对于那些换行,空格,我们处理好了,接着我们就开始处理数据里面的异常值还有缺失值
3. 查看数据集中的列名、非空值数量、数据类型等信息
print(data.info())
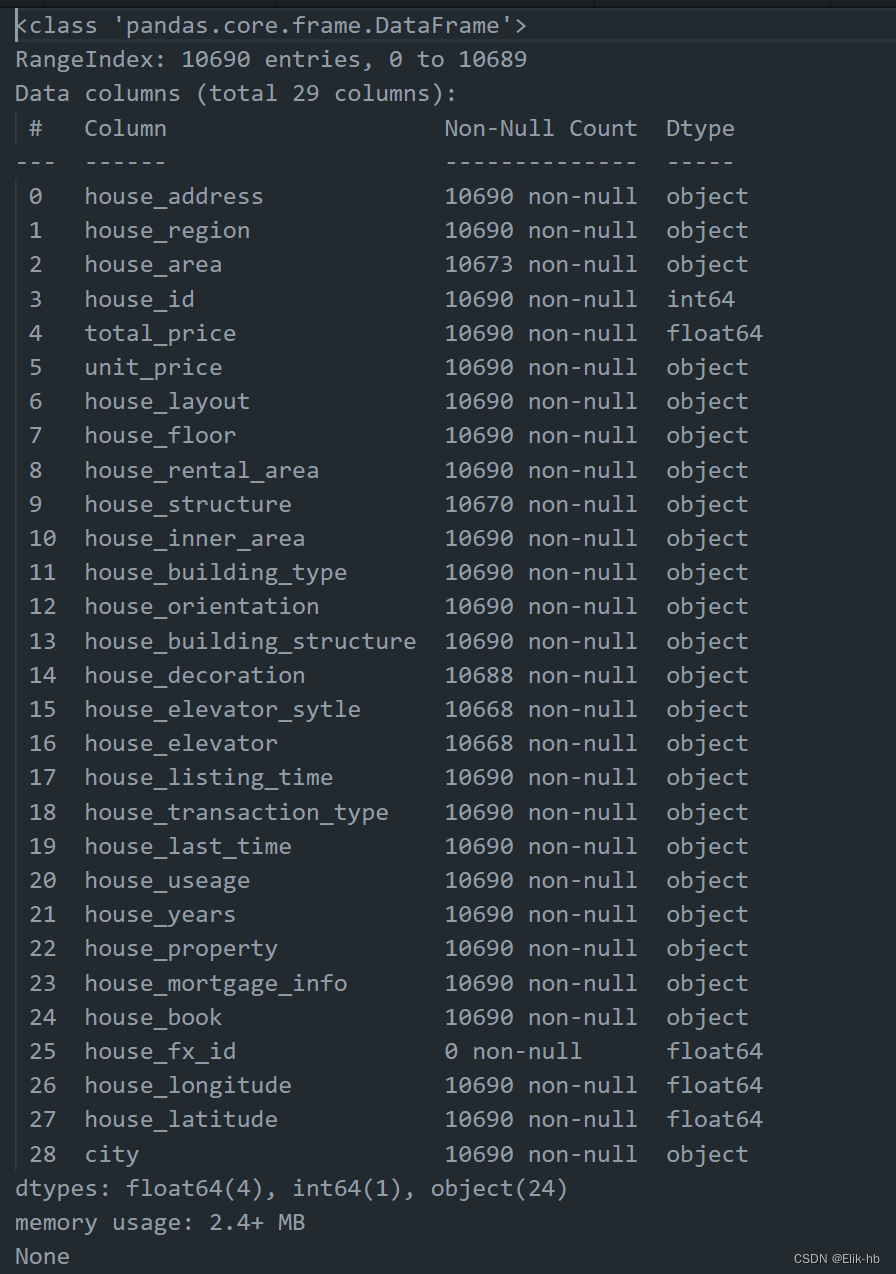
可以看到,数据有很多列是有缺失值的,然后数据里面的异常值我们也是不清楚的,我们要对每列进行详细清洗得到干净数据。
4. 查看unit_price列,house_rental_area列进行处理
使用在前提了解说过的遍历代码,运行得到输出结果。
在输出结果中,我们查看这两列的唯一值
可以看到数据是数值类型,我们对数据进行数值提取。
代码如下:
# 阀值设为 0.4
threshold = 0.4
# 移除单价字段中的特殊字符并转换为数值
data['unit_price'] = data['unit_price'].replace({'元/平米': ''}, regex=True)
data['unit_price'] = pd.to_numeric(data['unit_price'], errors='coerce')
unit_price_missing_ratio = data['unit_price'].isna().mean()
if unit_price_missing_ratio > threshold:
print("Warning: 超过阈值,删除'unit_price'字段.")
data.drop(columns=['unit_price'], inplace=True)
else:
unit_price_mode = data['unit_price'].mode()[0]
data['unit_price'].fillna(unit_price_mode, inplace=True)
data['unit_price'] = data['unit_price'].astype(float)
# 提取面积数据并转换为浮点数
data['house_rental_area'] = data['house_rental_area'].str.extract(r'(\d+\.?\d*)')[0]
data['house_rental_area'] = pd.to_numeric(data['house_rental_area'], errors='coerce')
house_rental_area_missing_ratio = data['house_rental_area'].isna().mean()
if house_rental_area_missing_ratio > threshold:
print("Warning: 超过阈值,删除'house_rental_area'字段.")
data.drop(columns=['house_rental_area'], inplace=True)
else:
house_rental_area_mode = data['house_rental_area'].mode()[0]
data['house_rental_area'].fillna(house_rental_area_mode, inplace=True)
data['house_rental_area'] = data['house_rental_area'].astype(float)
# 转换总价为浮点数
data['total_price'] = data['total_price'].astype(float)
处理后可以用遍历代码查看唯一值可以看到处理完成:
接着我们继续处理下一列
5. 查看于house_area列,查看缺失值有多少
可以看到缺失了17个数据,这个列数据是房子地名,我们可以考虑用地址名代替,即使用house_address替代
# 对于缺失值,使用对应的house_address填充
data['house_area'] = data['house_area'].fillna(data['house_address'])
# 删除重复值
data.drop_duplicates(inplace=True)
# 重置索引
data.reset_index(drop=True, inplace=True)
data.info()
data.isnull().sum()
6. house_last_time和 house_listing_time数据处理(时间数据)
遍历查看数据
可以看到数据里有暂无数据,我们可以查看他是否缺失超过阀值0.5,如果超过则删除该列
代码处理如下:
# 阀值设为 0.5
threshold = 0.5
# 处理缺失值和转换类型的函数
def process_column(data, column, dtype, threshold):
data[column] = pd.to_numeric(data[column], errors='coerce') if dtype == 'numeric' else pd.to_datetime(data[column], errors='coerce')
missing_ratio = data[column].isna().mean()
if missing_ratio > threshold:
print(f"Warning: Missing values in '{column}' exceed the threshold of {threshold}, deleting the column.")
data.drop(columns=[column], inplace=True)
else:
if dtype == 'numeric':
mode_value = data[column].mode()[0]
elif dtype == 'datetime':
mode_value = data[column].dropna().mode()[0] if not data[column].dropna().empty else pd.NaT
data[column].fillna(mode_value, inplace=True)
# 处理 house_last_time 列
process_column(data, 'house_last_time', 'datetime', threshold)
# 处理 house_listing_time 列
process_column(data, 'house_listing_time', 'datetime', threshold)
# 确保数值列为数值类型并处理缺失值
for col in ['total_price', 'unit_price', 'house_rental_area']:
process_column(data, col, 'numeric', threshold)
data.reset_index(drop=True, inplace=True)
查看输出
可以得出该列缺失数据异常多,去除了该列
查看处理后的house_listing_time数据唯一值
7. 处理house_floor楼层这一列数据,提取出里面两层数据,进行阀值判断,超过0.4则删除该列
遍历查看数据
在数据中看到他是有两层信息,第一是他的总楼层数还有他所处的是否是高低中楼层,我们可以在里面提取出两个数据,变成新列。代码设置阀值0.4超过则删除该列。代码如下:
import re
# 提取所在楼层和总楼层数
def extract_floor_info(floor_info):
match = re.match(r'所在楼层(.+?) \(共(\d+)层\)', str(floor_info))
if match:
return match.groups()
return (None, None)
data[['floor_level', 'total_floors']] = data['house_floor'].apply(lambda x: pd.Series(extract_floor_info(x)))
# 转换总楼层数为数值类型
data['total_floors'] = pd.to_numeric(data['total_floors'], errors='coerce')
total_floors_missing_ratio = data['total_floors'].isna().mean()
# 阀值设为 0.4
threshold = 0.4
if total_floors_missing_ratio > threshold:
print(f"Warning: Missing values in 'total_floors' exceed the threshold of {threshold}, deleting the column.")
data.drop(columns=['total_floors'], inplace=True)
else:
total_floors_mode = data['total_floors'].mode()[0]
data['total_floors'].fillna(total_floors_mode, inplace=True)
data.drop(columns=['house_floor'], inplace=True)
# 重置索引
data.reset_index(drop=True, inplace=True)
遍历查看新列结果
8. 处理house_layout该列,可以看出房型有4种信息,我们进行关键信息提取,提取为新列,定义阀值为0.4
遍历查看数据
可以看到数据里面是有四种信息在里面,我们可以在后面分析哪种信息的重要性比较高,我们就可以拿来使用,代码如下:
# 阀值设为 0.4
threshold = 0.4
# 计算 house_layout 列的缺失值和无效值比例
invalid_layout_ratio = data['house_layout'].isna().mean() + data['house_layout'].apply(lambda x: bool(re.match(r'^\d+室\d+厅\d+厨\d+卫$', str(x))) if pd.notna(x) else False).mean()
if invalid_layout_ratio > threshold:
print(f"Warning: Missing or invalid values in 'house_layout' exceed the threshold of {threshold}, deleting the column.")
data.drop(columns=['house_layout'], inplace=True)
else:
# 定义提取数量的函数
def extract_layout_info(layout_info):
room_match = re.search(r'(\d+)室', str(layout_info))
hall_match = re.search(r'(\d+)厅', str(layout_info))
kitchen_match = re.search(r'(\d+)厨', str(layout_info))
bathroom_match = re.search(r'(\d+)卫', str(layout_info))
room = int(room_match.group(1)) if room_match else 0
hall = int(hall_match.group(1)) if hall_match else 0
kitchen = int(kitchen_match.group(1)) if kitchen_match else 0
bathroom = int(bathroom_match.group(1)) if bathroom_match else 0
return pd.Series([room, hall, kitchen, bathroom])
# 提取数量并创建新的列
data[['rooms', 'halls', 'kitchens', 'bathrooms']] = data['house_layout'].apply(extract_layout_info)
# 删除house_layout列
data.drop(columns=['house_layout'], inplace=True)
提取后查看新列
9. 处理房屋朝向数据,我们使用标准的朝南,北,东,西,其他朝向归为其他
查看爬取到的数据,他是有很多指向的,我们对他们进行处理得到标准的朝向,在图中可以看到有很多异常数据,对于异常数据这里选择使用众数进行填充,代码处理:
# 定义标准朝向
standard_orientations = ['南', '北', '东', '西', '东南', '东北', '西南', '西北']
# 映射函数
def map_orientation(orientation):
for std_orientation in standard_orientations:
if std_orientation in orientation:
return std_orientation
return '其他'
# 创建新的列 standard_orientation
data['standard_orientation'] = data['house_orientation'].apply(map_orientation)
data.drop(columns=['house_orientation'], inplace=True)
# 重置索引
data.reset_index(drop=True, inplace=True)
#查看新的列standard_orientation的唯一值
print(data['standard_orientation'].unique())
#查看新的列standard_orientation的不同类型的数量
print(data['standard_orientation'].value_counts())
# 显示处理后的数据基本信息和前几行数据
""" print(data.info())
print(data.head()) """
遍历查看处理后的结果
10. 处理户型结构数据,可以看出爬取过程中出现异常数据,我们对异常数据和暂无数据进行使用众数填充
遍历查看数据:
代码处理:
# 定义有效的 house_structure 类型
valid_structures = ['户型结构平层', '户型结构跃层', '户型结构复式', '户型结构错层']
# 提取有效的 house_structure 类型
data['house_structure'] = data['house_structure'].apply(lambda x: x if x in valid_structures else None)
# 用众数填充异常数据
mode_structure = data['house_structure'].mode()[0]
data['house_structure'].fillna(mode_structure, inplace=True)
查看处理后数据:
11. 处理套内面积,提取有效数据并且转换类型,对暂无数据进行判断是否超过阀值否则使用众数填充,定义阀值为0.5
遍历查看数据:
代码处理:
# 提取 house_inner_area 列中的数值部分
def extract_area(area):
match = re.match(r'套内面积([\d.]+)㎡', str(area))
if match:
return float(match.group(1))
return None
data['house_inner_area'] = data['house_inner_area'].apply(extract_area)
# 计算缺失值的比例
missing_ratio = data['house_inner_area'].isna().mean()
# 阀值设为 0.
threshold = 0.5
if missing_ratio > threshold:
print("Warning: 超过阀值 0.5,删除 house_inner_area 列")
data.drop(columns=['house_inner_area'], inplace=True)
else:
# 用众数填充无法提取数值的项
mode_area = data['house_inner_area'].mode()[0]
data['house_inner_area'].fillna(mode_area, inplace=True)
# 转换为数值类型
data['house_inner_area'] = data['house_inner_area'].astype(float)
查看结果:
可以看到超过阀值,所以不考虑该列
12. 处理house_building_type的异常数据
查看该列的唯一值,可以看到有3种异常值,一种未知数据,我们设定标准类型,对异常数据进行先查看占比,如果超过0.4阀值,则不考虑该列
代码处理:
# 定义建筑类型的有效值
valid_building_types = {'建筑类型板楼', '建筑类型塔楼', '建筑类型板塔结合', '建筑类型平房'}
# 阀值设为 0.4
threshold = 0.4
# 判断异常数据占比
invalid_ratio = data['house_building_type'].apply(lambda x: x not in valid_building_types).mean()
if invalid_ratio > threshold:
print(f"Warning: Invalid values in 'house_building_type' exceed the threshold of {threshold}, deleting the column.")
data.drop(columns=['house_building_type'], inplace=True)
else:
# 使用众数填充异常值
mode_building_type = data[data['house_building_type'].isin(valid_building_types)]['house_building_type'].mode()[0]
data['house_building_type'] = data['house_building_type'].apply(lambda x: mode_building_type if x not in valid_building_types else x)
查看处理后的唯一值:
13. 处理house_building_structure异常数据
查看该列的唯一值,可以看到结构数据掺杂了类型数据,还有一些未知结构,我们先判断是否超过规定的阀值0.4,如果没有对于这些数据进行众数填充。
代码处理:
# 定义建筑结构的有效值
valid_building_structures = {'建筑结构混合结构', '建筑结构框架结构', '建筑结构钢混结构', '建筑结构砖混结构', '建筑结构钢结构', '建筑结构砖木结构'}
# 阀值设为 0.4
threshold = 0.4
# 判断异常数据占比
invalid_ratio = data['house_building_structure'].apply(lambda x: x not in valid_building_structures).mean()
if invalid_ratio > threshold:
print(f"Warning: Invalid values in 'house_building_structure' exceed the threshold of {threshold}, deleting the column.")
data.drop(columns=['house_building_structure'], inplace=True)
else:
# 使用众数填充异常值
mode_building_structure = data[data['house_building_structure'].isin(valid_building_structures)]['house_building_structure'].mode()[0]
data['house_building_structure'] = data['house_building_structure'].apply(lambda x: mode_building_structure if x not in valid_building_structures else x)
查看处理后的数据唯一值
14. 处理装修情况数据house_decoration
查看唯一值
可以看到有很多异常情况,进行判断异常值占比是否超过阀值,如果大于则删除该列,反之使用众数填充
# 定义装修情况的有效值
valid_decorations = {'装修情况精装', '装修情况毛坯', '装修情况简装', '装修情况其他'}
# 阀值设为 0.4
threshold = 0.4
# 判断异常数据占比
invalid_ratio = data['house_decoration'].apply(lambda x: x not in valid_decorations).mean()
if invalid_ratio > threshold:
print(f"Warning: Invalid values in 'house_decoration' exceed the threshold of {threshold}, deleting the column.")
data.drop(columns=['house_decoration'], inplace=True)
else:
# 使用众数填充异常值
mode_decoration = data[data['house_decoration'].isin(valid_decorations)]['house_decoration'].mode()[0]
data['house_decoration'] = data['house_decoration'].apply(lambda x: mode_decoration if x not in valid_decorations else x)
查看唯一值结果:
15. 处理楼户数据house_elevator_sytle(楼户比例越小越清净)
首先判断缺失值是否超过0.4,如果没有进行众数填充,然后对数据进行拆分为两列数据,一个是电梯数量,还有一个是可以给多少户使用
# 中文数字到阿拉伯数字的映射
chinese_to_arabic = {
'一': 1, '二': 2, '两': 2, '三': 3, '四': 4,
'五': 5, '六': 6, '七': 7, '八': 8, '九': 9,
'十': 10, '十一': 11, '十二': 12, '十三': 13,
'十四': 14, '十五': 15, '十六': 16, '十七': 17,
'十八': 18, '十九': 19, '二十': 20, '二十一': 21,
'二十二': 22, '二十三': 23, '二十四': 24, '二十五': 25,
'二十六': 26, '二十七': 27, '二十八': 28, '二十九': 29,
'三十': 30, '三十一': 31, '三十二': 32, '三十三': 33,
'三十四': 34, '三十五': 35, '三十六': 36, '三十七': 37,
'三十八': 38, '三十九': 39, '四十': 40, '四十一': 41,
'四十二': 42, '四十三': 43, '四十四': 44, '四十五': 45,
'四十六': 46, '四十七': 47, '四十八': 48
}
# 处理梯户比例列
def handle_elevator_style(data, column_name='house_elevator_sytle', threshold=0.4):
# 计算 '梯户比例暂无数据' 的占比
unknown_data_count = data[data[column_name] == '梯户比例暂无数据'].shape[0]
total_count = data.shape[0]
unknown_data_ratio = unknown_data_count / total_count
if unknown_data_ratio > threshold:
data.drop(columns=[column_name], inplace=True)
print(f"'{column_name}' 列暂无数据占比为 {unknown_data_ratio:.2%},已删除该列。")
else:
# 用众数填充 '梯户比例暂无数据'
mode_value = data[column_name].mode()[0]
data[column_name] = data[column_name].apply(lambda x: mode_value if x == '梯户比例暂无数据' else x)
# 提取数值部分并转换为数值类型
def extract_elevator_ratio(style):
if isinstance(style, str):
match = re.match(r'梯户比例([一二两三四五六七八九十]+)梯([一二两三四五六七八九十]+)户', style)
if match:
elevators = chinese_to_arabic.get(match.group(1), None)
households = chinese_to_arabic.get(match.group(2), None)
return elevators, households
return None, None
data[['elevator_count', 'household_count']] = data[column_name].apply(
lambda x: pd.Series(extract_elevator_ratio(x))
)
data.drop(columns=[column_name], inplace=True)
# 调用处理函数
handle_elevator_style(data, column_name='house_elevator_sytle')
# 使用众数填充 NaN 值
""" elevator_count_mode = data['elevator_count'].mode()[0]
household_count_mode = data['household_count'].mode()[0]
data['elevator_count'].fillna(elevator_count_mode, inplace=True)
data['household_count'].fillna(household_count_mode, inplace=True) """
# 根据梯户比例计算梯户比
data['elevator_ratio'] = (data['elevator_count'] / data['household_count']).round(2)
import numpy as np
# 根据楼梯数量>0设置为有电梯,等于0设置为无电梯
data['elevator_status'] = np.where(data['elevator_count'] > 0, '有电梯', '无电梯')
# 删除不需要的列
data.drop(columns=[ 'house_elevator'], inplace=True)
# 重置索引
data.reset_index(drop=True, inplace=True)
查看处理后的唯一值
16. 处理电梯数据house_elevator和计算楼户比
遍历查看电梯数据
对于电梯数据,我们不使用众数进行填充,而是使用有的数据进行补充替换,在上面14中,我们对数据进行处理,可以得到楼层配置了多少电梯,如果电梯数大于或等于1我们填充为有电梯,如果为0则为无电梯
代码处理:
# 根据梯户比例计算梯户比
data['elevator_ratio'] = (data['elevator_count'] / data['household_count']).round(2)
import numpy as np
# 根据楼梯数量>0设置为有电梯,等于0设置为无电梯
data['elevator_status'] = np.where(data['elevator_count'] > 0, '有电梯', '无电梯')
# 删除不需要的列
data.drop(columns=[ 'house_elevator'], inplace=True)
# 重置索引
data.reset_index(drop=True, inplace=True)
17. 处理房屋使用年份house_year如果暂无数据占比较大,进行删除该列处理
首先查看唯一值
# 计算"暂无数据"的占比
missing_data_ratio = data['house_years'].value_counts(normalize=True).get('暂无数据', 0)
if missing_data_ratio > 0.4:
print(f"Warning: '暂无数据' ratio in 'house_years' exceeds the threshold of 0.4, deleting the column.")
data.drop(columns=['house_years'], inplace=True)
查看输出结果
可以看到缺失信息数据占比超过0.4我们选择删除
18. 对于房屋末文本信息数据,首先进行数据拆分后面在进行计算占比是否超过0.5阀值,如果超过删除该列
遍历查看数据
进行数据拆分
# 去除字符串前后的空格并标准化文本
data['house_mortgage_info'] = data['house_mortgage_info'].str.strip()
# 提取抵押状态
def extract_mortgage_status(info):
if '无抵押' in info:
return '无抵押'
elif '有抵押' in info:
return '有抵押'
else:
return '未知'
# 提取抵押金额
def extract_mortgage_amount(info):
match = re.search(r'(\d+(\.\d+)?)(万|万元|十几万|几十万|多万)', info)
if match:
amount = match.group(1)
unit = match.group(3)
if unit == '万' or unit == '万元':
return float(amount) * 10000
elif unit == '十几万':
return 100000
elif unit == '几十万':
return 500000
elif unit == '多万':
return float(amount) * 10000
return '未知'
# 提取抵押银行
def extract_mortgage_bank(info):
banks = ['中国银行', '建设银行', '工商银行', '农业银行', '邮政银行', '平安银行', '光大银行', '中信银行', '招商银行', '交通银行']
for bank in banks:
if bank in info:
return bank
return '未知'
# 提取还款责任
def extract_repayment_responsibility(info):
if '业主自还' in info:
return '业主自还'
elif '客户偿还' in info:
return '客户偿还'
else:
return '未知'
# 应用特征提取函数
data['抵押状态'] = data['house_mortgage_info'].apply(extract_mortgage_status)
data['抵押金额'] = data['house_mortgage_info'].apply(extract_mortgage_amount)
data['抵押银行'] = data['house_mortgage_info'].apply(extract_mortgage_bank)
data['还款责任'] = data['house_mortgage_info'].apply(extract_repayment_responsibility)
# 删除原始列
data = data.drop(columns=['house_mortgage_info'])
查看数据唯一值
# 获取新列的唯一值
unique_values = {
'抵押状态': data['抵押状态'].unique(),
'抵押金额': data['抵押金额'].unique(),
'抵押银行': data['抵押银行'].unique(),
'还款责任': data['还款责任'].unique()
}
print(unique_values)
# 获取新列的唯一值和数量
unique_values_counts = {
'抵押状态': data['抵押状态'].value_counts(),
'抵押金额': data['抵押金额'].value_counts(),
'抵押银行': data['抵押银行'].value_counts(),
'还款责任': data['还款责任'].value_counts()
}
print(unique_values_counts)
计算未知值占比,对于超过阀值0.5的列进行删除
# 计算每列未知数据的占比
unknown_ratios = {
'抵押状态': data['抵押状态'].value_counts(normalize=True).get('未知', 0),
'抵押金额': data['抵押金额'].value_counts(normalize=True).get('未知', 0),
'抵押银行': data['抵押银行'].value_counts(normalize=True).get('未知', 0),
'还款责任': data['还款责任'].value_counts(normalize=True).get('未知', 0)
}
print(unknown_ratios)
# 删除未知数据占比超过50%的列
columns_to_delete = [col for col, ratio in unknown_ratios.items() if ratio > 0.5]
data = data.drop(columns=columns_to_delete)
# 用众数填充未知值
for col in data.columns:
if data[col].dtype == 'object': # 对于类别型数据
if '未知' in data[col].values:
mode = data[col].mode().iloc[0] if not data[col].mode().empty else '无'
data[col] = data[col].replace('未知', mode)
else: # 对于数值型数据
mode = data[col].mode().iloc[0] if not data[col].mode().empty else 0
data[col] = data[col].fillna(mode)
# 检查处理后的数据
data.head()
输出结果
19. 我们进行删除无用列,这些列对后面分析没有很大意义的列,还有对重复行进行再次去除
首先进行查看转换处理后的数据特征
# 删除house_book,city列
data.drop(columns=['house_book', 'city'], inplace=True)
# 移除 house_fx_id 列
data.drop(columns=['house_fx_id'], inplace=True)
# 去除重复行
data.drop_duplicates(inplace=True)
20. 查看最终处理结果,保存结果
# 查看处理后数据的基本信息
print(data.info())
# 保存数据
data.to_csv('./final.csv', index=False, encoding='utf-8')