目录
1. 整体项目中的功能定位
多模态聚类器在项目中的作用是什么呢?
前端时间我构建了特征库(具体见【博客链接】),当我们接受到一个新的垃圾图片时,我们会与特征库中四大垃圾类别进行比较:如果可以被归到某一个类别,这里不做特殊处理;反之,即与四大类别的最近距离也超过了阈值,就会触发异常情况处理机制。
如图1所示,图片会被转化为视觉特征向量+文本特征向量的形式,如果此时预先没有遇到任何异常情况,那么当前的这种异常就会自动被归为一类进行存储并由人工给出处理方案。后续再遇到异常时,会尝试将其聚类到已知的异常情况;如果无法成功,就会新建异常类,人工解决后存储,如此循环往复。
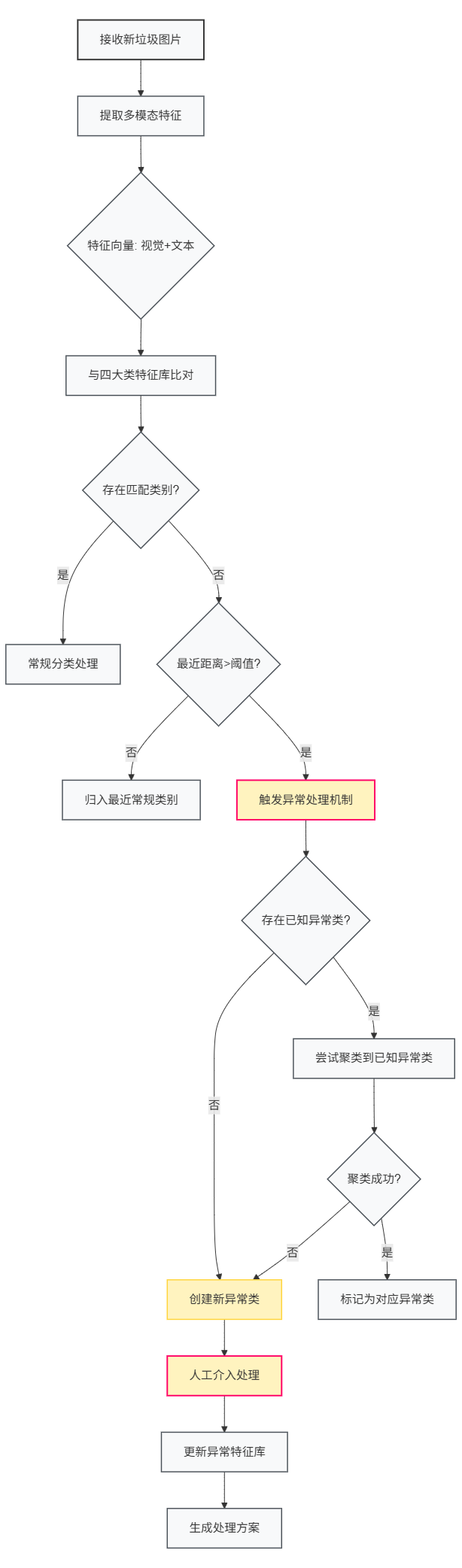
2. 多模态聚类器构建
模型会记录视觉特征权重和文本特征权重,当一个新的数据到来时,会首先对其进行L2归一化,随后会针对每个已知异常类别,计算二者间视觉特征向量的距离和文本特征向量距离,再将两个距离进行加权平均。找到与其最接近的点,判断二者间的距离,如果超过了限定阈值,就会将此异常单独归为一个异常类;反之,则会将此异常归为与其最接近的已知异常类,同时会实时更新聚类中心。
此外,聚类器会将特征向量、样本数据及超参数打包为单一pkl文件'cluster_state.pkl
',避免不必要的重复计算。具体来讲,pkl文件中会包括所有类别的"标准特征",即视觉和文字的平均特征;每个类别下包含的所有具体样本数据;算法参数设置,包括权重、阈值等。核心代码架构如下(省略了实际代码):
class MultiModalDistanceCluster:
def __init__(self, visual_weight: float = 0.6, text_weight: float = 0.4,
threshold: float = 0.5, update_decay: float = 0.9):
"""
多模态聚类器
:param visual_weight: 视觉特征权重(0-1)
:param text_weight: 文本特征权重(0-1)
:param threshold: 新类别阈值(0-1)
:param update_decay: 特征更新衰减系数(0-1)
"""
assert abs(visual_weight + text_weight - 1.0) < 1e-5, "权重之和必须为1"
self.visual_weight = visual_weight
self.text_weight = text_weight
self.threshold = threshold
self.update_decay = update_decay
# 核心数据存储
self.visual_features: List[np.ndarray] = []
self.text_features: List[np.ndarray] = []
self.cluster_samples: List[List[Dict[str, Any]]] = []
def _normalize(self, vec: np.ndarray) -> np.ndarray:
"""L2归一化"""
norm = np.linalg.norm(vec)
return vec / norm if norm != 0 else vec
def add_cluster(self, visual: np.ndarray, text: np.ndarray, sample: Dict[str, Any]):
"""添加新类别"""
... ...
def predict(self, new_sample: Dict[str, Any]) -> Tuple[int, bool]:
"""
预测样本类别
:return: (类别索引, 是否新增)
"""
assert 'visual' in new_sample and 'text' in new_sample, "样本必须包含视觉和文本特征"
new_visual = self._normalize(np.array(new_sample['visual']))
new_text = self._normalize(np.array(new_sample['text']))
if not self.visual_features:
self.add_cluster(new_visual, new_text, new_sample)
return 0, True
# 直接计算距离
... ....
closest_cluster = np.argmin(combined_dist)
if combined_dist[closest_cluster] > self.threshold:
self.add_cluster(new_visual, new_text, new_sample)
return len(self.visual_features) - 1, True
else:
# 更新聚类中心
... ...
def save_state(self, path: str):
"""保存核心状态到单个pkl文件"""
... ...
@classmethod
def load_state(cls, path: str):
"""从pkl文件加载完整状态"""
... ...
下方的图2展示了多模态聚类器的框架图:
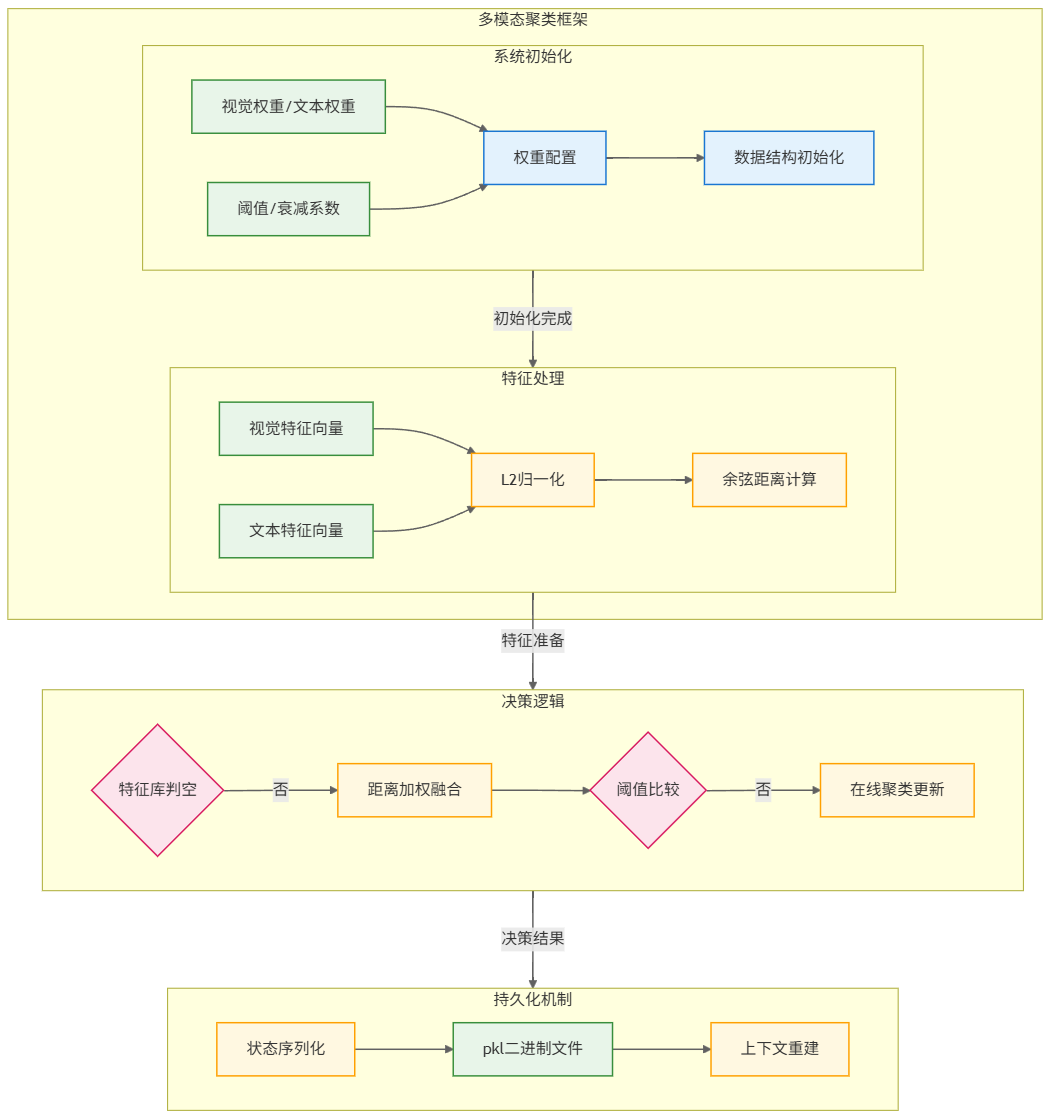
3. 实际使用及示例输出
我针对上述构建好的系统,进行了测试:首先测试了无已知异常库的情况,系统对于新检测到的异常情况可以自动将其归为新的类别,然后暂时将“系统状态存储”,当下次再次检测到异常情况时,可以直接使用,不用重复计算;后续我又设置了若干个测试数据样本,通过调整合适的阈值,观察到系统可以做出有效的分类,系统部分输出如图3所示:
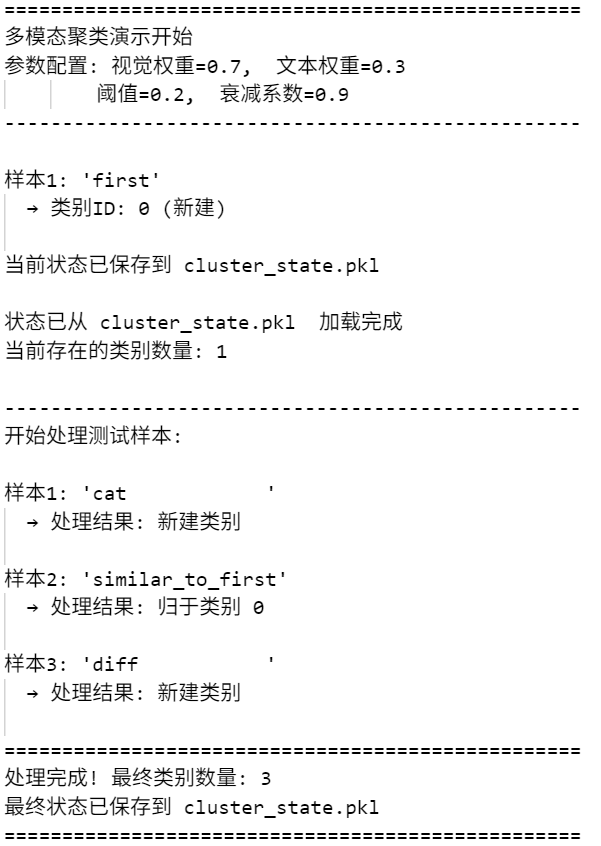
(by S.H.C)