循环神经网络
循环神经网络概述
在前馈神经网络中,信息的传递是单向的,这种限制虽然使得网络变得更容易学习,但在一定程度上也减弱了神经网络模型的能力1。
在生物神经网络中,神经元之间的连接关系要复杂得多。前馈神经网络不能解决的两个问题:
-
前馈神经网络每次输入都是独立的,但是在很多现实任务中,网络的输入不仅和当前时刻的输入相关,也和其过去一段时间的输出相关;
-
前馈网络难以处理时序数据(比如视频、语音、文本等)。时序数据的长度一般是不固定的,而前馈神经网络要求输入和输出的维数都是固定的,不能任意改变。
因此,当处理这一类和时序数据相关的问题时,就需要一种能力更强的模型。
循环神经网络(Recurrent Neural Network,RNN)是一类具有短期记忆能力的神经网络。在循环神经网络中,神经元不但可以接受其他神经元的信息,也可以接受自身的信息,形成具有环路的网络结构。
循环神经网络的参数学习可以通过随时间反向传播算法来学习。随时间反向传播算法即按照时间的逆序将错误信息一步步地往前传递。当输入序列比较长时,会存在梯度爆炸和消失问题 ,也称为长程依赖问题。为了解决这个问题,人们对循环神经网络进行了很多的改进,其中最有效的改进方式引入门控机制(Gating Mechanism)。
此外,循环神经网络可以很容易地扩展到两种更广义的记忆网络模型:递归神经网络和图网络。
给网络增加记忆能力
为了处理这些时序数据并利用其历史信息,我们需要让网络具有短期记忆能力。而前馈网络是一种静态网络,不具备这种记忆能力。
一般来讲,我们可以通过以下三种方法来给网络增加短期记忆能力。
延时神经网络
一种简单的利用历史信息的方法是建立一个额外的延时单元,用来存储网络的历史信息(可以包括输入、输出、隐状态等)。比较有代表性的模型是延时神经网络(Time Delay Neural Network,TDNN)。
延时神经网络是在前馈网络中的非输出层都添加一个延时器,记录神经元的最近几次活性值。在第 t t t 个时刻,第 l l l 层神经元的活性值依赖于第 l − 1 l-1 l−1 层神经元的最近 K K K 个时刻的活性值,即
h t ( l ) = f ( h t ( l − 1 ) , h t − 1 ( l − 1 ) , ⋯ , h t − K ( l − 1 ) ) , h_t^{(l)}=f(h_t^{(l-1)},h_{t-1}^{(l-1)},\cdots,h_{t-K}^{(l-1)}), ht(l)=f(ht(l−1),ht−1(l−1),⋯,ht−K(l−1)),
其中 h t ( l ) ∈ R M l h_t^{(l)}\in\mathbb{R}^{M_l} ht(l)∈RMl 表示第 l l l 层神经元在时刻 t t t 的活性值, M l M_l Ml 为第 l l l 层神经元的数量。通过延时器,前馈网络就具有了短期记忆的能力。
有外部输入的非线性自回归模型
自回归模型(AutoRegressive Model,AR)是统计学上常用的一类时间序列模型,用一个变量 y t y_t yt 的历史信息来预测自己。
y t = ω 0 + ∑ k = 1 K ω k y t − k + ϵ t , y_t=\omega_0+\sum_{k=1}^K\omega_k\textbf{y}_{t-k}+\epsilon_t, yt=ω0+k=1∑Kωkyt−k+ϵt,
其中 K K K 为超参数, ω 0 , ⋯ , ω K \omega_0,\cdots,\omega_K ω0,⋯,ωK 为可学习参数, ϵ t ∼ N ( 0 , σ 2 ) \epsilon_t\sim N(0,\sigma^2) ϵt∼N(0,σ2) 为第 t t t 个时刻的噪声,方差 σ 2 \sigma^2 σ2 和时间无关。
有外部输入的非线性自回归模型(Nonlinear AutoRegressive with Exoge-nous Inputs Model,NARX)是自回归模型的扩展,在每个时刻 t t t 都有一个外部输入 x t x_t xt ,产生一个输出 y t y_t yt 。NARX 通过一个延时器记录最近 K x K_x Kx 次的外部输入和最近 K y K_y Ky 次的输出,第 t t t 个时刻的输出 y t y_t yt 为
y t = f ( x t , x t − 1 , ⋯ , x t − K x , y t − 1 , y t − 2 , ⋯ , y t − K y ) , y_t=f(x_t,x_{t-1},\cdots,x_{t-K_x},y_{t-1},y_{t-2},\cdots,y_{t-K_y}), yt=f(xt,xt−1,⋯,xt−Kx,yt−1,yt−2,⋯,yt−Ky),
其中 f ( ⋅ ) f(\cdot) f(⋅) 表示非线性函数,可以是一个前馈网络, K x K_x Kx 和 K y K_y Ky 为超参数。
循环神经网络
循环神经网络(Recurrent Neural Network,RNN)通过使用带自反馈的神经元,能够处理任意长度的时序数据。
给定一个输入序列 x 1 : T = ( x 1 , x 2 , ⋯ , x t , ⋯ , x T ) \textbf{x}_{1:T}=(\textbf{x}_1,\textbf{x}_2,\cdots,\textbf{x}_t,\cdots,\textbf{x}_T) x1:T=(x1,x2,⋯,xt,⋯,xT),循环神经网络通过下面公式更新带反馈边的隐藏层的活性值 h t \textbf{h}_t ht:
h t = f ( h t − 1 , x t ) \textbf{h}_t=f(\textbf{h}_{t-1},\textbf{x}_t) ht=f(ht−1,xt)
其中 h 0 = 0 , f ( ⋅ ) \textbf{h}_0=0,f(\cdot) h0=0,f(⋅) 为一个非线性函数,可以是一个前馈网络。
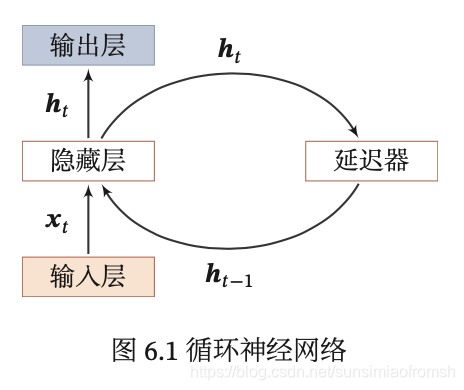
从数学上讲,公式 h t = f ( h t − 1 , x t ) \textbf{h}_t=f(\textbf{h}_{t-1},\textbf{x}_t) ht=f(ht−1