前言
遥感技术很早就开始用于洪涝灾害调查。遥感技术可以利用卫星获取的信息,连续、及时、准确地把洪灾情况上报给中央和地方防汛部门,并通过对不同卫星数据的融合处理,综合利用各自的优势,清晰地反映出洪灾态势。本文基于GEE
在本博客中,我们将使用Landsat8绘制柬埔寨的水体。我们将应用云移除并计算NDWI。
1、输入柬埔寨的行政边界
//Get the study area country boundary
var StudyArea = ee.FeatureCollection("projects/servir-mekong/Cambodia-Dashboard-tool/boundaries/cambodia_country");
//Add Shape file layer to the Map
Map.addLayer(StudyArea,{},"Cambodia");
//Center the Map with the Study Area
Map.centerObject(StudyArea, 8);
2、为柬埔寨导入landsat 8数据
// Get a Landsat-8 TOA collection.
var L8_collection = ee.ImageCollection('LANDSAT/LC08/C01/T1_RT_TOA');
//Filter L8 image collection using shape file (or) boundary
var L8_StudyArea = L8_collection.filterBounds(StudyArea);
3、我们筛选 2020 年
// Define time range
var startyear = 2019;
var endyear = 2020;
// Set date in ee date format
var startdate = ee.Date.fromYMD(startyear,1,1);
var enddate = ee.Date.fromYMD(endyear,12,31);
//Filter L8 Image Collection with Date
var L8_SA_Date = L8_StudyArea.filterDate(startdate,enddate);
print("Total Images:",L8_SA_Date.size());
4、我们使用云函数从图像中移除云
//Create Cloud Masking Function to remove clouds
var cloudfunction = function(image){
// set cloud threshold
var cloud_thresh = 40;
//use add the cloud likelihood band to the image
var CloudScore = ee.Algorithms.Landsat.simpleCloudScore(image);
//isolate the cloud likelihood band
var quality = CloudScore.select('cloud');
//get pixels above the threshold
var cloud01 = quality.gt(cloud_thresh);
//create a mask from high likelihood pixels
var cloudmask = image.mask().and(cloud01.not());
//mask those pixels from the image
return image.updateMask(cloudmask);
};
// mask all clouds in the image collection
var L8_NoCloud = L8_SA_Date.map(cloudfunction);
Map.addLayer(L8_NoCloud.mosaic().clip(StudyArea), { min:0.05, max: 0.8, bands: ['B6', 'B5', 'B4']},'Landsat 8 in study region');
5、我们计算集合中每个图像的 NDWI
// Create function to calculate NDWI from landsat 8
//NDWI is Normalized Difference Water Index of NIR (B5) and Green (B3) bands
function Calculate_ndwi(img) {
var ndwi = img.normalizedDifference(['B3', 'B5']).rename('NDWI');
return img.addBands(ndwi);
}
var L8_ndwi = L8_NoCloud.map(Calculate_ndwi);
6、我们计算 2 个百分位数并使用该信息推导出被淹没的区域
//Set the threshold to difference between Dry and Wet Seasons
var DIFF_THRESHOLD = 0.4;
// select dry and wet conditions
var dry = L8_ndwi.select("NDWI").reduce(ee.Reducer.percentile([10]));
var wet = L8_ndwi.select("NDWI").reduce(ee.Reducer.percentile([90]));
var diff = wet.subtract(dry);
var indundatedArea = diff.updateMask(diff.gt(DIFF_THRESHOLD)).clip(StudyArea);
// add layers
Map.addLayer(dry.clip(StudyArea),{min:-0.3, max:0.4, palette:"white,blue,darkblue"},"dry");
Map.addLayer(wet.clip(StudyArea),{min:-0.3, max:0.8, palette:"white,blue,darkblue"}, "wet");
Map.addLayer(indundatedArea,{palette:"purple"}, "Indundated Area");
7、我们用道路网络覆盖淹没区域
//import road feature
var roads = ee.FeatureCollection('projects/servir-mekong/osm/cambodia/gis_osm_roads');
// create lists to categorize the road networks
var primary = ["primary","primary_link"];
var secondary = ["secondary","secondary_link"];
var tertiary = ["tertiary","tertiary_link"];
var other = ee.List(roads.aggregate_histogram("fclass").keys()).removeAll(primary).removeAll(secondary).removeAll(tertiary);
// filter for primary, secondary and tertiary roads
var primaryRoads = roads.filter(ee.Filter.inList("fclass",primary));
var secondaryRoads = roads.filter(ee.Filter.inList("fclass",secondary));
var tertiaryRoads = roads.filter(ee.Filter.inList("fclass",tertiary));
var otherRoads = roads.filter(ee.Filter.inList("fclass",other));
Map.addLayer(primaryRoads.draw("red"),{},"primary roads")
Map.addLayer(secondaryRoads.draw("blue"),{},"secondary roads")
Map.addLayer(tertiaryRoads.draw("green"),{},"tertiary roads")
Map.addLayer(otherRoads.draw("gray"),{},"other roads")
8、最终效果
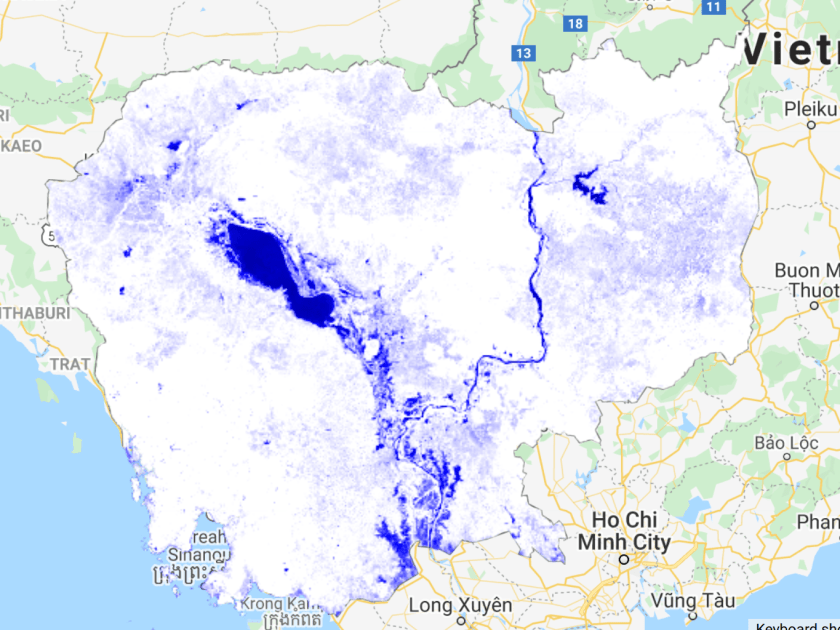