SVM分类
线性SVM分类
- SVM是二分类器,线性SVM分类是画出一条决策边界,使得到两个类样本的最短距离最大。
- 主要有hard margin classification与soft margin classification,可以通过调节超参数来调节margin
- SVM直接输出样本的列别,而不是像Logistic回归那样,输出概率
- sklearn中,有多种方式实现线性SVM
- LinearSVC
- SVC中,kernel设置为linear
- SGDClassifier中,loss设置为hinge,alpha设置为1/(m*C)
import numpy as np
from sklearn import datasets
from sklearn.pipeline import Pipeline
from sklearn.preprocessing import StandardScaler
from sklearn.svm import LinearSVC
iris = datasets.load_iris()
X = iris["data"][:, (2,3)]
y = (iris["target"] == 2).astype( np.float64 )
svm_clf = Pipeline(( ("scaler", StandardScaler()),
("linear_svc", LinearSVC(C=1, loss="hinge")) ,))
svm_clf.fit( X, y )
res = svm_clf.predict( [[5.5, 1.7]] )
print( res )
[ 1.]
非线性SVM
- 一般现实问题中,线性模型难以解决问题,可以采用非线性SVM
- 对于非线性回归问题,可以先添加一个多项式的特征,即将一维数据转化为多项式数据,然后采用线性SVM;或者也可以直接采用多项式的kernel,直接进行非线性SVM的分类
- SVC中的参数C越大,对于训练集来说,其误差越小,但是很容易发生过拟合;C越小,则允许有更多的训练集误分类,相当于soft margin
- SVC中的参数coef0反映了高阶多项式相对于低阶多项式对模型的影响,如果发生了过拟合的现象,则可以减小coef0;如果发生了欠拟合的现象,可以试着增大coef0
%matplotlib inline
import matplotlib.pyplot as plt
from sklearn.datasets import make_moons
from sklearn.preprocessing import PolynomialFeatures
X, y = make_moons( n_samples=100, noise=0.15, random_state=42 )
def plot_dataset(X, y, axes):
plt.plot( X[:,0][y==0], X[:,1][y==0], "bs" )
plt.plot( X[:,0][y==1], X[:,1][y==1], "g^" )
plt.axis( axes )
plt.grid( True, which="both" )
plt.xlabel(r"$x_l$")
plt.ylabel(r"$x_2$")
def plot_predict(clf, axes):
x0s = np.linspace(axes[0], axes[1], 100)
x1s = np.linspace(axes[2], axes[3], 100)
x0, x1 = np.meshgrid( x0s, x1s )
X = np.c_[x0.ravel(), x1.ravel()]
y_pred = clf.predict( X ).reshape( x0.shape )
y_decision = clf.decision_function( X ).reshape( x0.shape )
plt.contour( x0, x1, y_pred, cmap=plt.cm.winter, alpha=0.5 )
plt.contour( x0, x1, y_decision, cmap=plt.cm.winter, alpha=0.2 )
polynomial_svm_clf = Pipeline([ ("poly_featutres", PolynomialFeatures(degree=3)),
("scaler", StandardScaler()),
("svm_clf", LinearSVC(C=10, loss="hinge", random_state=42) )
])
polynomial_svm_clf.fit( X, y )
plot_dataset( X, y, [-1.5, 2.5, -1, 1.5] )
plot_predict( polynomial_svm_clf, [-1.5, 2.5, -1, 1.5] )
plt.show()
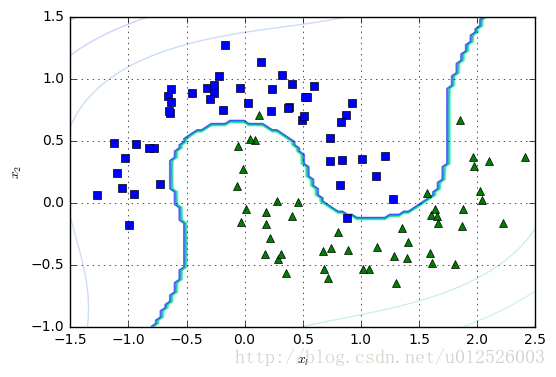
from sklearn.svm import SVC
poly_kernel_svm_clf = Pipeline([ ( "scaler", StandardScaler()),
("svm_clf", SVC(kernel="poly", degree=3, coef0=1, C=0.5))
])
poly_kernel_svm_clf.fit(X,y)
plt.figure( figsize=(9,3) )
plt.subplot(131)
plot_dataset( X, y, [-1.5, 2.5, -1, 1.5] )
plot_predict( poly_kernel_svm_clf, [-1.5, 2.5, -1, 1.5] )
poly_kernel_svm_clf = Pipeline([ ( "scaler", StandardScaler()),
("svm_clf", SVC(kernel="poly", degree=3, coef0=1, C=10))
])
poly_kernel_svm_clf.fit(X,y)
plt.subplot(132)
plot_dataset( X, y, [-1.5, 2.5, -1, 1.5] )
plot_predict( poly_kernel_svm_clf, [-1.5, 2.5, -1, 1.5] )
poly_kernel_svm_clf = Pipeline([ ( "scaler", StandardScaler()),
("svm_clf", SVC(kernel="poly", degree=3, coef0=100, C=0.5))
])
poly_kernel_svm_clf.fit(X,y)
plt.subplot(133)
plot_dataset( X, y, [-1.5, 2.5, -1, 1.5] )
plot_predict( poly_kernel_svm_clf, [-1.5, 2.5, -1, 1.5] )
plt.show()
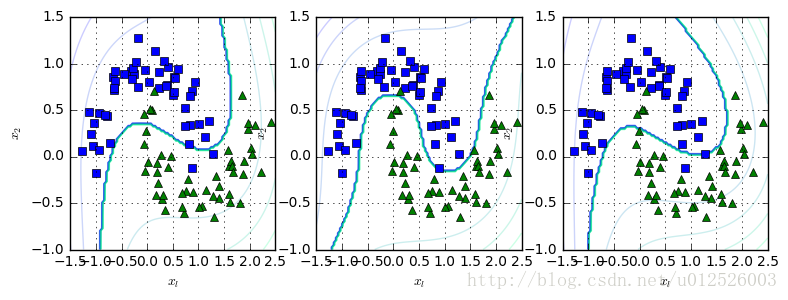
Gasuusian RBF核函数
- 当数据在低维空间中不可分割的时候,可以尝试将它们映射到高维空间,通过核函数来进行这样的映射操作,Gaussian RBF核函数为
ϕγ(x,l)=e(−γ∥x−l∥2)
复杂度分析
- skleanr中,SVM是利用了libsvm,线性SVM的时间复杂度为
O(mn)
,带有核函数的SVM一般是
O(m2n)
与
O(m3n)
之间
- SVM一般适用于数据量不是很大,但是很复杂的问题的求解。
rbf_kernel_svm_clf = Pipeline([
("scaler", StandardScaler()),
("svm_clf", SVC(kernel="rbf", gamma=5, C=0.001))
])
plt.figure(figsize=(6,3))
plt.subplot(121)
rbf_kernel_svm_clf.fit( X, y )
plot_dataset( X, y, [-1.5, 2.5, -1, 1.5] )
plot_predict( rbf_kernel_svm_clf, [-1.5, 2.5, -1, 1.5] )
rbf_kernel_svm_clf = Pipeline([
("scaler", StandardScaler()),
("svm_clf", SVC(kernel="rbf", gamma=0.1, C=0.001))
])
plt.subplot(122)
rbf_kernel_svm_clf.fit( X, y )
plot_dataset( X, y, [-1.5, 2.5, -1, 1.5] )
plot_predict( rbf_kernel_svm_clf, [-1.5, 2.5, -1, 1.5] )
plt.show( )
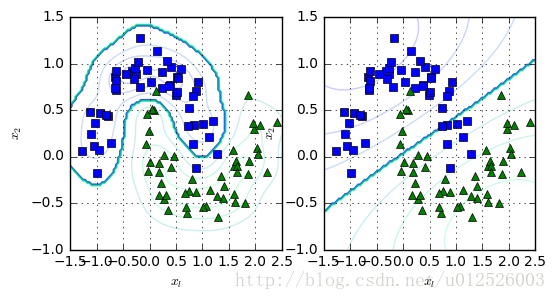
SVM回归
线性SVM
- SVM也可以用于回归问题,有线性SVM与非线性SVM回归
- 线性回归时,有一个变量
ε
,这是SVM的margin
非线性SVM回归
- 多项式回归,指定SVM的kernel为poly即可
from sklearn.svm import LinearSVR
np.random.seed( 42 )
m = 50
X = 2*np.random.rand(m, 1)
y = (4+3*X+np.random.randn(m,1)).ravel()
def find_support_vectors(svm_reg, X, y):
y_pred = svm_reg.predict( X )
off_margin = np.abs(y-y_pred) >= svm_reg.epsilon
return np.argwhere( off_margin )
def plot_svm_regression( svm_reg, X, y, axes ):
x1s = np.linspace( axes[0], axes[1], 100 ).reshape(-1,1)
y_pred = svm_reg.predict(x1s)
plt.plot( x1s, y_pred, "r-", linewidth=2, label=r"$\hat{y}$" )
plt.plot( x1s, y_pred-svm_reg.epsilon, "k--" )
plt.plot( x1s, y_pred+svm_reg.epsilon, "k--" )
plt.plot( X, y, "bo" )
plt.scatter( X[svm_reg.support_], y[svm_reg.support_], s=180, facecolors="#FFAAAA" )
plt.xlabel(r"$x_1$", fontsize=18)
plt.legend(loc="upper left", fontsize=18)
plt.axis(axes)
svm_reg_1 = LinearSVR(epsilon=1.5, random_state=42)
svm_reg_2 = LinearSVR(epsilon=0.5, random_state=42)
svm_reg_1.fit( X, y )
svm_reg_2.fit( X, y )
svm_reg_1.support_ = find_support_vectors( svm_reg_1, X, y )
svm_reg_2.support_ = find_support_vectors( svm_reg_2, X, y )
eps_x1 = 1
eps_y_pred = svm_reg_1.predict([[eps_x1]])
plt.figure( figsize=(8,3) )
plt.subplot(121)
plot_svm_regression( svm_reg_1, X, y, [0, 2, 3, 11] )
plt.title(r"$\epsilon = {}$".format(svm_reg_1.epsilon), fontsize=18)
plt.ylabel(r"$y$", fontsize=18, rotation=0)
plt.annotate(
'', xy=(eps_x1, eps_y_pred), xycoords='data',
xytext=(eps_x1, eps_y_pred - svm_reg_1.epsilon),
textcoords='data', arrowprops={'arrowstyle': '<->', 'linewidth': 1.5}
)
plt.text(0.9, 5.6, r"$\epsilon$", fontsize=20)
plt.subplot(122)
plot_svm_regression(svm_reg_2, X, y, [0, 2, 3, 11])
plt.title(r"$\epsilon = {}$".format(svm_reg_2.epsilon), fontsize=18)
plt.show()
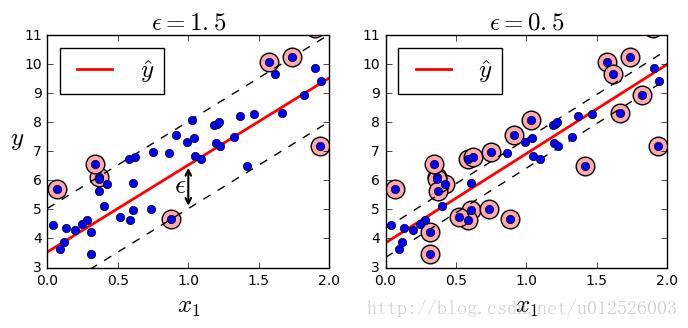
from sklearn.svm import SVR
np.random.seed(42)
m = 100
X = 2 * np.random.rand(m, 1) - 1
y = (0.2 + 0.1 * X + 0.5 * X**2 + np.random.randn(m, 1)/10).ravel()
svm_poly_reg1 = SVR(kernel="poly", degree=2, C=100, epsilon=0.1)
svm_poly_reg2 = SVR(kernel="poly", degree=2, C=0.01, epsilon=0.1)
svm_poly_reg1.fit(X, y)
svm_poly_reg2.fit(X, y)
plt.figure(figsize=(8, 3))
plt.subplot(121)
plot_svm_regression(svm_poly_reg1, X, y, [-1, 1, 0, 1])
plt.title(r"$degree={}, C={}, \epsilon = {}$".format(svm_poly_reg1.degree, svm_poly_reg1.C, svm_poly_reg1.epsilon), fontsize=18)
plt.ylabel(r"$y$", fontsize=18, rotation=0)
plt.subplot(122)
plot_svm_regression(svm_poly_reg2, X, y, [-1, 1, 0, 1])
plt.title(r"$degree={}, C={}, \epsilon = {}$".format(svm_poly_reg2.degree, svm_poly_reg2.C, svm_poly_reg2.epsilon), fontsize=18)
plt.show()
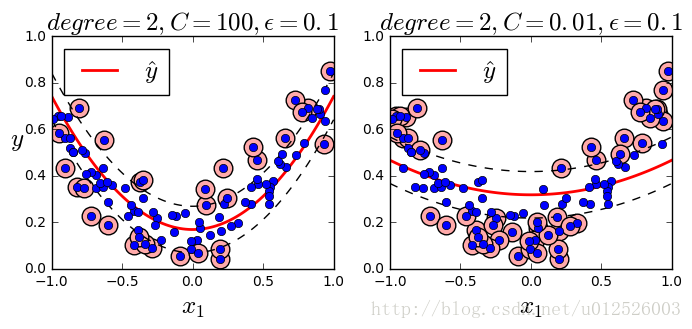
SVM的一些探讨
- hard margin模式时,线性SVM分类的预测方法为
y^={01ifwTx+b<0,ifwTx+b≥0
- 减小权重,会增大margin
- software margin的SVM分类器的目标函数为
minw,b,ζs.t.12wTw+C∑i=1mζ(i)t(i)(wTx(i)+b)≥1−ζ(i)andζ(i)≥0fori=1,2,⋯,m
可以将原始问题转化为对偶问题 - 对于kernel SVM来说,解决方法也是类似,不过可以化简核函数对样本之间的求解
- SVM一般是offline的,因为训练SVM可以需要大量时间,如果需要online SVM,即模型随着数据量的不断增加而不断改进,有一种原始的方法是使用梯度下降的方法,最小化代价函数,但是这种方法的收敛速度远小于QP方法
- 关于SVM更多的推导:http://www.cnblogs.com/LeftNotEasy/archive/2011/05/02/basic-of-svm.html