http://blog.csdn.net/jiandanjinxin/article/details/70835495
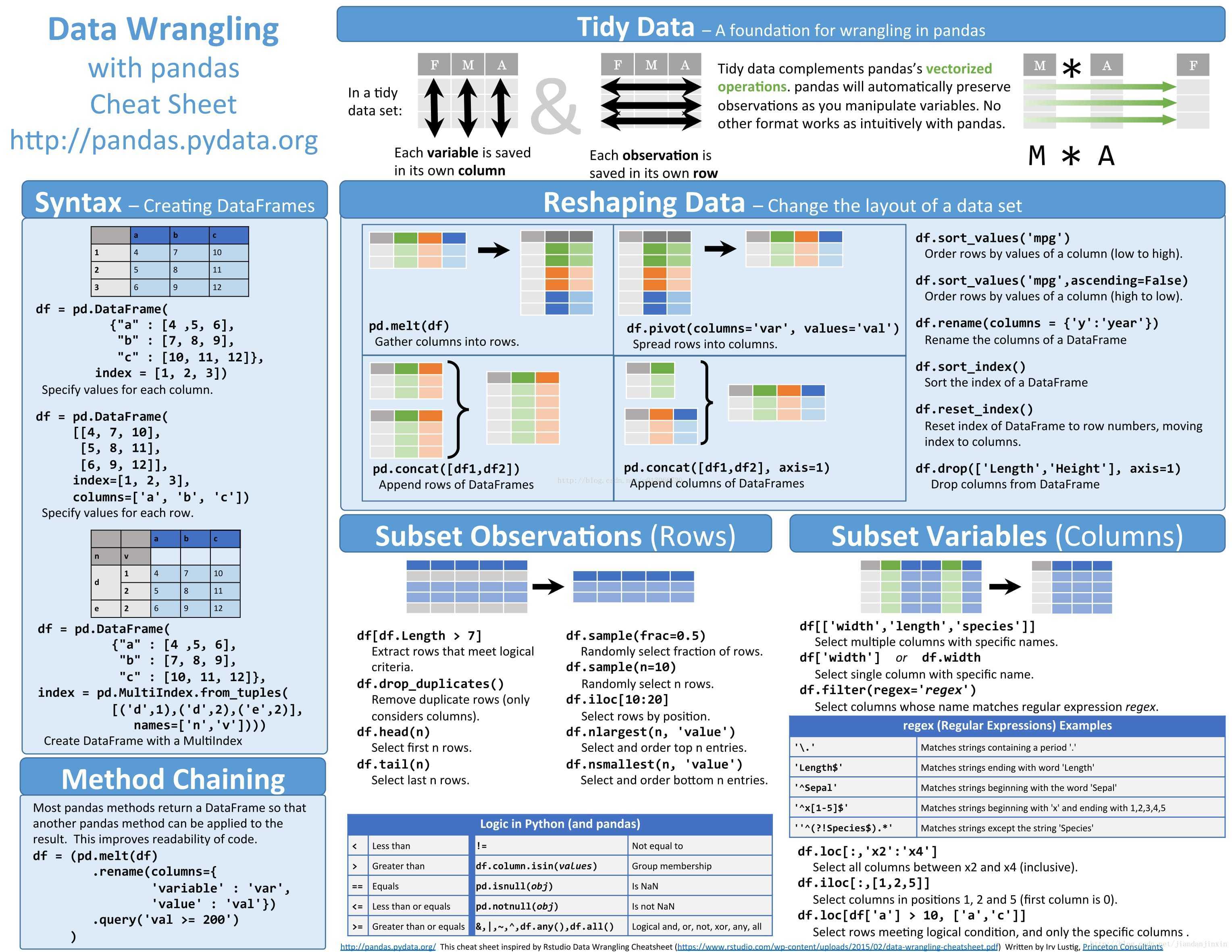
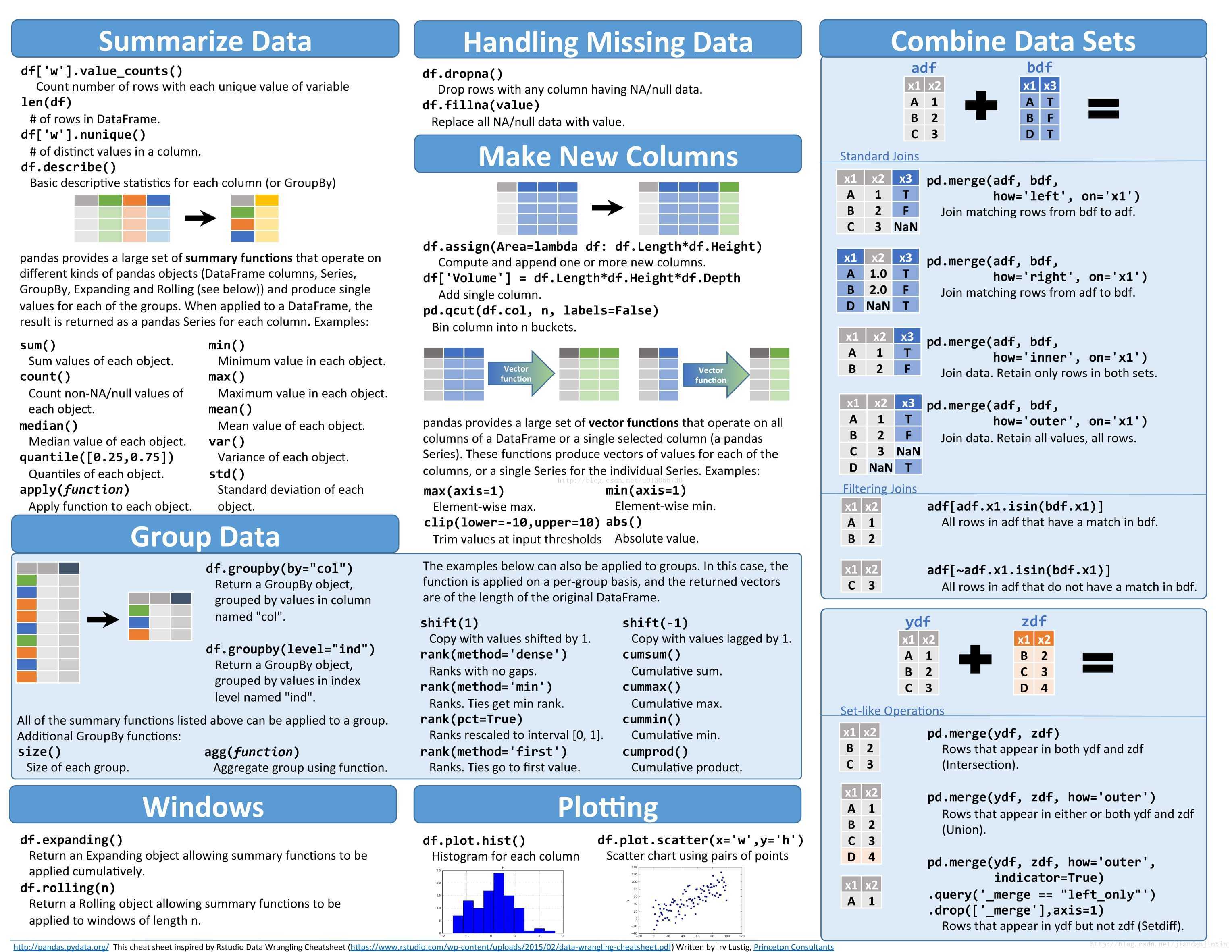
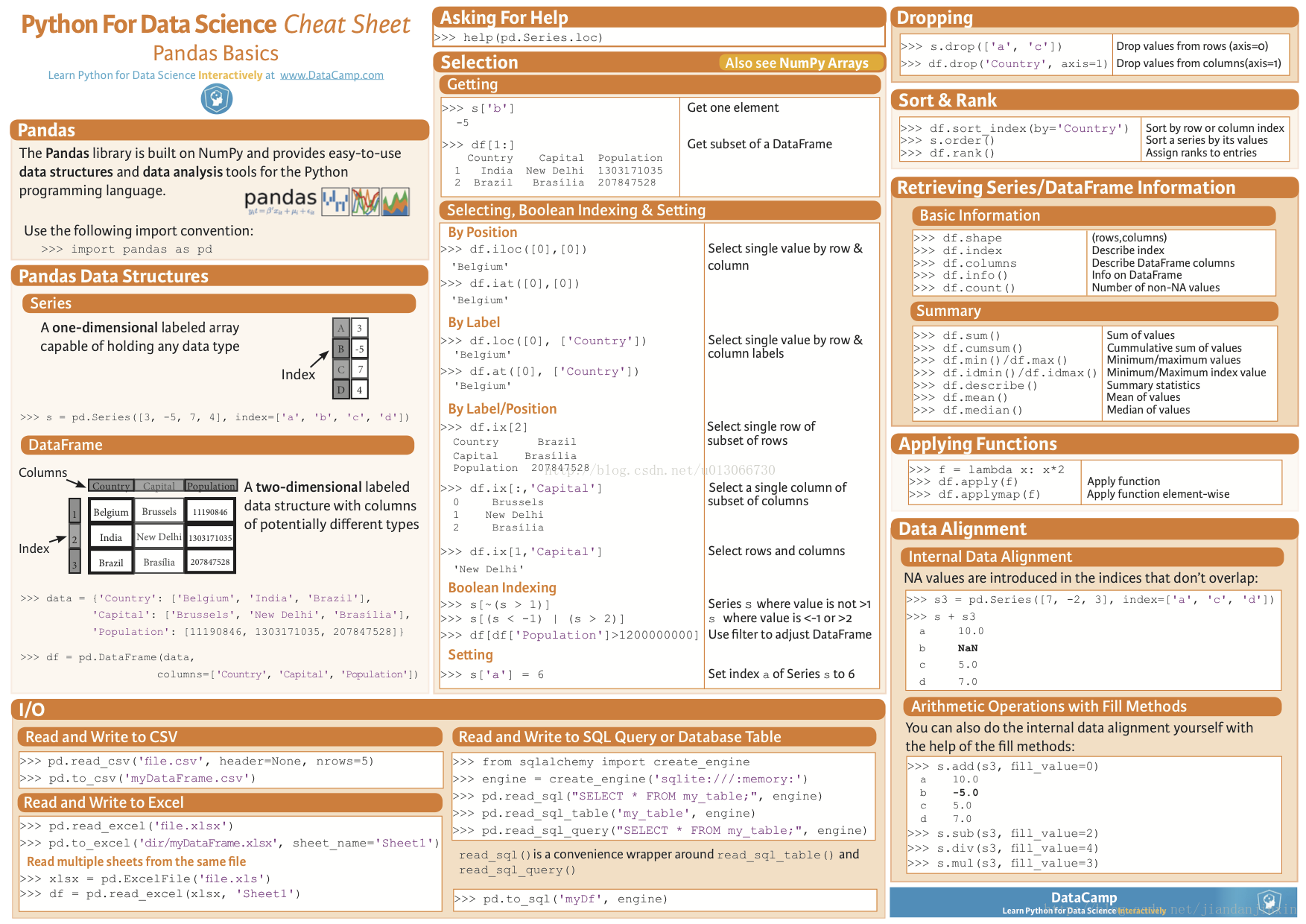
Data Analysis with Python and Pandas Tutorial Introduction
numpy是序列化的矩阵或者序列
pandas是字典形式的numpy,可给不同行列进行重新命名
Pandas 小抄
1. Reading and Writing Data
import pandas as pd
#a. Reading a csv file
df=pd.read_csv('Analysis.cav')
#b. Writing content of data frame to csv file
df.to_csv('werfer.csv')
# c.Reading an Excel file
df=pd.read_excel('sdfsdgsd.xlsx', 'sheeet1')
#d. Writing content of data frame to Excel file
df.to_excel('sddg.xlsx', sheet_name='sheet2')
# pandas 导入导出,读取和储存
# The pandas I/O API is a set of top level reader functions accessed like
# pd.read_csv() that pandas object.
# read_csv # excel files
# read_excel
# read_hdf
# read_sql
# read_json
# read_msgpack(experimental)
# read_html
# read_gbq(experimental)
# read_stata
# read_sas
# read_clipboard
# read_pickle #自带的亚索
# The corresponding writer functions are object methods that are accessed like
# df.to_csv
# to_csv
# to_excel
# to_hdf
# to_sql
# to_json
# to_msgpack
# to_html
# to_gbq
# to_stata
# to_clipboard
# to_pickle
import pandas as pd
data = pd.read_csv('student.csv')
print(data)
data.to_packle('student.pickle')
- 1
- 2
- 3
- 4
- 5
- 6
- 7
- 8
- 9
- 10
- 11
- 12
- 13
- 14
- 15
- 16
- 17
- 18
- 19
- 20
- 21
- 22
- 23
- 24
- 25
- 26
- 27
- 28
- 29
- 30
- 31
- 32
- 33
- 34
- 35
- 36
- 37
- 38
- 39
- 40
- 1
- 2
- 3
- 4
- 5
- 6
- 7
- 8
- 9
- 10
- 11
- 12
- 13
- 14
- 15
- 16
- 17
- 18
- 19
- 20
- 21
- 22
- 23
- 24
- 25
- 26
- 27
- 28
- 29
- 30
- 31
- 32
- 33
- 34
- 35
- 36
- 37
- 38
- 39
- 40
2. Getting Preview of Dataframe
#a.Looking at top n record
df.head(5)
#b.Looking at bottom n record
df.tail(5)
#c.View columns name
df.columns
—————————————————
***3. Rename Columns of Data Frame***
#a. Rename method helps to rename column of data frame
df2 = df.rename(columns={'old_columnname':'new_columnname'})
#This method will create a new data frame with new column name.
#b.To rename the column of existing data frame, set inplace=True.
df.rename(columns={'old_columnname':'new_columnname'}, inplace=True)
—————————————————
***4. Selecting Columns or Rows***
#a. Accessing sub data frames
df[['column1','column2']]
#b.Filtering Records
df[df['column1']>10]
df[(df['column1']>10) & df['column2']==30]
df[(df['column1']>10) | df['column2']==30]
# pandas 数据选择
import pandas as pd
import numpy as np
dates = pd.date_range('20170101',periods=6)
df = pd.DataFrame(np.arange(24).reshape((6,4)),index=dates,columns=['A','B','C','D'])
print(df)
print(df['A'],df.A)
print(df[0:3],df['20170101':'20170104'])
# select by label:loc
print(df.loc['20170102'])
print(df.loc[:,['A','B']])
print(df.loc['20170102',['A','B']])
#select by position:iloc
print(df.iloc[3])
print(df.iloc[3,1])
print(df.iloc[1:3,1:3])
print(df.iloc[[1,3,5],1:3])
#mixed selection:ix
print(df.ix[:3,['A','C']])
# Boolean indexing
print(df)
print(df[df.A>8])
- 1
- 2
- 3
- 4
- 5
- 6
- 7
- 8
- 9
- 10
- 11
- 12
- 13
- 14
- 15
- 16
- 17
- 18
- 19
- 20
- 21
- 22
- 23
- 24
- 25
- 26
- 27
- 28
- 29
- 30
- 31
- 32
- 33
- 1
- 2
- 3
- 4
- 5
- 6
- 7
- 8
- 9
- 10
- 11
- 12
- 13
- 14
- 15
- 16
- 17
- 18
- 19
- 20
- 21
- 22
- 23
- 24
- 25
- 26
- 27
- 28
- 29
- 30
- 31
- 32
- 33
5. Handing Missing Values
This is an inevitale part of dealing wiht data. To overcom this hurdle, use dropna or fillna function
#a. dropna: It is used to drop rows or columns having missing data
df1.dropna()
#b.fillna: It is used to fill missing values
df2.fillna(value=5) # It replaces all missing values with 5
mean = df2['column1'].mean()
df2['column1'].fillna(mean) # It replaces all missing values of column1 with mean of available values
————-
from pandas import Series,DataFrame
import pandas as pd
ser = Series([4.5,7.2,-5.3,3.6],index=['d','b','a','c'])
ser
ser.drop('c')
ser
.drop() 返回的是一个新对象,元对象不会被改变。
from pandas import Series,DataFrame
import pandas as pd
import numpy as np
df = pd.DataFrame([[np.nan, 2, np.nan, 0], [3, 4, np.nan, 1],
... [np.nan, np.nan, np.nan, 5]],
... columns=list('ABCD'))
df
df.dropna(axis=1, how='all')
A B D
0 NaN 2.0 0
1 3.0 4.0 1
2 NaN NaN 5
>>> df.dropna(axis=1, how='any')
D
0 0
1 1
2 5
>>> df.dropna(axis=0, how='all')
A B C D
0 NaN 2.0 NaN 0
1 3.0 4.0 NaN 1
2 NaN NaN NaN 5
>>> df.dropna(axis=0, how='any')
Empty DataFrame
Columns: [A, B, C, D]
Index: []
>>> df.dropna(thresh=2)
A B C D
0 NaN 2.0 NaN 0
1 3.0 4.0 NaN 1
>>> df.dropna(how='all')
A B C D
0 NaN 2.0 NaN 0
1 3.0 4.0 NaN 1
2 NaN NaN NaN 5
>>> df.dropna( how='any')
Empty DataFrame
Columns: [A, B, C, D]
Index: []
dfnew = pd.DataFrame([[3435234, 2, 5666, 0], [3, 4, np.nan, 1],
...: ... [np.nan, np.nan, np.nan, 5]],
...: ... columns=list('ABCD'))
dfnew.dropna()
A B C D
0 3435234 2 5666 0
- 1
- 2
- 3
- 4
- 5
- 6
- 7
- 8
- 9
- 10
- 11
- 12
- 13
- 14
- 15
- 16
- 17
- 18
- 19
- 20
- 21
- 22
- 23
- 24
- 25
- 26
- 27
- 28
- 29
- 30
- 31
- 32
- 33
- 34
- 35
- 36
- 37
- 38
- 39
- 40
- 41
- 42
- 43
- 44
- 45
- 46
- 47
- 48
- 49
- 50
- 51
- 52
- 53
- 54
- 55
- 56
- 57
- 58
- 59
- 60
- 61
- 62
- 63
- 64
- 65
- 66
- 67
- 68
- 69
- 70
- 71
- 72
- 73
- 1
- 2
- 3
- 4
- 5
- 6
- 7
- 8
- 9
- 10
- 11
- 12
- 13
- 14
- 15
- 16
- 17
- 18
- 19
- 20
- 21
- 22
- 23
- 24
- 25
- 26
- 27
- 28
- 29
- 30
- 31
- 32
- 33
- 34
- 35
- 36
- 37
- 38
- 39
- 40
- 41
- 42
- 43
- 44
- 45
- 46
- 47
- 48
- 49
- 50
- 51
- 52
- 53
- 54
- 55
- 56
- 57
- 58
- 59
- 60
- 61
- 62
- 63
- 64
- 65
- 66
- 67
- 68
- 69
- 70
- 71
- 72
- 73
import numpy as np
import pandas as pd
dates = pd.date_range('20170101',periods=6)
df = pd.DataFrame(np.arange(24).reshape((6,4)),index=dates,columns=['A','B','C','D'])
print(df)
df.iloc[0,1]=np.nan
df.iloc[1,2]=np.nan
print(df.dropna(axis=0,how='any'))
print(df.dropna(axis=1,how='all'))
print(df.fillna(value=0))
print(df.isnull())
print(np.any(df.isnull())==True)
- 1
- 2
- 3
- 4
- 5
- 6
- 7
- 8
- 9
- 10
- 11
- 12
- 13
- 14
- 15
- 16
- 17
- 18
- 19
- 20
- 1
- 2
- 3
- 4
- 5
- 6
- 7
- 8
- 9
- 10
- 11
- 12
- 13
- 14
- 15
- 16
- 17
- 18
- 19
- 20
6. Creating New Columns
New column is a function of existing columns
df['NewColumn1'] = df['column2'] # Create a copy of existing column2
df['NewColumn2'] = df['column2'] + 10 # Add 10 to existing column2 then create a new one
df['NewColumn3'] = df['column1'] + df['column2'] # Add elements of column1 and column2 then create new column
import pandas as pd
import numpy as np
s = pd.Series([1,3,5,np.nan,55,2])
print(s)
dates = pd.date_range('20160101',periods=6)
print(dates)
df = pd.DataFrame(np.random.random(6,4),index=dates,columns=['a','b','c','d'])
print(df)
df1 = pd.DataFrame(np.arange(12).reshape((3,4)))
print(df1)
df2 = pd.DataFrame({'A':1.,
'B':pd.Timestamp('20170101'),
'C':pd.Series(1,index=list(range(4)),dtype='float32'),
'D':np.array([3]*4,dtype='int32'),
'E':pd.Categorical(["test","train,"test","train"]),
'F':'foo'})
print(df2.dtypes)
print(df2.columns)
print(df2.values)
print(df2.describe)
print(df2.T)
print(df2.sort_index(axis=1,ascending=False))
df2.sort_values(by='E')
- 1
- 2
- 3
- 4
- 5
- 6
- 7
- 8
- 9
- 10
- 11
- 12
- 13
- 14
- 15
- 16
- 17
- 18
- 19
- 20
- 21
- 22
- 23
- 24
- 25
- 26
- 27
- 28
- 29
- 30
- 31
- 32
- 33
- 34
- 1
- 2
- 3
- 4
- 5
- 6
- 7
- 8
- 9
- 10
- 11
- 12
- 13
- 14
- 15
- 16
- 17
- 18
- 19
- 20
- 21
- 22
- 23
- 24
- 25
- 26
- 27
- 28
- 29
- 30
- 31
- 32
- 33
- 34
df['F'] = np.nan
print(df)
df['E']=pd.Series([1,2,3,4,5,6],index=pd.date_range('20170101',periods=6))
print(df)
7. Aggregate
a. Groupby: Groupby helps to perform three operations.
i. Splitting the data into groups
ii. Applying a function to each group individually
iii. Combining the result into a data structure
df.groupby('column1').sum()
df.groupby(['column1','column2']).count()
b. Pivot Table: It helps to generate data structure. It has three components index, columns and values(similar to excel)
pd.pivot_table(df, values='column1',index=['column2','column3'],columns=['column4'])
By default, it shows the sum of values column but you can change it using argument aggfunc
pd.pivot_table(df, values='column1',index=['column2','column3'],columns=['column4'], aggfunc=len)
It shows count c. Cross Tab: Cross Tab computes the simple cross tabulation of two factors
pd.crosstab(df.column1, df.column2)
—————————————————
***8. Merging /Concatenating DataFrames***
a. Concatenating: It concatenate two or more data frames based on their columns
pd.concat([df1, df2])
b. Merging: We can perform left, right and inner join also.
pd.merge(df1,df2, on='column1',how='inner')
pd.merge(df1,df2, on='column1',how='left')
pd.merge(df1,df2, on='column1',how='right')
pd.merge(df1,df2, on='column1',how='outer')
import pandas as pd
import numpy as np
df1 = pd.DataFrame(np.ones((3,4))*0,columns=['a','b','c','d'])
df2 = pd.DataFrame(np.ones((3,4))*1,columns=['a','b','c','d'])
df3 = pd.DataFrame(np.ones((3,4))*2,columns=['a','b','c','d'])
print(df1)
print(df2)
print(df3)
result = pd.concat([df1,df2,df3],axis=0)
print(result)
result = pd.concat([df1,df2,df3],axis=0,ignore_index=True)
print(result)
df1 = pd.DataFrame(np.ones((3,4))*0,columns=['a','b','c','d'],index=[1,2,3])
df2 = pd.DataFrame(np.ones((3,4))*1,columns=['a','b','c','d'],index=[2,3,4])
print(df1)
print(df2)
result2 = pd.concat([df1,df2],join='outer',ignore_index=True)
print(result2)
result22 = pd.concat([df1,df2],join='outer')
print(result22)
result3 = pd.concat([df1,df2],join='inner',ignore_index=True)
print(result3)
result33 = pd.concat([df1,df2],join='inner')
print(result33)
df1 = pd.DataFrame(np.ones((3,4))*0,columns=['a','b','c','d'],index=[1,2,3])
df2 = pd.DataFrame(np.ones((3,4))*1,columns=['a','b','c','d'],index=[2,3,4])
res = pd.concat([df1,df2],axis=1,join_axes=[df1.index])
print(res)
res1 = pd.concat([df1,df2],axis=1)
print(res1)
df1 = pd.DataFrame(np.ones((3,4))*0,columns=['a','b','c','d'])
df2 = pd.DataFrame(np.ones((3,4))*1,columns=['a','b','c','d'])
df3 = pd.DataFrame(np.ones((3,4))*1,columns=['a','b','c','d'])
res11 = df1.append(df2,ignore_index=True)
print(res11)
res12 = df1.append([df2,df3],ignore_index=True)
print(res12)
s1 = pd.Series([1,2,3,4],index=['a','b','c','d'])
res13=df1.append(s1,ignore_index=True)
print(res13)
import pandas as pd
left = pd.DataFrame({'key':['K0','K1','K2','K3'],
'A':['A0','A1','A2','A3'],
'B':['B0','B1','B2','B3']})
right = pd.DataFrame({'key':['K0','K1','K2','K3'],
'C':['C0','C1','C2','C3'],
'D':['D0','D1','D2','D3']})
print(lef)
print(right)
res14 = pd.merge(left,right,on='key')
print(res14)
left = pd.DataFrame({'key1':['K0','K0','K1','K2'],
'key2':['K0','K1','K0','K1'],
'A':['A0','A1','A2','A3'],
'B':['B0','B1','B2','B3']})
right = pd.DataFrame({'key1':['K0','K1','K1','K2'],
'key2':['K0','K0','K0','K0'],
'C':['C0','C1','C2','C3'],
'D':['D0','D1','D2','D3']})
print(left)
print(right)
res15 = pd.merge(left,right,on=['key1','key2'])
print(res15)
res16 = pd.merge(left,right,on=['key1','key2'],how='inner')
print(res16)
- 1
- 2
- 3
- 4
- 5
- 6
- 7
- 8
- 9
- 10
- 11
- 12
- 13
- 14
- 15
- 16
- 17
- 18
- 19
- 20
- 21
- 22
- 23
- 24
- 25
- 26
- 27
- 28
- 29
- 30
- 31
- 32
- 33
- 34
- 35
- 36
- 37
- 38
- 39
- 40
- 41
- 42
- 43
- 44
- 45
- 46
- 47
- 48
- 49
- 50
- 51
- 52
- 53
- 54
- 55
- 56
- 57
- 58
- 59
- 60
- 61
- 62
- 63
- 64
- 65
- 66
- 67
- 68
- 69
- 70
- 71
- 72
- 73
- 74
- 75
- 76
- 77
- 78
- 79
- 80
- 81
- 82
- 83
- 84
- 85
- 86
- 87
- 88
- 89
- 90
- 91
- 92
- 93
- 94
- 95
- 96
- 97
- 98
- 99
- 100
- 101
- 102
- 103
- 1
- 2
- 3
- 4
- 5
- 6
- 7
- 8
- 9
- 10
- 11
- 12
- 13
- 14
- 15
- 16
- 17
- 18
- 19
- 20
- 21
- 22
- 23
- 24
- 25
- 26
- 27
- 28
- 29
- 30
- 31
- 32
- 33
- 34
- 35
- 36
- 37
- 38
- 39
- 40
- 41
- 42
- 43
- 44
- 45
- 46
- 47
- 48
- 49
- 50
- 51
- 52
- 53
- 54
- 55
- 56
- 57
- 58
- 59
- 60
- 61
- 62
- 63
- 64
- 65
- 66
- 67
- 68
- 69
- 70
- 71
- 72
- 73
- 74
- 75
- 76
- 77
- 78
- 79
- 80
- 81
- 82
- 83
- 84
- 85
- 86
- 87
- 88
- 89
- 90
- 91
- 92
- 93
- 94
- 95
- 96
- 97
- 98
- 99
- 100
- 101
- 102
- 103
9. Applying function to element, column or dataframe
a. Map: It iterates over each element of a series
df['column1'].map(lambda x: 10+x) #this will add 10 to each element of column1
df['column2'].map(lambda x:'AV'+x) # this will concatenate 'AV' at the beginning of each element of column2(column format is string)
b. Apply: As the name suggests, applies a function along any axis of the DataFrame
df[['column1','column2']].apply(sum) #It will returns the sum of all the values of column1 and column2
c. ApplyMap: This helps to apply a function to each element of dataframe
func = lambda x: x+2
df.applymap(func) # it will add 2 to each element of dataframe(all columns of dataframe must be numeric type)
—————————————————
***10. Identify unique value***
Function unique helps to return unique values of a column
df['Column1'].unique()
—————————————————
***11. Basic Stats***
Pandas helps to understand the data using basic statistical methods. a. describe: This returns the quick stats(count, mean, std, min, first quartile, median, third quartile, max) on suitable columns
df.describe()
b. covariance: It returns the co-variance between suitable columns
df.cov()
c.correlation: It returns the co-variance between suitable columns.
df.corr()
——— 本文中的 Python-Pandas.ipynb格式见[CSDN下载](http://download.csdn.net/detail/jiandanjinxin/9826981)。
import pandas as pd
df = pd.read_csv('uk_rain_2014.csv', header=0)
df.head(5)
| Water Year | Rain (mm) Oct-Sep | Outflow (m3/s) Oct-Sep | Rain (mm) Dec-Feb | Outflow (m3/s) Dec-Feb | Rain (mm) Jun-Aug | Outflow (m3/s) Jun-Aug |
---|
0 | 1980/81 | 1182 | 5408 | 292 | 7248 | 174 | 2212 |
---|
1 | 1981/82 | 1098 | 5112 | 257 | 7316 | 242 | 1936 |
---|
2 | 1982/83 | 1156 | 5701 | 330 | 8567 | 124 | 1802 |
---|
3 | 1983/84 | 993 | 4265 | 391 | 8905 | 141 | 1078 |
---|
4 | 1984/85 | 1182 | 5364 | 217 | 5813 | 343 | 4313 |
---|
df.tail(5)
| Water Year | Rain (mm) Oct-Sep | Outflow (m3/s) Oct-Sep | Rain (mm) Dec-Feb | Outflow (m3/s) Dec-Feb | Rain (mm) Jun-Aug | Outflow (m3/s) Jun-Aug |
---|
28 | 2008/09 | 1139 | 4941 | 268 | 6690 | 323 | 3189 |
---|
29 | 2009/10 | 1103 | 4738 | 255 | 6435 | 244 | 1958 |
---|
30 | 2010/11 | 1053 | 4521 | 265 | 6593 | 267 | 2885 |
---|
31 | 2011/12 | 1285 | 5500 | 339 | 7630 | 379 | 5261 |
---|
32 | 2012/13 | 1090 | 5329 | 350 | 9615 | 187 | 1797 |
---|
df.columns
Index([’Water Year’, ‘Rain (mm) Oct-Sep’, ‘Outflow (m3/s) Oct-Sep’, ‘Rain (mm) Dec-Feb’, ‘Outflow (m3/s) Dec-Feb’, ‘Rain (mm) Jun-Aug’, ‘Outflow (m3/s) Jun-Aug’], dtype=’object’)
df.columns = ['water_year', 'rain_octsep', 'outflow_octsep', 'rain_decfeb', 'outflow_decfeb', 'rain_junaug', 'outflow_junaug']
df.head(5)
| water_year | rain_octsep | outflow_octsep | rain_decfeb | outflow_decfeb | rain_junaug | outflow_junaug |
---|
0 | 1980/81 | 1182 | 5408 | 292 | 7248 | 174 | 2212 |
---|
1 | 1981/82 | 1098 | 5112 | 257 | 7316 | 242 | 1936 |
---|
2 | 1982/83 | 1156 | 5701 | 330 | 8567 | 124 | 1802 |
---|
3 | 1983/84 | 993 | 4265 | 391 | 8905 | 141 | 1078 |
---|
4 | 1984/85 | 1182 | 5364 | 217 | 5813 | 343 | 4313 |
---|
len(df)
33
pd.options.display.float_format = '{:,.3f}'.format
df.describe()
| rain_octsep | outflow_octsep | rain_decfeb | outflow_decfeb | rain_junaug | outflow_junaug |
---|
count | 33.000 | 33.000 | 33.000 | 33.000 | 33.000 | 33.000 |
---|
mean | 1,129.000 | 5,019.182 | 325.364 | 7,926.545 | 237.485 | 2,439.758 |
---|
std | 101.900 | 658.588 | 69.995 | 1,692.800 | 66.168 | 1,025.914 |
---|
min | 856.000 | 3,479.000 | 206.000 | 4,578.000 | 103.000 | 1,078.000 |
---|
25% | 1,053.000 | 4,506.000 | 268.000 | 6,690.000 | 193.000 | 1,797.000 |
---|
50% | 1,139.000 | 5,112.000 | 309.000 | 7,630.000 | 229.000 | 2,142.000 |
---|
75% | 1,182.000 | 5,497.000 | 360.000 | 8,905.000 | 280.000 | 2,959.000 |
---|
max | 1,387.000 | 6,391.000 | 484.000 | 11,486.000 | 379.000 | 5,261.000 |
---|
df['rain_octsep']
0 1182 1 1098 2 1156 3 993 4 1182 5 1027 6 1151 7 1210 8 976 9 1130 10 1022 11 1151 12 1130 13 1162 14 1110 15 856 16 1047 17 1169 18 1268 19 1204 20 1239 21 1185 22 1021 23 1165 24 1095 25 1046 26 1387 27 1225 28 1139 29 1103 30 1053 31 1285 32 1090 Name: rain_octsep, dtype: int64
df.rain_octsep
0 1182 1 1098 2 1156 3 993 4 1182 5 1027 6 1151 7 1210 8 976 9 1130 10 1022 11 1151 12 1130 13 1162 14 1110 15 856 16 1047 17 1169 18 1268 19 1204 20 1239 21 1185 22 1021 23 1165 24 1095 25 1046 26 1387 27 1225 28 1139 29 1103 30 1053 31 1285 32 1090 Name: rain_octsep, dtype: int64
df.rain_octsep <1000
df['rain_octsep'] <1000
0 False 1 False 2 False 3 True 4 False 5 False 6 False 7 False 8 True 9 False 10 False 11 False 12 False 13 False 14 False 15 True 16 False 17 False 18 False 19 False 20 False 21 False 22 False 23 False 24 False 25 False 26 False 27 False 28 False 29 False 30 False 31 False 32 False Name: rain_octsep, dtype: bool
df[(df.rain_octsep <1000) & (df.outflow_octsep <4000)]
| water_year | rain_octsep | outflow_octsep | rain_decfeb | outflow_decfeb | rain_junaug | outflow_junaug |
---|
15 | 1995/96 | 856 | 3479 | 245 | 5515 | 172 | 1439 |
---|
df[df.water_year.str.startswith('199')]
| water_year | rain_octsep | outflow_octsep | rain_decfeb | outflow_decfeb | rain_junaug | outflow_junaug |
---|
10 | 1990/91 | 1022 | 4418 | 305 | 7120 | 216 | 1923 |
---|
11 | 1991/92 | 1151 | 4506 | 246 | 5493 | 280 | 2118 |
---|
12 | 1992/93 | 1130 | 5246 | 308 | 8751 | 219 | 2551 |
---|
13 | 1993/94 | 1162 | 5583 | 422 | 10109 | 193 | 1638 |
---|
14 | 1994/95 | 1110 | 5370 | 484 | 11486 | 103 | 1231 |
---|
15 | 1995/96 | 856 | 3479 | 245 | 5515 | 172 | 1439 |
---|
16 | 1996/97 | 1047 | 4019 | 258 | 5770 | 256 | 2102 |
---|
17 | 1997/98 | 1169 | 4953 | 341 | 7747 | 285 | 3206 |
---|
18 | 1998/99 | 1268 | 5824 | 360 | 8771 | 225 | 2240 |
---|
19 | 1999/00 | 1204 | 5665 | 417 | 10021 | 197 | 2166 |
---|
df.iloc[30]
water_year 2010/11 rain_octsep 1053 outflow_octsep 4521 rain_decfeb 265 outflow_decfeb 6593 rain_junaug 267 outflow_junaug 2885 Name: 30, dtype: object
df = df.set_index(['water_year'])
df.head(5)
| rain_octsep | outflow_octsep | rain_decfeb | outflow_decfeb | rain_junaug | outflow_junaug |
---|
water_year | | | | | | |
---|
1980/81 | 1182 | 5408 | 292 | 7248 | 174 | 2212 |
---|
1981/82 | 1098 | 5112 | 257 | 7316 | 242 | 1936 |
---|
1982/83 | 1156 | 5701 | 330 | 8567 | 124 | 1802 |
---|
1983/84 | 993 | 4265 | 391 | 8905 | 141 | 1078 |
---|
1984/85 | 1182 | 5364 | 217 | 5813 | 343 | 4313 |
---|
df.loc['2000/01']
rain_octsep 1239 outflow_octsep 6092 rain_decfeb 328 outflow_decfeb 9347 rain_junaug 236 outflow_junaug 2142 Name: 2000/01, dtype: int64
df.ix['1999/00']
rain_octsep 1204 outflow_octsep 5665 rain_decfeb 417 outflow_decfeb 10021 rain_junaug 197 outflow_junaug 2166 Name: 1999/00, dtype: int64
df.sort_index(ascending=False).head(5)
| rain_octsep | outflow_octsep | rain_decfeb | outflow_decfeb | rain_junaug | outflow_junaug |
---|
water_year | | | | | | |
---|
2012/13 | 1090 | 5329 | 350 | 9615 | 187 | 1797 |
---|
2011/12 | 1285 | 5500 | 339 | 7630 | 379 | 5261 |
---|
2010/11 | 1053 | 4521 | 265 | 6593 | 267 | 2885 |
---|
2009/10 | 1103 | 4738 | 255 | 6435 | 244 | 1958 |
---|
2008/09 | 1139 | 4941 | 268 | 6690 | 323 | 3189 |
---|
df = df.reset_index('water_year')
df.head(5)
| water_year | rain_octsep | outflow_octsep | rain_decfeb | outflow_decfeb | rain_junaug | outflow_junaug |
---|
0 | 1980/81 | 1182 | 5408 | 292 | 7248 | 174 | 2212 |
---|
1 | 1981/82 | 1098 | 5112 | 257 | 7316 | 242 | 1936 |
---|
2 | 1982/83 | 1156 | 5701 | 330 | 8567 | 124 | 1802 |
---|
3 | 1983/84 | 993 | 4265 | 391 | 8905 | 141 | 1078 |
---|
4 | 1984/85 | 1182 | 5364 | 217 | 5813 | 343 | 4313 |
---|
def base_year(year):
base_year = year[:4]
base_year = pd.to_datetime(base_year).year
return base_year
df['year'] = df.water_year.apply(base_year)
df.head(5)
| water_year | rain_octsep | outflow_octsep | rain_decfeb | outflow_decfeb | rain_junaug | outflow_junaug | year |
---|
0 | 1980/81 | 1182 | 5408 | 292 | 7248 | 174 | 2212 | 1980 |
---|
1 | 1981/82 | 1098 | 5112 | 257 | 7316 | 242 | 1936 | 1981 |
---|
2 | 1982/83 | 1156 | 5701 | 330 | 8567 | 124 | 1802 | 1982 |
---|
3 | 1983/84 | 993 | 4265 | 391 | 8905 | 141 | 1078 | 1983 |
---|
4 | 1984/85 | 1182 | 5364 | 217 | 5813 | 343 | 4313 | 1984 |
---|
df.groupby(df.year // 10*10).max()
| water_year | rain_octsep | outflow_octsep | rain_decfeb | outflow_decfeb | rain_junaug | outflow_junaug | year |
---|
year | | | | | | | | |
---|
1980 | 1989/90 | 1210 | 5701 | 470 | 10520 | 343 | 4313 | 1989 |
---|
1990 | 1999/00 | 1268 | 5824 | 484 | 11486 | 285 | 3206 | 1999 |
---|
2000 | 2009/10 | 1387 | 6391 | 437 | 10926 | 357 | 5168 | 2009 |
---|
2010 | 2012/13 | 1285 | 5500 | 350 | 9615 | 379 | 5261 | 2012 |
---|
decade_rain = df.groupby([df.year // 10*10,
df.rain_octsep // 1000*1000])[['outflow_octsep',
'outflow_decfeb','outflow_junaug']].mean()
decade_rain
| | outflow_octsep | outflow_decfeb | outflow_junaug |
---|
year | rain_octsep | | | |
---|
1980 | 0 | 4,297.500 | 7,685.000 | 1,259.000 |
---|
1000 | 5,289.625 | 7,933.000 | 2,572.250 |
---|
1990 | 0 | 3,479.000 | 5,515.000 | 1,439.000 |
---|
1000 | 5,064.889 | 8,363.111 | 2,130.556 |
---|
2000 | 1000 | 5,030.800 | 7,812.100 | 2,685.900 |
---|
2010 | 1000 | 5,116.667 | 7,946.000 | 3,314.333 |
---|
decade_rain.unstack(0)
| outflow_octsep | outflow_decfeb | outflow_junaug |
---|
year | 1980 | 1990 | 2000 | 2010 | 1980 | 1990 | 2000 | 2010 | 1980 | 1990 | 2000 | 2010 |
---|
rain_octsep | | | | | | | | | | | | |
---|
0 | 4,297.500 | 3,479.000 | nan | nan | 7,685.000 | 5,515.000 | nan | nan | 1,259.000 | 1,439.000 | nan | nan |
---|
1000 | 5,289.625 | 5,064.889 | 5,030.800 | 5,116.667 | 7,933.000 | 8,363.111 | 7,812.100 | 7,946.000 | 2,572.250 | 2,130.556 | 2,685.900 | 3,314.333 |
---|
decade_rain.unstack(1)
| outflow_octsep | outflow_decfeb | outflow_junaug |
---|
rain_octsep | 0 | 1000 | 0 | 1000 | 0 | 1000 |
---|
year | | | | | | |
---|
1980 | 4,297.500 | 5,289.625 | 7,685.000 | 7,933.000 | 1,259.000 | 2,572.250 |
---|
1990 | 3,479.000 | 5,064.889 | 5,515.000 | 8,363.111 | 1,439.000 | 2,130.556 |
---|
2000 | nan | 5,030.800 | nan | 7,812.100 | nan | 2,685.900 |
---|
2010 | nan | 5,116.667 | nan | 7,946.000 | nan | 3,314.333 |
---|
high_rain = df[df.rain_octsep > 1250]
high_rain
| water_year | rain_octsep | outflow_octsep | rain_decfeb | outflow_decfeb | rain_junaug | outflow_junaug | year |
---|
18 | 1998/99 | 1268 | 5824 | 360 | 8771 | 225 | 2240 | 1998 |
---|
26 | 2006/07 | 1387 | 6391 | 437 | 10926 | 357 | 5168 | 2006 |
---|
31 | 2011/12 | 1285 | 5500 | 339 | 7630 | 379 | 5261 | 2011 |
---|
high_rain.pivot('year', 'rain_octsep')[['outflow_octsep',
'outflow_decfeb','outflow_junaug']].fillna('')
| outflow_octsep | outflow_decfeb | outflow_junaug |
---|
rain_octsep | 1268 | 1285 | 1387 | 1268 | 1285 | 1387 | 1268 | 1285 | 1387 |
---|
year | | | | | | | | | |
---|
1998 | 5,824.000 | | | 8,771.000 | | | 2,240.000 | | |
---|
2006 | | | 6,391.000 | | | 10,926.000 | | | 5,168.000 |
---|
2011 | | 5,500.000 | | | 7,630.000 | | | 5,261.000 | |
---|
rain_jpn = pd.read_csv('jpn_rain.csv')
rain_jpn.column = ['year', 'jpn_rainfall']
uk_jpn_rain = df.merge(rain_jpn, on = 'year')
uk_jpn_rain.head(5)
%matplotlib inline
high_rain.plot(x='year', y='rain_octsep')
<matplotlib.axes._subplots.AxesSubplot at 0x7f1214a5d748>
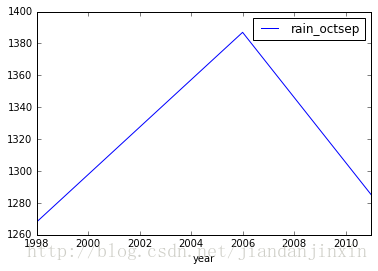
df.to_csv('high_rain.csv')
# pandas plot
import pandas as pd
import numpy as np
import matplotlib.pyplot as plt
#plot data
# Series
data = pd.Series(np.random.randn(1000),index=np.arange(1000))
data = data.cumsum()
data.plot()
plt.show()
plt.plot(x= , y = )
#DataFrame
data = pd.DataFrame(np.random.randn(1000,4),
index=np.arange(1000),
columns=list("ABCD"))
data =data.cumsum()
print(data.head())
data.plot()
plt.show()
#plot methods:
#'bar','hist','box','area','scatter','hexbin','pie'
data.plot.scatter(x='A',y='B',color='DarkBlue',label='Class1')
data.plot.scatter(x='A',y='C',color='DarkGreen',lable='Class2',ax=ax)
plt.show()
- 1
- 2
- 3
- 4
- 5
- 6
- 7
- 8
- 9
- 10
- 11
- 12
- 13
- 14
- 15
- 16
- 17
- 18
- 19
- 20
- 21
- 22
- 23
- 24
- 25
- 26
- 27
- 28
- 29
- 1
- 2
- 3
- 4
- 5
- 6
- 7
- 8
- 9
- 10
- 11
- 12
- 13
- 14
- 15
- 16
- 17
- 18
- 19
- 20
- 21
- 22
- 23
- 24
- 25
- 26
- 27
- 28
- 29
References
Python科学计算之Pandas(上)
Python科学计算之Pandas(下)
An Introduction to Scientific Python – Pandas
CheatSheet: Data Exploration using Pandas in Python
机器学习入门必备的13张小抄
numpy教程 pandas教程 Python数据科学计算简介