1、Deeplearning4j
深度学习,人工智能今天已经成了IT界最流行的词,而tensorflow,phython又是研究深度学习神经网络的热门工具。tensorflow是google的出品,而phython又以简练的语法,独特的代码结构和语言特性为众多数据科学家和AI工程师们所喜爱。但今天介绍的不是这两个炙手可热的东东,而是相对冷门,但对于国内大多数的工程师而言更友好,基于java的另一个深度学习框架deeplearning4j。截止本文编写时间为止,dl4j已经发布了1.0版本的beta版。
本文这里不会花太多笔墨去介绍神经网络的原理,有关这方面的知识,网络上面很多,我就不在此献丑。对于我等工程师而言,最关心的不外乎是神经网络是什么,神经网络有什么用,如何构建神经网络这三个问题。
啥是神经网络
神经网络是一个模拟生物(更确切的说,就是人)大脑结构的数学模型。人的大脑由1000亿个神经元构成,神经元通过各自间的连接,形成一个网络,就是所谓的神经网络。人的大脑的功能,不言自喻。人工神经网络就是通过数学建模的办法模拟大脑神经网络结构,实现类似人脑的功能。而人脑,较之于电脑,最显著的特征就是有自主意识,能识别一些相同或相似的物体,并能对这些物体进行有效分类。例如我们看到一幅徐悲鸿的画,就马上能知道画中所画的是一种叫马的生物。同样,我们希望电脑也能达到这样的效果。但可惜的是,计算机无法形成意识,只能处理与数字相关的工作。因此,要想计算机也能正确识别出马,就必须通过数学建模和一套相应的算法,使计算机“知道”这是马。这里知道二字我打了个双引号,为什么?因为计算机的理解最后还是一堆数字,真正的解读还是人。后面我会透过一个具体的例子,来说明为什么我要这么说。
3、神经网络的作用
正如第二节所说,人工神经网络是模拟人的大脑结构。因此神经网络的处理能力及程度取决于人对自身大脑结构的理解。就目前而言,神经网络最常被应用在分类和模式识别上面。最著名的例子就是百度首席科学家吴达恩(Andrew Wu)用youtube上的视频训练神经网络,使神经网络准确识别出猫。这有什么用?汽车自动驾驶系统就必须用到这方面的能力。试想下,如果汽车自动驾驶系统连基本的红绿灯,行人,车辆都识别不出来,谁敢让计算机帮忙开车。
但对于我等程序员吊丝,又不是搞自动驾驶,计算机这种连三岁小儿都不如的识别能力,有什么用?有用!神经网络有模式识别的能力,举个例子,某个购物网站通过数据统计,统计出每个注册用户的每天的订单数据情况,这些订单数据可能千差万别。数据之间也没有必然联系,如果要统计这些用户的类别,分析出他们的一些属性,该如何操作。人手操作肯定不行,像某宝网每天PB级的数据,那要请多少人来干这活。那计算机能干这活吗?能,关键是要把这些数据转换成计算机,更准确的说,神经网络,能识别的数据模型,然后,根据人们的一些直观认识,例如买化妆品的大多是小女生,买保健品的多是老大妈老大爷,诸如此类的所谓先验知识,来设定神经网络的分类目标。
虽说通过不断学习,网络总能与人们的直观认识达成一致(至少是大部分一致),但这仅对于训练样本而言。我们训练网络的目的,是为了最终的预测(也称为泛化)。如果网络对于除训练样本之外的数据,识别出来的分类未能与人们直观的认识相一致,就会出现所谓的过拟合的问题。
事实上,究竟人们直观认识上的东西是不是真能找到这样一个数学公式来近似模拟,不好说。因此,神经网络擅长于一些模识较为固定的领域,例如图像识别。因为大部分的图形,都可以找到其相应的解析函数。但如果遇到一些比较高级的概念时,例如某某是不是个骗子,某某是不是好人等等,神经网络可能就会懵B。因此神经网络不是万能的。
2 神经网络的一些基本概念
神经网络有多种,不同的神经网络有不同的用途。如正向前馈网络(BP网络),递归网络(RNN),卷积网(CNN)。但不管什么样的神经网络都离不开以下这几个概念。这里只粗浅的介绍其中一些概念(不一定全)及在dl4j中相对应的部分,其中一些复杂的数学推导请参看相关的参考文章,这里不作展开。
神经元
这是神经网络最基本的元素,神经元接收输入,并产生输出。
最经典的结构如下图所示:
神经元输入输出的数学模型大致可以简化成如下数学公式
其中xi是输入,wi是权值(),b是偏置,f(·)是激活函数(activation function,常用的有sigmod,RELU,SOFTMAX)。这个公式就是神经元实际执行的计算操作。在dl4j里面没有单独为神经元建模,因为框架实际上已经把网络模型转换为矩阵运算。
网络模型
可以看得出来,神经元的输出计算是一个简单的线性函数,所以一层的神经网络(称为感知器perceptor)只能处理线性可分的分类问题。为了打破感知器的局限,才有了BP网络等多层网络模型。理论证明,经过神经元之间的相互连接,形成一个更复杂的确网络,能解决更多的分类问题。
神经元的输入可能是原始数据样品,也可能是上一层数据的输入,这取决于神经元所处的层,对于一些复杂的神经网络来说,神经元可能有多层分布,每一层的神经元接收上一层(如果存在)神经元的输出数据,产出下一层(如果存在)神经元的输入数据。输入层接收的是原始输入,输出层输出的是最终输出。
传统的三层BPN的是长下面这副模样的:
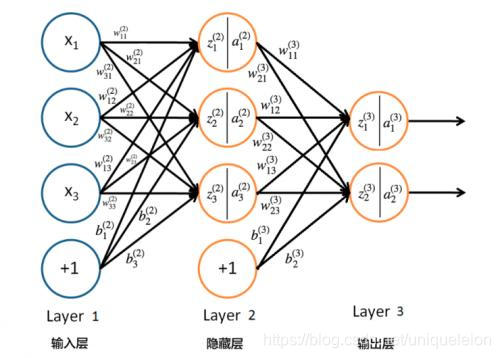
如果把整个神经网络看作一个黑盒子,那输入输出的关系更可以进一步简化成Y=F(X)。理论上,通过选择不同的权值和激活函数,函数F(X)的输出能逼近任意连续函数。神经网络能实现分类的原理即在此。
举例,2维平面直角坐标系(可推广到n维)上有一堆点(事先不知道其规律),如果能过这些点作一个曲线(推广到n维上就是超平面),那么这个曲线的解析函数即为神经网络所演化出来的F(X)。如果有n条曲线刚好穿过这些点,实际上就相当于把这些点分成了n类。如果网络自己学习到的这些曲线与人们直观认识上的分类相一致,我们就认为网络已经成功实现了分类。
下面再来聊聊何谓“深度学习”。如果说一个人的思想有深度,大概你可以例举他说过的一些话来证明。那计算机的自主学习怎样才算作有深度?最简单的判断标准,就是用于学习的网络有多少层,有多深。
在dl4j中,可以为每一层进行单独建模。建模的过程中,可以自由选择参数,参数包括输入输出的个数,偏置值,权值初始化方案,激活函数,损失计算函数等。
训练样本
训练样本就是你能给神经网络提供的数据,训练样本在dl4j里面分成两部分,分别为特征(features)和标签(labels),特征就是网络实际用于训练的数据,例如人的身高,体重等,标签顾名思义就是给样本打标签,在dl4j的一些demo里,就是一个某维为1,其余全为0的n维向量。如果按照身高,把人们分成三类,高个,中等身材,矮个。那么转换成dl4j的标签即为一个三维向量,如要把一个人标签为高个,那么他的标签向量即为(1.0,0,0)。
为了保证学习质量,训练样本一般成批地进行输入,所以这里又有一个最小批量(miniBatch)的概念。为什么要设定最小批量,因为神经网络是一个复杂的计算模型,要考虑到一个性能与精度的问题,样本量太大,会导致性能下降,样本量太小,样本覆盖范围不够广,精度又会降低,因此设定一个最小批量值,有助于得到一个性能与精度之间的平衡。
而训练的过程,就是不断向神经网络输入相同的数据样本,不断调整网络参数,并最终得到输出误差达到指定范围内的网络模型。
误差与收敛
如前文所述,我们其实是想找到一个近似函数来模拟点集在n维空间的超平面轨迹F(X)。但是神经网络找到的F(X),未必就是我们想要的东西。例如我们要求张量(即n维向量)T应当是属于某一个分类,从数学的角度来说,就是对于分类函数F'(X),这个张量会有预期的输出。如果网络训练的结果与预期输出不一致,就会出现误差。
误差会通过所谓的损失函数(英文称loss function,或cost function,有好几种,常用的有log似然函数)来计算,再通过反向传播算法来修正网络参数(权重)来缩小误差。我们希望误差透过上述算法可以变得越来越小,用数学的语言来说,就是所谓的收敛。但收敛并不是越快越好,因为收敛得快,不一定代表后面预测得准,因此会有所谓的学习率,学习率就是用来控制收敛速度。
上述概念,如偏置,激活函数,网络层数,神经元个数,损失函数,学习率等,在dl4j中都被称为神经网络的超参数(hyper parameter)。通过调节这些参数,能得到不同功能,不同分类特征的神经网络,因此称为超参数。
3 实战
介绍完一堆看似复杂的基本概念后,接下来不废话,马上进入实战。实战的内容并不是随便地拿别人的示例跑跑就完事。我的目标是自己出题,自己解决。因为别人的example都是预先设计好的,而且每个模型都只能针对特定的问题,不能照搬。只有自已找问题自己解决,这样才能验证自己的理解是不是正确,和发现这里面可能存在着的坑。
先出题:现在把人们按经济情况和家庭状况分成五类,一类是穷吊丝(特征:没积蓄,收入低,无车无房无家室),第二类是中层白领(稍有积蓄,收入较高,可能有车有房有家室),第三类是高管(有一定存款,收入高,有车有楼有家室),第四类是有钱人(有大量存款,收入极高,有车有楼有家室),第五类是钻石王老五(还没结婚的有钱人)
解题思路:
- 按照题目把样本数据抽取五个特征值组成一个五维的特征向量(存款,收入,是否有车,是否有房,是否有家室)
- 生成随机样本数据和对应的标签
- 构建神经网络,设置神经网络的超参数
- 把样本数据和标签打包,预处理(正则化),传给神经网络进行训练
- 生成一批新的样本数据来评价学习结果,如果结果较差,返回第2步,如果结果理想,则输出评价结果
下面是dl4j打印出来的输出结果:
========================Evaluation Metrics========================
# of classes: 5
Accuracy: 0.9420
Precision: 0.9519
Recall: 0.9411
F1 Score: 0.9381
Precision, recall & F1: macro-averaged (equally weighted avg. of 5 classes)
=========================Confusion Matrix=========================
0 1 2 3 4
---------------------
183 0 0 0 0 | 0 = 0
58 139 0 0 0 | 1 = 1
0 0 221 0 0 | 2 = 2
0 0 0 193 0 | 3 = 3
0 0 0 0 206 | 4 = 4
Confusion matrix format: Actual (rowClass) predicted as (columnClass) N times
==================================================================
这里我简单解释一下结果:
Accuracy 精确率,正确识别数/总样本数
Precision Recall & F1 : 各分类准确率的宏观平均值 ,(一直没弄懂Precision 和F1是怎么来的,只知道Recall是各个分类的准确率的算术平均值,例子中就是100% + 70% + 100 % + 100 % + 100% / 500 % = 94%) 。
对于我们来说关心一个总体准确率就够了,下面的混淆矩阵才是重点,很多时候超参调优就是看这鬼东西。
混淆矩阵的格式为实际的分类(以行作为标记)被预测为某个分类(以列作为标次)的次数,例如矩阵第1行1列的数字为183,就是说实际上的1(第1行)分类被预测为1(第1列)分类的次数为183次,也就是1分类的样品被正确识别了183次。
从结果矩阵的格式可以看出,对角线上的数据就是正常识别的次数,其余的皆为误识别。最理想的矩阵就是对角阵。
以上就是我历经多次手动调整网络超差数所得到的结果,识别率达9成以上,虽然结果并不完美,还有部分白领被错误识别为屌丝,原因在于我将白领的有车有房的特征设置成随机的。随机数据本来就没啥规律,机器识别不出来我觉得也不奇怪。
下面介绍一下我用到的超参数:BP网络;层数(3层,输入-隐层-输出);隐层神经元1295个(也就是能产生1295个输出);输入层、隐层的激活函数RELU,输出层的激活函数SOFTMAX;损失函数为负log似然函数;学习率0.5;各层偏置值(0.4,0.08,1);
整个输出结果是我用了1000个样本数据(样本数据加入了一定的噪音)训练了150次得到的。
看到这个结果,我也是挺激动,毕竟是苦战了三天三夜所训练出来的第一个效果不错的神经网络。
为什么看似这么low 逼的题目居然要花这么久的时间。下面就开始要扒一扒用dl4j训练神经网络时踩过的一些大坑了。
天坑一:超参数的选择
这个可以说是天坑中的天坑。至本文编写之日止,学术界对于如何选择超参数,一直是个难题。反卷积网就是在这个背景下产生的。对于大部分的拿来主义者来说,最简单快捷的学习方法就是直接用别人的代码复制粘贴,然后应用到自己的业务场景上面去,但我可以很负责任地告诉你,对于训练神经网络来说,你还真不能拿别人现成的东西来用。
正如我在本节开头所说的,神经网络模型并不具有普遍性。你要处理什么样的数据,要识别出什么东西,就需要一个特定的模型。更坑的是,影响神经网络精确率的因素,除了隐层数,隐层神经元个数外,还有学习率,偏置值,冲量值等一大堆。更让人吐血的是,这些数据的选择没有任何理论上的支持。正如一些文章里面说的,训练神经网络变成了一个抽奖过程。
天坑二:数据的标准化(Normaliztion)
神经网络更适合处理标准化的数据,何谓标准化数据,就是经过处理后,特征值在-1到1之间。数据标准化过程在dl4j提供的官方example里面一点都看不到,如果不去看官网,不仔细比对官方提供的训练集数据,光学着它的示例来写code,正如官方文档所说,性能会比较差。
4、代码
下面贴出实现上述例子所用到的代码。
首先是主测试类:
package org.aztec.dl_common;
import java.io.File;
import java.io.IOException;
import java.io.RandomAccessFile;
import java.nio.ByteBuffer;
import java.nio.channels.FileChannel;
import java.util.List;
import java.util.Random;
import org.apache.commons.lang.ArrayUtils;
import org.apache.commons.lang3.RandomUtils;
import org.datavec.api.records.reader.RecordReader;
import org.datavec.api.records.reader.impl.csv.CSVRecordReader;
import org.datavec.api.split.FileSplit;
import org.deeplearning4j.datasets.datavec.RecordReaderDataSetIterator;
import org.nd4j.linalg.dataset.api.iterator.DataSetIterator;
import com.google.common.collect.Lists;
import junit.framework.TestCase;
/**
* BP网络测试类
*/
public class BP_NetworkTest
extends TestCase{
private static final Random random = new Random();
private static final int inputNum = 5;
private static final int labelNum = 5;
public static void main(String[] args) {
//testTrain();
//testMultiSample();
//generateData();
train(new File("test/csv/test_classfy_1.csv"),new File("test/csv/test_classfy_2.csv"));
//testRead(new File("E:\\lm/git/dl4j/examples/dl4j-examples/dl4j-examples/src/main/resources/classification/linear_data_train.csv"));
}
/**
* 生成数据
*/
private static void generateData() {
try {
//testMain(args);
File oldFile = new File("test/csv/test_classfy_1.csv");
oldFile.delete();
File newFile = new File("test/csv/test_classfy_2.csv");
newFile.delete();
generateCSVData(1000, oldFile);
generateCSVData(1000, newFile);
} catch (Exception e) {
// TODO Auto-generated catch block
e.printStackTrace();
}
}
/**
* 读取csv文件数据,并训练网络
* @param trainFile
* @param testFile
*/
public static void train(File trainFile,File testFile) {
try {
int batchSize = 50;
RecordReader rr = new CSVRecordReader();
rr.initialize(new FileSplit(trainFile));
DataSetIterator trainIter = new RecordReaderDataSetIterator(rr,batchSize,0,labelNum);
RecordReader rr2 = new CSVRecordReader();
rr2.initialize(new FileSplit(testFile));
DataSetIterator testIter = new RecordReaderDataSetIterator(rr2,batchSize,0,labelNum);
SimpleNetworkConfiguration snc = new SimpleNetworkConfiguration(inputNum, labelNum);
SimpleBPNN bpnn = new SimpleBPNN();
//1295 5x
snc.setHiddenLayerNeuronNum(1295);
snc.setLayNum(1);
//snc.setBias(0.201);
snc.setLearnRatio(0.5);
snc.setBiasArray(new double[] {0.04,0.080,1});
snc.setMomentum(0.001);
snc.setNumEpochs(150);
bpnn.buildNetwork(snc);
bpnn.train(trainIter, snc.getNumEpochs(),false);
bpnn.validate(testIter, snc.getOutputNum(),false);
} catch (IOException e) {
// TODO Auto-generated catch block
e.printStackTrace();
} catch (InterruptedException e) {
// TODO Auto-generated catch block
e.printStackTrace();
}
}
/**
* 生成csv文件数据
* @param sampleSize
* @param csvFile
* @throws IOException
*/
private static void generateCSVData(int sampleSize,File csvFile) throws IOException {
if(!csvFile.exists()) {
csvFile.createNewFile();
}
RandomAccessFile raf = new RandomAccessFile(csvFile, "rw");
FileChannel fc = raf.getChannel();
double[] peopleData = new double[] {};
for(int i = 0;i < sampleSize;i++) {
int testNum = RandomUtils.nextInt() % labelNum;
String writeLine = new String("" + testNum);
switch(testNum) {
case 0 :
peopleData = generatePoorData();
break;
case 1 :
peopleData = generateWriteCollarData();
break;
case 2 :
peopleData = generateLeaderData();
//labels[0][i] = 2;
break;
case 3 :
peopleData = generateRichData();
//labels[0][i] = 3;
break;
case 4 :
peopleData = generateGoldenBachelorData();
//labels[0][i] = 4;
break;
}
for(int j = 0;j < peopleData.length;j++) {
writeLine += "," + peopleData[j];
}
writeLine += "\n";
byte[] lineBytes = writeLine.getBytes();
ByteBuffer bb = ByteBuffer.allocate(lineBytes.length);
bb.put(lineBytes);
bb.flip();
fc.write(bb);
}
fc.close();
}
/**
* 生成样品数据
* @param sampleSize
* @return
*/
private static List<double[][]> generateSampleData(int sampleSize){
List<double[][]> sampleAllDatas = Lists.newArrayList();
double[][] features = new double[sampleSize][];
double[][] labels = new double[sampleSize][];
//double[][] labels = new double[1][sampleSize];
for(int i = 0;i < sampleSize;i++) {
int testNum = RandomUtils.nextInt() % 5;
switch(testNum) {
case 0 :
features[i] = generatePoorData();
labels[i] = new double[]{1,0,0,0,0};
//labels[0][i] = 0;
break;
case 1 :
features[i] = generateWriteCollarData();
labels[i] = new double[]{0,1,0,0,0};
//labels[0][i] = 1;
break;
case 2 :
features[i] = generateLeaderData();
labels[i] = new double[]{0,0,1,0,0};
//labels[0][i] = 2;
break;
case 3 :
features[i] = generateRichData();
labels[i] = new double[]{0,0,0,1,0};
//labels[0][i] = 3;
break;
case 4 :
features[i] = generateGoldenBachelorData();
labels[i] = new double[]{0,0,0,0,1};
//labels[0][i] = 4;
break;
}
}
sampleAllDatas.add(features);
sampleAllDatas.add(labels);
return sampleAllDatas;
}
/**
* 生成随机噪声
* @param base
* @return
*/
private static double getRandomNoise(double base) {
double randomNum = random.nextDouble();
return randomNum;
//return base * randomNum;
//return 0;
}
/**
* 生成吊丝数据
* @param base
* @return
*/
private static double[] generatePoorData() {
//为了简化数据模样,提高训练速度,钱被简化成了一个倍数的概念。当然使用真实的人民币单位也可以,只是性能会差一点而已经
double base = 1;
double deposit = base + getRandomNoise(10);
double income = base + getRandomNoise(10);
return new double[] {deposit,income,0d,0d,0d};
//return new double[] {deposit,income};
}
/**
* 生成白领数据
* @return
*/
private static double[] generateWriteCollarData() {
double base = 3;
double deposit = base + getRandomNoise(10);
double hasChild = 0;
double income = base + getRandomNoise(10);
double hasHouse = random.nextDouble() > 0.5 ? 1 : 0;
double hasCar = random.nextDouble() > 0.5 ? 1 : 0;
return new double[] {deposit,income,hasHouse,hasCar,hasChild};
}
/**
* 生成高管数据
* @return
*/
private static double[] generateLeaderData() {
double base = 9;
double deposit = base + getRandomNoise(10);
double income = base + getRandomNoise(10) ;
return new double[] {deposit,income,1,1,1};
}
/**
* 生成有钱人数据
* @return
*/
private static double[] generateRichData() {
double base = 100;
double deposit = base + getRandomNoise(10);
double income = base + getRandomNoise(10);
return new double[] {deposit,income,1,1,1};
}
/**
* 生成钻石王老五数据
* @return
*/
private static double[] generateGoldenBachelorData() {
double base = 100;
double deposit = base + getRandomNoise(10);
double income = base + getRandomNoise(10);
return new double[] {deposit,income,1,1,0d};
}
}
在测试类中,出于程序员的强迫症发作,我对dl4j进行一些简单的封装。
下面是这些封装类:
package org.aztec.dl_common;
import org.deeplearning4j.eval.Evaluation;
import org.deeplearning4j.nn.api.OptimizationAlgorithm;
import org.deeplearning4j.nn.conf.MultiLayerConfiguration;
import org.deeplearning4j.nn.conf.NeuralNetConfiguration;
import org.deeplearning4j.nn.conf.NeuralNetConfiguration.Builder;
import org.deeplearning4j.nn.conf.NeuralNetConfiguration.ListBuilder;
import org.deeplearning4j.nn.conf.layers.DenseLayer;
import org.deeplearning4j.nn.conf.layers.OutputLayer;
import org.deeplearning4j.nn.multilayer.MultiLayerNetwork;
import org.deeplearning4j.nn.weights.WeightInit;
import org.deeplearning4j.optimize.listeners.ScoreIterationListener;
import org.nd4j.linalg.activations.Activation;
import org.nd4j.linalg.api.ndarray.INDArray;
import org.nd4j.linalg.dataset.DataSet;
import org.nd4j.linalg.dataset.api.iterator.DataSetIterator;
import org.nd4j.linalg.factory.Nd4j;
import org.nd4j.linalg.learning.config.Nesterovs;
import org.nd4j.linalg.lossfunctions.LossFunctions.LossFunction;
public class SimpleBPNN {
protected Builder networkBuilder;
protected MultiLayerNetwork network;
public SimpleBPNN() {
// TODO Auto-generated constructor stub
}
public void reset() {
}
public void buildNetwork(SimpleNetworkConfiguration networkConfig) {
networkBuilder = new NeuralNetConfiguration.Builder();
int layNum = networkConfig.getLayNum();
ListBuilder listBuilder = networkBuilder.seed(networkConfig.getRngSeed()) //include a random seed for reproducibility
// use stochastic gradient descent as an optimization algorithm
.optimizationAlgo(OptimizationAlgorithm.STOCHASTIC_GRADIENT_DESCENT)
.biasInit(networkConfig.getBias())
.updater(new Nesterovs(networkConfig.getLearnRatio(), networkConfig.getMomentum()))
//.activation(Activation.ELU)
.l2(networkConfig.getL2())
.list();
int fistLayOutput = networkConfig.getLayNum() > 0 ? networkConfig.getHiddenLayerNeuronNum() :
networkConfig.getOutputNum() ;
double[] biasArray = networkConfig.getBiasArray();
if(biasArray == null) {
biasArray = new double[layNum + 2];
for(int i = 0;i < biasArray.length;i++) {
biasArray[i] = networkConfig.getBias();
}
}
int layCursor = 0;
listBuilder = listBuilder.layer(0,new DenseLayer.Builder() //create the first, input layer with xavier initialization
.nIn(networkConfig.getInputNum())
.nOut(fistLayOutput)
.biasInit(biasArray[layCursor])
.activation(Activation.RELU)
.weightInit(WeightInit.XAVIER)
.build());
int lastOut = fistLayOutput;
layCursor ++;
for(int i = 0;i < layNum;i++) {
System.out.println("adding hidden layer!!");
int nIn = networkConfig.getHiddenLayerNeuronNum();
int nOut = networkConfig.getHiddenLayerNeuronNum();
if(i == layNum - 1) {
nOut = networkConfig.getOutputNum();
}
lastOut = nOut;
listBuilder = listBuilder.layer(i + 1,new DenseLayer.Builder()
.nIn(nIn)
.nOut(nOut)
.activation(Activation.RELU)
.weightInit(WeightInit.XAVIER)
.biasInit(biasArray[layCursor])
.build());
layCursor ++;
}
listBuilder = listBuilder.layer(layNum + 1,new OutputLayer.Builder(LossFunction.NEGATIVELOGLIKELIHOOD)
.activation(Activation.SOFTMAX)
.nIn(lastOut).nOut(networkConfig.getOutputNum())
.biasInit(biasArray[layCursor])
.build());
MultiLayerConfiguration mlc = listBuilder.pretrain(false).backprop(true).build();
network = new MultiLayerNetwork(mlc);
network.init();
//print the score with every 1 iteration
ScoreIterationListener sil = new ScoreIterationListener();
network.setListeners(sil);
/*
*/
}
private DataSetIterator normalize(DataSetIterator trainningDatas) {
return NormalizeUtils.transform(trainningDatas);
}
private boolean hasNormalizeBefore(DataSetIterator trainningDatas) {
if(NormalizeAware.class.isAssignableFrom(trainningDatas.getClass())) {
return ((NormalizeAware) trainningDatas).isNormalized();
}
return false;
}
public void train(DataSetIterator trainningDatas,int numEpochs,boolean normalized) {
DataSetIterator normalizedDatas = trainningDatas;
if (!normalized) {
normalizedDatas = normalize(trainningDatas);
}
network.pretrain(normalizedDatas);
for (int i = 0; i < numEpochs; i++) {
network.fit(normalizedDatas);
// preprocess(trainningDatas);
//normalizedDatas.reset();
}
}
public double[] predict(double[] features) {
if(features != null && features.length > 0) {
INDArray outArray = network.output(Nd4j.create(features));
return outArray.toDoubleVector();
}
return null;
}
public Evaluation validate(DataSetIterator dataSet,int outputNum,boolean normalized) {
DataSetIterator normalizedDatas = dataSet;
if (!normalized) {
normalizedDatas = normalize(dataSet);
}
Evaluation eval = new Evaluation(outputNum); //create an evaluation object with 10 possible classes
if(normalizedDatas.resetSupported()) {
normalizedDatas.reset();
}
while(normalizedDatas.hasNext()){
DataSet next = normalizedDatas.next();
INDArray output = network.output(next.getFeatures()); //get the networks prediction
eval.eval(next.getLabels(), output); //check the prediction against the true class
}
System.out.println(eval.stats());
return eval;
}
}
package org.aztec.dl_common;
public class SimpleNetworkConfiguration {
protected int inputNum = 10;
protected int outputNum = 10; // number of output classes
protected int batchSize = 50; // batch size for each epoch
protected int rngSeed = 123; // random number seed for reproducibility
protected int numEpochs = 150; // number of epochs to perform
protected int layNum = 1;
protected double bias = 0.3;
protected double[] biasArray;
protected double learnRatio = 0.006;
protected double momentum = 0.01;
protected double l2 = 1e-4;
protected double l1 = 1e-1;
protected int hiddenLayerNeuronNum = 1000;
public SimpleNetworkConfiguration(int inputNum,int outputNum) {
this.inputNum = inputNum;
this.outputNum = outputNum;
}
public SimpleNetworkConfiguration(SimpleTensorIterator iterator) {
this.inputNum = iterator.inputColumns();
this.outputNum = iterator.totalOutcomes();
}
public int getOutputNum() {
return outputNum;
}
public void setOutputNum(int outputNum) {
this.outputNum = outputNum;
}
public int getBatchSize() {
return batchSize;
}
public void setBatchSize(int batchSize) {
this.batchSize = batchSize;
}
public int getRngSeed() {
return rngSeed;
}
public void setRngSeed(int rngSeed) {
this.rngSeed = rngSeed;
}
public int getNumEpochs() {
return numEpochs;
}
public void setNumEpochs(int numEpochs) {
this.numEpochs = numEpochs;
}
public int getLayNum() {
return layNum;
}
public void setLayNum(int layNum) {
this.layNum = layNum;
}
public int getHiddenLayerNeuronNum() {
return hiddenLayerNeuronNum;
}
public void setHiddenLayerNeuronNum(int hiddenLayerNeuronNum) {
this.hiddenLayerNeuronNum = hiddenLayerNeuronNum;
}
public int getInputNum() {
return inputNum;
}
public void setInputNum(int inputNum) {
this.inputNum = inputNum;
}
public double getLearnRatio() {
return learnRatio;
}
public void setLearnRatio(double learnRatio) {
this.learnRatio = learnRatio;
}
public double getBias() {
return bias;
}
public void setBias(double bias) {
this.bias = bias;
}
public double getMomentum() {
return momentum;
}
public void setMomentum(double momentum) {
this.momentum = momentum;
}
public double getL2() {
return l2;
}
public void setL2(double l2) {
this.l2 = l2;
}
public double getL1() {
return l1;
}
public void setL1(double l1) {
this.l1 = l1;
}
public double[] getBiasArray() {
return biasArray;
}
public void setBiasArray(double[] biasArray) {
this.biasArray = biasArray;
}
}
还有一些工具类:
package org.aztec.dl_common;
import org.apache.commons.math3.stat.descriptive.moment.Mean;
import org.apache.commons.math3.stat.descriptive.moment.StandardDeviation;
import org.nd4j.linalg.api.ndarray.INDArray;
import org.nd4j.linalg.dataset.DataSet;
import org.nd4j.linalg.dataset.api.iterator.DataSetIterator;
import org.nd4j.linalg.dataset.api.preprocessor.NormalizerStandardize;
import org.nd4j.linalg.factory.Nd4j;
public class NormalizeUtils {
private NormalizeUtils() {
}
public static INDArray getMean(INDArray features) {
int colNum = features.columns();
double[] means = new double[colNum];
for(int i = 0;i < colNum;i++) {
double[] colDatas = features.getColumn(i).toDoubleVector();
Mean mean = new Mean();
means[i] = mean.evaluate(colDatas);
}
return Nd4j.create(means);
}
public static INDArray getStandardDeviation(INDArray features) {
int colNum = features.columns();
double[] stds = new double[colNum];
for(int i = 0;i < colNum;i++) {
double[] colDatas = features.getColumn(i).toDoubleVector();
StandardDeviation std = new StandardDeviation();
stds[i] = std.evaluate(colDatas);
}
return Nd4j.create(stds);
}
public static DataSetIterator transform(DataSetIterator itr) {
NormalizerStandardize ns = new NormalizerStandardize();
//ns.fitLabel(true);
ns.fit(itr);
SimpleTensorIterator tensorIterator = new SimpleTensorIterator();
itr.reset();
while(itr.hasNext()) {
DataSet ds = itr.next();
ns.transform(ds);
tensorIterator.addDataSet(ds);
}
tensorIterator.reset();
return tensorIterator;
}
}
package org.aztec.dl_common;
import java.util.Iterator;
import java.util.List;
import org.nd4j.linalg.api.ndarray.INDArray;
import org.nd4j.linalg.dataset.DataSet;
import org.nd4j.linalg.dataset.api.DataSetPreProcessor;
import org.nd4j.linalg.dataset.api.iterator.DataSetIterator;
import org.nd4j.linalg.dataset.api.preprocessor.NormalizerStandardize;
import org.nd4j.linalg.factory.Nd4j;
import com.google.common.collect.Lists;
public class SimpleTensorIterator implements DataSetIterator,NormalizeAware {
protected List<DataSet> datas;
protected Iterator<DataSet> trainningDatas;
protected List<String> labels;
protected int inputNums;
protected DataSetPreProcessor preprocessor;
protected int batch;
protected boolean normalized = false;
public SimpleTensorIterator() {
datas = Lists.newArrayList();
labels = Lists.newArrayList();
preprocessor = new NormalizerStandardize();
}
public SimpleTensorIterator(NetworkInput input) {
init(Lists.newArrayList(input),1);
}
public SimpleTensorIterator(List<NetworkInput> inputs,int batch) {
init(inputs,batch);
}
private void init(List<NetworkInput> inputs,int batch) {
List<DataSet> dataList = Lists.newArrayList();
double[][] features = new double[inputs.size()][];
double[][] labels = new double[inputs.size()][];
for(int i = 0;i < inputs.size();i++) {
NetworkInput input = inputs.get(i);
inputNums = input.getFeatures().length;
if(this.labels == null && input.getLabelNames() != null) {
this.labels = input.getLabelNames();
}
features[i] = inputs.get(i).getFeatures();
labels[i] = inputs.get(i).getLables();
}
dataList.add(getDataSet(features, labels));
/*for(NetworkInput input : inputs) {
inputNums = input.getFeatures().length;
if(labels == null && input.getLabelNames() != null) {
labels = input.getLabelNames();
}
dataList.add(getDataSet(input.getFeatures(), input.getLables()));
}*/
trainningDatas = dataList.iterator();
this.batch = batch;
datas = Lists.newArrayList();
datas.addAll(dataList);
preprocessor = new NormalizerStandardize();
}
private DataSet getDataSet(double[] featureRawDatas,double[] lableRawDatas) {
INDArray features = Nd4j.create(featureRawDatas);
INDArray lables = null;
if(lableRawDatas != null) {
lables = Nd4j.create(lableRawDatas);
}
return new DataSet(features, lables);
}
private DataSet getDataSet(double[][] featureRawDatas,double[][] lableRawDatas) {
INDArray features = Nd4j.create(featureRawDatas);
INDArray lables = null;
if(lableRawDatas != null && lableRawDatas.length > 0) {
lables = Nd4j.create(lableRawDatas);
}
return new DataSet(features, lables);
}
public boolean hasNext() {
// TODO Auto-generated method stub
return trainningDatas.hasNext();
}
public DataSet next() {
// TODO Auto-generated method stub
return trainningDatas.next();
}
public DataSet next(int num) {
// TODO Auto-generated method stubf
for(int i = 0;i < num;i++) {
trainningDatas.next();
}
return trainningDatas.next();
}
public int inputColumns() {
// TODO Auto-generated method stub
return inputNums;
}
public int totalOutcomes() {
// TODO Auto-generated method stub
return labels.size();
}
public boolean resetSupported() {
// TODO Auto-generated method stub
return false;
}
public boolean asyncSupported() {
// TODO Auto-generated method stub
return false;
}
public void reset() {
// TODO Auto-generated method stub
trainningDatas = datas.iterator();
}
public int batch() {
// TODO Auto-generated method stub
return batch;
}
public void setPreProcessor(DataSetPreProcessor preProcessor) {
// TODO Auto-generated method stub
this.preprocessor = preProcessor;
}
public DataSetPreProcessor getPreProcessor() {
// TODO Auto-generated method stub
return preprocessor;
}
public List<String> getLabels() {
return labels;
}
public boolean isNormalized() {
return normalized;
}
public void setNormalized(boolean flag) {
// TODO Auto-generated method stub
normalized = flag;
}
public void addDataSet(DataSet set) {
datas.add(set);
}
}
5 小结
人工智能,深度学习虽然已经不是什么新鲜事了,但对于我等普通程序猿来说,其普及率并不算高,主要是由于深度学习涉及到很多艰涩的概念和数学原理,是一般程序员极少用到的。因此,本文仅算是一个科普文章,只从一些最基本的概念原理出发,并从这些基本原理开始,讨论神经网络的模型与调优,并应用到一些实际例子中,去体现神经网络的神奇。
另外,鉴于国内还是java程序员占多数,大部分公司的软件架构都是java生态体系为主,因此介绍java系的深度学习框架应该更为国内程序员所接收。