杰弗里·辛顿 反向传播
Winter is coming for artificial intelligence, and it may be years before AI research picks up again.
人工智能的冬天来临了,人工智能研究可能需要几年的时间才能再次兴起。
For anyone who’s not a data scientist, Baymax in Disney’s Big Hero 6 may seem like machine learning’s holy grail. But a truly human-like, intelligent algorithm is so far-fetched that most data scientists shrug off its possibility entirely.
F或任何人谁不是一个数据科学家,大白于迪斯尼的大英雄6可能看起来像是机器学习的圣杯。 但是,真正意义上的类似于人类的智能算法是如此牵强,以至于大多数数据科学家完全忽略了它的可能性。
Since Big Hero 6 was released in 2014, data science as a field hasn’t changed. Forget intelligent robots — our best learning models struggle to match the intelligence of a cockroach. Today’s artificial intelligence is relegated to identifying niche patterns for well-defined mathematical applications, like product pricing.
自《 Big Hero 6 》于2014年发布以来,数据科学作为一个领域并没有改变。 忘记智能机器人-我们最好的学习模型很难与蟑螂的智能相匹配。 如今的人工智能已被淘汰,只能为产品定价等定义明确的数学应用识别利基模式。
The hype surrounding AI is waning. Industry leaders are replacing “artificial intelligence” with “augmented intelligence” in the face of an anticipated imminent halt in academic interest in machine learning. The AI winter is coming.
围绕AI的炒作正在减弱。 面对预期对机器学习的兴趣即将停止的情况,行业领导者正在用“增强型智能”代替“人工智能”。 人工智能的冬天来了。
So how do we prepare for it? Can we even avoid it?
那么我们该如何准备呢? 我们甚至可以避免吗?
The AI field is plagued by irrational optimism and irrational despair.
AI领域受到非理性的乐观和非理性的绝望困扰。
In 1973, Sir James Lighthill was asked to compile a report on the then-present state of artificial intelligence. His report criticized the hype surrounding artificial intelligence research, suggesting that AI’s best algorithms would always fail at solving real world problems and could really only work for solving “baby” problems.
1973年,詹姆斯·莱特希尔爵士(Sir James Lighthill)被要求编写一份有关当时人工智能现状的报告。 他的报告批评了围绕人工智能研究的炒作,认为人工智能的最佳算法将始终无法解决现实世界中的问题,并且实际上只能解决“婴儿”问题。
His report followed almost twenty-five years of fervent research into human-like algorithms. The AI “summer” between the 1950s and 1970s saw DARPA investing millions into undirected research that touched on natural language processing. The air force dumped gargantuan resources onto scientists who were developing symbolic reasoning. English universities raced to create the first general purpose robot that could adapt to any situation, learn anything, and interact with people like a human would.
他的报告是在将近25年的时间里,对类人算法的热心研究。 1950年代至1970年代的AI“夏季”,DARPA投入了数百万美元用于涉及自然语言处理的无定向研究。 空军将庞大的资源倾泻到正在发展象征性推理的科学家身上。 英国大学竞相创建第一个通用机器人,该机器人可以适应任何情况,学习任何东西并像人一样与人互动。
The economic promise surrounding general purpose algorithms was free labor. This dream was a perfect “computer human” that took care of itself, didn’t need sustenance and worked in any situation. Once cracked, the productivity gains from a human-intelligence AI would instantly make the space race look like child’s play.
围绕通用算法的经济承诺是自由劳动。 这个梦想是一个完美的“计算机人”,可以照顾自己,不需要寄托并且可以在任何情况下工作。 一旦破解,从人类智能AI获得的生产力提升将立即使太空竞赛看起来像是儿童游戏。
Sir James’s “Lighthill Report” accused the general purpose robot of “being a mirage.” Lighthill debated premier AI researchers in the BBC broadcast “Controversy,” ultimately defeating pro-AI-research sentiment by making one simple observation: the complexity of the AI algorithms was far from the complexity of anything like a human brain. He argued that we’d need decades of transistor miniaturization, increased memory capacity, and hardware development before the question of AI could even be raised.
詹姆士爵士(Sir James)的“ Lighthill报告”指责通用机器人“海市rage楼”。 Lighthill在BBC广播的“争议”中辩论了首席AI研究人员,最终通过做一个简单的观察就击败了赞成AI研究的观点: AI算法的复杂性远没有像人脑那样复杂 。 他认为,在提出AI问题之前,我们需要数十年的晶体管小型化,增加的存储容量和硬件开发。
Thus began the first AI winter as universities and governments alike pulled their funding overnight. Public support for AI disappeared as well —after all, weren’t they a blatant waste of taxpayer dollars?
随着大学和政府一夜之间撤资,第一个AI冬季由此开始。 公众对人工智能的支持也消失了-毕竟,它们不是在浪费纳税人的钱吗?
This phenomenon of an idea’s rise and fall extends to any novelty, including bitcoin, fine art, unique college programs and space exploration (look up the Gartner Hype Cycle). But AI is unique in that its development spans several decades rather than years. Careers can begin and end within a single AI winter, never seeing the light of summer.
这个想法兴衰的现象扩展到任何新颖性,包括比特币,美术,独特的大学课程和太空探索(请查阅Gartner Hype Cycle)。 但是AI的独特之处在于其发展跨越了几十年而不是几年。 职业生涯可以在一个AI冬季内开始和结束,而从未见过夏日的阳光。
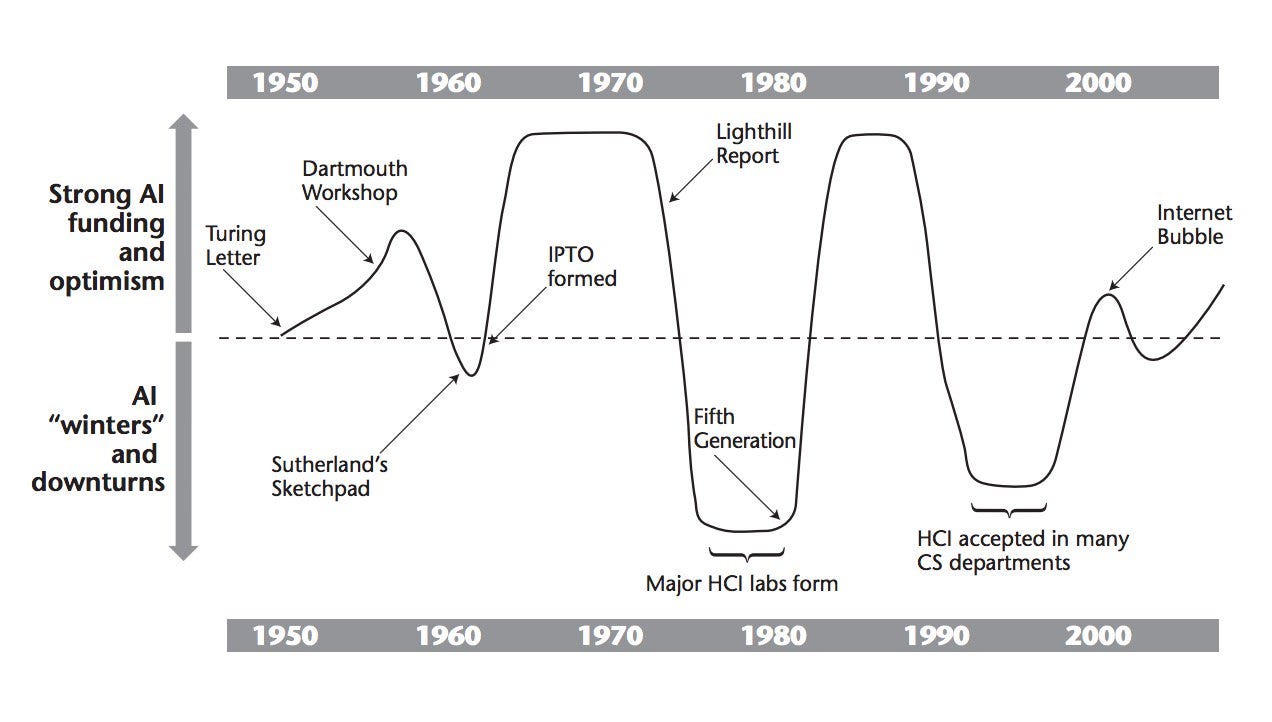
AI summers are always triggered by a fundamental algorithmic breakthrough like Warren McCulloch’s and Walter Pitts’s neural network in 1943. AI winters are always triggered by a fundamental hardware limitation: too few transistors in the 1970s and too little memory in the 1990s.
AI之夏总是由诸如Warren McCulloch和Walter Pitts的神经网络在1943年这样的基本算法突破触发的。AI Winters总是受到基本硬件限制的触发:1970年代晶体管太少,1990年代存储器太少。
Fast forward to today. By spec, our supercomputers are on par with human brains. The transistor counts are much greater, the memory amount is larger, and we even know how individual neurons interact with one another in limited capacity. But over the course of the last decade, our algorithms haven’t improved more than a couple percentage points in very niche applications.
快进到今天。 从规格上说,我们的超级计算机与人脑相当。 晶体管的数量要多得多,存储量也要大得多,我们甚至知道单个神经元如何以有限的容量彼此交互。 但是在过去的十年中,我们的算法在非常特殊的应用程序中的改进没有超过几个百分点。
Without a clear hardware limitation, how is machine learning failing to progress in general purpose intelligence? If the upcoming AI winter has little to do with a hardware limitation, something else must be triggering it.
如果没有明确的硬件限制,机器学习如何无法在通用智能中发展? 如果即将到来的AI冬季与硬件限制无关,则必须触发其他因素。
Fei-Fei Li, a computer science professor at Princeton, published ImageNet in 2009. By using Amazon’s Mechanical Turk, Dr. Li outsourced the task of labeling millions of pictures to online freelancers (often paid pennies to do so). The aim was to improve the volume of high-quality labelled data available to classification algorithms. The result was a database comprising 3.2 million labelled images, sorted into 12 “branches” and 5000 subcategories.
普林斯顿大学计算机科学教授李飞飞(Fei-Fei Li)于2009年出版了ImageNet。李博士通过使用亚马逊的Mechanical Turk,将标记数百万张图片的任务外包给了在线自由职业者(通常这样做是有偿的)。 目的是提高分类算法可使用的高质量标记数据的数量。 结果是一个数据库,其中包含320万个带标签的图像,分为12个“分支”和5000个子类别。
ImageNet kicked off an annual classification competition where entrants would submit their own algorithms, train them on ImageNet, and compete based on their accuracy. This competition is often credited for generating the hype around the current AI summer; winners were consistently gobbled up by Fortune 500 companies hoping to capitalize on AI. The last competition in 2017 featured a winning accuracy of 97.3%, meaning that the AI correctly identified and categorized images in the database 97.3% of the time.
ImageNet启动了年度分类竞赛,参赛者将提交自己的算法,在ImageNet上对其进行训练,然后根据其准确性进行竞争。 这场比赛通常是因为在当前的AI夏季引起了炒作。 希望利用人工智能的《财富》 500强公司一直在吞噬赢家。 2017年的最后一场比赛的获胜准确度为97.3%,这意味着AI可以97.3%的时间正确识别并分类数据库中的图像。
But it wasn’t just ImageNet that triggered the current AI summer we’re in — it was an idea that came from that original 2009 paper:
但是,不仅仅是ImageNet触发了我们所处的当前AI盛夏-这是源自2009年原始论文的一个想法:
It’s not about the algorithm. It’s about the data.
这与算法无关。 这与数据有关。
Every machine learning algorithm performs differently with a small training dataset, like a couple hundred or thousand data points. If I pitted logistic regression against a convolutional neural network in identifying cats and dogs, the neural network would perform significantly better.
每种机器学习算法在一个小的训练数据集(例如数百或数千个数据点)上的执行效果都不同。 如果我在识别猫和狗时将逻辑回归与卷积神经网络相对比,则神经网络的性能会明显更好。
But when I increase the size of the training dataset, the accuracy difference becomes smaller. In a lot of cases, as the number of training data points increases, the algorithmic performances all approach similar plateaus:
但是,当我增加训练数据集的大小时,精度差异变小。 在很多情况下,随着训练数据点数量的增加,算法的性能都接近相似的平稳状态:
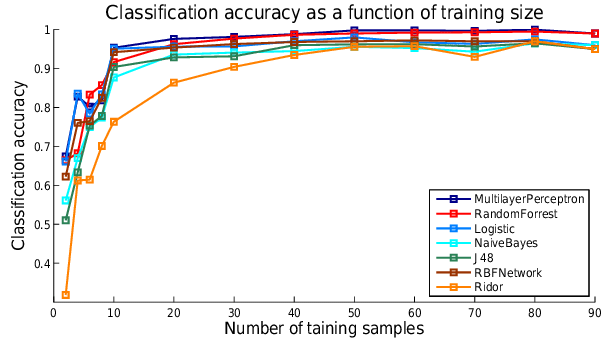
Why improve the underlying algorithm when you can simply throw more data at it?
当您可以简单地向其添加更多数据时,为什么要改进其基础算法呢?
Thusly, machine learning skyrocketed to new heights, achieving classification accuracies that were previously unheard of. Training databases ballooned in size as previously impractical tasks like recognizing handwriting became trivial and were packaged into everyday products.
因此,机器学习飞速发展,达到了以前闻所未闻的分类精度。 由于以前不切实际的任务(例如识别笔迹)变得微不足道,因此培训数据库的规模Swift膨胀,并被打包到日常产品中。
But people soon recognized a fundamental flaw with this framework: the algorithms were training on larger and larger datasets often had incapabilities that prevented their accuracies from increasing beyond a certain limit.
但是人们很快意识到这个框架的一个根本缺陷:算法在越来越大的数据集上进行训练,这些数据集通常都具有无法阻止其准确度超过一定限制的功能。
Consider a picture of a cat. Since ImageNet was first published, algorithms could identify cats really well — as long as the training data accurately represented the cat in three dimensions from every angle. The best neural network for this classification — the convolutional neural network — can’t create a 3D representation of the image it’s trained on. So the second you showed the algorithm a picture of a cat from an angle that the algorithm has not seen, the algorithm fails (this issue is called “pose estimation”).
考虑一张猫的照片。 自从ImageNet首次发布以来,只要训练数据可以从各个角度准确地在三个维度上表示猫,算法就可以很好地识别猫。 用于此分类的最佳神经网络-卷积神经网络-无法为其所训练的图像创建3D表示。 因此,第二次从该算法未看到的角度向该算法显示了一张猫的图片,该算法失败了(此问题称为“姿势估计”)。
Fundamental flaws like these are scattered everywhere in artificial intelligence. Flaws like these were uncovered in the development of IBM Watson, which led to its ultimate demise as a general purpose AI. Without any kind of algorithm development to remove these flaws, it’s easy to see how we’re on the cusp of an AI winter.
诸如此类的基本缺陷分散在人工智能中。 在IBM Watson的开发中发现了类似的缺陷,这最终导致其最终消失为通用AI。 如果没有任何类型的算法开发来消除这些缺陷,就很容易看出我们正处于AI冬季的风口浪尖上。
Enter Geoffrey Hinton, a computer science professor at the University of Toronto. Dr. Hinton has focused on algorithmic development since 1978, was the first to apply the backpropagation algorithm to deep neural networks, and solved the pose estimation problem by introducing an entirely new “capsule” neural network.
输入多伦多大学计算机科学教授Geoffrey Hinton。 Hinton博士自1978年以来一直专注于算法开发,是第一个将反向传播算法应用于深度神经网络的人,并通过引入全新的“胶囊”神经网络解决了姿态估计问题。
Instead of increasing the size of the dataset, Dr. Hinton focuses on specific algorithm-level issues and solves them methodically by tweaking the algorithm’s core architecture. He developed AlexNet, the pioneering neural network that destroyed the competition in ImageNet 2012.
Hinton博士没有增加数据集的大小,而是关注特定算法级别的问题,并通过调整算法的核心体系结构来有条理地解决这些问题。 他开发了AlexNet,这是一种开创性的神经网络,它破坏了ImageNet 2012的竞争。
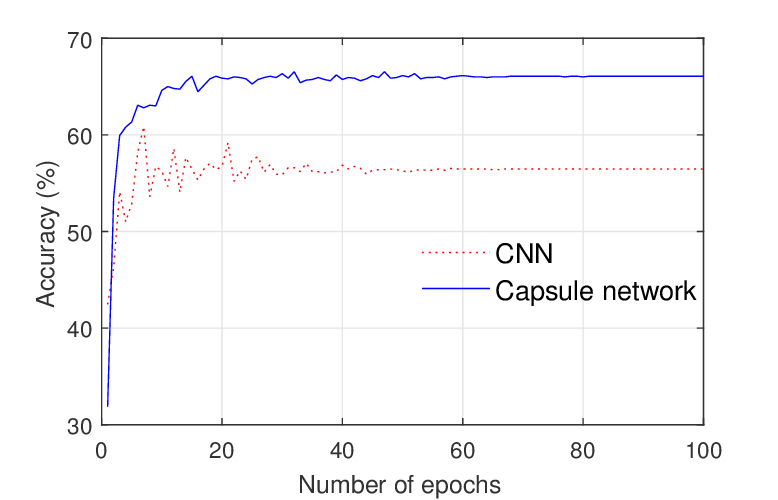
Dr Hinton’s focus away from increasing pure data volume is, for the time being, an uncommon one, likely because successful algorithmic development requires a rare and deep understanding of how the machine learning algorithms work. The focus defies the “marginal improvement” paradigm that data scientists presently believe, since the work put into improving an algorithm may not reliably translate into results. In other words, while adding data will always yield some kind of (albeit diminishing) benefit, you could develop a hundred new algorithms entirely before stumbling across one that works.
Hinton博士目前关注的重点不是增加纯数据量,这是一个罕见的问题,这可能是因为成功的算法开发需要对机器学习算法的工作原理有一个罕见而深刻的了解。 该焦点无视数据科学家目前认为的“边际改进”范式,因为改进算法的工作可能无法可靠地转化为结果。 换句话说,虽然添加数据将始终带来某种(尽管在减少)收益,但是您可以在遇到一个可行的算法之前完全开发出一百种新算法。
But the rewards for better algorithms often dwarf anything that larger datasets can provide. The development of deep neural networks, for instance, introduced the very idea of chaotic predictions and high accuracy object recognition.
但是,使用更好的算法所获得的回报通常使大型数据集所能提供的一切相形见war。 例如,深度神经网络的发展引入了混沌预测和高精度目标识别的思想。
In the literature, the duel between data volume and algorithm development can be seen by the sheer volume of papers published on the subject. And data volume is winning. For every paper on algorithm improvement, there are hundreds of papers dealing with increasing training data volume.
在文献中,有关该主题的大量论文可以看出数据量与算法开发之间的决斗。 数据量大增。 关于算法改进的每篇论文,都有数百篇涉及增加训练数据量的论文。
Venture capitalists, entrepreneurs and professors alike pounce on the latest datasets; companies are making their own internal datasets to edge out the competition; there are even entire Mechanical Turk communities whose sole job is to painstakingly scour the internet, label image data and sell those datasets for profit. In machine learning, data is king and has been since 2009.
风险资本家,企业家和教授都涌向了最新的数据集。 公司正在建立自己的内部数据集来竞争。 甚至还有整个Mechanical Turk社区,其唯一的工作就是艰苦地搜寻互联网,标记图像数据并出售这些数据集以获取利润。 自2009年以来,在机器学习中,数据为王。
And so the AI game of thrones continues. On the one hand, scientists in Fei Fei Li’s camp are pushing for better and better quality data to gradually boost performance. On the other hand, scientists in Geoffrey Hinton’s camp are developing new algorithms entirely to “leapfrog” over the entire field by solving a fundamental flaw in current machine learning models. (The former camp feels like the Baratheons as they hold the throne, and the latter seems like the Starks — they’re waiting for winter.)
因此,宝座的AI游戏仍在继续。 一方面,李飞飞营地里的科学家们正在努力寻找越来越好的质量数据,以逐步提高性能。 另一方面,杰弗里·欣顿(Geoffrey Hinton)阵营的科学家们正在开发新的算法,以通过解决当前机器学习模型中的一个基本缺陷来完全“跨越”整个领域。 (前一个阵营感觉就像是巴拉松家族,因为他们占据了王位,而后者似乎像斯塔克斯-他们在等待冬天。)
Clearly, the best solution is a combination of the two. But it likely errs on the side of algorithm development. There’s only so much that larger data volumes can achieve, and training on millions of photos is certainly not how humans learn. If you show me just a few pictures of a mancun, for example, chances are that I’ll be pretty good at identifying them afterward.
显然,最好的解决方案是两者的结合。 但是它可能会在算法开发方面出错。 只能实现更大的数据量,而对数百万张照片进行培训无疑不是人类学习的方式。 例如,如果您只给我看几张有关曼昆的照片,那么我以后很可能会很好地识别它们。
Dr. Hinton seems to believe that the AI winter is inevitable. In an informal meeting with Dr. Nick Bostrom (the “Universe Simulation Hypothesis” guy), he’s quoted as stating that he didn’t expect general AI to be developed any sooner than 2070. If that’s true, then this upcoming AI winter may be the longest on record as scientists edge toward the “master algorithm” that will eventually lead to sentient AI like Baymax.
Hinton博士似乎认为AI冬天是不可避免的。 在与尼克·博斯特罗姆博士(“宇宙模拟假说”专家)举行的非正式会议上,他被引述说,他不希望通用人工智能的发展要早于2070年。如果的确如此,那么这个即将来临的人工智能冬天可能是这是有记录以来时间最长的,因为科学家们趋向于“主算法”,最终将导致像Baymax这样有感觉的AI。
To have meaningful advances in AI, I think we need to pursue heavy algorithm development. While no one is questioning the need for good data, the benefit from increased data volume has had its day. It’s time to set aside our attachment to the current art, and work on novel methods that learn in new ways. It’s hard work, but well worth it for the promises of general purpose AI.
为了在AI方面取得有意义的进步,我认为我们需要进行繁重的算法开发。 尽管没有人质疑对优质数据的需求,但不断增加的数据量所带来的好处却已初露端倪。 现在该抛弃我们对当前艺术的依恋,并研究以新方式学习的新颖方法。 这是一项艰苦的工作,但对于通用AI的承诺来说却是值得的。
And because winter is coming.
而且因为冬天来了。
翻译自: https://medium.com/tri-pi-media/fei-fei-li-geoffrey-hinton-and-the-ai-game-of-thrones-afcb76d54f5e
杰弗里·辛顿 反向传播