我们应该从思想与机器之间的张力中学习 (We should learn from the tension between mind and machine)
Every human bliss and kindness, every suspicion, cruelty, and torment ultimately comes from the whirring 3-pound “enchanted loom” that is our brain and its other side, the cloud of knowing that is our mind.
每个人类的幸福和善良,每个怀疑,残酷和折磨最终都来自呼brain的三磅重的“魔法织机”,这是我们的大脑,另一端是知之云,这是我们的思想。
It’s an odd coincidence that serious study of the mind and the brain bloomed in the late 20th century when we also started to make machines that had some mind-like qualities.
奇怪的巧合是,在20世纪后期,当我们也开始制造具有某些类似于心理的特质的机器时,对思维和大脑进行了认真的研究。
Now, with information technology we have applied an untested amplifier to our minds, and cranked it up to eleven, running it around the clock, year after year. Because we have become a culture of crisis, we are good at asking, what has gone wrong? What could go wrong?
现在,借助信息技术,我们在脑海中应用了未经测试的放大器,并将其放大到11个,并且年复一年地全天候运行。 因为我们已经成为一种危机文化,所以我们善于问,哪里出了问题? 可能出什么问题了?
But is the conjunction of natural and artificial mind only ill-favored, or might we not learn from both by comparison?
但是,自然思维和人工思维的结合是否只是不好用的,还是我们不能通过比较从两者中学习?
一个学习的机会。 (A learning opportunity.)

Years ago I decided to study animal behavior, hoping that it would help us to better understand our nature. But the culture then was indifferent and sometimes hostile to the idea of human nature having anything to do with biology. The enlightened “narrative” was radically tilted towards nurture, not nature.
多年前,我决定研究动物的行为,希望它能帮助我们更好地了解我们的天性。 但是,当时的文化是冷漠的,有时对与生物学有关的人性观念持敌对态度。 开明的“叙事”从根本上倾向于培养,而不是自然。
Once my monkey studies were published, facing society’s antipathy, I had to become some other cog in the Great Grinding Wheel. Long afterward, I looked around and found that (a) in the interim much had been learned about the mind, and (b) our species was still fanning a social, political, economic, environmental dumpster-fire.
当我的猴子研究发表后,面对社会的反感,我不得不成为“大砂轮”中的其他齿轮。 不久之后,我环顾四周,发现(a)在此期间,人们对思想有了很多了解,并且(b)我们的物种仍在煽动社会,政治,经济,环境垃圾场。
True to my previously thwarted nature, I started looking for some truth in the revived mind sciences.
出于我以前受挫的本性,我开始在复兴的思维科学中寻找一些真理。
2009: Philosopher Thomas Metzinger publishes The Ego Tunnel, which says that consciousness is constructed by the brain like a virtual reality.
2009年:哲学家托马斯·梅琴格(Thomas Metzinger)出版了《自我隧道》 ,它说意识是由大脑像虚拟现实一样构造的。
But what truths would be helpful? Would it do us any good to know how our minds arise from matter? How our ego tunnels burrow through time and space to create our own reality? How that same ego is the tail wagged by the dog of the unconscious? Who will care, given our multiple unfolding crises?
但是,哪些真相会有所帮助? 知道我们的思想是如何从物质中产生的,对我们有好处吗? 我们的自我隧道如何穿越时空探索创造我们自己的现实? 昏迷的狗的尾巴怎么摇同样的自我? 考虑到我们多重危机的爆发,谁会关心?
Some think that our minds set a distant, unreachable boundary on what machines could ever do. Others think that machines could someday surpass us like we surpass a bug. These extremes and many views in between offer ways for us to explore the nature of minds, what they can do, and what they might achieve.
有人认为我们的思想为机器可以做什么设定了遥不可及的界限。 其他人则认为,机器有朝一日可能会像我们超过错误一样超越我们。 这些极端情况和介于两者之间的许多观点为我们提供了探索思想的本质,他们可以做什么以及他们可能取得的成就的方式。
2038: The Nobel Foundation establishes a new Mind Science prize amid cries of ‘wrong-headed’, ‘unprecedented’, and ‘long overdue’.
2038年:诺贝尔基金会在“错误的头脑”,“前所未有的”和“长期逾期”的呼声中设立了新的思维科学奖。
Some areas of research connecting minds and machines are weighty indeed but obscured from public attention.
确实,连接思想和机器的某些研究领域确实很重要,但是却被公众的关注所掩盖。
价值观和决策理论。 (Values and decision theory.)
Our ongoing attempts to make artificial minds are starting to teach us about ourselves. When we give machines the ability to make judgments we run into now-familiar moral dilemmas. The self-driving car as executioner. Pernicious biases.
我们不断进行的使人为的想法的尝试开始教会我们关于自己的知识。 当我们赋予机器进行判断的能力时,我们会遇到现在已经熟悉的道德困境。 自动驾驶汽车担任execution子手。 有害的偏见。
From a higher-level view, AI theorists see the above as questions about values. Before they get too powerful, we need our intelligent machine agents to act as if they had values like ours. Research currently calls the problem, AI alignment, meaning ‘aligned with our values.’
从更高的角度来看,人工智能理论家将以上内容视为关于价值的问题。 在它们变得无能为力之前,我们需要我们的智能机器代理像他们一样拥有自己的价值。 目前,研究将问题称为AI对齐 ,意思是“与我们的价值观保持一致”。
Alignment researchers prefer to work with formal, math-like systems, and a favorite is a framework called decision theory. It concerns how to make decisions about reaching goals when you’re assuming a set of values. The values are in a utility function that rates the preferences for all possible outcomes of the decisions.
一致性研究人员更喜欢使用类似于数学的形式系统,最喜欢的是称为决策理论的框架。 它涉及在假设一组价值时如何做出有关达成目标的决策。 这些值位于效用函数中,该函数对决策的所有可能结果的优先级进行评估。
Decision theories let researchers tackle general questions like: how do you formalize values so that pursuing one value doesn’t violate another? So far, decision theory shows how hard it can be to use values to guide even the simplest behavior for a powerful agent. Suppose you tell the AI to cure cancer, but not to cause any other side effects in the world. It might conclude that “no side effects” means continuing to let people die of their cancer. Instead of stopping after filling the bucket the sorcerer’s apprentice might indeed flood the castle or even the whole world.
决策理论使研究人员可以解决以下一般性问题:如何将价值观形式化,以便追求一种价值观不会违反另一种价值观? 到目前为止,决策理论表明,使用值来指导功能强大的代理即使是最简单的行为也可能非常困难。 假设您告诉AI治愈癌症,但不引起世界上任何其他副作用。 它可能得出结论,“无副作用”意味着继续让人们死于癌症。 巫师的学徒可能不会在装满水桶之后停下来, 实际上确实会淹没城堡甚至整个世界。

Humans have developed ethical systems without gaining any consensus. Ethicist William Frankena made the following list of things that many people value, nearly all of which can conflict with each other.
人类已经建立了伦理体系,而没有获得任何共识。 伦理学家威廉·弗兰肯纳(William Frankena)列出了许多人认为有价值的东西,几乎所有这些东西都可能相互冲突。
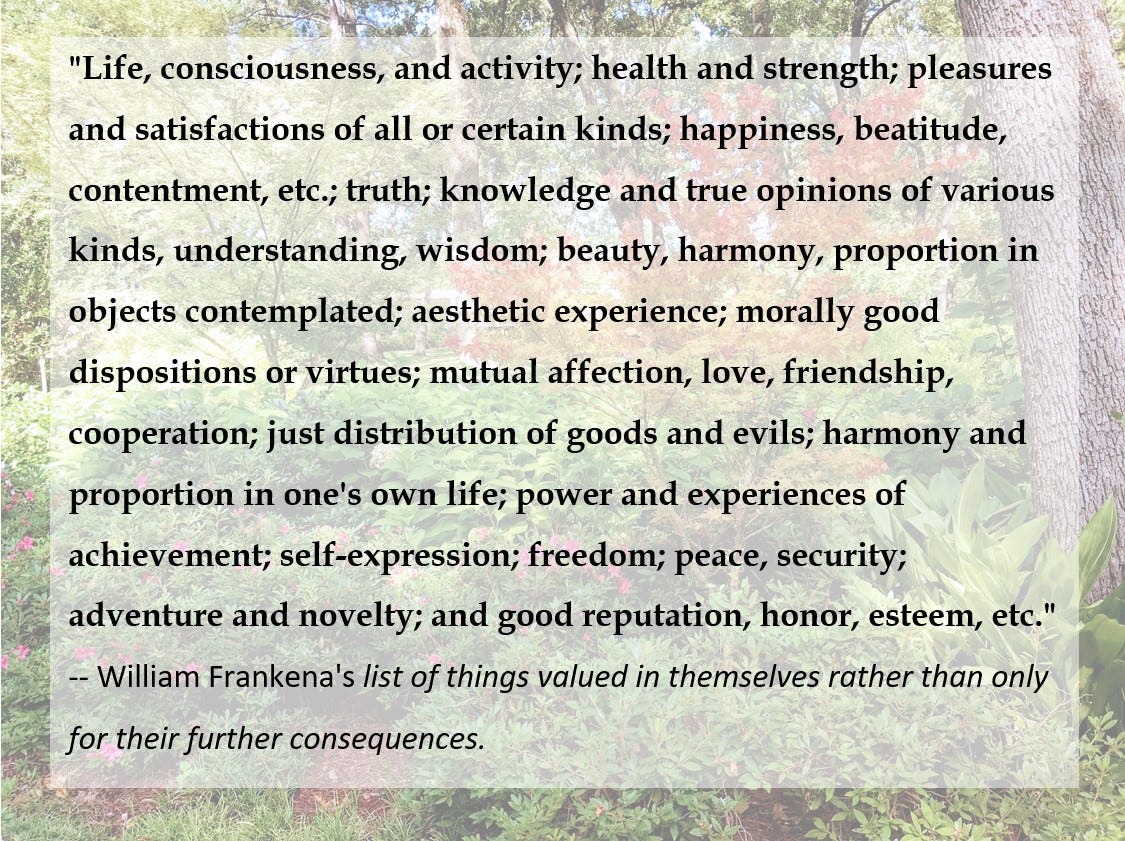
How do we resolve the fact that human values are incomplete, contradictory, and can change with circumstances?
我们如何解决人类价值观不完整,自相矛盾并且会随环境而变化的事实?
2004: Eliezer Yudkowsky defines ideal human values to be our “Coherent Extrapolated Volition”
2004年:Eliezer Yudkowsky将 理想的人类价值观 定义 为我们的“相干推断意志”
Some have proposed having the machines themselves help us discover more coherent, long-term values. Others have found that the value learning problem, at least for machines, is fiendishly complex. They have also found that, if you somehow can specify the values, it is hard to see how to make a machine retain them, especially if it is smart enough to be capable of modifying itself.
有些人建议让机器本身帮助我们发现更连贯的长期价值。 其他人发现,至少对于机器而言, 价值学习问题非常复杂。 他们还发现,如果您能以某种方式指定值,则很难看到如何使机器保留它们,特别是如果它足够聪明以能够自我修改。
意识和自我塑造。 (Consciousness and self-modeling.)
Researchers are trying to devise how to make machines that behave adaptively. The crux is that to improve how to do some task you need to model the situation in which it occurs and then use the model to evaluate (decision theory again) different approaches. For a machine operating in the real world, the situation includes the machine itself. So a flexibly adaptive machine must model its very self as part of its operating model.
研究人员正在尝试设计如何制造具有自适应性能的机器。 问题的关键是要改进如何完成某些任务,您需要对发生的情况进行建模,然后使用该模型评估(再次是决策理论)不同的方法。 对于在现实世界中运行的机器,情况包括机器本身。 因此,灵活自适应的机器必须将其自身建模为运行模型的一部分。
2007: “Consciousness stems from the structure of the self-models that intelligent systems use to reason about themselves.” — Drew McDermott, AI researcher
2007年: “意识源于智能系统用来自我思考的自我模型的结构。” — AI研究人员Drew McDermott
But wait — there’s a strong theory that human beings do the same thing, and that our mental models of ourselves-in-the-world cause the experience of consciousness. So will we, intentionally or not, make machines that are phenomenally conscious? Would their conscious experience resemble ours in some ways (could they suffer? be happy? trustworthy? devious?) or be completely alien? Would we believe what they said to us about their internal conscious states?
但是,等等。有一个强有力的理论,即人类会做同样的事情 ,而我们在世间的心理模型会导致意识体验。 那么,我们是否会有意制造非凡的意识机器? 他们的有意识经历会在某种程度上类似于我们的经历(他们会遭受痛苦吗?会快乐吗?值得信赖吗?会变态?)还是完全陌生? 我们相信他们对我们的内在意识状态所说的话吗?
1995: “The really hard problem of consciousness is the problem of [having] experience.” — David Chalmers
1995年:“意识上真正困难的问题是[拥有]经验的问题。” — 大卫·查默斯 ( David Chalmers)
We all know that living a good life — and for many of us, living any life — is fraught with difficulty. We are getting a fresh look at that bedrock fact. This happens when we try to imagine safely getting help from our intelligent machines in realistic settings: where they are choice-making agents who have to model their world and themselves embedded in it. The theorists call the issue “embedded agency.”
我们都知道,过上美好的生活-对我们许多人而言,过着任何生活-都充满了困难。 我们正在重新审视这一基石事实。 当我们试图想象在现实的环境中从智能机器安全地获得帮助时,就会发生这种情况:他们是做出选择的代理商,他们必须对自己的世界进行建模并嵌入其中。 理论家称该问题为“嵌入式代理”。
嵌入式代理商。 (Embedded agency.)

2018: “This is Emmy. Emmy is playing real life … Emmy is within the environment that she is trying to optimize.” Garrabrant, Embedded Agents
2018年:“这是艾美奖。 艾美(Emmy)扮演着现实生活……艾美(Emmy)处在她试图优化的环境中。” 垃圾邮件, 嵌入式代理
To decide on future courses of action in a changing world, you have to predict what new situations will arise to which you must respond. But your own previous behavior will affect what those new situations will be. And likewise for your behavior before those previous situations, and so on, all the way back to Start. The more you chain together these predictions over time, the less accurate they will be. It also gets rapidly more difficult to compute longer chains of actions. This prediction issue alone might paralyze a literal-minded AI.
要决定在变化的世界中未来的行动方针,您必须预测将要应对的新情况。 但是您以前的行为会影响这些新情况。 同样,对于您在以前情况之前的行为,等等,一直到开始。 随着时间的推移,将这些预测链接在一起的次数越多,它们的准确性就越低。 计算更长的动作链也变得越来越困难。 仅此预测问题就可能使字面意义上的AI瘫痪。
In the same situation, people just “go for it.” We make a decision, telling ourselves we’ll work it out somehow. We certainly don’t want a powerful machine doing that.
在相同情况下,人们只是“追求”。 我们做出决定,告诉自己我们将以某种方式解决 。 我们当然不希望功能强大的机器这样做。
Furthermore, if you are an agent who makes choices in the world, then when you see the outcome of a choice you also know that you could have done otherwise. In people, this can cause feelings of justification (I made the right choice) or regret (oh, if only I hadn’t done that). These feelings help integrate us into our society, and so one theory (Frith & Metzinger, What's the Use of Consciousness?) is that we evolved these feelings to get along with others.
此外,如果您是一个在世界上做出选择的代理商,那么当您看到选择的结果时,您也知道您可以做其他事情。 在人中,这可能会引起称义的感觉( 我做出了正确的选择 )或后悔( 哦,如果只是我没有这样做的话 )。 这些感觉有助于我们融入社会,因此一种理论(Frith&Metzinger, “意识的用途是什么?” )是我们发展了这些感觉来与他人相处。
Any problem-solving machine should also respond to feedback on its choices in ways that improve future ones. The first thing it should do is what we can do but usually don’t: adjust our world model based on what happened with our choice. We could also think harder about the next decision. For “big” decisions we often do that. But research says that most of our decisions are made unconsciously, and our conscious thinking merely justifies them.
任何解决问题的机器也应以改进未来的方式响应其选择的反馈。 它应该做的第一件事是我们可以做但通常不做的事情:根据我们的选择来调整我们的世界模型。 我们也可以为下一个决定加倍考虑。 对于“重大”决策,我们经常这样做。 但是研究表明,我们的大多数决策都是在无意识的情况下做出的,而我们有意识的思考只是为它们辩护。
A machine could improve a decision by thinking more, but there are three issues, which we can call the think-more problems. One is the chaining prediction issue mentioned above. Another is knowing when it has thought enough before taking action. The third is logical twists that can arise when trying to predict its own behavior in order to plan that same behavior.
机器可以通过考虑更多问题来改善决策,但是存在三个问题,我们可以将其称为更多思考问题。 一种是上面提到的链接预测问题。 另一个是在采取行动之前知道何时已足够考虑。 第三是在试图预测自己的行为以计划相同行为时可能出现的逻辑扭曲。
A very advanced machine might decide to push this farther by trying to improve its basic intelligence. That idea leads to a paradox. For a successful improvement, it would need to predict what its smarter self would do. But if it could predict that, then it would have to already be the smarter self! Both the think-more and improve-me problems are similar from a computer science point of view, and researchers are trying to figure them out.
一台非常先进的机器可能决定通过尝试提高其基本智能来进一步推动这一发展。 这个想法导致了悖论。 为了获得成功的改进,它需要预测其更聪明的自我将做什么。 但是,如果它可以预测这一点,那么它就必须已经是更聪明的自我! 从计算机科学的角度来看,“思考更多”问题和“改进我”问题都是相似的,研究人员正在设法解决这些问题。
Again, faced with the issue of possible and unknowable improvement, people eventually decide to just do or not do. We can’t trust an AI with that decision.
再次,面对可能的和不可知的改进问题,人们最终决定做还是不做。 我们不能凭借这一决定信任AI。
The problem just described is named Vingean Reflection in honor of sci-fi author Vernor Vinge, who said that a writer is unable to predict the behavior of a character smarter than the writer. Other versions are: a child can’t write (or reason) realistically about an adult; humans can’t predict what a superintelligent AI would do.
刚刚描述的问题被命名为Vingean Reflection,以纪念科幻作家Vernor Vinge,他说作家无法预测比作家聪明的角色的举止。 其他版本是:儿童不能真实地写(或说)关于成年人的东西; 人类无法预测超级智能AI会做什么。
We know that people can find it hard to trust their experts, because of Vinge’s reason. If we find a way to think usefully about AIs smarter than us, maybe it would apply to our own trust issues as well.
我们知道,由于Vinge的原因,人们很难相信自己的专家。 如果我们找到一种比我们更聪明地思考AI的方法,也许它也适用于我们自己的信任问题。
代理和思想化。 (Agency and mentalizing.)
Our minds are primed to explain events as if they were the result of intentions. We have our own intentions, we believe that other people have theirs, and we even assign intentions to random natural events. To apply such a mentalizing analysis to another person is called using a Theory of Mind. Our predictions are weak, but we use them to muddle through.
我们的头脑已准备好将事件解释为好像它们是意图的结果。 我们有自己的意图,我们相信其他人也有他们的意图,我们甚至将意图分配给随机的自然事件。 使用心理理论将这种精神分析应用于他人。 我们的预测很微弱,但是我们用它们来弄糊涂。
An intelligent machine interacting with humans would need a theory of others’ minds as part of its world model. But would an AI model a human differently than it would model a car, or a tornado? You could say that, sure, a human has goals, so the AI tries to infer the goals and use that to predict behavior. Maybe we would learn something about our own goals and behavior if a really smart AI modeled how we use them. And that’s an encouraging idea.
与人互动的智能机器将需要其他人的思想理论作为其世界模型的一部分。 但是,人工智能对人的建模与汽车或龙卷风的建模有区别吗? 您可以说,当然,一个人有目标,因此AI会尝试推断目标并将其用于预测行为。 如果真正智能的AI能够模拟我们如何使用目标和行为,我们可能会学到一些有关我们自己的目标和行为的知识。 这是一个令人鼓舞的想法。
First, consider how an AI might model things that aren’t alive.
首先,请考虑一下AI如何建模尚未出现的事物。
The AI could say that the car has goals, which are quite simple: when the piston goes down, the goal is to draw in an optimal fuel/air mixture into the cylinder. So the fuel injector implements that goal, given input from various places, like current road speed and accelerator position. When the brake pedal is depressed, the goal is to stop smoothly.
人工智能可以说汽车的目标很简单:当活塞下降时,目标是将最佳的燃油/空气混合物吸入气缸。 因此,燃油喷射器会在给定来自不同位置的输入(例如当前道路速度和油门位置)的情况下实现该目标。 踩下制动踏板时,目标是平稳停止。
In the case of a tornado, the “goals” would be things like absorbing and dispersing heat energy from the air. Or, conservation of angular momentum. And maybe transfer of energy and momentum to whatever stands in the tornado’s way, like buildings and trees. So, what makes people different from “things” like cars and tornados?
在龙卷风的情况下,“目标”就像是吸收和分散空气中的热能。 或者,保持角动量。 也许将能量和动量传递到龙卷风所阻挡的任何地方,例如建筑物和树木。 那么,是什么使人们与汽车和龙卷风之类的“事物”不同?
2007: In order to explain how an information processing system can have a model of something, there must be a prior notion of intentionality, that explains why and how symbols inside the system can refer to things. — Drew McDermott
2007年:为了解释信息处理系统如何具有事物模型,必须先有一个意图性概念,该概念解释了系统中的符号为何以及如何引用事物。 — 德鲁·麦克德莫特 ( Drew McDermott)
The pursuit of goals is evidence that we have intentions. But, to current philosophers and psychologists (and, originally, Franz Brentano) “intentionality” means that all our mental states are directed towards something: either other mental states or things in the outside world. In our mentalizing, we imagine elaborate “levels of intentionality”. Such as (count the italicized verbs to get the level): “Sue believes that Ralph thinks that Alan wants to join his book club, but Alan doesn’t even like books.”
追求目标是我们有意图的证据。 但是,对于当前的哲学家和心理学家(最初是弗朗兹·布伦塔诺,最初是弗朗茨·布伦塔诺)而言,“意图性”意味着我们所有的精神状态都针对某种事物 :其他精神状态或外界事物。 在我们的思维过程中,我们想象着精心设计的“意向性水平”。 例如(算出斜体动词即可获得水平):“ Sue 认为 Ralph 认为 Alan 希望加入他的读书俱乐部,但Alan甚至不喜欢读书。”
A lot of philosophy and science has been written about the difference between animate and inanimate things, or things that have agency and intentionality versus those that don’t. We might clear some of that up if we build an AI that models intentionality correctly.
关于无生命的东西和无生命的东西,或者具有代理和有意向的东西与没有代理和有意向的东西之间的区别,已经有很多哲学和科学的著作。 如果我们构建能够正确建模意向性的AI,我们可能会清除其中的一些内容。
Another implication of such modeling takes us back to the consciousness issue. Maybe an AI that successfully models the intentions of human agents would do like we do. It would apply the same modeling principles to itself and wind up being, or at least acting like it was, conscious. Hence an ethical can o’ worms: how do we treat the AI, and how would it treat us in return?
这种建模的另一个含义使我们回到了意识问题。 也许成功地模仿人类行为者意图的AI会像我们一样做。 它将对自身应用相同的建模原理,并最终获得意识 ,或至少表现得像意识一样 。 因此,有道德的人会蠕虫:我们如何对待AI,以及它将如何回报我们?
倾覆的石头。 (Overturning Stones.)
Our attempts to understand what it would take to have aligned AIs are also a fresh look at some issues of being human. Old problems of philosophy and psychology are being approached using new tools such as decision theory, game theory, logic, and probability.
我们试图理解拥有一致的AI所需要的尝试,也重新审视了人类的某些问题。 哲学和心理学的旧问题正在使用新工具来解决,例如决策理论,博弈论,逻辑和概率。
The AI researchers who study decision theory know that the extreme rationality of their theorizing could seem cold and alien, as well as hard to follow. They also know that our behavior is riddled with unconscious cognitive biases, learned prejudices, and narrow ideologies. We don’t want our lousy thinking skills transferred to our machines. When we research how they can do a better job, we might improve ourselves.
研究决策理论的AI研究人员知道,他们理论上的极端合理性似乎很冷漠,陌生,并且很难遵循。 他们还知道,我们的行为充满了无意识的认知偏见,博学的偏见和狭窄的意识形态。 我们不希望将糟糕的思维能力转移到我们的机器上。 当我们研究他们如何做得更好时,我们可能会改善自己。
2049: First melding of multiple humans into a multi-mind. Was predicted in 2020 by an obscure informaticist.
2049年:首先将多个人融为一体。 一位不知名的信息学家在2020年预测到了这一点。
Even if advanced AI never happens the effort might be helpful with human problems. Nick Bostrom and others have noted the parallel between advanced AIs and human organizations like governments and corporations. Both exceed the capabilities of individual humans. Both tend towards the amoral. We need a better consensus on the values for aligning them. Then we need ways to sustain that alignment, so we can trust them when their successors gain new powers.
即使高级AI永远不会发生,这种努力也可能有助于解决人为问题。 尼克·波斯特伦(Nick Bostrom)等人指出,先进的AI与政府和企业等人类组织之间的相似之处。 两者都超出了个人的能力。 两者都倾向于不道德的。 我们需要更好地达成一致的价值观共识。 然后,我们需要保持这种一致的方式,以便在他们的继任者获得新权力时可以信任他们。
翻译自: https://medium.com/swlh/two-kinds-of-minds-c77d72b4ccf3