美剧迷失
Machine translation doesn’t generate as much excitement as other emerging areas in NLP these days, in part because consumer-facing services like Google Translate have been around since April 2006.
如今,机器翻译并没有像其他NLP新兴领域那样令人兴奋,部分原因是自2006年4月以来,面向消费者的服务(例如Google Translate)就已经出现了。
But recent advances, particularly the work by Hugging Face in making transformer models more accessible and easy to use, have opened up interesting new possibilities for those looking to translate more than just piecemeal sentences or articles.
但是,最近的进步,尤其是Hugging Face在使转换器模型更易于访问和易于使用方面所做的工作,为那些希望翻译不只是零碎的句子或文章的人提供了有趣的新可能性。
For one, small batch translation in multiple languages can now be run pretty efficiently from a desktop or laptop without having to subscribe to an expensive service. No doubt the translated works by neural machine translation models are not (yet) as artful or precise as those by a skilled human translator, but they get 60% or more of the job done, in my view.
首先,现在可以从台式机或笔记本电脑高效运行多种语言的小批量翻译,而无需订阅昂贵的服务 。 毫无疑问, 神经机器翻译模型的翻译作品还不如熟练的人工翻译者那么巧妙或精确,但我认为它们完成了60%或更多的工作。
This could be a huge time saver for workplaces that run on short deadlines, such as newsrooms, to say nothing of the scarcity of skilled human translators.
对于工作期限很短的工作场所(例如新闻编辑室)而言,这可以节省大量时间,更不用说熟练的人工翻译人员的匮乏了。
Over three short notebooks, I’ll outline a simple workflow for using Hugging Face’s version of MarianMT to batch translate:
在三个简短的笔记本上 ,我将概述一个简单的工作流程,以使用Hugging Face的MarianMT版本批量翻译:
1.数据集和翻译后的输出 (1. DATA SET AND TRANSLATED OUTPUT)
There are two datasets for this post. The first comprises 11 speeches in four languages (English, Malay, Chinese, and Tamil) taken from the website of the Singapore Prime Minister’s Office. The second dataset consists of five random English news stories on Covid-19 published on Singapore news outlet’s CNA’s website in March 2020.
此帖子有两个数据集。 在第一个包括11个演讲从网站采取四种语言(英语,马来语,中国语和泰米尔语) 新加坡总理办公室 。 第二个数据集由2020年3月在新加坡新闻媒体的CNA网站上发布的Covid-19上的五个随机英语新闻报道组成。
Results of the output CSV files with the machine translated text and original copy can be downloaded here.
带有机器翻译文本和原始副本的输出CSV文件的结果可在此处下载。
At the time of writing, you can tap over 1,300 open source models on Hugging Face’s model hub for machine translation. As no MarianMT models for English-Malay and English-Tamil (and vice versa) have been released to date, this series of notebooks will not deal with these two languages for now. I’ll revisit them as and when the models are available.
在撰写本文时,您可以在Hugging Face的模型中心上挖掘1,300多种开源模型进行机器翻译。 由于迄今为止尚未发布针对英语-马来语和英语-泰米尔语(反之亦然)的MarianMT模型,因此该系列笔记本目前暂不支持这两种语言。 我将在可用模型时重新访问它们。
I won’t dive into the technical details behind machine translation. Broad details of Hugging Face’s version of MarianMT can be found here, while those curious about the history of machine translation can start with this fairly recent article.
我不会深入探讨机器翻译背后的技术细节。 可以在此处找到Hugging Face版本的MarianMT的广泛细节,而对机器翻译历史感到好奇的人可以从这篇相当新的文章开始 。
2A。 3种语言的英语到汉语机器翻译 (2A. ENGLISH-TO-CHINESE MACHINE TRANSLATION OF 3 SPEECHES)
The three English speeches I picked for machine translation into Chinese range from 1,352 to 1,750 words. They are not highly technical but cover a wide enough range of topics — from Covid-19 to Singapore politics and domestic concerns — to stretch the model’s capability.
我选择将机器翻译成中文的三个英语演讲的范围是1,352至1,750个单词。 它们的技术性不是很高,但是涵盖了足够广泛的主题(从Covid-19到新加坡政治以及国内关注的问题),以扩展模型的功能。
The text was lightly cleaned. For best results, the sentences were translated one at a time (you’ll notice a significant drop in translation quality if you run the entire speech through the model without tokenizing at the sentence level). The notebook took just minutes to run on my late-2015 iMac (32Gb RAM) — but may vary depending on your hardware.
文本被轻轻清理了。 为了获得最佳结果,句子一次被翻译一次(如果您在整个模型中运行整个语音而没有在句子级别进行标记化,则翻译质量会明显下降)。 这款笔记本在我2015年末的iMac(32Gb RAM)上运行仅需几分钟,但具体取决于您的硬件。

Some of the familiar problems with machine translation are apparent right away, particularly with the literal translation of certain terms, phrases and idioms.
机器翻译中的一些常见问题会立即显现出来,尤其是某些术语,短语和习语的直译时。
May Day, for instance, was translated as “五月节” (literally May Festival) instead of 劳动节. A reference to “rugged people” was translated as “崎岖不平的人”, which would literally mean “uneven people”. Clearly the machine translation mixed up the usage of “rugged” in the context of terrain versus that of a society.
例如,“五月节”被翻译为“五月节”(实际上是五月节),而不是劳动节。 提到“崎people的人”被翻译为“崎岖不平的人”,字面意思是“参差不齐的人”。 显然,机器翻译在地形环境和社会环境中混淆了“坚固”的用法。
Here’s a comparison with Google Translate, using a snippet of the second speech. Results from the Hugging Face — MarianMT model held up pretty well against Google’s translation, in my view:
这是使用第二段语音的片段与Google Translate的比较。 在我看来,Hugging Face — MarianMT模型的结果与Google的翻译非常吻合:

To be sure, neither version can be used without correcting for some obvious errors. Google’s results, for instance, translated the phrase “called the election” to “打电话给这次选举”, or to literally make a telephone call to the election. The phrasing of the translated Chinese sentences is also awkward in many instances.
可以肯定的是,如果不纠正一些明显的错误,则无法使用这两个版本。 谷歌的结果,比如,翻译成“叫选”的短语“打电话给这次选举”,或从字面上打了一个电话给选举 。 在许多情况下,中文翻译句子的措词也很尴尬。
A skilled human translator would definitely do a better job at this point. But I think it is fair to say that even a highly experienced translator won’t be able to translate all three speeches within minutes.
在这一点上,熟练的人工翻译肯定会做得更好。 但我认为可以说,即使是经验丰富的翻译人员也无法在几分钟之内翻译全部三篇演讲稿。
The draw of machine translation, for now, appears to be scale and relative speed rather than precision. Let’s try the same model on a different genre of writing to see how it performs.
到目前为止,机器翻译的吸引力似乎是比例和相对速度,而不是精度。 让我们在不同的写作风格上尝试相同的模型,看看其表现如何。
2B。 英文到中文的机器翻译5条新闻文章 (2B. ENGLISH-TO-CHINESE MACHINE TRANSLATION OF 5 NEWS ARTICLES)
Speeches are more conversational, so I picked five random news articles on Covid-19 (published in March 2020) to see how the model performs against a more formal style of writing. To keep things simple, I selected articles that are under 500 words.
演讲更具对话性,因此我在Covid-19(于2020年3月发布)上选择了五篇随机新闻文章,以了解该模型在更正式的写作风格下的表现。 为了简单起见,我选择了500字以内的文章。
The workflow in this second trial is identical to the first, except for additional text cleaning rules and the translation of the articles’ headlines alongside the body text. Here’s what the output CSV file looks like:
除了附加的文本清理规则以及文章标题与正文文本的翻译之外, 第二个试验的工作流程与第一个试验的工作流程相同。 这是输出CSV文件的样子:
Let’s compare one of the examples with the results from Google Translate:
让我们将其中一个示例与Google Translate的结果进行比较:

Both Google and MarianMT tripped up on the opening paragraph, which was fairly long and convoluted. The two models performed slightly better on the shorter sentences/paragraphs, but the awkward literal translations of simple phrases continue to be a problem.
谷歌和MarianMT都在开头段中跳了起来,该段相当长且令人费解。 这两个模型在较短的句子/段落上的表现稍好一些,但是简单短语的尴尬字面翻译仍然是一个问题。
For instance, the phrase “as tourists stay away” was translated by the MarianMT model as “游客离家出走” or “tourists ran away from home”, while Google translated it as “游客远离了当地” or “tourists kept away from the area”.
例如,MarianMT模型将“游客离家出走”一词翻译为“游客离家出走”或“游客离家出走”,而Google将其翻译为“游客离了本地”或“游客离家出走”该区域”。
These issues could result in misinterpretations of factual matters, and cause confusion. I haven’t conducted a full-fledged test, but based on the trials I’ve done so far, both MarianMT and Google Translate appear to do better with text that’s more conversational in nature, as opposed to more formal forms of writing.
这些问题可能导致对事实问题的误解,并引起混乱。 我还没有进行全面的测试,但是根据我到目前为止所做的测试,与更正式的写作形式相比,MarianMT和Google Translate似乎在本质上更具对话性的文本上表现更好。
2C。 达世币APP (2C. DASH APP)
Plotly has released a good number of sample interactive apps for transformer-based NLP tasks, including one that works with Hugging Face’s version of MarianMT. Try it out on Colab, or via Github (I edited the app’s headline in the demo gif below).
Plotly已发布了大量用于基于变压器的NLP任务的示例交互式应用程序 ,其中包括可与Hugging Face版本的MarianMT一起使用的应用程序。 在Colab上或通过Github尝试一下(我在下面的演示gif中编辑了应用程序的标题)。

3.汉译英三种语言的翻译 (3. CHINESE-TO-ENGLISH MACHINE TRANSLATION OF 3 SPEECHES)
Machine translation of Chinese text into English tend to be a trickier task in general, as most NLP libraries/tools focus are English-based. There isn’t a straightforward equivalent of the sentence tokenizer in NLTK, for instance.
由于大多数NLP库/工具的重点都是基于英语的,因此将中文文本机器翻译成英语通常是一项棘手的任务 。 例如,NLTK中没有直接与句子标记器等效的功能。
I experimented with jieba and hanlp but didn’t get very far. As a temporary workaround, I adapted a function to split the Chinese text in the dataframe column into individual sentences prior to running them via the Chinese-to-English MarianMT model.
我尝试用解霸和hanlp但没有得到很远。 作为临时的解决方法,我修改了一个功能,以在通过中英文MarianMT模型运行它们之前,将数据框列中的中文文本拆分为单个句子。
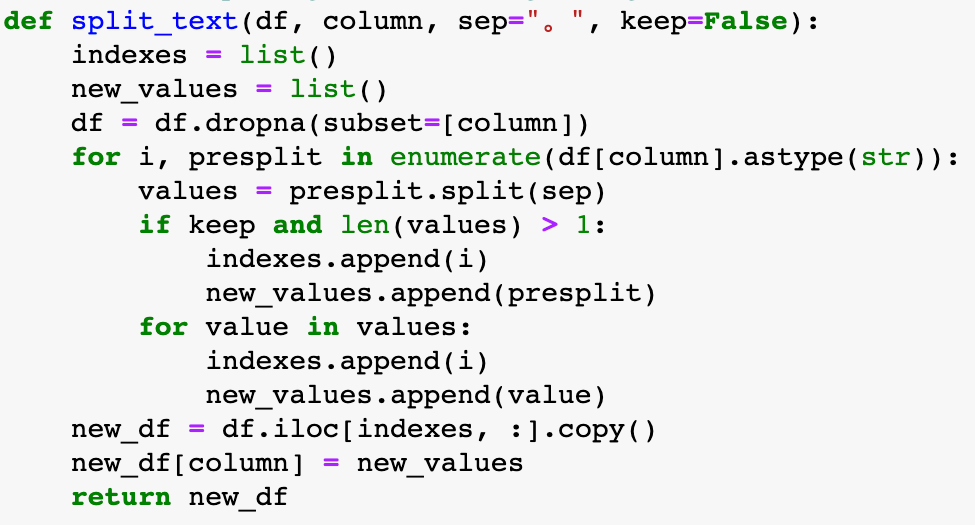
But even with the somewhat clumsy workaround, the batch translation of the three Chinese speeches took just about 5 minutes. Do note that these three speeches are the official Chinese versions of the three earlier English speeches. The two sets of speeches cover the same ground but there are some slight variations in the content of the Chinese speeches, which aren’t direct, word-for-word translations of the English versions.
但是即使有一些笨拙的解决方法,三项中文语音的批量翻译也只花了大约5分钟。 请注意,这三个演讲是之前三个英文演讲的官方中文版本。 两组演讲内容相同,但中文演讲内容略有不同,这并不是英文版本的直接逐字翻译。
You can download the output CSV file here. Let’s see how the results for the third speech compare with Google Translate:

The Google Translate version reads much better, and didn’t have the glaring error of translating “National Day” as “Fourth of July”. Overall, the results of Chinese-to-English machine translation appear (to me at least) considerably better than that for English-to-Chinese translation. But one possible reason is that the Chinese speeches in my sample were more simply written, and did not push the neural machine translation models that hard.
Google翻译版本的阅读效果更好,而且没有将“国庆日”翻译为“ 7月四日”的明显错误。 总体而言,汉英机器翻译的结果(至少对我而言)比英汉翻译的结果要好得多。 但是一个可能的原因是,我的样本中的中文语音写得更简单,并且没有使神经机器翻译模型如此困难。
4.食物的思考 (4. FOOD FOR THOUGHT)
While Hugging Face has made machine translation more accessible and easier to implement, some challenges remain. One obvious technical issue is the fine-tuning of neural translation models for specific markets.
尽管Hugging Face使机器翻译更易于访问且更易于实现,但仍存在一些挑战。 一个明显的技术问题是针对特定市场的神经翻译模型的微调 。
For instance, China, Singapore and Taiwan differ quite significantly in their usage of the written form of Chinese. Likewise, Bahasa Melayu and Bahasa Indonesia have noticeable differences even if they sound/look identical to non-speakers. Assembling the right datasets for such fine tuning won’t be a trivial task.
例如,中国,新加坡和台湾在使用中文书面形式方面有很大的不同。 同样,马来语和印尼语也有明显的不同,即使它们的发音/外观与非母语者相同。 组装合适的数据集以进行微调将不是一件容易的事。
In terms of the results achievable by the publicly available models, I would argue that the machine translated English-to-Chinese and Chinese-to-English texts aren’t ready for public publication unless a skilled translator is on hand to check and make corrections. But that’s just one use case. In situations where the translated text is part of a bigger internal workflow that does not require publication, machine translation could come in very handy.
就可以通过公开模型获得的结果而言,我认为机器翻译的英文译成中文和中文译成英文的文本尚未准备好公开发布,除非有熟练的翻译人员在手进行检查和更正。 。 但这只是一个用例。 如果翻译后的文本是不需要发布的较大内部工作流程的一部分,则机器翻译可能会非常方便。
For instance, if I’m tasked to track disinformation campaigns on social media by Chinese or Russian state actors, it just wouldn’t make sense to try to translate the torrent of tweets and FB posts manually. Batch machine translation of these short sentences or paragraphs would be far more efficient in trying to get a broad sense of the messages being peddled by the automated accounts.
例如,如果我受命由中国或俄罗斯国家行为者在社交媒体上追踪虚假信息宣传活动,那么尝试手动翻译大量推文和FB帖子就没有意义。 这些简短句子或段落的批处理机器翻译在试图获得由自动帐户兜售的消息的广泛意义上将更加有效。
Likewise, if you are tracking social media feedback for a new product or political campaign in a multi-lingual market, batch machine translation will likely be a far more efficient way of monitoring non-English comments collected from Instagram, Twitter or Facebook.
同样,如果您要在多语言市场中跟踪针对新产品或政治活动的社交媒体反馈,则批处理机器翻译可能是监视从Instagram,Twitter或Facebook收集的非英语评论的更有效的方法。
Ultimately, the growing use of machine translation will be driven by the broad decline of language skills, even in nominally multi-lingual societies like Singapore. You may scoff at some of the machine translated text above, but most working adults who have gone through Singapore’s bilingual education system are unlikely to do much better if asked to translate the text on their own.
最终,机器语言的广泛使用将受到语言技能广泛下降的推动,即使在名义上讲多种语言的社会中,例如新加坡。 您可能会嘲笑上面的一些机器翻译文本,但如果要求自己翻译文本,大多数经过新加坡双语教育系统的在职成年人不太可能做得更好。
The Github repo for this post can be found here. This is the fourth in a series on practical applications of new NLP tools. The earlier posts/notebooks focused on:
这篇文章的Github仓库可以在这里找到。 这是有关新NLP工具的实际应用的系列文章中的第四篇。 较早的帖子/笔记本着重于:
If you spot mistakes in this or any of my earlier posts, ping me at:
如果您发现此错误或我以前的任何帖子中的错误,请通过以下方式对我进行ping:
Twitter: Chua Chin Hon
Twitter: 蔡展汉
LinkedIn: www.linkedin.com/in/chuachinhon
领英(LinkedIn): www.linkedin.com/in/chuachinhon
翻译自: https://towardsdatascience.com/lost-in-machine-translation-3b05615d68e7
美剧迷失