文档词频统计
Term Frequency (TF) and Inverse Document Frequency(IDF) are the two terms which is commonly observe in Natural Language Processing techniques. It is used to find the word occurences and their contribution or impact or rather we can say importance in any given sentence of a document. This techniques are more often used in sentiment classification . The retrival of information in the form of emotions from the given word is more easier when a machine knows the significance of a word. The classification of positive and negative messages conveyed from any given sentence is generally taken care of by the above techniques. We will be following few steps in order to understand the concept in a better ways.
术语频率(TF)和逆文档频率(IDF)是在自然语言处理技术中经常观察到的两个术语。 它用于查找“出现”及其作用或影响的词,或者我们可以说它在文档的任何给定句子中的重要性。 这种技术在情感分类中更常用。 当机器知道单词的意义时,从给定单词中以情感形式检索信息会更加容易。 从任何给定句子传达的肯定和否定消息的分类通常由上述技术来处理。 我们将按照几个步骤操作,以更好地理解该概念。
Suppose we are given a huge document given below which has many sentences and want to perform text classification and conclude using the TF and IDF techniques that what is the emotion or message that is conveyed through the below sentences.
假设下面给出了一个巨大的文档,该文档包含许多句子,并且希望执行文本分类,并使用TF和IDF技术得出结论,即通过以下句子传达的情感或信息是什么。
今天早上,各小组开始练习。 由男孩组成的Kabaddi队经过了1轮练习。 男孩足球队已经开始练习。男孩板球队一直在练习。 女子排球队已经准备好。女子接力比赛队成立了。 (Today morning the teams began their practice session. The boys Kabaddi team has gone through 1 round of practice. The boys football team has started practice.The boys cricket team has been doing the practice. The girls volleyball team is ready.The boys relay race team is up .)
Step1 : Convert the sentences into bag of words
第一步:将句子转换成单词袋

This is the process of removing the stopwords like (is,are,they,them etc) which represent the pronoun or the words whose presence hardly contribute in classifying the meaning of the sentences. The next thing which we do is to perform the stemming operation on the given words which means coverting the words(which are in noun,verb ,adjective forms) to their base or root form. For e.g. consider the word training is getting converted into train verb that’s the base form. Now all these set of words which remain after performing the above cleaning process are collected in a list which represents the bag of words.
这是删除表示代词或停用词的存在的停用词的过程,这些停用词表示代词或存在不多的句子的含义。 接下来要做的是对给定的单词执行词干操作,这意味着将单词(名词,动词,形容词形式)覆盖为其基本或词根形式。 例如,考虑将单词training转换为基本形式的train动词。 现在,在执行上述清洁过程之后剩余的所有这些单词集合被收集在代表单词袋的列表中。
Bag_of_words=[‘team’ , ’boys’ , ’girls’ , ’training’ , ’kabaddi’ , ’football’ , ’cricket’ , ’volleyball’ , ’practice’ , ’round’ , ’relay’ , ’race’ , ’session’ , ‘today’ , ‘begin’ , ’go’ , ‘1’ , ‘start’ , ’ready’ , ]
Bag_of_words = ['team','boys','girls','training','kabaddi','football','cricket','排球','practice','round','relay','race' ,“会话”,“今天”,“开始”,“开始”,“ 1”,“开始”,“就绪”,]
Step 2 : Select Top frequency words
步骤2:选择最高频率字词
In the above given bag of words we take of the top 4 occuring highest frequency individual words and separate it out in the table.
在上述给定的单词包中,我们从出现频率最高的前4个单个单词中抽取出来,并在表格中将其分开。

Step 3: Calculate the Term Frequency
步骤3:计算字词频率
Term frequency is defined as the total frequency of any particular word in any given sentence .The formula of Term Frequency is defined as below :
术语频率定义为任何给定句子中任何特定单词的总频率。术语频率的公式定义如下:
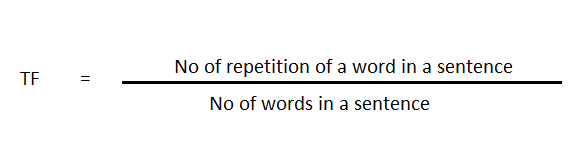
We know that is the doc we created highlighted in yellow above has total in all 5 sentences and we calculate the occurence of top 4 high frequency words in each of these sentence.
我们知道这是我们创建的文档(上面以黄色突出显示)在所有5个句子中都有总计,并且我们计算出每个句子中前4个高频词的出现。
sent 1 : Today morning teams begin practice session.
已发送1:今天上午,团队开始练习。
sent 2 : boys Kabaddi team go 1 round practice.
派出2名:男孩卡巴迪队参加1轮练习。
sent 3 : boys football team start practice.
派出3名:男孩足球队开始练习。
sent 4 : boys cricket team practice.
派出4名:男孩队练习。
sent 5 : girls volleyball team ready .
送5:女排队准备好了。
sen 6 : boys relay race team .
参6:男孩接力比赛队。
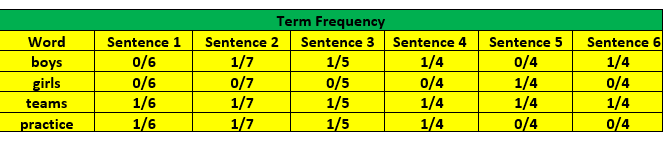
Step 4 : Calculate the Inverse Document Frequency
步骤4:计算逆文档的频率
IDF gives us the measure of occurance of any particular word across all the given sentences in a document.
IDF为我们提供了文档中所有给定句子中任何特定单词出现的度量。
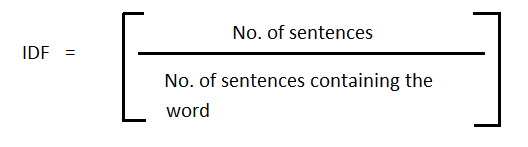

Step 5: Calculate weightage of word in a sentence
步骤5:计算句子中单词的权重
In this step we evaluate the impact of each word in a sentence by evaluating the product of each word Term Frequency in a sentence with the total IDF for the word.
在此步骤中,我们通过评估一个句子中每个单词的词频与该单词的总IDF的乘积来评估句子中每个单词的影响。

From the above table we can have below conclusions.
从上表我们可以得出以下结论。
Sentence 1 : The practice word is having more weightage indicating that the college is putting efforts in practice session.
句子1:练习字的权重更大,表明学院正在努力进行练习。
Sentence 2 : The boys team is preparing for the game
句子2:男孩队正在为比赛做准备
Sentence 3 : The boys team is preparing for the game
句子3:男孩队正在为比赛做准备
Sentence 4 : The boys team is practicing for game
句子4:男孩队正在练习比赛
Sentence 5 : The girls team is practicing for game
句子5:女子团体正在练习比赛
Sentence 6 : Boys team is practicing hard for game.
句子6:男孩队正在努力练习比赛。
And by calculating the total weightage for each of the words in the entire document it can be observed that the word boy has more weightage compared to others. Hence we can conclude that the college is focusing more on encouraging boys to compete in the upcoming competition.
并且通过计算整个文档中每个单词的总权重,可以看出男孩这个单词比其他单词具有更大的权重。 因此,我们可以得出结论,该大学将更多的精力放在鼓励男孩参加即将到来的比赛中。
In this way the TF and IDF helped us to identify the contribution of words in individual sentences. Also we could be able to identify that the college is focussing on which area more from the given document.
通过这种方式,TF和IDF帮助我们确定了单个句子中单词的作用。 我们还可以从给定的文档中识别出该大学正在将重点放在哪个领域上。
Hope this example helps you to understand things better!!
希望这个例子可以帮助您更好地理解!!
Thanks for reading :)
谢谢阅读 :)
文档词频统计