冒烟测试和随机测试
Disclosure: I am not a health scientist. The information included in this article comes from an analysis that has not been peer-reviewed nor supervised by a health scientist. Please refer to more accurate and validated resources if you are looking for clear answers on Covid-19.
披露:我不是健康科学家。 本文中包含的信息来自未经卫生科学家同行评审或监督的分析。 如果您在Covid-19上寻求明确的答案,请参考更准确和经过验证的资源。
We all remember the WHO’s statement at the beginning of the pandemic: “Test, test, and test”. A clear strategy that many countries have adopted, including Italy. After China, Italy has been the first country to experience the rapid spread of Covid-19 but also the first one to recover from a portrayed scene of Dante’s Inferno.
我们都记得世卫组织在大流行开始时的声明:“测试,测试和测试”。 许多国家(包括意大利)已采用了一项明确的战略。 仅次于中国,意大利是第一个经历Covid-19Swift传播的国家,也是第一个从但丁的地狱照片中复苏的国家。
Everything happened so fast that hospitals and labs were not ready to handle thousands of help requests. Hence, the Italian Health Organization decided to proceed with tests only for people with critical symptoms. This decision has been severely criticized by many doctors like Andrea Crisanti, who, while conducting research in a small community, discovered the danger of asymptomatic transmission (2020). According to his study, a good amount of people without symptoms tested positive for Coronavirus and possibly transmitted the disease to other fellows.
一切发生得如此之快,以至于医院和实验室还没有准备好处理成千上万的帮助请求。 因此,意大利卫生组织决定只对有严重症状的人进行检查。 像安德里亚·克里斯蒂(Andrea Crisanti)这样的许多医生严厉批评了这一决定,他们在一个小社区进行研究时发现了无症状传播的危险(2020年)。 根据他的研究,大量无症状的人冠状病毒检测呈阳性,并可能将疾病传播给其他人。
As time passed, labs became fully equipped to process several thousands of tests daily. As of today, September 18th 2020, Italy is processing more than 100.000 tests per day, testing symptomatic and asymptomatic citizens randomly. Now, more than ever, Italian citizens can get a free test in a few hours and know if they are positive or negative. Is this, however, a good strategy to fight the pandemic?
随着时间的流逝,实验室变得设备齐全,每天可以处理数千个测试。 截至今天,即2020年9月18日,意大利每天处理超过100.000次检测,随机检测有症状和无症状的公民。 现在,意大利公民比以往任何时候都可以在几个小时内获得免费测试,并知道他们是正面还是负面。 但是,这是与大流行作斗争的好策略吗?
We should not forget that tests are not 100% accurate and we should take a Bayesian approach into consideration to understand whether massive testing is increasing the chance of isolating infected patients. Indeed, when determining a test result, we should take into account the probability that the person is infected. When very few people are infected, massive testing, without repeated testing, could become useless to fight the pandemic.
我们不要忘记测试不是100%准确的,我们应该考虑贝叶斯方法,以了解大规模测试是否会增加隔离感染患者的机会。 确实,在确定测试结果时,我们应该考虑该人被感染的可能性。 当很少的人被感染时,未经反复测试的大规模测试可能对抵抗大流行毫无用处。
数据集 (Dataset)
For this analysis, we will consider the public dataset of the Italian Civil Protection (Protezione Civile). The dataset is updated daily and it contains information on tests, positive cases, intensive care beds, hospitalization rates, and other important figures since the 24th of February 2020.
对于此分析,我们将考虑意大利民事保护(Protezione Civile)的公共数据集。 该数据集每天更新一次,其中包含有关2020年2月24日以来的检查,阳性病例,重症监护病床,住院率以及其他重要数据的信息。
贝叶斯定理简介 (Introduction to Bayes’ Theorem)
According to Bayes’ Theorem, before determining the probability of an event (like a virus test), we need to take into consideration our prior knowledge on the conditions that might affect this event. In the case of random massive testing, we need to consider 3 important figures:
根据贝叶斯定理,在确定事件的可能性(如病毒测试)之前,我们需要考虑可能影响该事件的条件的先验知识。 在随机大规模测试的情况下,我们需要考虑3个重要数据:
- The probability that the person has the virus 人感染病毒的可能性
- The true positive rate (when a person who has the virus is tested positive), also known as the sensitivity of a test真实阳性率(当一个人被检测为阳性时),也称为检测的敏感性
- The true negative rate (when a person who does not have the virus is tested negative), also known as the specificity of a test真实阴性率(当没有病毒的人被检测为阴性时),也称为检测的特异性
Why? Since many tests are not 100% reliable, the probability that a test is positive or negative is conditional to the reliability of the test, but also to the probability that the tested person has the virus. Indeed, the more are the people who are not affected by the virus, the higher the probability that the test will give a false positive.
为什么? 由于许多测试并非100%可靠,因此测试为阳性或阴性的可能性取决于测试的可靠性,但也取决于被测试者感染病毒的可能性。 事实上,更多的是谁,不会受病毒感染的人,高的概率试验将给假阳性。
Once we have these figures, the calculation becomes very easy. By following Bayes’ formula, we need to perform the following calculation to find the probability that a person who tested positive for the test is truly positive:
一旦有了这些数字,计算就变得非常容易。 通过遵循贝叶斯公式,我们需要执行以下计算,以发现测试阳性的人确实是阳性的概率:
Probability that the person has the virus * True positive rate / {(Probability that the person has the virus * True positive rate) + (Probability that the person does not have the virus * False positive rate)}
人感染病毒的概率*真阳性率/ {(人感染病毒的概率*真阳性率)+(人没有病毒的概率*假阳性率)}
and this formula to find the probability that a person who tested negative is truly negative:
和这个公式来找出一个测试阴性的人确实是阴性的概率:
Probability that the person does not have the virus * True negative rate / {(Probability that the person does not have the virus * True negative rate) + (Probability that the person has the virus * False negative rate)}
人没有病毒的概率*真阴性率/ {(人没有病毒的概率*真阴性率)+(人有病毒的概率*假阴性率)}
Let’s start the analysis.
让我们开始分析。
感染者的奥秘 (The mystery of the infected)
It is very hard to know how many people had Covid-19 in the first months of the pandemic. Some studies, conducted in different parts of the world, revealed that the number of infected people could be 10 times higher than the number of positive tests due to the impossibility of massive testing (New York Times, 2020). We will consider this hypothesis as true for our analysis and I will show later why, but first, we need to look at the real numbers, which have been collected by hospitals.
大流行的头几个月,很难知道有多少人患有Covid-19。 在世界各地进行的一些研究表明,由于不可能进行大规模检测,被感染的人数可能比阳性检测的人数高10倍(《纽约时报》,2020年)。 对于我们的分析,我们将认为该假设是正确的,稍后将说明原因,但首先,我们需要查看医院收集的实际数字。
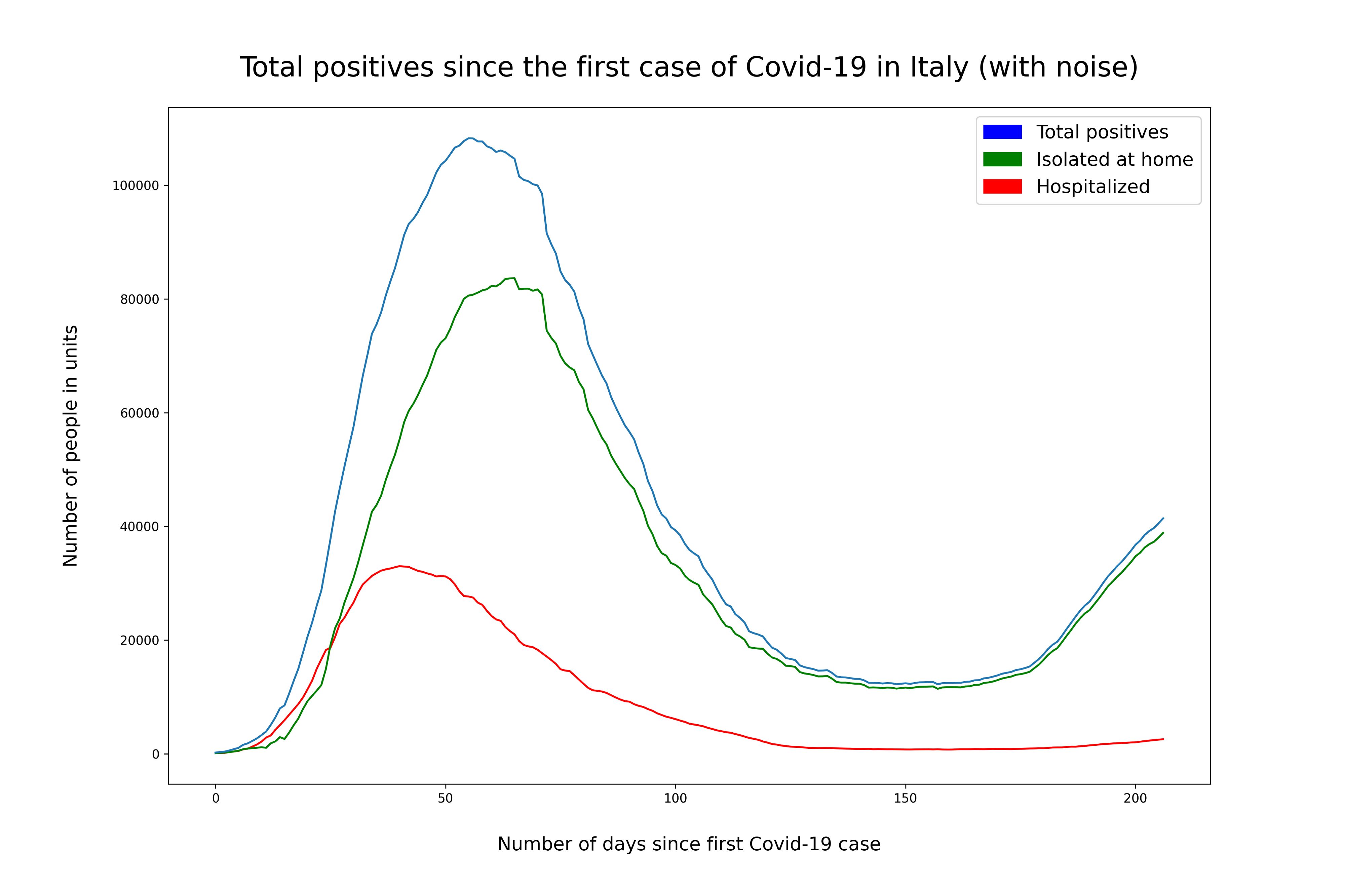
As you can see from the plot above, the blue and the green curves follow the same distribution and almost converge at the end, while the red curve looks flatter, without second peaks at the end. This means that, at the moment, many people are testing positive for Covid-19, but are not being hospitalized because they are either asymptomatic or because their symptoms are not severe.
从上面的图中可以看出,蓝色和绿色曲线遵循相同的分布,并且几乎在末端收敛,而红色曲线看起来较为平坦,末端没有第二个峰值。 这意味着,目前,许多人的Covid-19检测呈阳性,但由于没有症状或症状不严重而没有住院。
正数与测试比率 (Positive vs tested ratio)
As the number of tests increased over time, we need to understand the percentage of how many people resulted positive when tested.
随着测试数量随时间增加,我们需要了解有多少人在测试时呈阳性的百分比。
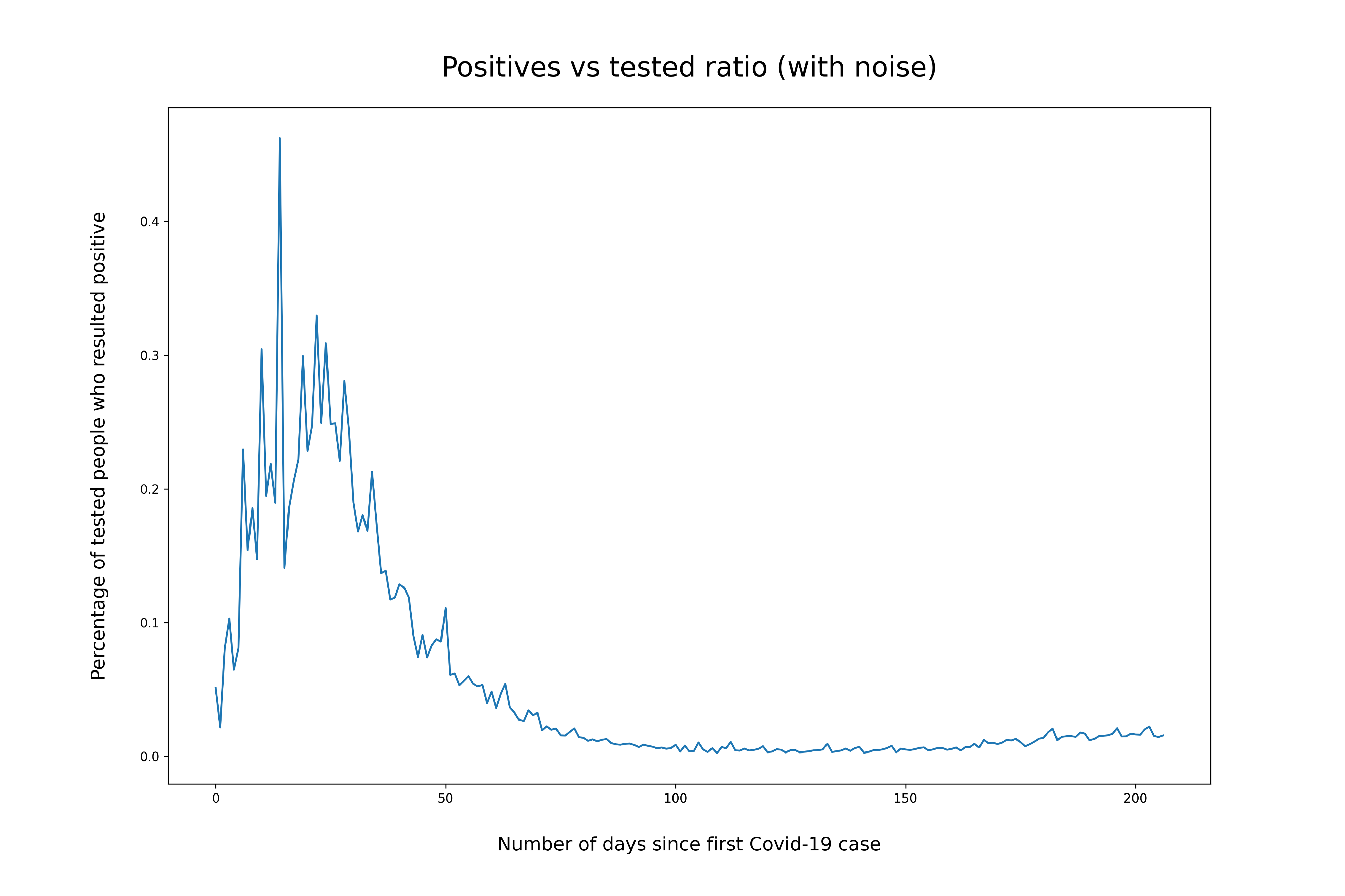
As we can see from the plot above, during the peak of the pandemic, almost 30% of tested people were resulting positive for Covid-19. Today, only 2% of tested people result positive. This means that today it is quite hard to find a positive test.
从上图可以看出,在大流行高峰期间,将近30%的受测人群Covid-19呈阳性。 如今,只有2%的受测者获得了阳性结果。 这意味着今天很难找到一个积极的测试。
找到我们的3个数字 (Finding our 3 figures)
Now that we have a clear understanding of the numbers, we need to find our 3 main figures to apply Bayes’ Theorem. We have the number of positive tests, which we are going to multiply by 10, and we need the true positive rate and false positive rate. There are different opinions on these numbers, so we will consider Harvard’s guidelines (Shmerling, 2020) which propose a true positive rate of 95% and a true negative rate of 70–98%. For the second rate, we will use 70% to show that if there are many non-infected people, the probability of testing negative is not influenced by a higher specificity rate.
现在我们对数字有了清楚的了解,我们需要找到3个主要数字来应用贝叶斯定理。 我们有阳性测试的数量,我们要乘以10,我们需要真实的阳性率和错误的阳性率。 关于这些数字有不同的意见,因此我们将考虑哈佛大学的指导原则(Shmerling,2020年),该指南提出了95%的真实阳性率和70-98%的真实阴性率。 对于第二个比率,我们将使用70%来表明,如果有许多未感染的人,则阴性React的可能性不受较高特异性比率的影响。
测试呈阳性时呈阳性的可能性 (Probability of being positive when testing positive)
By following Bayes’ Theorem, random massive testing with a low number of infected people can give very ambiguous results.
通过遵循贝叶斯定理,对少量感染者进行随机大规模测试可以得出非常模糊的结果。
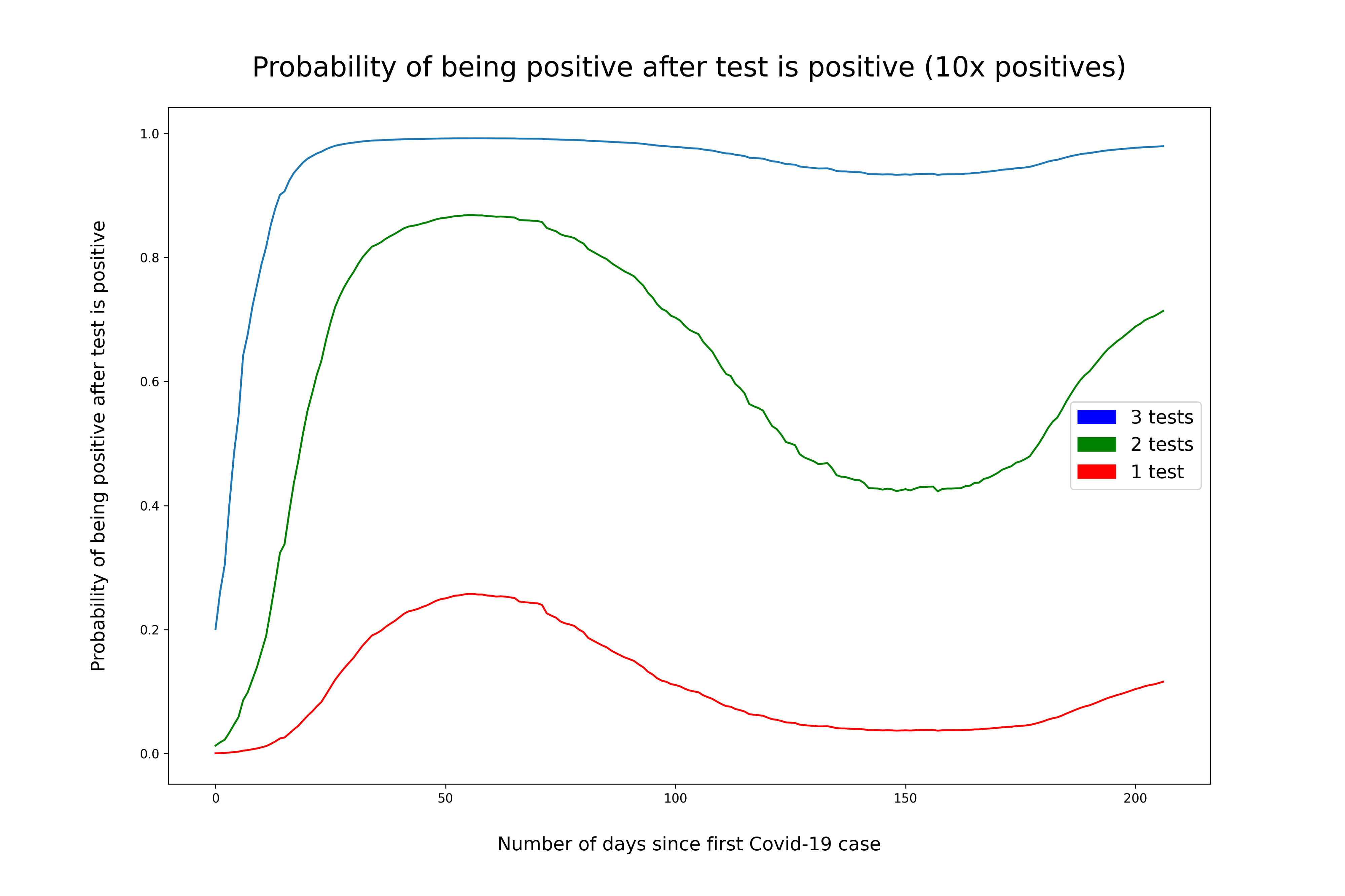
As we can see from the plot above, the probability of being positive after one positive test is significantly low. During the peak of the pandemic, the probability went up to around 25%, but we have considered a number of infected people 10 times higher than the number of people who tested positive. Today, the probability is around 15%. What happens if we consider the number of infected people as the number of people who tested positive (without 10x multiplication)?
从上图可以看出,一项阳性测试后成为阳性的概率非常低。 在大流行高峰期间,这种可能性上升到25%左右,但我们认为受感染的人数是检测为阳性的人数的10倍。 如今,可能性约为15%。 如果我们将被感染的人数视为检测为阳性的人数(没有10倍乘法),会发生什么?
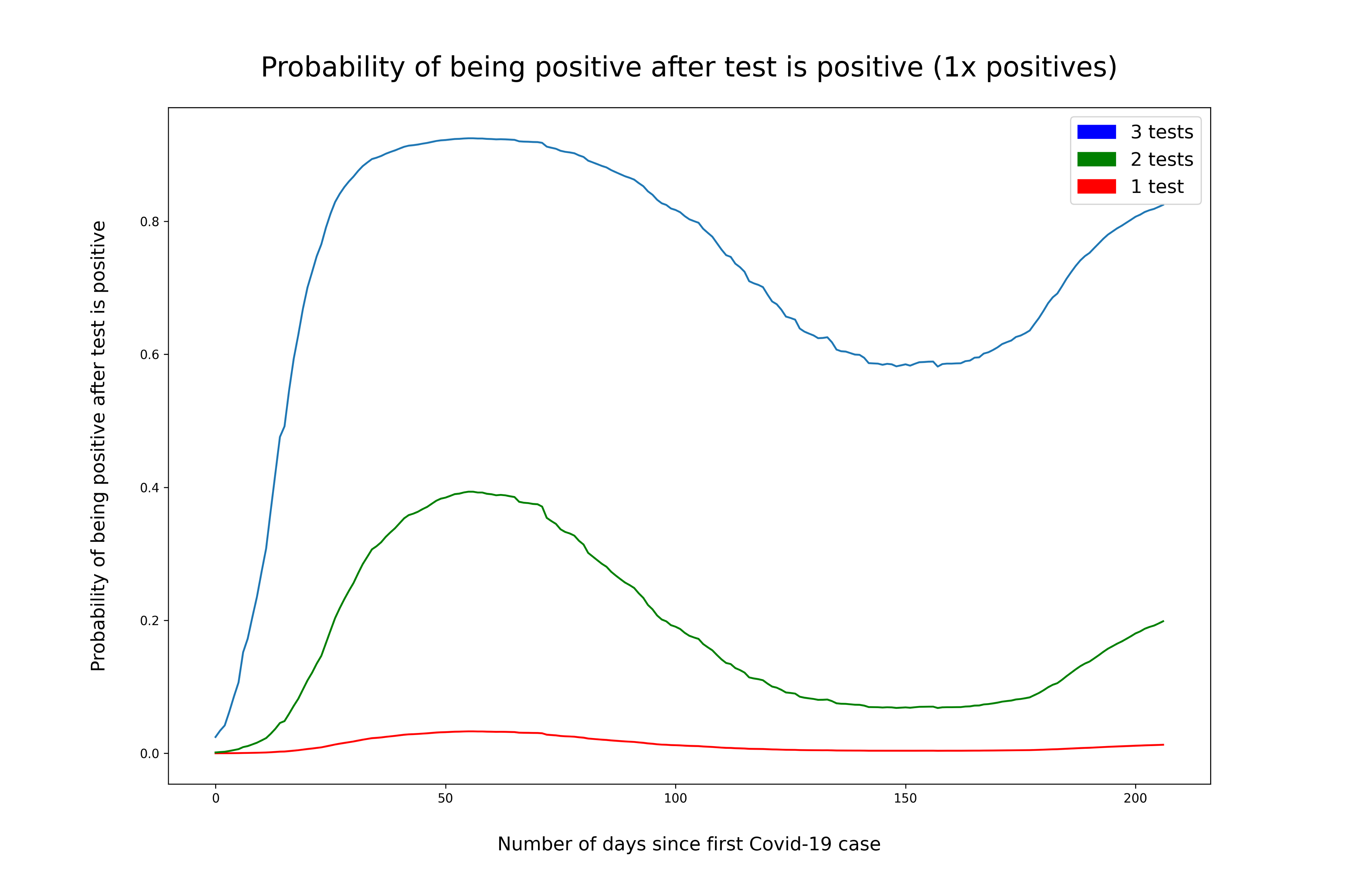
The probability of being positive after one positive test is close to 0. The good news is that there is a solution to this problem and it is called repeated testing. By repeating the test 2 or 3 times and updating the formula with the new probability of being infected, we can reach a high level of certainty. A third positive test tells us that we are certainly positive with a probability of at least 80% (considering today’s numbers). Hence, random one-time testing may be completely useless without repeated testing because the confidence level of the test is too low.
在一项正面测试之后成为正面的概率接近于0。好消息是,这个问题有解决方案,这被称为重复测试。 通过重复测试2或3次并以新的被感染概率更新公式,我们可以达到很高的确定性。 第三次阳性测试告诉我们,我们肯定是肯定的,至少有80%的概率(考虑到今天的数字)。 因此,由于测试的置信度太低,因此如果不进行重复测试,则随机的一次性测试可能完全没有用。
测试阴性时出现阴性的可能性 (Probability of being negative when testing negative)
Now that you have seen the previous plots, what do you expect to see for the probability of being negative after a negative test? Yes, a very different outlook. As the probability of being non-infected is much higher than the probability of being infected, the resulting probability of being negative after a negative test is very high.
既然您已经看到了前面的图,那么在阴性测试之后您希望看到阴性的可能性是什么? 是的,前景截然不同。 由于未被感染的可能性比被感染的可能性高得多,因此在阴性测试后产生阴性的可能性非常高。
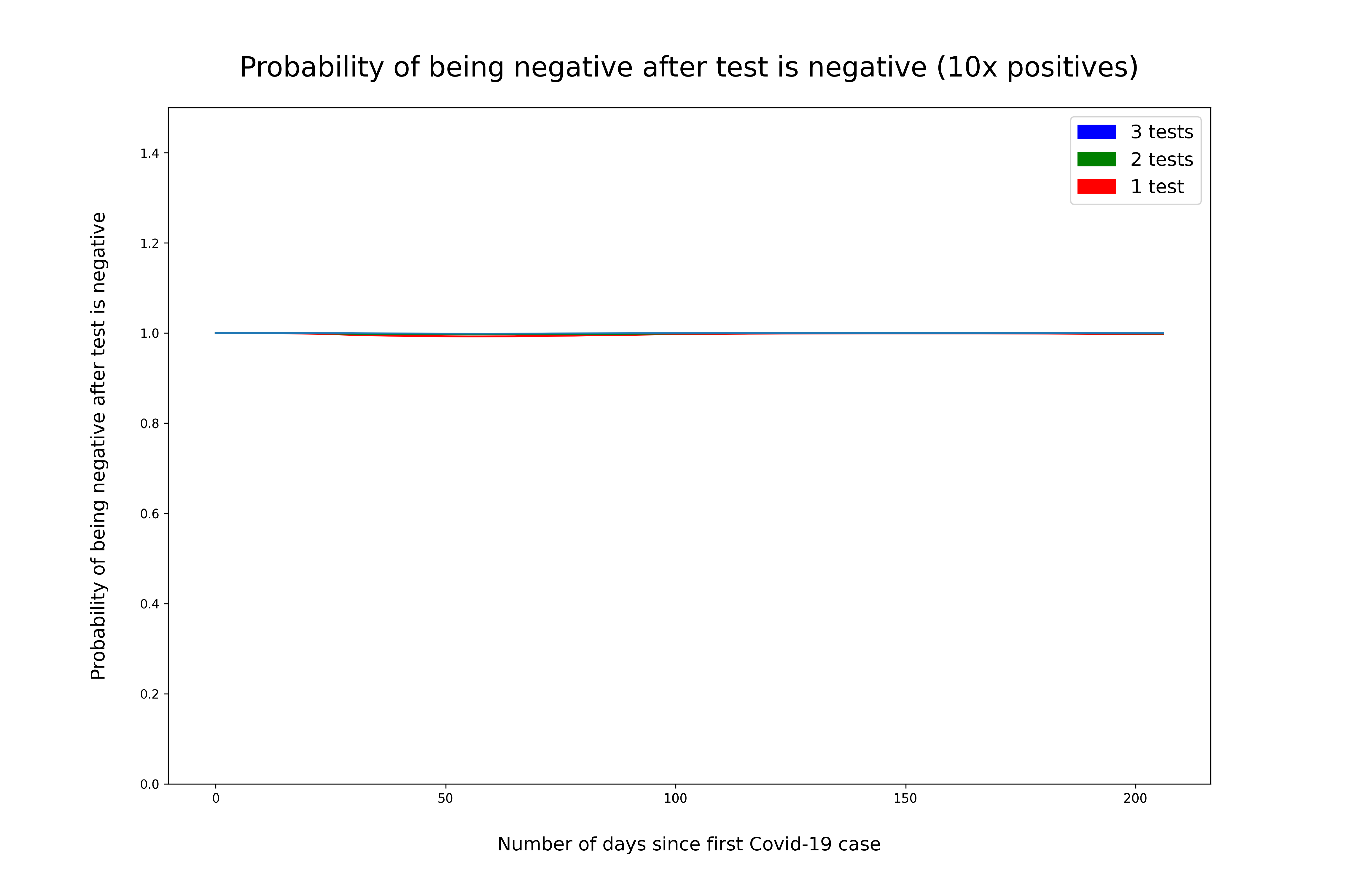
As you can see from the plot above, doing one, two or three tests does not change the probability of being a true negative.
从上图中可以看到,进行一次,两次或三个测试不会改变成为真实阴性的可能性。
如何验证感染者的真实人数并决定要执行多少次重复测试 (How to verify the real number of infected people and decide how many repeated tests to perform)
As mentioned before, studies have hypothesized that the real number of infected people may be 10 times higher than the number of positive tests due to the impossibility of massive testing. If we consider that many tests are performed just one time, we can try to assume the real number of infected people by creating the same Bayesian model and comparing the probability of being positive (when testing positive) to the positive/tested ratio.
如前所述,研究假设由于无法进行大规模检测,实际感染人数可能比阳性检测人数高10倍。 如果我们认为一次只进行了多次测试,则可以通过创建相同的贝叶斯模型并比较阳性(当测试为阳性时)的概率与阳性/测试比率来假定感染者的真实人数。
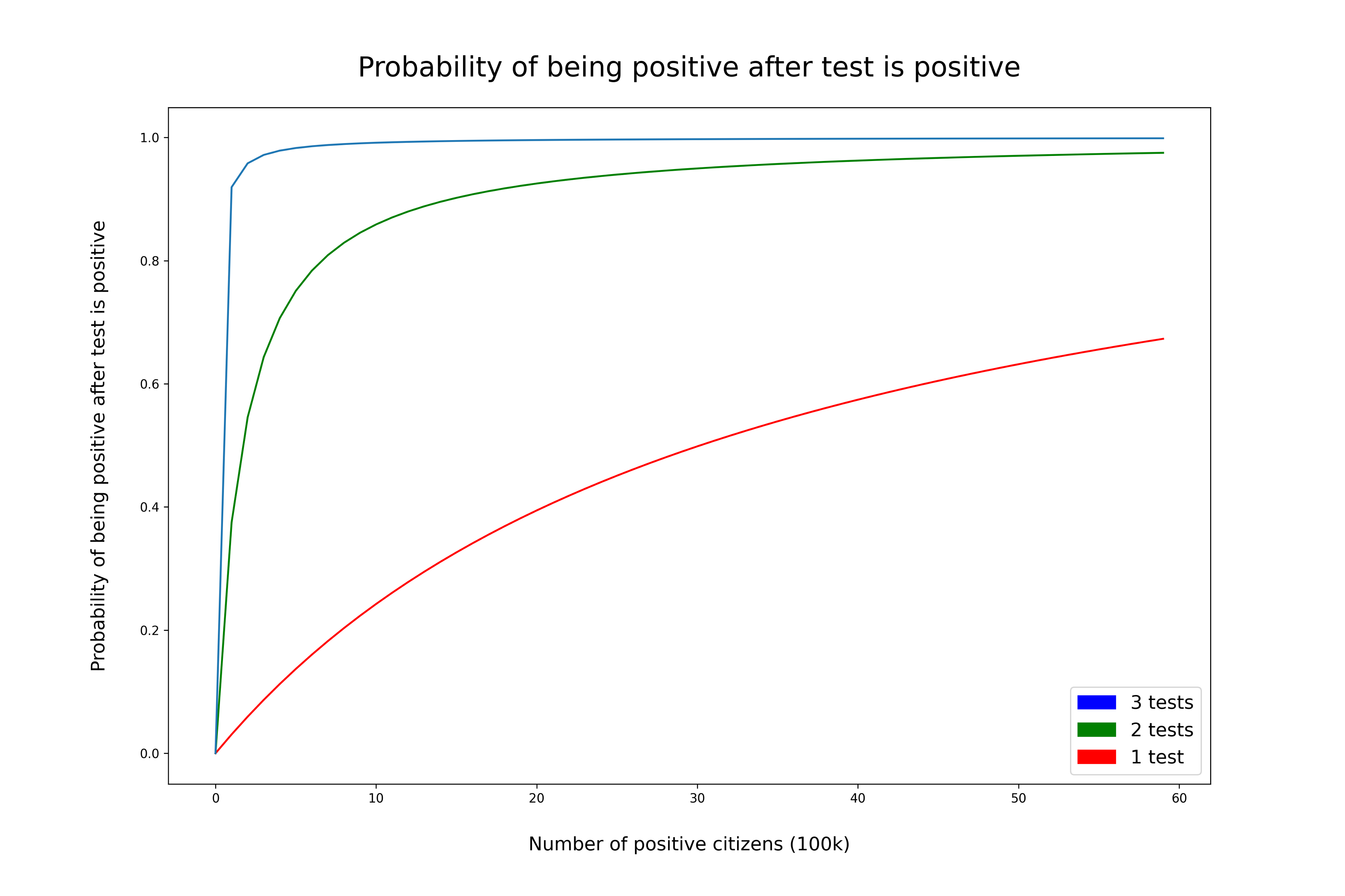
The plot above was created with the same model we have used for the previous analyses. In this case, we have a sequence of 6.000.000 numbers because we would like to calculate the probability of being positive after testing positive assuming that infected people could be at most 6 million (10% of Italy’s population).
上面的图是使用与先前分析相同的模型创建的。 在这种情况下,我们有一个6.000.000的数字序列,因为我们想在假设阳性感染者最多为600万人(占意大利人口的10%)后测试阳性后计算阳性的可能性。
For example, if there are 1.000.000 infected people, the probability of being positive after one positive test is 0.2 or 20%. Now, if we go back to the positive/tested ratio’s plot, we can see that at the peak of the pandemic the percentage of people who tested positive was around 30%. By looking at the plot above, the probability of being positive after testing positive reaches 30% when there are at least 1.300.000 infected people. During the peak, the number of confirmed positive cases was around 80.000. Considering that many people could not be tested at the time so the number of positive cases could have been higher, we can assume that the real number of infected people may have been 10 times higher.
例如,如果有1.000.000感染者,则在一项阳性测试后被阳性的可能性为0.2或20%。 现在,如果我们回到阳性/检验比率的图,可以看到在大流行高峰时,检验阳性的人的百分比约为30%。 通过查看上面的图,当存在至少1.300.000感染者时,在测试阳性后呈阳性的可能性达到30%。 在高峰期,确诊阳性病例的数量约为80.000。 考虑到当时无法对许多人进行检测,因此阳性病例的数量可能会更高,我们可以假设实际感染者的数量可能高出10倍。
As we saw in the previous analyses, one random test is not enough to assure that a person is truly infected. However, we can see how a second test gives a very high certainty after a threshold of around 500.000 infected people, while a third test gives certainty even with a very small amount of infected people. In case you wonder how the probability of being negative after testing negative changes with the number of infected people, the plot below answers your doubts.
正如我们在之前的分析中所看到的,仅仅进行一次随机测试不足以确保一个人真正受到感染。 但是,我们可以看到在大约50万感染者的阈值之后,第二次测试如何给出很高的确定性,而即使只有很少量的感染者,第三次测试也可以给出确定性。 如果您想知道在测试负面影响后随着感染者数量的变化而成为阴性的可能性的话,下面的图表将回答您的疑问。
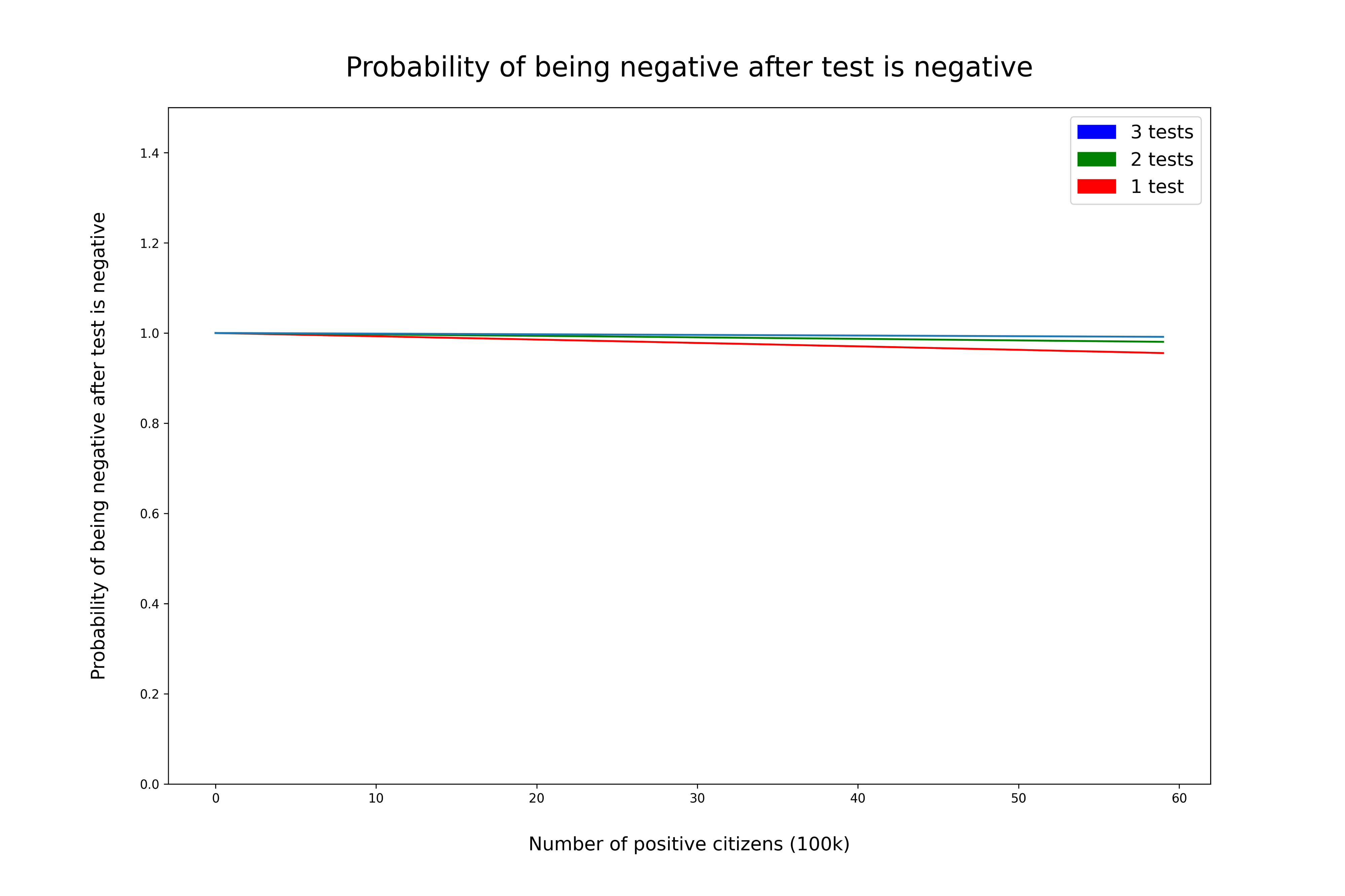
误报的危险 (The danger of false negatives)
Now, imagine for a moment that we are not calculating probabilities of being negative based on random testing, but on targeted testing. For example, a symptomatic patient gets tested by his doctor, who, based on the symptoms, sets the probability of being negative at 0.2 (0.8 probability of being positive).
现在,想象一下,我们不是基于随机测试来计算否定概率,而是基于目标测试。 例如,有症状的患者由其医生检查,该医生根据症状将阴性概率设置为0.2(阳性概率为0.8)。
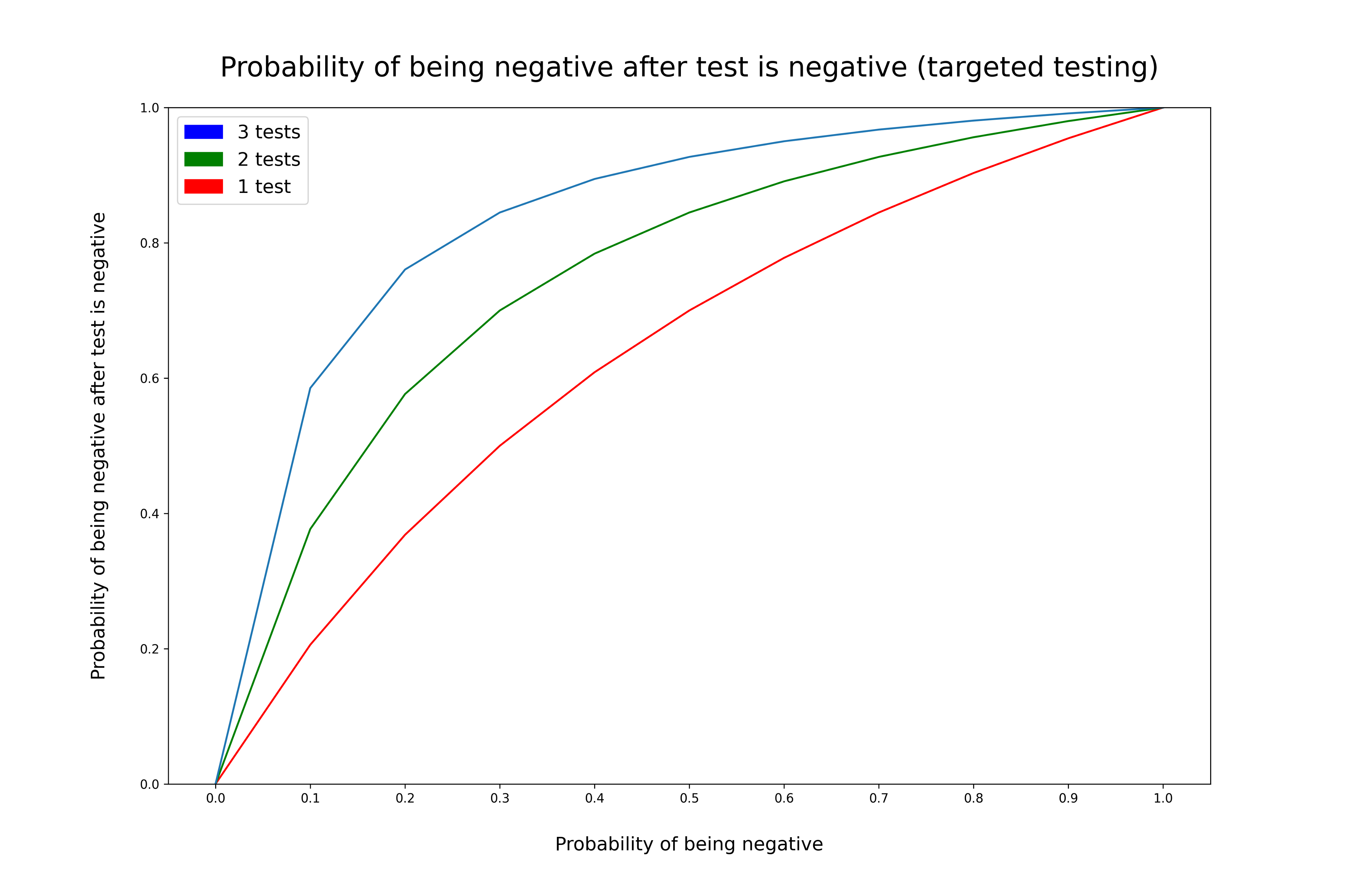
As the conditional probability is set by the doctor and not by the probability of being negative based on the whole population, the probability of being negative after one test becomes at most 0.4. Is this enough? Considering that having a false negative person going around the streets is much worse than having a false positive person staying at home, one test is not enough. According to the model, three tests should be the most indicated measure when testing symptomatic patients.
由于条件概率是由医生确定的,而不是由整个人口为阴性的概率来确定的,因此一项检查后成为阴性的概率最多为0.4。 这够了吗? 考虑到有一个假阴性的人到街上走要比让一个假阳性的人待在家里差很多,一个测试是不够的。 根据该模型,对有症状患者进行检查时,最有指征的措施应为三项检查。
结论 (Conclusion)
Despite governments are turning their attention to random massive testing, this may not be the most efficient strategy to tackle the pandemic. As shown above, random one-time testing does not provide enough certainty to declare if a person is truly positive, causing a high rate of false positives. This issue may be prevented with targeted and repeated testing, which may be seen as a more efficient method to increase the conditional probability of being positive.
尽管政府将注意力转向了随机大规模测试,但这可能不是解决大流行病的最有效策略。 如上所示,随机的一次性测试不能提供足够的确定性来宣布一个人是否真正为阳性,从而导致很高的误报率。 可以通过有针对性的重复测试来避免此问题,这可以看作是一种提高条件的可能性为肯定的更有效的方法。
While the WHO’s recommendation is to “test, test, and test”, a better recommendation would be “test 1, test 2, and test 3”. Indeed, a third test, updated with the previous tests’ probability of being positive, confirms the presence of a positive case with high certainty. The opposite holds for the probability of being negative after one negative test. When the rate of infected patients is low, one negative test confirms the presence of a negative case with probability close to 1. However, when testing symptomatic patients, the conditional probability of being negative should be updated in order to avoid false negatives.
世卫组织的建议是“测试,测试和测试”,而更好的建议是“测试1,测试2和测试3”。 确实,第三项测试以先前测试为阳性的可能性进行了更新,可以高度肯定地确认存在阳性病例。 在一次阴性测试后阴性的可能性相反。 当感染患者的比率较低时,一项阴性试验会确认存在阴性病例的可能性接近1。但是,在测试有症状患者时,应更新条件阴性的可能性,以避免假阴性。
Crisanti A. et al., Suppression of COVID-19 outbreak in the municipality of Vo, Italy (2020), Medrxiv The Preprint Server for Health Sciences.
Crisanti A.等人,《意大利Vo镇的COVID-19爆发抑制》(2020年) ,Medrxiv健康科学预印服务器。
Mandavilli A., Actual Coronavirus Infections Vastly Undercounted, C.D.C. Data Shows (2020), New York Times
Mandavilli A.,《实际冠状病毒感染被严重低估》,CDC数据显示(2020年) ,《纽约时报》
Shmerling R. H., Which test is best for COVID-19? (2020), Harvard Health Publishing.
呼吸RH,哪种测试最适合COVID-19? (2020) ,哈佛健康出版社。
Watson J., Interpreting a Covid-19 Test Result (2020), The BMJ.
Watson J.,解释Covid-19测试结果(2020) ,BMJ。
Woloshin S. et al., False Negative Tests for SARS-CoV-2 Infection (2020), The New England Journal of Medicine.
Woloshin S.等人, 《 SARS-CoV-2感染的假阴性试验》(2020年) ,《新英格兰医学杂志》。
翻译自: https://medium.com/illumination/should-we-randomly-test-everybody-for-covid-19-84ce0659a18c
冒烟测试和随机测试