分类评估指标有哪些
Evaluation metric refers to a measure that we use to evaluate different models. Choosing an appropriate evaluation metric is a decision problem that requires a thorough understanding of the goal of a project and is a fundamental step before all modeling process that follows. So why is it so important, and how should we choose?
评估指标是指我们用来评估不同模型的度量。 选择适当的评估指标是一个决策问题,需要对项目目标有透彻的了解,并且是随后进行所有建模过程的基本步骤。 那么为什么它如此重要,我们应该如何选择呢?
它为什么如此重要? (Why is it important?)
Let’s say you live in a city where it is sunny about 300 days a year. During the other 65 days, it gets very uncomfortable with heavy rain or snow. In this imaginary world, there are only two weather apps. One app predicts the weather correctly 80% of the time, while the other one is right about 70% of the time. Which app would you choose?
假设您居住在一个每年约300天阳光明媚的城市。 在其他65天中,大雨或大雪会使人非常不舒服。 在这个虚构的世界中,只有两个天气应用程序。 一个应用程序有80%的时间可以正确预测天气,而另一个应用程序有70%的时间可以正确预测天气。 您会选择哪个应用程序?
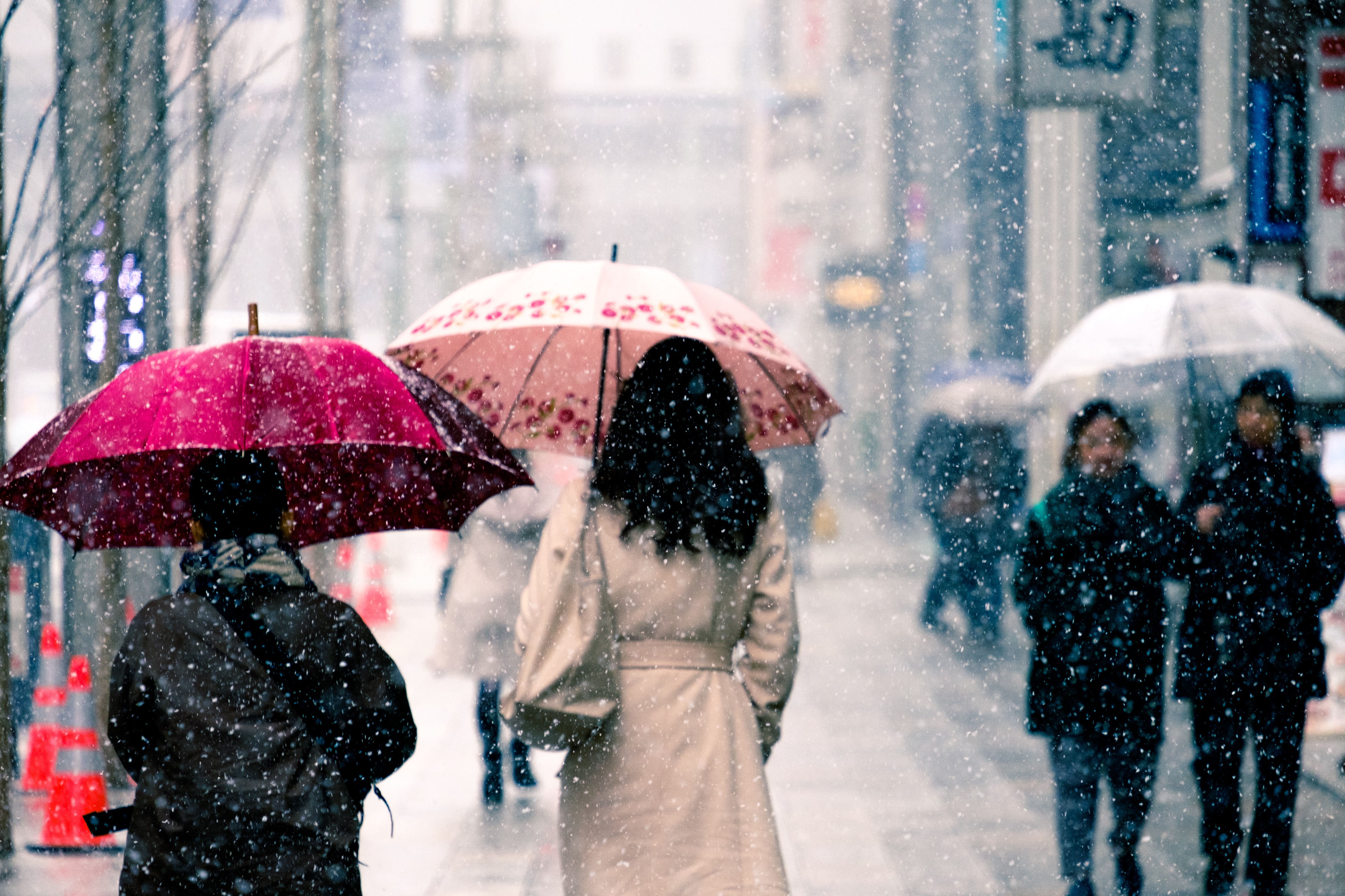
If an app always predicts sunny in this city, this app will be 80% correct, even though it never predicts bad weather accurately. In this case, this app is useless! On the other hand, the other app might fail to predict the sunny weather about 30% of the time, but it will tell you about bad weather accurately over 80% of the time. In this case, this app that correctly predicts the weather 70% of the time is much more useful than the 80% app.
如果某个应用始终预测该城市晴天,那么即使该应用永远无法准确预测恶劣的天气,该应用也将达到80%的正确率。 在这种情况下,此应用程序无用! 另一方面,另一个应用程序可能无法在大约30%的时间中预测晴天,但是在80%的时间内它会准确地告诉您有关恶劣天气的信息。 在这种情况下,此应用程序可以在70%的时间内正确预测天气,比80%的应用程序有用得多。
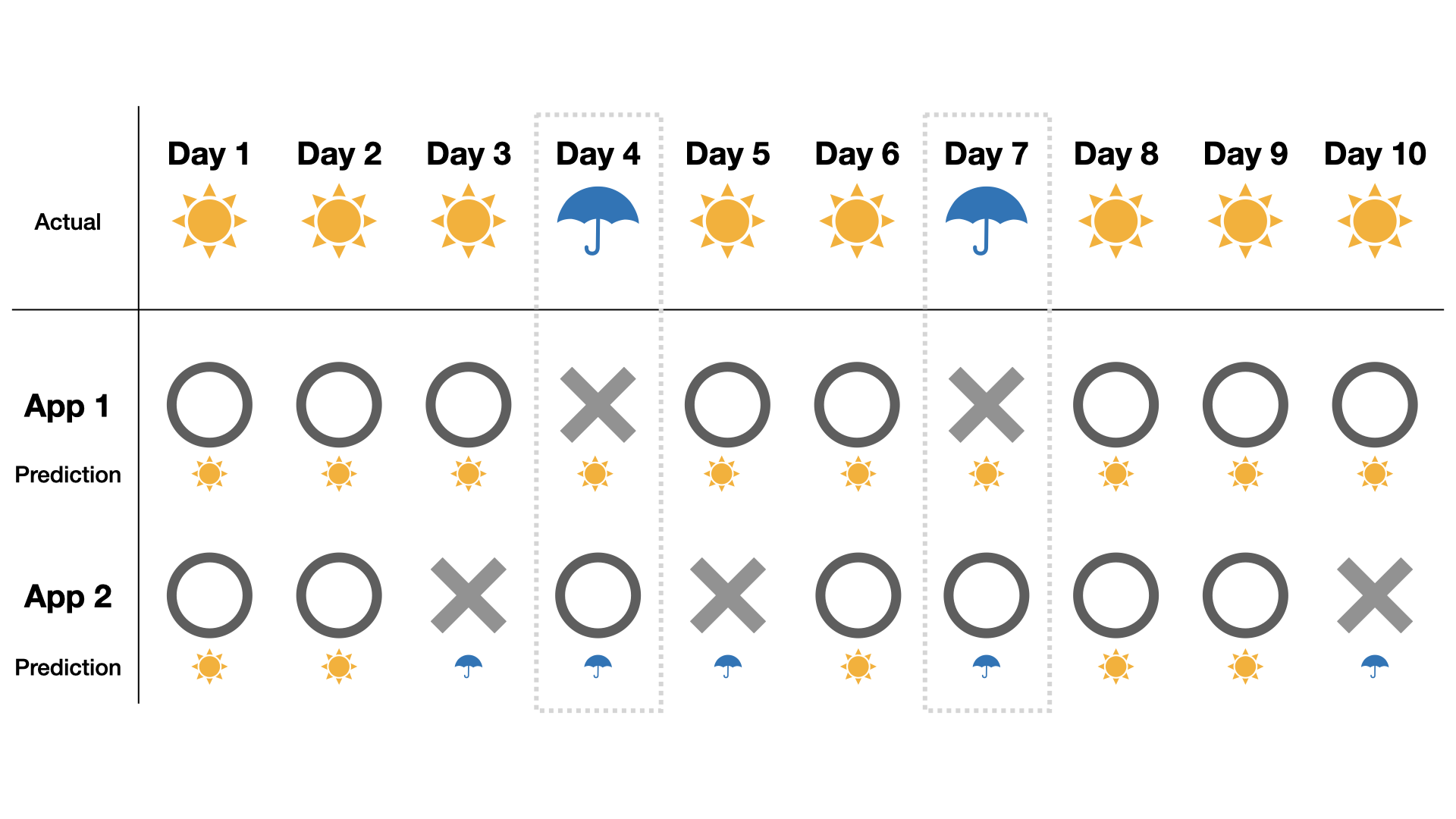
As we can see here, how we choose to evaluate our model can tell vastly different information about our data. It must be determined by the goal of the project and the properties of the dataset. No data will be without its variance, and no model can explain the world perfectly. So we must know where we would rather have such prediction errors to happen.
正如我们在这里看到的,我们如何选择评估模型可以告诉我们有关数据的极大不同信息。 它必须由项目的目标和数据集的属性确定。 没有数据就没有其变异性,没有任何模型可以完美地解释世界。 因此,我们必须知道在哪里发生此类预测错误。
是非题,正面或负面 (True or False, Positive or Negative)
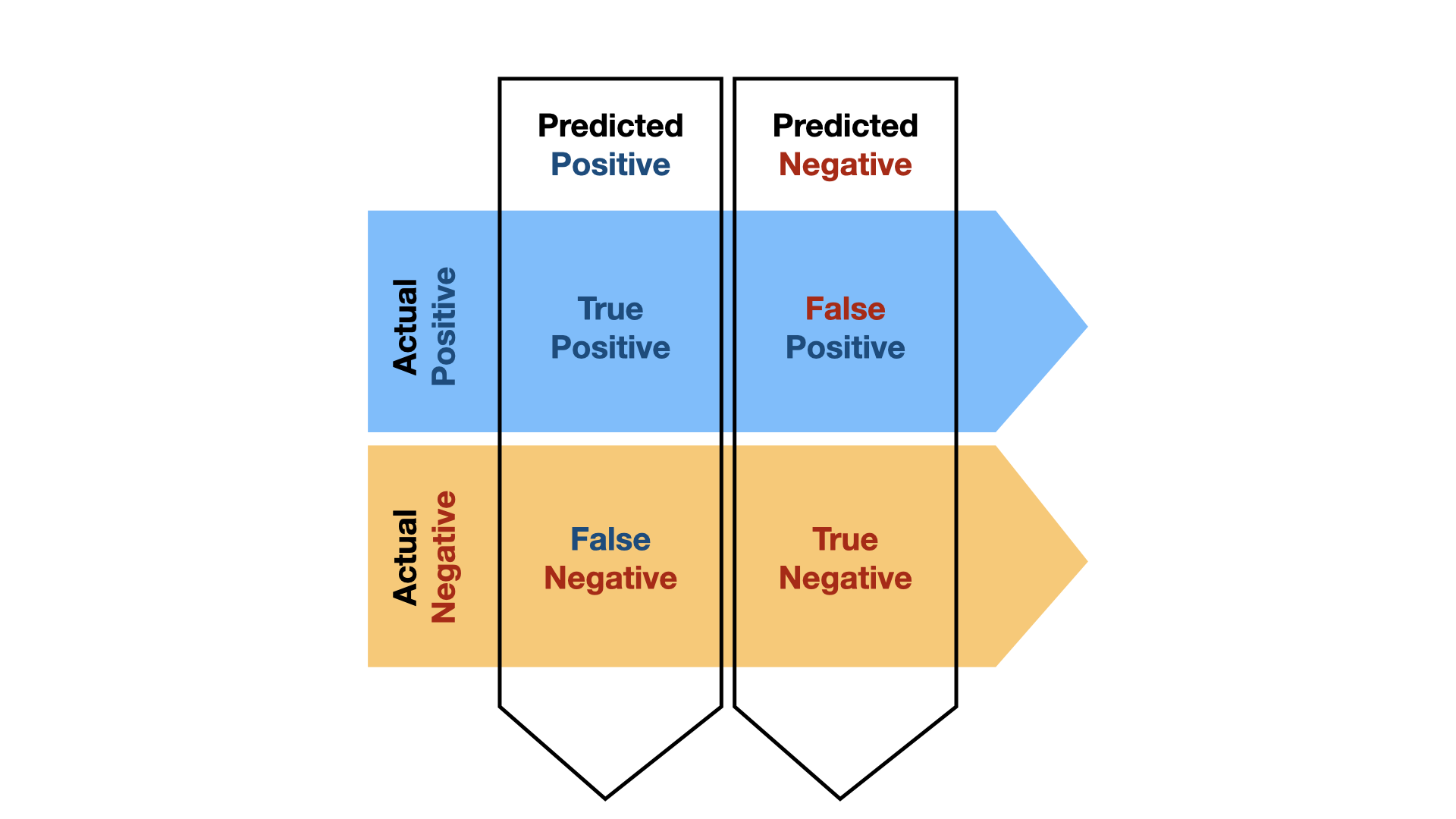
When a model makes a binary prediction, there are 4 possible outcomes. A model can predict yes (positive) or no (negative), and it can either be correct (true) or wrong (false). The same logic applies when a model is making a multi-class prediction. The model predicts yes or no for an individual class, and it can be right or it can be wrong, and we decide how to combine these individual outcomes.
当模型进行二进制预测时,有4种可能的结果。 模型可以预测是( 正 )或否( 负 ),并且可以是正确的( tr