科研图像压缩算法比例,雷娜

抽筋 (Swiping cramps)
I’ve been using Tinder for the last 2 years and since my first swipes, I’ve had a hunch that Tinder loads the deck against me to keep me on the app for longer. I promise I’m not salty about not getting enough matches, I just feel I could be getting these matches with less swiping. Currently, I average 9 left swipes (rejection) to 1 right swipe (hopeful acceptance), and would argue I don’t have a specific “type”.
我过去两年一直在使用Tinder,自从第一次刷卡以来,我就有一种直觉,那就是Tinder会对着我的平台加载,以使我在应用程序上的停留时间更长。 我保证我不会因为没有足够的比赛而感到不安,我只是觉得我可以减少刷卡就可以得到这些比赛。 目前,我平均有9次向左滑动(拒绝)到1次向右滑动(希望接受),并认为我没有特定的“类型”。
However, a definite side effect of an app that shows you the world and values quantity over quality, is that you develop some conscious and unconscious bias on which way you’re swiping. Whether that would be profiles with sporty photos that irrationally make me feel lazy or profiles with bios that are only used to plug Instagram profiles, there are certain things I always half-consciously swipe left on. All this may sound shallow to non-Tinder users, but online daters will all agree this is the reality of dating in 2020.
但是,向您显示世界并重视数量而不是质量的应用程序的明确副作用是, 您会在刷卡方式上产生自觉和不自觉的偏见 。 无论是带有运动性照片的个人资料让我感到不懒惰,还是带有个人简介的个人资料(仅用于插入Instagram个人资料),某些事情我总是半意识地向左滑动。 对于非Tinder用户来说,这一切听起来似乎很浅,但是在线约会者都同意这是2020年约会的现实。
So I asked myself:
所以我问自己:
Why is it that, in an age where Spotify can accurately create playlists based on my music taste and Youtube is able to feed me video after video I will passively consume, Tinder only shows me profiles I want to swipe right on 10% of the time?
为什么在这个时代,Spotify可以根据我的音乐喜好准确地创建播放列表,而Youtube能够在我被动消费视频之后向我提供视频,Tinder仅显示我想在10%的时间向右滑动的个人资料?
It’s important to say here that Tinder does use an algorithm to help curate peoples experience on the app. They claim it helps people match more frequently, get off the app and meet IRL. However, they are very vague on how this algorithm actually works, but they do say that the more you use the app, the more matches you get. Who would’ve guessed?
重要的是在这里说Tinder确实使用了一种算法来帮助组织人们在该应用程序上的体验。 他们声称它可以帮助人们更频繁地进行比赛,离开应用程序并满足IRL。 但是,他们对这种算法的实际工作方式非常不清楚,但是他们的确说您使用该应用程序的次数越多,获得的匹配项就越多。 谁会猜到?
So, if Tinder has an algorithm that is so focused around matches, why does it show me profiles I don’t want to match with 90% of the time. Is Tinder’s algorithm just bad? Am I more random and spontaneous than I think? Or am I just weirdly picky and hard to please?
因此,如果Tinder的算法专注于比赛,为什么它会向我显示我不想90%的时间匹配的配置文件。 Tinder的算法不好吗? 我比我想像的更加随意和自发吗? 还是我只是奇怪地挑剔而难以取悦?
建议很重要 (Recommendations matter)
My hypothesis/conspiracy theory is that Tinder knows who I am likely to swipe right on and rations out those profiles, and in an attempt to gamify the experience, keep me swiping for longer and hook me to their app. A tactic akin to slot machines, video game loot boxes called “variable rate reinforcement”, and one of the widely recognized dark design patterns.
我的假设/阴谋论是,Tinder知道我很可能会向谁滑动并分配这些配置文件,并且为了使游戏体验游戏化,请让我滑动更长的时间并吸引我使用他们的应用程序。 一种类似于老虎机的策略,被称为“ 可变速率强化 ”的视频游戏战利品箱以及广泛认可的深色设计模式之一 。
“The player is basically working for reward by making a series of responses, but the rewards are delivered unpredictably… Dopamine cells are most active when there is maximum uncertainty, and the dopamine system responds more to an uncertain reward than the same reward delivered on a predictable basis.”
“玩家基本上是通过做出一系列React来争取报酬,但是报酬却无法预料地传递……当存在最大不确定性时,多巴胺细胞最活跃,而多巴胺系统对不确定报酬的React要比对获得的相同报酬更多。可预测的基础。”
Dr Luke Clark, director at the Center for Gambling Research at the University of British Columbia
不列颠哥伦比亚大学赌博研究中心主任卢克·克拉克博士
调查方法 (Methods of investigation)
To test this hypothesis, I decided to assess whether there was a distinct pattern of my “Matches” compared to my “Left Swipes”.
为了检验该假设,我决定评估与“左挥杆”相比,我的“比赛”是否有明显的模式。
To work out these patterns, I trained various machine learning models with data from both my “Matches” and random “Left Swipes”. I split them into Pictures, Bios, Music and in the end, the full profile.
为了弄清楚这些模式,我使用“比赛”和随机“左挥杆”中的数据训练了各种机器学习模型。 我将它们分为“图片”,“个人简介”,“音乐”,最后是完整的个人资料。
Consequently, I tested the generated results made by these models side by side, choosing my favourite of the two options presented each time. If I choose the result generated by the model trained on my “Matches” more often, then it would prove my match preferences were learnable by an AI and hence, Tinder could consistently fill my feed more with people I would like to swipe right on.
因此,我并排测试了这些模型产生的结果,并从每次出现的两个选项中选择了我最喜欢的。 如果我更频繁地选择在“比赛”上训练的模型所生成的结果,那么这将证明我的比赛偏好是AI可以学习的,因此,Tinder可以不断用我想滑过的人继续填充我的供稿。
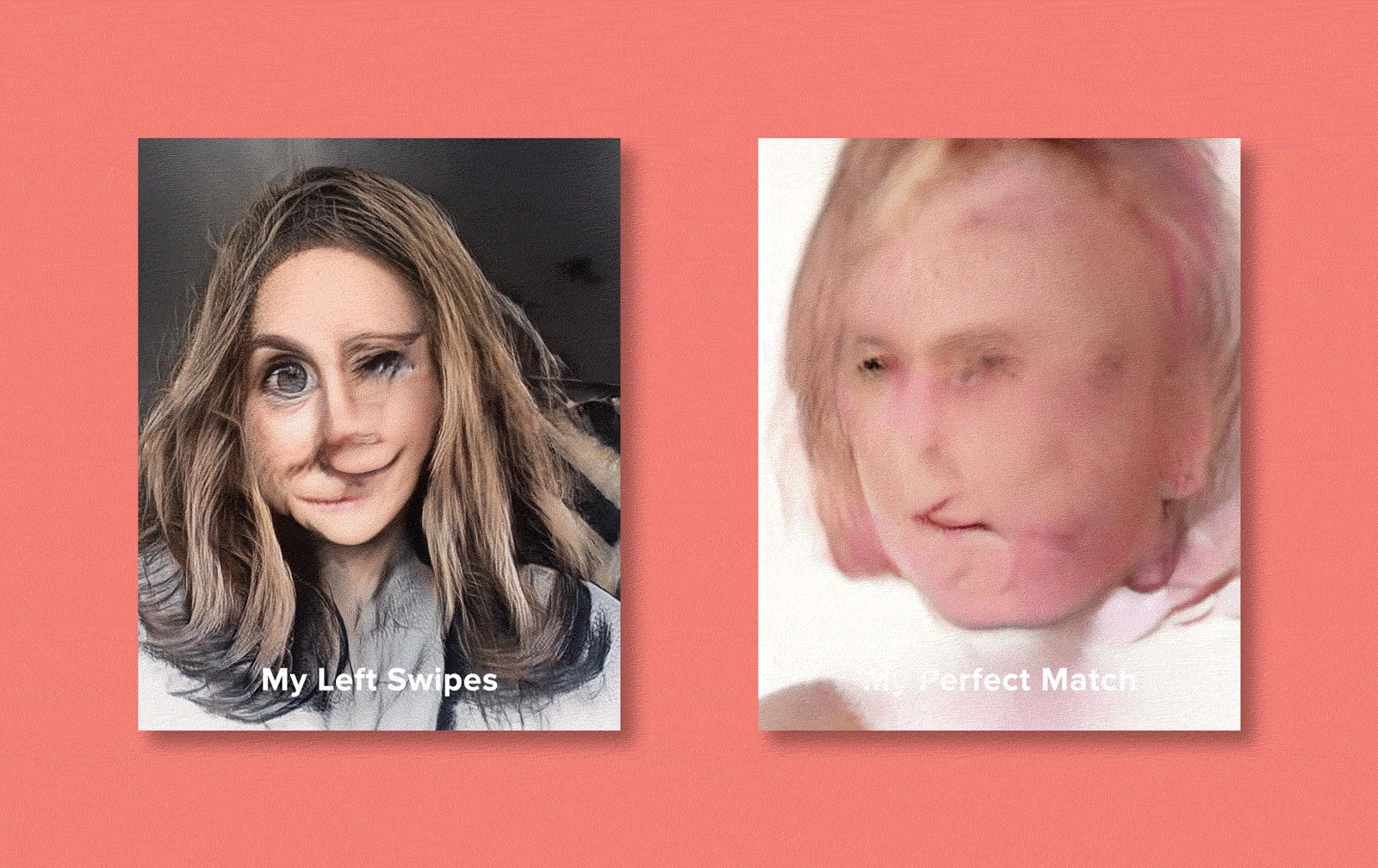
生成(弗兰肯斯坦的)头像 (Generating (Frankenstein’s) Profile Pictures)
Profile pictures are a key part of the Tinder experience — it’s the first thing you see and I would be lying if I didn’t say it’s probably the most important aspect of a profile. Tinder evidently thinks so too, as throughout the apps short history it has continued to increase the size of the profile picture in its UI.
个人资料图片是Tinder体验的关键部分-这是您看到的第一件事,如果我不说这可能是个人资料中最重要的方面,我会撒谎。 Tinder显然也这么认为,因为在整个应用程序的短历史中,它一直在增加UI中个人资料图片的大小。
So to generate fake profile pictures, I trained a Stylegan2 Model on RunwayML with 212 images of the faces of my “Left Swipes” and a separate model on 212 “Match” faces, both for 5000 steps.
因此,要生成伪造的个人资料图片,我在RunwayML上训练了一个Stylegan2模型, 其中包含212张“左挥杆”面Kong的图像,并在212张“匹配”面Kong上建立了单独的模型,均进行了5000步。
Due to my small data set, the resulting generated images were very abstract. However, going into the test I was pretty confident I would be easily able to discern which generated images were based on “The Perfect Match” model, based on the colour, composition and frankensteined facial features.
由于我的数据集很小,因此生成的生成图像非常抽象。 但是,在测试中, 我非常有信心,我将能够轻松识别出生成的图像是基于“完美匹配”模型的,该模型基于颜色,成分和坦率的面部特征。
This was not the case — during the test, I only selected “The Perfect Match”-generated picture 5 out of 10 times. Not too promising.
情况并非如此-在测试期间,我只选择了“完美匹配”生成的图片,其中有10幅是5幅。 不太有前途。
Tool used: https://runwayml.com/
使用的工具: https : //runwayml.com/
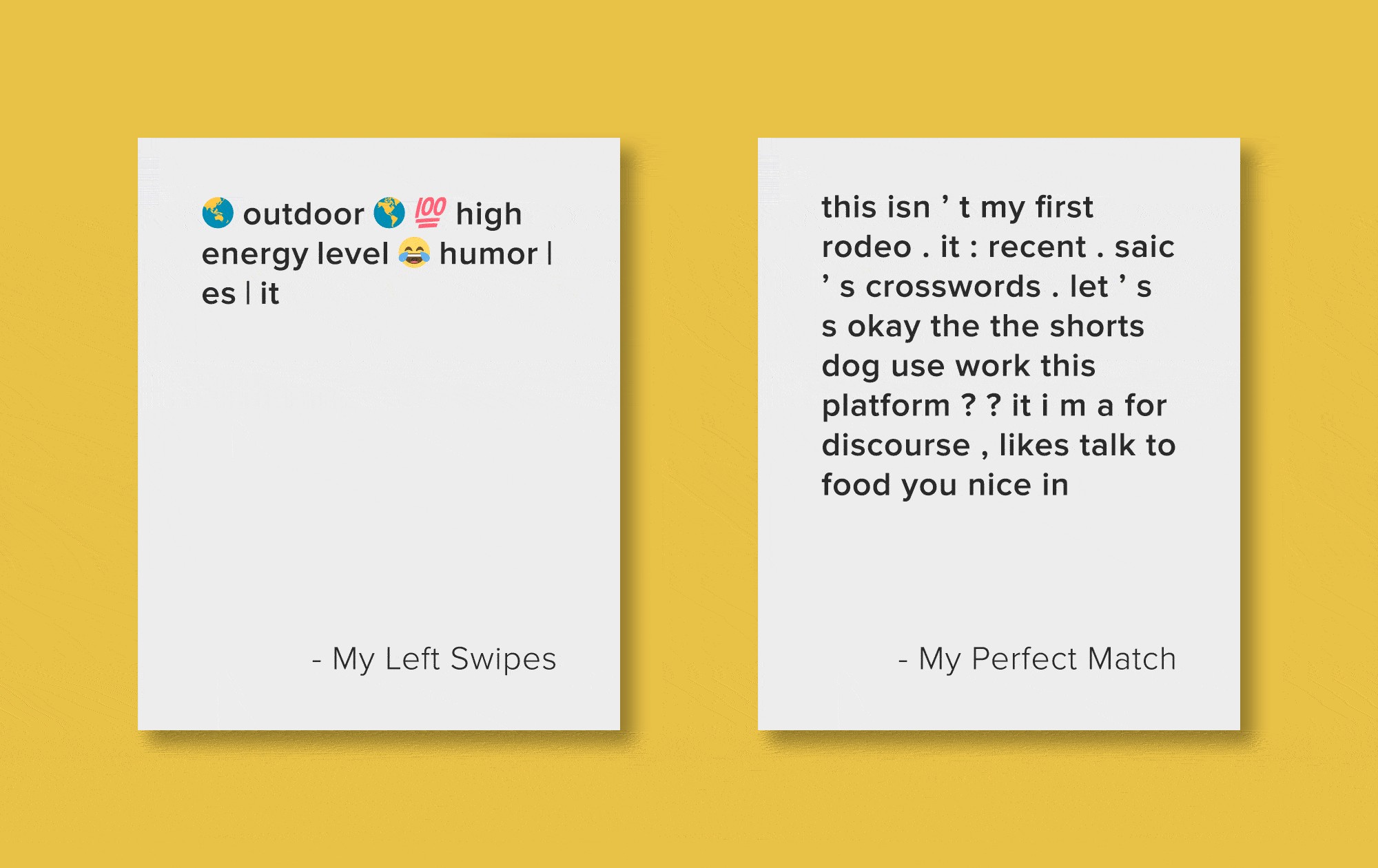
生成个人资料 (Generating Profile Bios)
Bios are a variable part of a Tinder profile: some users writing only their height, others a thesis on their perfect partner and a good proportion of users have no bio at all. For me, a bio is an important part of my swiping decision, but no bio is definitely better than a bad bio. Hence, out of my 212 matches, only 116 had bios. I also found 116 bios from random people I would normally swipe left on. These were used to train the Tensor flow-based Textgenrnn word-level model for 100 steps.
简历是Tinder个人资料中可变的部分:有些用户只写自己的身高,另一些用户则是他们完美伴侣的论文,并且很大一部分用户根本没有简历。 对我而言,生物是我刷卡决定的重要组成部分,但没有任何生物肯定比不良生物更好。 因此,在我的212场比赛中,只有116场得到了个人简历。 我还从通常会向左滑动的随机人群中找到了116张个人简历。 这些用于训练基于Tensor流的Textgenrnn单词级模型100个步骤。
Again, as in the previous step, the generated results were very abstract but definitely recognisable as Tinder bios, even if they made no sense.
再次,与上一步一样,生成的结果非常抽象,但即使没有意义,也可以肯定地识别为Tinder bios。
During the test, I went for “The Perfect Match”-generated bio 7 out of 10 times. However, 2 were easy to spot because I clearly recognised some generated text from the datasets, so the real result was 5 out of 8.
在测试期间,我参加了10次“完美匹配”生成的bio 7。 但是,很容易发现2个,因为我清楚地从数据集中识别出一些生成的文本,因此实际结果是8个中的5个。
Tool used: https://colab.research.google.com/
使用的工具: https : //colab.research.google.com/

产生“我们的歌” (Generating “Our Song”)
The final piece of a Tinder profile is music, as users have the option to add an Anthem song, as well as a feed of their current most-listened artists. For my test, I focused solely on the user’s Anthems as these are more common and they would give me cleaner data points. For me, these anthems are quite important and help to add detail to my picture of someone.
Tinder资料的最后一部分是音乐,因为用户可以选择添加一首Anthem歌曲,以及他们目前最受关注的艺术家的供稿。 对于我的测试,我只专注于用户的国歌,因为这些国歌更常见,它们可以为我提供更清晰的数据点。 对我而言,这些国歌非常重要,有助于为我的某人照片添加细节。
Through brute force, I compiled a playlist on Spotify of the 99 songs from my “Matches” and 99 others from random “Left Swipes”. I then used Spotify’s similar playlist feature via Skiley to generate new playlists. I was worried that the diverse data sets used would lead to very random playlists but the resulting playlist felt very close to the input data.
通过蛮力,我在Spotify上为我的“比赛”中的99首歌曲和随机的“左击”中的99首歌曲编写了一个播放列表。 然后,我通过Skiley使用Spotify的类似播放列表功能来生成新的播放列表。 我担心使用的各种数据集会导致播放列表非常随机,但是生成的播放列表感觉非常接近输入数据。
During the test, I was shown the song name, artist and album artwork but not allowed to listen to the song because the majority of the time this is how I engage with Tinder profile Anthems.
在测试过程中,向我显示了歌曲名称,艺术家和专辑插图,但不允许我听这首歌,因为在大多数情况下,这是我与Tinder简介Anthems互动的方式。
As a result, I picked the “The Perfect Match”-generated song 6 out of 10 times.
结果,我从10次歌曲中挑选了6首“ The Perfect Match”生成的歌曲。
Tool used: https://skiley.net/
使用的工具: https : //skiley.net/
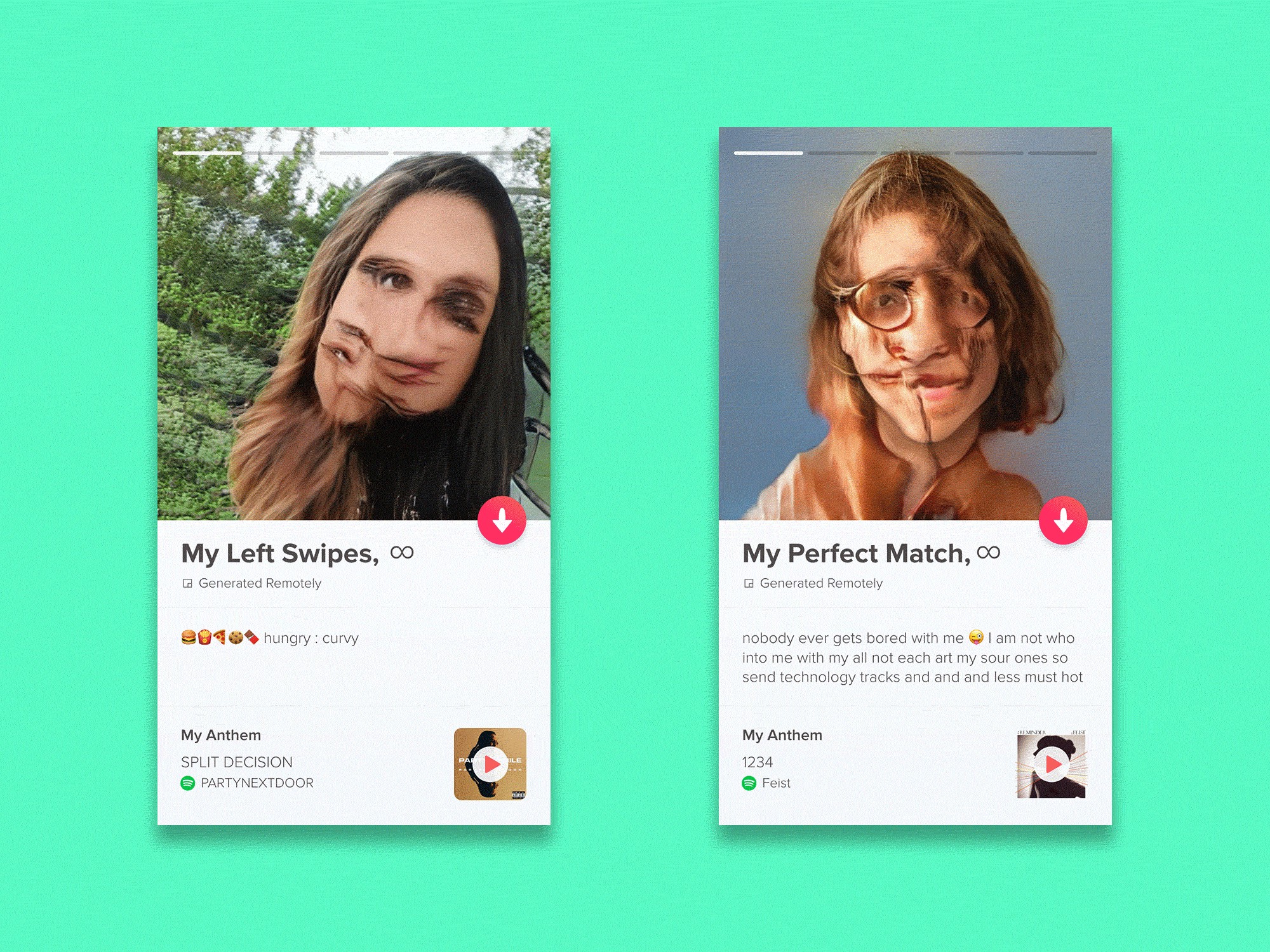
最终测试-比较完全生成的配置文件 (The Final Test — Comparing fully generated profiles)
For the last part of the test, I compared full profiles of generated photos, bio and anthems. This was most true to life of the test, as the combination of all the different points gave me a really clear picture of a profile. As such, I managed to pick “The Perfect Match” generated profile 8 out of 10 times. However, because of the bios, I again clearly recognised 2 of the generated bios from the data sets — the real result was 6 out of 8.
在测试的最后一部分,我比较了生成的照片,个人简介和国歌的完整资料。 这是测试生活中最真实的情况,因为所有不同点的结合使我对配置文件有了非常清晰的了解。 因此,我设法从10次中选择8次“完美匹配”生成的配置文件。 但是,由于有履历表,我再次清楚地从数据集中识别出2个生成的履历表-实际结果是8分之6。
结论 (Conclusion)
In the end, I managed to correctly pick “The Perfect Match” generated profile 61.1% of the time. This was all done with very crude models (except Spotify) and tiny data sets. Whereas Tinder would have access to more sophisticated models, a lot more data and real data scientists. Hence I would tentatively say my hypothesis is correct. If Tinder wanted to, I believe they could learn my preferences and show me profiles I would match with at a much more consistent rate.
最后,我设法在61.1%的时间内正确选择了“完美匹配”生成的配置文件。 所有这些都是通过非常原始的模型(Spotify除外)和微小的数据集完成的。 Tinder可以访问更复杂的模型,而更多的数据和真实数据科学家也可以使用。 因此,我会暂时说出我的假设是正确的。 如果Tinder愿意,我相信他们可以了解我的喜好并向我显示与我匹配的个人资料,并且速度要一致得多。
There are many reasons why I think they choose not to implement a more accurate and user-focused algorithm — perhaps it would ruin the fun of the app or maybe people wouldn’t opt for subscription plans if they found matches so easily. Cynically, I believe they tune their algorithm to balance out the outcomes of Matches, Fun and Profit. They are a company after all, not a benevolent cupid armed with digital arrows.
我认为他们选择不实施更准确和以用户为中心的算法有很多原因-可能会破坏应用程序的乐趣,或者如果人们如此轻松地找到匹配项,人们就不会选择订阅计划。 玩世不恭的是,我相信他们会调整算法以平衡比赛,娱乐和利润的结果。 毕竟,他们是一家公司,而不是用数字箭头武装的仁慈丘比特。
总结思想 (Closing Thoughts)
At the end of the day, I feel my results were quite obvious and entirely predictable. However, I feel that fact I was able to create this experiment and put Tinder on trial with no Machine Learning and very little coding experience, tells us of a new paradigm emerging. One where companies or governments can no longer hide behind or outright blame their algorithms for anti-user features or more importantly discrimination. People (Companies or Governments) make choices about what they want their algorithm to do, and now with new easy to use the software we can check that we agree with their choices.
归根结底,我觉得我的结果非常明显,完全可以预测。 但是,我觉得我能够创建此实验并在没有机器学习且几乎没有编码经验的情况下将Tinder投入试用的事实告诉我们新的范式正在出现。 公司或政府不能再将其算法隐藏或完全归咎于反用户功能或更重要的歧视 。 人们(公司或政府)可以选择他们想要的算法,现在,有了易于使用的新软件,我们可以检查我们是否同意他们的选择。
On a personal level, what I am also taking away from this experiment is a greater understanding of Machine Learning but most importantly a deeper awareness and questioning of my conscious and unconscious biases.
在个人层面上,我还从这个实验中脱颖而出,是对机器学习有了更深入的了解,但最重要的是,对我的有意识和无意识偏见有了更深的认识和质疑。
Meet the authorFred Wordie is a critical designer based in Berlin. His work uses design fiction to explore how we as a society engage with technology and social issues. His work can be found here.
认识作者 Fred Wordie是一位驻柏林的重要设计师。 他的作品使用设计小说来探索我们这个社会如何处理技术和社会问题。 他的工作可以在这里找到。
About AIxDesignAIxDesign is a place to unite practitioners and evolve practices at the intersection of AI/ML and design. Currently, we are organizing monthly virtual events, sharing content, exploring collaborative projects, and looking to developing fruitful partnerships.
关于AIxDesign AIxDesign是在AI / ML与设计的交汇处团结实践者和发展实践的地方。 目前,我们正在组织每月一次的虚拟活动,共享内容,探索协作项目以及寻求发展卓有成效的合作伙伴关系。
To stay in the loop, follow us on Instagram, Linkedin, or subscribe to our monthly newsletter to capture it all in your inbox.
要保持循环状态,请在Instagram , Linkedin上关注我们,或订阅我们的每月时事通讯以将其全部捕获到您的收件箱中。
翻译自: https://medium.com/aixdesign/an-unscientific-investigation-of-tinders-algorithm-2c086bf3deb4
科研图像压缩算法比例,雷娜