arima模型怎么拟合
什么是ARIMA? (What is ARIMA?)
ARIMA models are one of the most classic and most widely used statistical forecasting techniques when dealing with univariate time series. It basically uses the lag values and lagged forecast errors to predict the feature values.
ARIMA模型是处理单变量时间序列时最经典,使用最广泛的统计预测技术之一。 它基本上使用滞后值和滞后的预测误差来预测特征值。
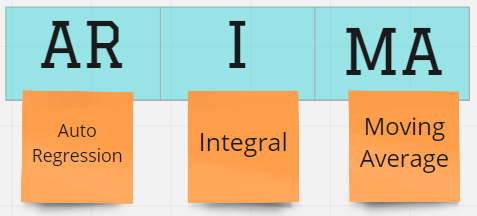
AR: using the lags of previous values
AR:使用先前值的滞后
I: non-stationary differencing
I: 非平稳差分
MA: moving average for the error term
MA: 移动平均线 对于错误项
Some of these terms are very commonly used when working with time-series data. ARIMA models can fit accurately if we deeply understand these terms or components of the data. Following are the few of them:
其中一些术语在处理时间序列数据时非常常用。 如果我们深刻理解数据的这些术语或组成部分,则ARIMA模型可以准确拟合。 以下是其中一些:
趋势: (Trend:)
Data is considered to have a trend when there is an increase or decrease direction in the data. E.g. increase of airline passengers during summer, reduction in a number of customers during weekdays, etc.
当数据中存在增加或减少的方向时,数据被认为具有趋势 。 例如,夏季航空乘客的增加,工作日乘客数量的减少等。
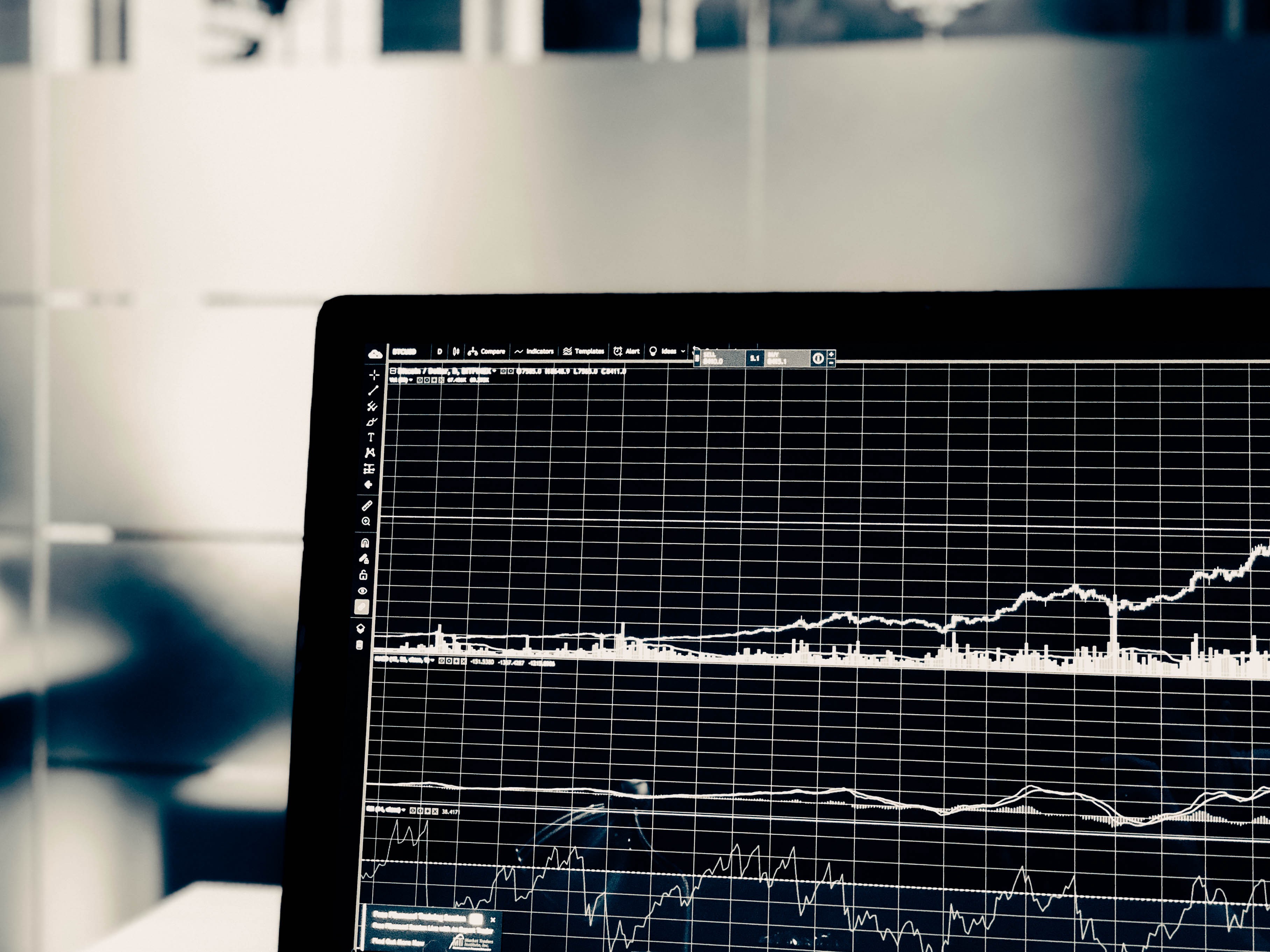
季节性: (Seasonality:)
Data is considered to have a seasonal pattern if the data is influenced by external factors. For instance, growth and fall of leaves are driven by the weather/season of mother nature.
如果数据受外部因素影响,则认为该数据具有季节性模式 。 例如,树叶的生长和下降是由自然的天气/季节驱动的。
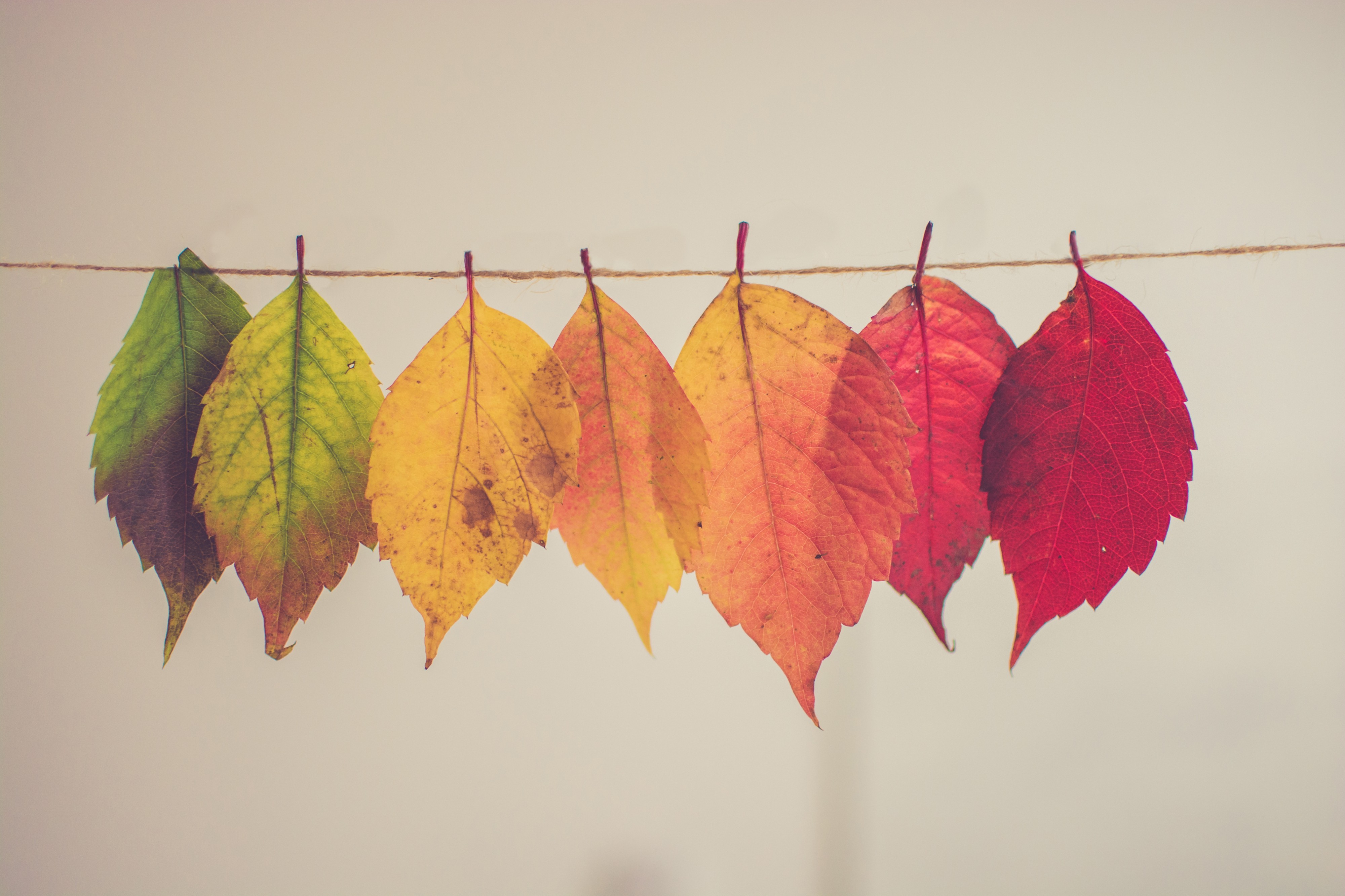