Our team at Jigsaw uses artificial intelligence to spot toxicity online, and part of our work focuses on how to make that information more useful to the platforms and publishers that need it to host better conversations. Sometimes that means helping platforms moderate conversations more effectively, but we’ve also been exploring how we can help the users — the people actually writing the comment or post — better understand the impact of their words.
我们在Jigsaw的团队使用人工智能在网上发现毒性,而我们的部分工作集中在如何使该信息对需要此信息以进行更好的对话的平台和发布者更有用。 有时,这意味着帮助平台更有效地缓解对话,但是我们也一直在探索如何帮助用户-实际写评论或帖子的人-更好地理解他们的话语的影响。
We all understand how toxicity online makes the internet a less pleasant place. But the truth is, many toxic comments are not the work of professional trolls or even people deliberately trying to derail a conversation. Independent research also points to how some people regret posting toxic comments in hindsight. A study we did with Wikipedia in 2018 suggested that a significant portion of toxicity comes from people who do not have a history of posting offensive content.
我们都知道在线的毒性如何使互联网不再那么令人愉快。 但事实是,许多有毒评论不是专业巨魔的工作,甚至不是人们故意使对话脱轨的工作。 独立研究还指出了一些人后悔发表有毒评论的遗憾。 我们在2018年与Wikipedia进行的一项研究表明,毒性的很大一部分来自没有过分冒犯性内容历史的人。
If a significant portion of toxic content was just people having a bad day or a moment of tactlessness, we wanted to know if there was a way for us to harness the power of Perspective to provide real-time feedback to people as they were writing the comment — just a little nudge for people to consider framing their comments in a less harmful way. Would that extra moment of consideration make any difference?
如果有毒成分的很大一部分只是一天的心情不好或一阵机智的人,我们想知道是否有一种方法可以利用Perspective的功能为正在编写的人提供实时反馈评论-促使人们考虑以一种危害较小的方式来构架他们的评论。 额外的考虑会有所作为吗?
Several of our partners using Perspective API added what we call “authorship feedback” into their comment systems, and we worked together to study how this feature affects conversations on their platforms. The idea is simple: they use Perspective’s machine learning models to spot potentially toxic contributions and the platform provides a signal to the author right as they’re writing the comment. (This required carefully crafting the feedback message: eg. less-than-encouraging messages can have completely the opposite effect.) By suggesting to the user that their comment might violate community guidelines, they have an extra few seconds to consider adjusting their language.
我们几个使用Perspective API的合作伙伴在他们的评论系统中添加了我们所谓的“作者反馈”,我们共同研究了此功能如何影响他们平台上的对话。 这个想法很简单:他们使用Perspective的机器学习模型发现潜在的有害贡献,并且该平台在撰写评论时向作者提供了正确的信号。 (这需要仔细设计反馈消息:例如,少于鼓励的消息可能会产生完全相反的效果。)通过向用户建议他们的评论可能违反社区准则,他们还有几秒钟的时间来考虑调整他们的语言。
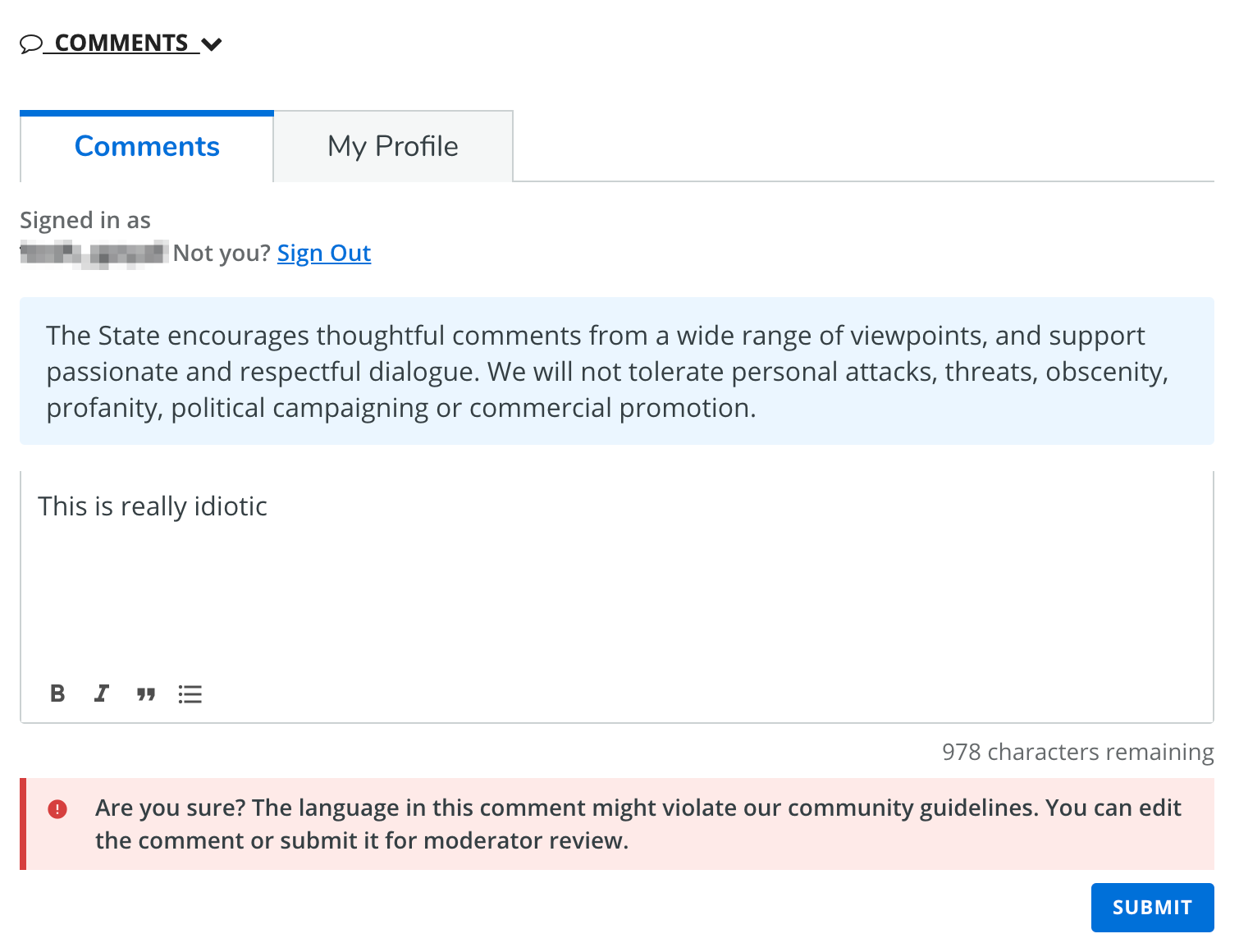
Here’s what we learned from those studies.
这是我们从那些研究中学到的东西。
Coral by Vox Media, a prominent commenting platform used by media organizations around the world has been integrating authorship feedback into their “Talk” platform since 2017. Commenters submit their comment, and if they used toxic language or made personal attacks, a message appears asking if they want to rephrase (shown above). The message was carefully designed to avoid active accusations, and focus on the language, and not the person. The feature is also designed to reduce the moderation load by encouraging commenters to improve their behavior without the need for human intervention. Coral partnered with McClatchy to run this experiment on two of their websites to assess whether the feedback prompted commenters to change what they wrote.
自2017年以来,Vox Media的Coral by Vox Media是世界各地媒体组织使用的著名评论平台,自此以来,该作者就一直将作者反馈信息整合到其“ Talk”平台中。评论者提交评论,并且如果他们使用有毒语言或进行人身攻击,则会出现一条询问消息如果他们想改写(如上所示)。 该消息经过精心设计,避免了积极的指责,并专注于语言,而不是人员。 此功能还旨在通过鼓励评论者在无需人工干预的情况下改善其行为,从而减轻审核负担。 Coral与McClatchy合作在他们的两个网站上进行了该实验,以评估反馈是否促使评论者更改了他们的写作。
Analysis shows that in a six-week experiment on one of the McClatchy websites 36% of those who received the feedback edited their comment to reduce toxicity. That number rose to 40% for a 12-week experiment on another site. In both cases, about 20% of commenters abandoned the comment after seeing the feedback and about 40% ignored the nudge and still submitted a toxic comment.
分析表明,在一个麦克拉奇网站上进行的为期六周的实验中,收到反馈的人中有36%编辑了他们的意见以降低毒性。 在另一个站点进行为期12周的实验时,该数字升至40%。 在这两种情况下,约20%的评论者在看到反馈后放弃了评论,约40%的评论者忽略了轻推,仍然提交了有毒评论。
These encouraging results were supported by another Authorship-Feedback study we conducted with OpenWeb, a leading audience engagement platform that hosts 100 million active users per month. After analyzing 400,000 comments from 50,000 users, we found that 34% of users who received feedback powered by Perspective API chose to edit their comment, and 54% of those revised comments met the community standards and were published.
这些令人鼓舞的结果得到了我们与OpenWeb进行的另一份Authorship-Feedback研究的支持, OpenWeb是一个领先的受众互动平台,每月可容纳1亿活跃用户。 在分析了50,000个用户的400,000条评论后,我们发现收到了Perspective API支持的反馈的用户中有34%选择编辑其评论,而这些修订后的评论中有54%符合社区标准并已发布。
Cynics might assume that these results don’t necessarily suggest that authorship feedback helps to reduce toxicity, it just helps people write comments that avoid the machine learning models that detect it. But when OpenWeb studied their results more closely and compared comments before and after receiving feedback, it turns out that of all commenters who edited their language 44.7% of commenters replaced or removed offensive words, and 7.6% elected to rewrite their comment entirely.
愤世嫉俗的人可能会认为这些结果不一定表明作者反馈有助于降低毒性,而只是帮助人们撰写评论,避免使用机器学习模型来检测毒性。 但是,当OpenWeb更仔细地研究他们的结果并在收到反馈之前和之后比较评论时,事实证明,所有编辑其语言的评论者中,有44.7%的评论者替换或删除了令人反感的单词,而有7.6%的评论者选择完全重写其评论。
On a smaller but no less instructive scale, The Southeast Missourian newspaper included authorship feedback when they redesigned the commenting system website in 2018 to include Perspective API. Just by providing minor feedback to the people writing comments, the percentage of submitted comments that were “very likely” to be toxic declined by 96%, and the percentage of all potentially toxic comments declined by 45%. Twenty-four percent of commenters revised their commentary to be less toxic after receiving a message. This number grew to more than 34% of users in the last year with each of those users’ comments declining in toxicity level by an average of 21%.
在较小但具有指导意义的规模上, 《东南密苏里州》报纸在2018年重新设计评论系统网站以包括Perspective API时包括作者反馈。 仅通过向撰写评论的人员提供少量反馈,“极有可能”成为毒性的已提交评论的百分比下降了96%,而所有具有潜在毒性的评论的百分比下降了45%。 收到消息后,有24%的评论者将其评论修改为毒性较小。 去年,这个数字增长到超过34%的用户,这些用户的评论中的每一个的毒性水平平均下降了21%。
It is worth noting that across multiple platforms, different durations of experiments, and varied timelines, approximately 35% of commenters do change their behavior for the better. This reproducibility suggests that while providing commenters feedback on their language won’t eliminate all toxicity, early results from platforms that have integrated the feature are encouraging. Providing subtle feedback — even just pointing out to people to reconsider their language — can measurably reduce the toxicity on a platform over time.
值得注意的是,在多个平台,不同的实验持续时间和不同的时间表上,大约35%的评论者的确改变了自己的行为。 这种可重复性表明,尽管向评论者提供有关其语言的反馈并不能消除所有毒性,但集成该功能的平台的早期结果令人鼓舞。 提供微妙的反馈-甚至只是指出要重新考虑他们的语言-都可以随着时间的推移显着降低平台的毒性。
Equally important, providing authorship feedback is another way for platforms to harness the power of Perspective API in a different, impactful way. Platforms and publishers around the world are using Perspective to make it easier to host better conversations and moderate discussions more efficiently. We’re excited to build on this success and find even more ways for this technology to help improve conversations online.
同样重要的是,提供作者反馈是平台以另一种有效的方式利用Perspective API的功能的另一种方法。 世界各地的平台和发行商都在使用Perspective来更轻松地主持更好的对话和更有效地主持讨论。 我们很高兴能够在此成功的基础上继续前进,并为该技术找到更多方法来帮助改善在线对话。