To improve your model’s performance on small objects, we recommend the following techniques:
为了提高模型在小对象上的性能,我们建议以下技术:
- Increasing your image capture resolution 提高图像捕获分辨率
- Increasing your model’s input resolution 增加模型的输入分辨率
- Tiling your images 平铺图片
Auto learning model anchors
自动学习模型锚
为什么小物体问题很难? (Why is the Small Object Problem Hard?)
The small object problem plagues object detection models worldwide. Not buying it? Check the COCO evaluation results for recent state of the art models YOLOv3, EfficientDet, and YOLOv4:
小物体问题困扰着全世界的物体检测模型。 不买吗? 查看最新模型YOLOv3 , EfficientDet和YOLOv4的COCO评估结果:
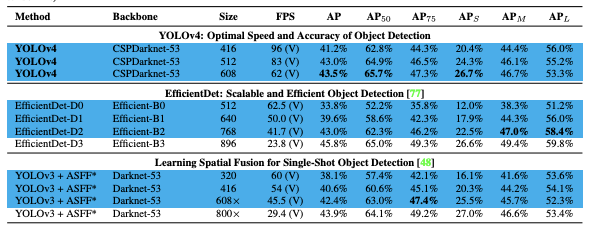
In EfficientDet for example, AP on small objects is only 12%, held up against an AP of 51% for large objects. That is almost a five fold difference!
例如,在EfficientDet中,小型对象的AP仅为12%,而大型对象的AP为51%。 那几乎是五倍的差距!
So why is detecting small objects so hard?
那么,为什么很难检测小物体呢?
It all comes down to the model. Object detection models form features by aggregating pixels in convolutional layers.
一切都取决于模型。 对象检测模型通过聚合卷积层中的像素来形成特征。
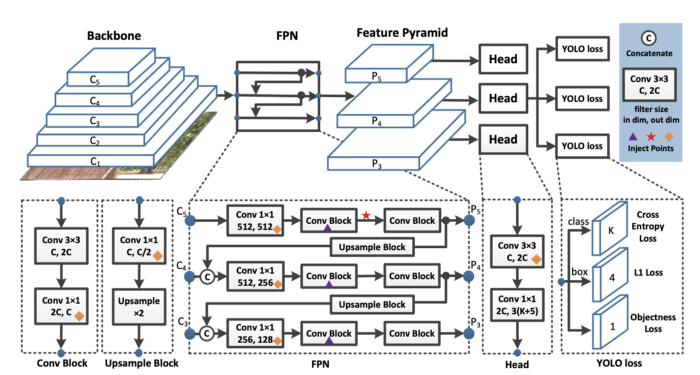
And at the end of the network a prediction is made based on a loss function, which sums up across pixels based on the difference between prediction and ground truth.
并且在网络的末端,基于损失函数进行预测,该损失函数基于预测和地面真实情况之间的差异对像素进行汇总。
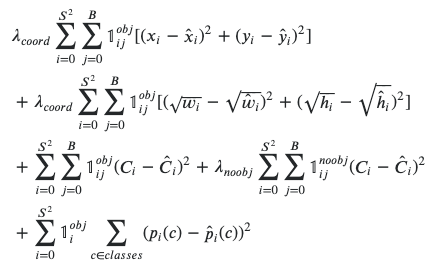
If the ground truth box is not large, the signal will small while training is occurring.
如果地面真理框不大,则在进行训练时信号会很小。
Furthermore, small objects are most likely to have data labeling errors, where their identification may be omitted.
此外,小物体最有可能出现数据标记错误,在此可能会省略其标识。
Empirically and theoretically, small objects are hard.
从经验和理论上讲,小物体很难。
提高图像捕获分辨率 (Increasing your image capture resolution)
Resolution, resolution, resolution… it is all about resolution.
分辨率,分辨率,分辨率……这全都与分辨率有关。
Very small objects may contain only a few pixels within the bounding box — meaning it is very important to increase the resolution of your images to increase the richness of features that your detector can form from that small box.
很小的物体在边界框内可能只包含几个像素,这意味着提高图像的分辨率以增加检测器可以从该小框形成的功能的丰富度非常重要。
Therefore, we suggest capturing as high of resolution images as possible, if possible.
因此,如果可能,我们建议捕获尽可能高分辨率的图像。
增加模型的输入分辨率 (Increasing your model’s input resolution)
Once you have your images at higher resolution, you can scale up your model’s input resolution. Warning: this will result in a large model that takes longer to train, and will be slower to infer when you start deployment. You may have to run experiments to find out the right tradeoff of speed with performance.
一旦获得更高分辨率的图像,就可以扩大模型的输入分辨率。 警告:这将导致大型模型需要花费较长的训练时间,并且在开始部署时将较慢地推断出来。 您可能需要进行实验,才能找到速度与性能之间的权衡。
You can easily scale your input resolution in our tutorial on training YOLOv4 by changing image size in the config file.
您可以在我们的有关YOLOv4培训的教程中轻松更改输入分辨率,方法是在配置文件中更改图像大小。
[net]
batch=64
subdivisions=36
width={YOUR RESOLUTION WIDTH HERE}
height={YOUR RESOLUTION HEIGHT HERE}
channels=3
momentum=0.949
decay=0.0005
angle=0
saturation = 1.5
exposure = 1.5
hue = .1
learning_rate=0.001
burn_in=1000
max_batches=6000
policy=steps
steps=4800.0,5400.0
scales=.1,.1
You can also easily scale your input resolution in our tutorial on how to train YOLOv5 by changing the image size parameter in the training command:
您还可以在我们的有关如何训练YOLOv5的教程中轻松调整输入分辨率,方法是在Training命令中更改image size参数:
!python train.py --img {YOUR RESOLUTON SIZE HERE} --batch 16 --epochs 10 --data '../data.yaml' --cfg ./models/custom_yolov5s.yaml --weights '' --name yolov5s_results --cache
Note: you will only see improved results up to the maximum resolution of your training data.
注意:您只会看到达到最大训练数据分辨率的改进结果。
平铺图片 (Tiling your images)
Another great tactic for detecting small images is to tile your images as a preprocessing step. Tiling effectively zooms your detector in on small objects, but allows you to keep the small input resolution you need in order to be able to run fast inference.
检测小图像的另一种很好的策略是将图像平铺作为预处理步骤。 平铺可以有效地将检测器放大到小物体上,但是可以保持所需的小输入分辨率,以便能够进行快速推理。
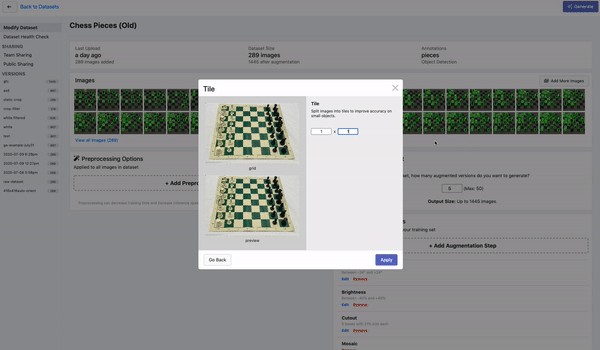
If you use tiling during training, it is important to remember that you will also need to tile your images at inference time.
如果在训练期间使用平铺,请务必记住,您还需要在推理时平铺图像。
通过扩充生成更多数据 (Generating More Data Via Augmentation)
Data augmentation generates new images from your base dataset. This can be very useful to prevent your model from overfitting to the training set.
数据扩充会从您的基本数据集中生成新图像。 这对于防止模型过度拟合训练集非常有用。
Some especially useful augmentations for small object detection include random crop, random rotation, and mosaic augmentation.
对于小物体检测,一些特别有用的增强包括随机裁剪,随机旋转和镶嵌增强 。
自动学习模型锚 (Auto Learning Model Anchors)
Anchor boxes are prototypical bounding boxes that your model learns to predict in relation to. That said, anchor boxes can be preset and sometime suboptimal for your training data. It is good to custom tune these to your task at hand. Thankfully, the YOLOv5 model architecture does this for you automatically based on your custom data. All you have to do is kick off training.
锚定框是模型学习预测的原型边界框。 就是说,锚框可以预先设置,有时对于训练数据而言不是最佳的。 自定义调整这些参数以适合您即将完成的任务是很好的。 幸运的是, YOLOv5模型架构会根据您的自定义数据自动为您完成此操作。 您要做的就是开始培训。
Analyzing anchors... anchors/target = 4.66, Best Possible Recall (BPR) = 0.9675. Attempting to generate improved anchors, please wait... WARNING: Extremely small objects found. 35 of 1664 labels are < 3 pixels in width or height. Running kmeans for 9 anchors on 1664 points... thr=0.25: 0.9477 best possible recall, 4.95 anchors past thr n=9, img_size=416, metric_all=0.317/0.665-mean/best, past_thr=0.465-mean: 18,24, 65,37, 35,68, 46,135, 152,54, 99,109, 66,218, 220,128, 169,228 Evolving anchors with Genetic Algorithm: fitness = 0.6825: 100%|██████████| 1000/1000 [00:00<00:00, 1081.71it/s] thr=0.25: 0.9627 best possible recall, 5.32 anchors past thr n=9, img_size=416, metric_all=0.338/0.688-mean/best, past_thr=0.476-mean: 13,20, 41,32, 26,55, 46,72, 122,57, 86,102, 58,152, 161,120, 165,204
过滤掉多余的类 (Filtering Out Extraneous Classes)
Class management is an important technique to improve the quality of your dataset. If you have one class that is significantly overlapping with another class, you should filter this class from your dataset. And perhaps, you decide that the small object in your dataset is not worth detecting, so you may want to take it out.
类管理是提高数据集质量的一项重要技术。 如果您有一个与另一个类明显重叠的类,则应从数据集中过滤该类。 也许您认为数据集中的小对象不值得检测,因此您可能希望将其取出。
Class omission and class renaming are all possible through Roboflow’s ontology management tools.
可以通过Roboflow的本体管理工具来实现类遗漏和类重命名。
结论 (Conclusion)
Properly detecting small objects is truly a challenge. In this post, we have discussed a few strategies for improving your small object detector, namely:
正确检测小物体确实是一个挑战。 在这篇文章中,我们讨论了一些改善小型物体检测器的策略,即:
- Increasing your image capture resolution 提高图像捕获分辨率
- Increasing your model’s input resolution 增加模型的输入分辨率
- Tiling your images 平铺图片
Auto learning model anchors
自动学习模型锚
As always, happy detecting!
一如既往,检测愉快!
翻译自: https://towardsdatascience.com/tackling-the-small-object-problem-in-object-detection-6e1c9976ee69