一次累加序列折线图解释
This article is the first part of a series of tutorials about interactive analytics in atoti’s dynamic pivot tables. Check also: Part 2: Marginal contribution.
本文是有关atoti动态数据透视表中的交互式分析的系列教程的第一部分。 另请检查:第2部分:边际贡献。
什么是非累加措施? (What are non-additive measures?)
We live in a complex world and often need to look at things from the bird-eye view to see the overall perspective. Say, we want to know the number of calories consumed during a day or total revenue of our e-commerce business.
我们生活在一个复杂的世界中,常常需要从鸟瞰的角度看待事物,以期从整体的角度来看待。 说,我们想知道一天中消耗的卡路里数量或我们电子商务业务的总收入。
Looking at the system from the bird-eye view means aggregating many contributing factors into summary measures. Oftentimes these aggregations are straightforward such as count or sum — we call them additive aggregations, but in certain cases aggregations are complex and things just don’t add up easily. In this case, we say aggregations are non-additive. The number of distinct items purchased in retail stores is a good example of a non-additive measure: when you break it down, say, by store name or by the city — the top-level value will not be equal to the sum of contributors.
从鸟瞰的角度看这个系统意味着将许多促成因素汇总为简易措施。 通常,这些聚合很简单,例如计数或总和-我们称它们为累加聚合,但在某些情况下,聚合很复杂,而且累加起来并不容易。 在这种情况下,我们说聚合是非加性的。 零售商店中购买的不同商品的数量是非加性度量的一个很好的例子:按商店名称或城市细分时,最高价值将不等于贡献者的总和。
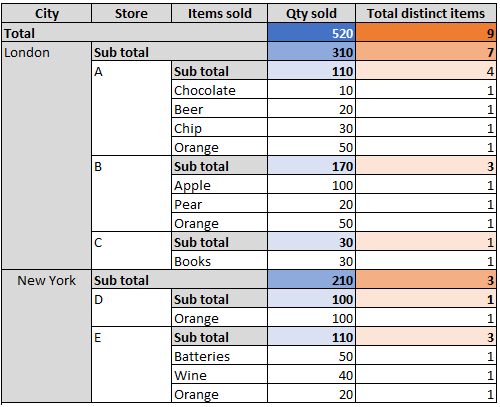
解释非累加措施:父级和同级聚合 (Explaining non-additive measures: parent and siblings aggregations)
Calculating non-linear aggregations is often an expensive and hard task, but what is even harder is to be able to dynamically explore and explain how this aggregation is driven by underlying contributors.
çalculating非线性聚集往往是昂贵而艰巨的任务,但什么是更难的是能够动态地探索和解释这是如何聚集是由潜在的贡献者驱动。
This problem is called contribution analysis. A typical example would be:
这个问题称为贡献分析。 一个典型的例子是:
- Allocating firm-wide economic capital down to trading desks, 将公司范围的经济资本分配到交易柜台,
- Line-by-line surplus requirements for insurance companies, 保险公司的逐笔盈余要求,
- Dynamic resource allocation. 动态资源分配。

In this post we will see how “parent” and “sibling” data relationships in atoti allow you to easily navigate any hierarchy of data and implement your on-the-fly allocation rules.
在这篇文章中,我们将看到atoti中的“父”和“兄弟”数据关系如何使您轻松导航数据的任何层次结构并实现即时分配规则。
I will be showing you different ways to explain non-additive measures against financial examples, as the calculations are often non-additive — think about Value-at-Risk, FRTB, EAD and others.
由于计算通常是非累加的,因此我将向您展示不同的方法来解释财务示例的非累加度量,请考虑风险价值,FRTB,EAD等。
解决方案1:将非累加措施分配给累加组件 (Solution #1: Allocating a non-additive measure into additive components)
In finance, the firm-level risk measure needs to be attributed down to desks in an additive manner to identify top risk drivers and allocate costs. Firm-level capital measure is non-additive, or more specifically it is sub-additive:
在金融领域,公司级风险度量需要以附加方式归因于服务台,以识别主要风险驱动因素并分配成本。 公司一级的资本计量是非累加的,或更具体地说,它是次累加的:

We can implement a measure allocating capital into additive components, formally the desired behaviour can be described with this formula:
我们可以实施一种将资本分配到附加组件中的措施,用以下公式可以正式描述所需的行为:

For example, the “Exposure-at-Default” (EAD) risk measure implemented in the SA-CCR notebook in the atoti gallery is a non-linear measure, i.e. if I break down the firm-level EAD, say, by a “book” — the sum of contributors will not be equal to the EAD calculated across “all books”. See, for instance, that EAD for the BusinessUnit_2 is 1,356 Mio vs the sum of EAD for the contributing books is 1,675 Mio.
例如,在atoti画廊的SA-CCR笔记本中实施的“默认暴露”(EAD)风险衡量是一种非线性衡量,即,如果我分解公司级别的EAD,例如用“图书” —贡献者的总和将不等于“所有图书”中计算出的EAD。 例如,请参见BusinessUnit_2的EAD为1,356 Mio,而贡献书籍的EAD总计为1,675Mio。
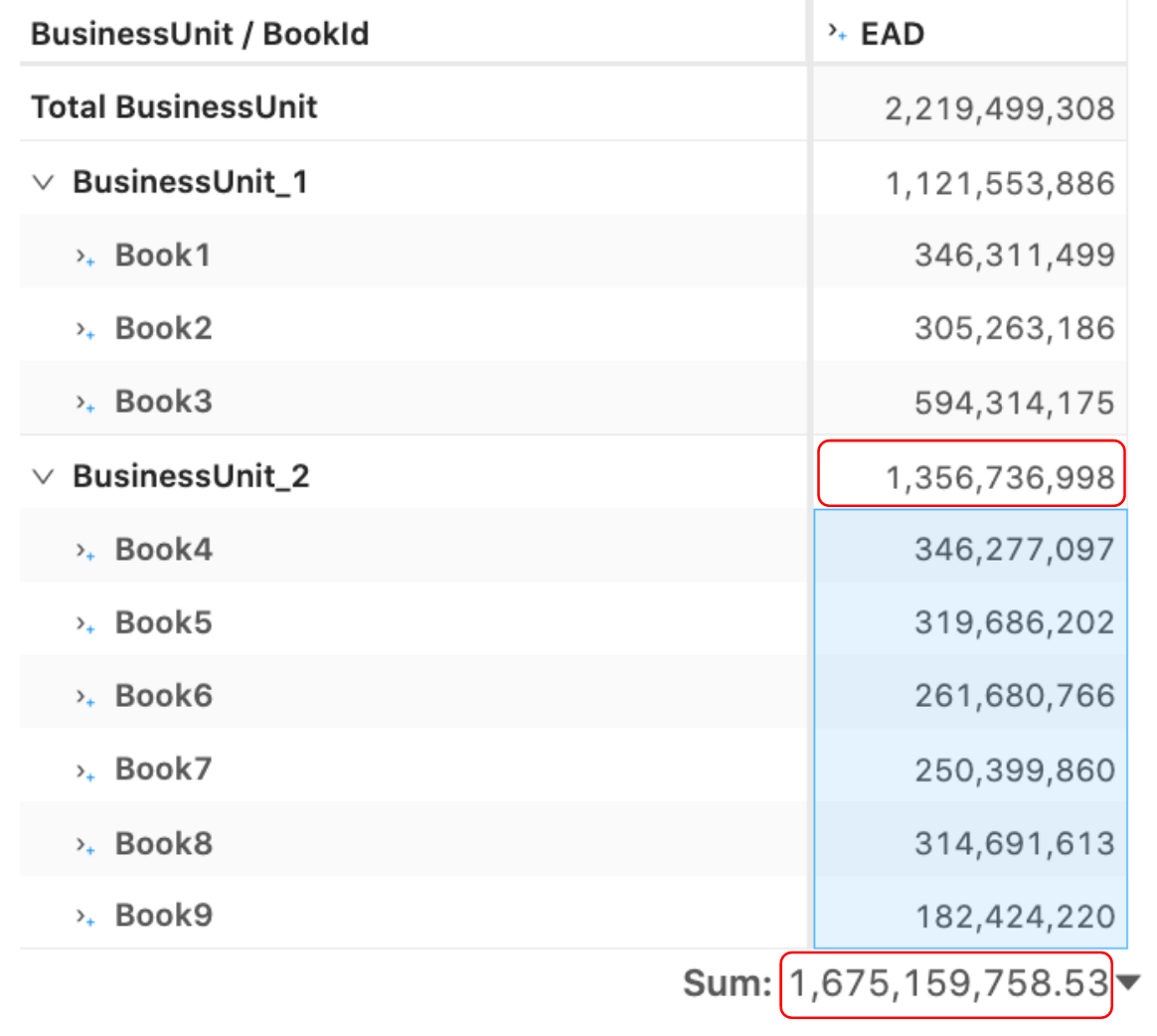
I need to implement an allocation methodology to make EAD behave “additively”: so that the sum of book level calculations match the firm-level number, as in the following screenshot for the “EAD Pro-Rata” where the sum of the highlighted books 1,356 Mio is equal to the EAD computed across those books:
我需要实现一种分配方法,以使EAD表现为“累加”:使帐簿级别的总和与公司级别的数字相匹配,如以下“ EAD Pro-Rata”的屏幕截图所示,其中突出显示的帐簿的总和1,356 Mio等于这些书中计算的EAD:
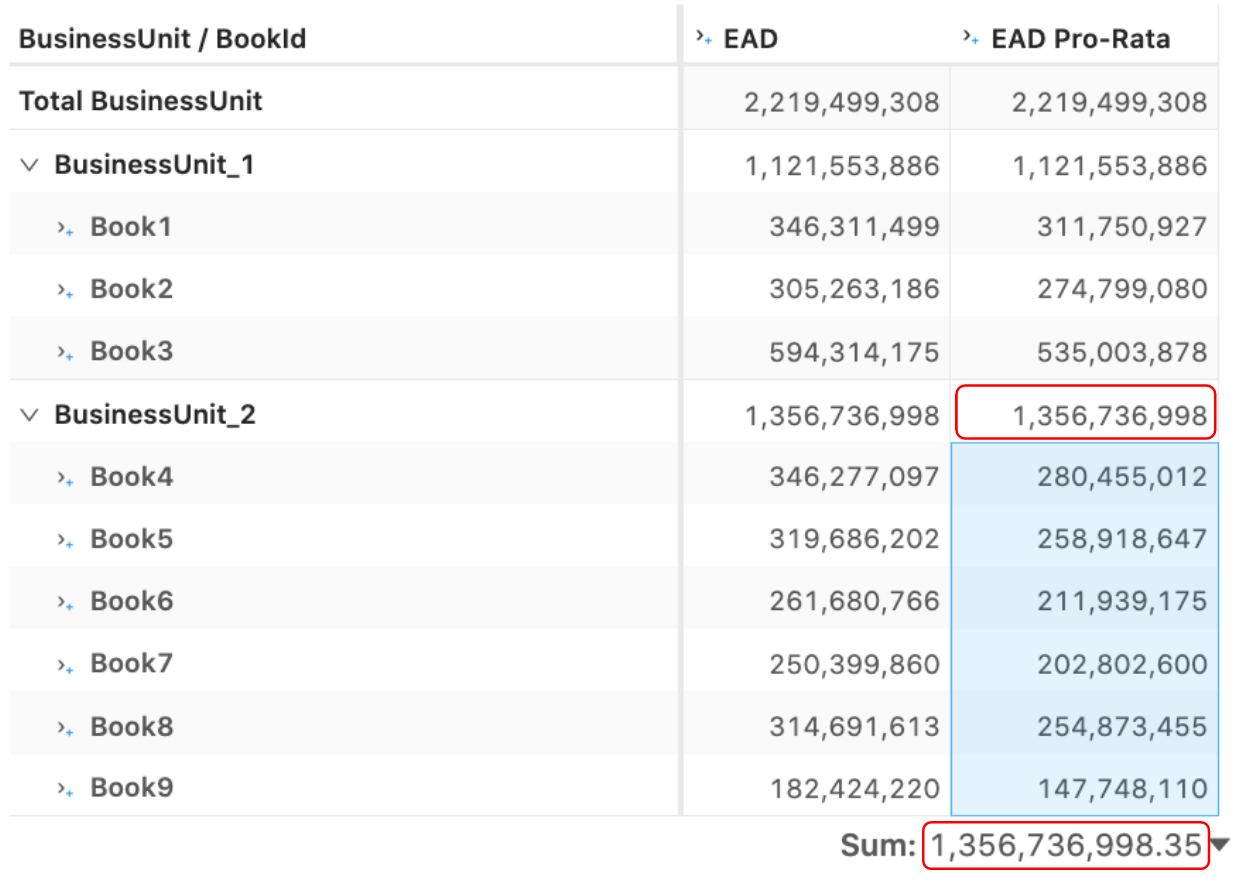
The allocation principle is a matter of a practitioner’s choice — see for instance, “Honour your contribution” by U. Koyluoglu and J. Stoker published in www.RISK.net in April 2002 or Capital Allocation to Business Units and Sub-Portfolios: the Euler Principle by D. Tasche.
分配原则取决于从业者的选择,例如,参见U. Koyluoglu和J. Stoker于2002年4月在www.RISK.net上发表的“为您的贡献感到荣幸”,或《业务部门和子组合的资本分配》: D. Tasche的欧拉原理。
In this post let’s take a simple example — pro-rata allocation — to illustrate the parent/child/sibling atoti concept.
在本文中,让我们以一个简单的示例(按比例分配)为例,说明父母/子女/兄弟姐妹的atoti概念。
To implement an allocation for a measure, I will multiply the value calculated above the contributor — at the parent level — by the weight of that contributor.
为了实现度量的分配,我将在贡献者上方计算的值(在父级)乘以该贡献者的权重。

Let’s compute the weight as a ratio of a book’s EAD by the sum of standalone calculations:
让我们将权重计算为书本EAD与独立计算之和的比值:
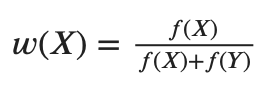
This is a simple way to scale the sum of elements back to the allocated value. As I mentioned earlier, the allocation principle can be more sophisticated to satisfy the desired properties. We’ll explore one more example — Euler allocations — in one of the next posts.
这是将元素总数缩放回分配值的简单方法。 正如我之前提到的,分配原理可以更复杂,以满足所需的属性。 在下一篇文章中,我们将探讨另一个示例-欧拉分配。
To implement the “EAD Pro-rata”, I first defined a measure which computes the sum of EADs for each of the book’s “siblings” including the current book itself (denoted as f(X)+f(Y) above):
为了实现“ EAD比例”,我首先定义了一种度量,该度量计算该书的每个“兄弟姐妹”(包括当前书籍本身)的EAD之和(上面表示为f(X)+ f(Y)):
m["EAD_sum_by_book"] = tt.agg.sum(m["EAD"], scope=tt.scope.siblings(h["BookId"]))
Then the weight w for each book can be defined as:
然后,每本书的权重w可以定义为:
m["Book_weight"] = m["EAD"] / m["EAD_sum_by_book"]
I will be using atoti’s parent_value function to retrieve the value above my current contributor (above current book) — you may read more about the parent_value function in the atoti doc:
我将使用atoti的parent_value函数来检索当前贡献者(当前书籍之上)之上的值-您可以在atoti文档中阅读有关parent_value函数的更多信息:
m["EAD_across_books"] = tt.parent_value(
m["EAD"], h["BookId"], degree=1, total_value=m["EAD"], apply_filters=True
)
As a recap of the above, this screenshot is showing what are the parent values and what are the siblings:
作为上述内容的回顾,此屏幕截图显示了哪些父级值和哪些同级项:
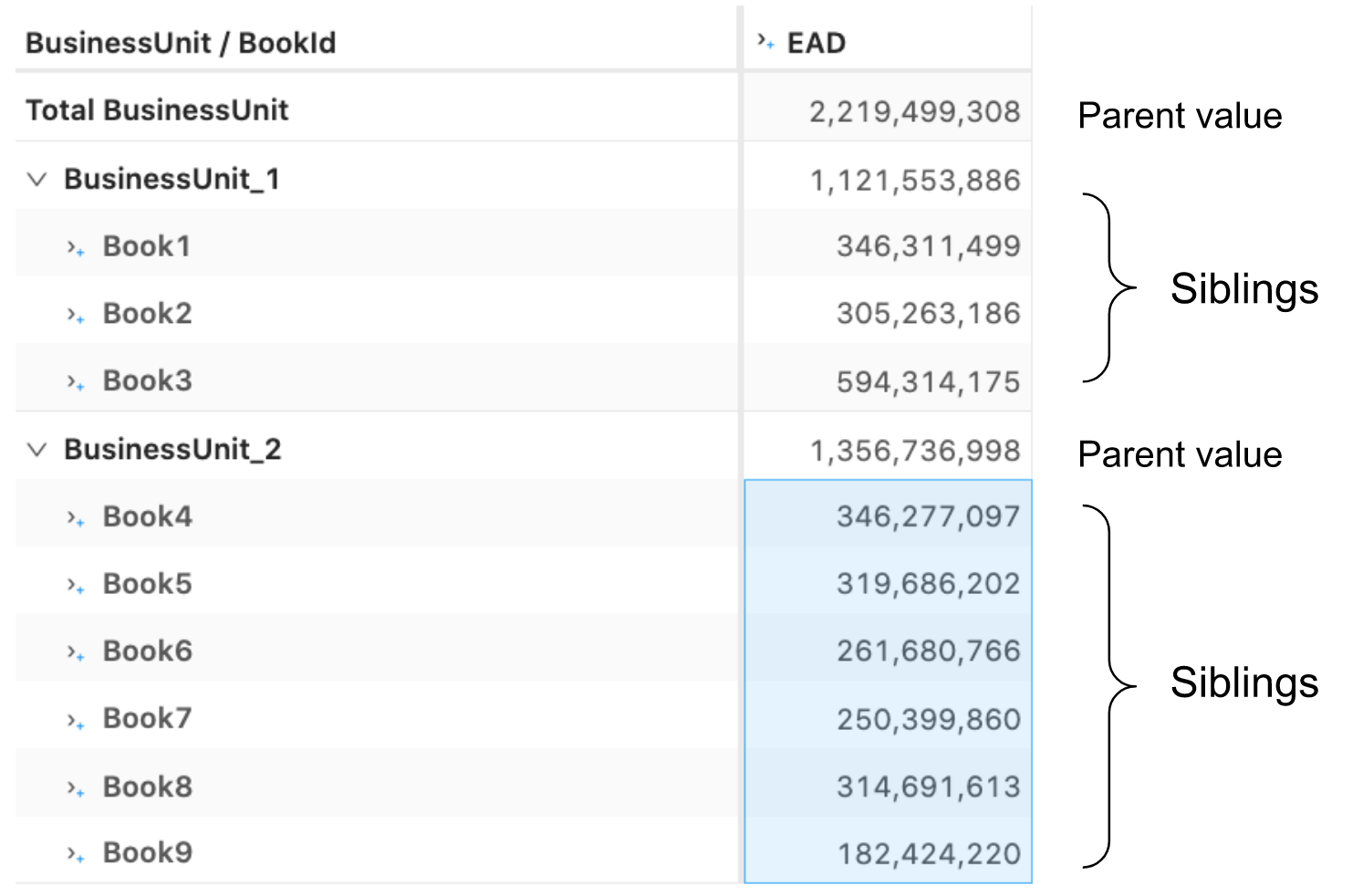
Finally the Pro-Rata measure is a simple product:
最后,Pro-Rata度量是一个简单的产品:
m["EAD Pro-Rata"] = m["Book_weight"] * m["EAD_across_books"]
If a hierarchy has multiple levels — for example, as in a banks’ organizational hierarchy — the allocation of the top-level values can apply recursively every time the user expands it.
如果某个层次结构具有多个级别(例如,在银行的组织层次结构中),则每次用户扩展它时,递归都可以应用顶级值的分配。
结论 (Conclusion)
In this post, we discussed how to use parent and sibling relationships in atoti to implement contributory measures and explained non-additive measures. I hope the described techniques can help you build powerful analytic applications!
在本文中,我们讨论了如何在atoti中使用父级和兄弟级关系来实施贡献性措施,并说明了非累加性措施。 我希望所描述的技术可以帮助您构建功能强大的分析应用程序!
一次累加序列折线图解释