Spiking Neural Networks (SNN) has recently been a topic of interest in the field of Artificial Intelligence. The premise behind SNN is that neurons in the brain, unlike our current modelling of it, communicate with one another via spike trains that occur at different frequencies and timings. Another way of visualizing the workings of natural neural netwoks is to image a pond with waves interacting with one another forming variety of patterns. The crucial advantage of SNN is the ability to encode time in a more meaningful way by making use of relative timings of the spikes.
尖刺神经网络(SNN)最近已成为人工智能领域的一个有趣话题。 SNN背后的前提是,与我们目前的模型不同,大脑中的神经元通过在不同频率和时间出现的尖峰序列相互通信。 可视化自然神经网络工作原理的另一种方式是对池塘成像,其中波浪相互影响,形成各种图案。 SNN的关键优势在于能够通过利用尖峰的相对定时以更有意义的方式对时间进行编码。
New hardware and math solutions are being worked on in the research community to make SNN practical. I argue that the existing deep learning framework is already capable of achieving what SNN promises without the need for new hardware or math solutions. The current deep learning framework using complex numbers instead of real numbers for neuronal activations and weights and also for input data representation can encode time as meaningfully as SNN.
研究社区正在研究新的硬件和数学解决方案,以使SNN切实可行。 我认为现有的深度学习框架已经能够实现SNN所承诺的功能,而无需新的硬件或数学解决方案。 当前的深度学习框架使用复数而不是实数进行神经元激活和权重以及输入数据表示,可以像SNN一样有意义地编码时间。
相位差和时间 (Phase delta and time)
In the current deep learning framework, the encoding of time remains clumsy. We encode it either explicitly as a numerical feature or implicitly via sequential data. The latter is only valid in the case of recurrent neural networks. However, even in recurrent networks, time does not fully enter the picture, because ordering alone does not encode relative time distances between input points.
在当前的深度学习框架中,时间的编码仍然很笨拙。 我们将其显式编码为数字特征,或者通过顺序数据隐式编码。 后者仅在递归神经网络中有效。 但是,即使在循环网络中,时间也无法完全进入画面,因为单独排序不能对输入点之间的相对时间距离进行编码。
In physics, interference is a phenomenon in which two waves superimpose to form a resultant wave of greater, lower, or the same amplitude. The shape of the resulting wave, assuming equal frequencies, depends on the phase delta. Check out this simulation to get a visual understanding.
在物理学中,干扰是一种现象,其中两个波叠加在一起,形成振幅更大,更低或相同的合成波。 假设频率相等,所得波的形状取决于相位差。 查看此模拟以获得视觉理解。
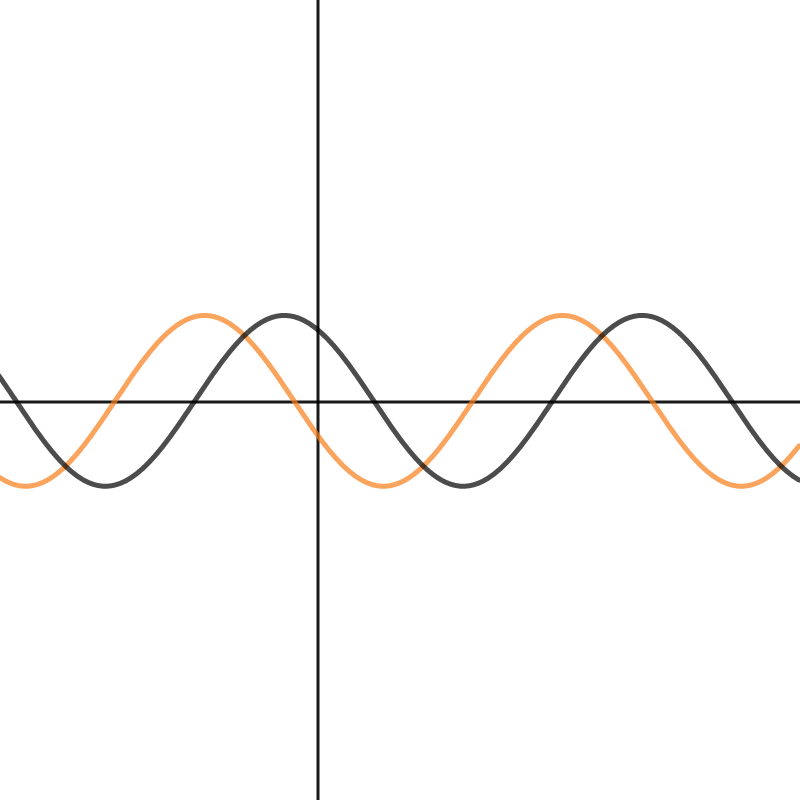
The interference dynamics of waves can be used to encode time. It can also be useful for other problems in deep learning that are not directly related to time. For a Convolutional Neural Network (CNN), a mere presence of eyes, a nose, and a mouth can be enough to declare something as a face. The relative spatial relations between these components and their orientation does not trigger the network to change its decisions. G.Hinton’s capsule networks idea aims at solving this problem in deep learning.
波的干扰动力学可用于编码时间。 对于深度学习中与时间不直接相关的其他问题,它也可能有用。 对于卷积神经网络(CNN),仅眼睛,鼻子和嘴巴的存在就足以将某物声明为一张脸。 这些组件之间的相对空间关系及其方向不会触发网络更改其决策。 G.Hinton的胶囊网络思想旨在解决深度学习中的这一问题。
SNN, with its neuromorphic hardware, is fit to make use of wave dynamics to solve these issues in AI. However, there is another option to consider before going for the expensive route. Complex numbers are used heavily in physics and engineering to formulate and model wave dynamics. Therefore their usage in deep learning can be a shortcut to teach AI about time.
SNN及其神经形态硬件适合利用波动动力学来解决AI中的这些问题。 但是,在选择昂贵的路线之前,还需要考虑另一种选择。 复数在物理学和工程学中大量使用,以制定和建模波动力学。 因此,它们在深度学习中的使用可以成为教AI时间的捷径。
复数编码波 (Complex numbers to encode waves)
I start with a simple assumption that all the waves we are going to deal with are pure cosine or sinusoid waves of the same frequency, and they differ only in amplitude and phase. I will revisit this assumption later in the discussion. Amplitude and phase can be represented with a complex number.
我从一个简单的假设开始,即我们要处理的所有波都是相同频率的纯余弦或正弦波,它们的幅度和相位不同。 在稍后的讨论中,我将重新讨论该假设。 幅度和相位可以用复数表示。
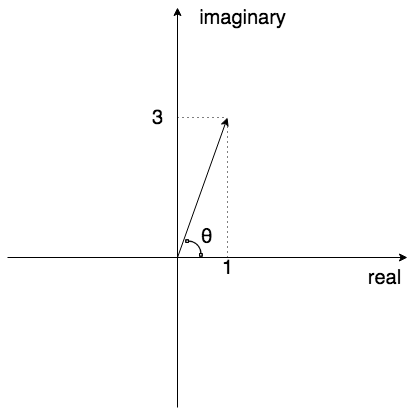
1+3i has an amplitude of √10, which will encode the amplitude. Its phase θ (~ 71.5°) is the phase of the wave, defined in degree distance from some reference point. Now, let’s see what follows if neuronal activations and weights are complex numbers instead of real. This switch is not just a math trick; it changes the way we perceive neural networks.
1 + 3i的振幅为√10 ,它将对振幅进行编码。 它的相位θ(〜71.5°)是波的相位,以距某个参考点的度数定义。 现在,让我们看看如果神经元的激活和权重是复数而不是实数,会发生什么。 这种切换不仅是数学上的窍门; 它改变了我们感知神经网络的方式。
在神经网络中使用复数的含义 (Implications of using complex numbers in neural networks)
The switch from real to complex numbers changes the way neurons excite and inhibit one another. Relative phase differences between incoming signals to a neuron affect its output, even when input amplitudes remain the same. A neuron can inhibit its neighbor by sending a signal that is out of phase with other inputs it receives. (see Figure 3).
从实数到复数的转换改变了神经元相互激励和抑制的方式。 即使输入幅度保持不变,输入到神经元的信号之间的相对相位差也会影响其输出。 神经元可以通过发送与其接收的其他输入异相的信号来抑制其邻居。 (请参见图3)。
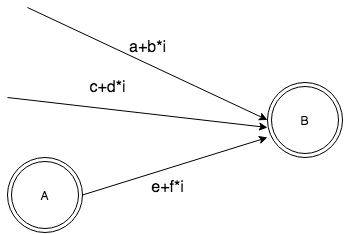
One might argue that the same effect of inhibition is achieved in real-valued networks through edges that bear negative weight. The problem of negative weight is that such an edge inhibits every neuron it connects to, unlike a complex-valued edge that can appear inhibitive to one neuron and excitatory to another. A single edge resulting in different outcomes depending on which neuron it is connected can result in richer data representations.
有人可能会说,通过具有负权重的边在实值网络中可以实现相同的抑制效果。 负权重的问题在于,这种边缘会抑制它连接的每个神经元,而不像复杂值的边缘会抑制一个神经元并刺激另一个神经元。 取决于所连接的神经元的单个边缘会导致不同的结果,从而可以产生更丰富的数据表示形式。
波形输入数据 (Feeding input data in waves)
Input data must also be represented as waves to make full use of complex-valued neural networks. Fourier transform interprets given data as a wave in space and time and represents it as a summation of pure cosine and sinusoid waves in space and time. These constituent pure waves are of different phases, amplitudes, and frequencies. For example, the Fourier transform of a 2-D image — pixel values spread in 2-D space — returns phase and magnitude maps spread in frequency space, whose coordinates are frequency values. By superimposing magnitude (or amplitude) and phase maps, we get a matrix of complex numbers (see Figure 4).
输入数据也必须表示为波,以充分利用复数值神经网络。 傅立叶变换将给定数据解释为时空波,并将其表示为时空中纯余弦波和正弦波的总和。 这些组成的纯波具有不同的相位,幅度和频率。 例如,二维图像的傅立叶变换(在二维空间中散布的像素值)返回在频率空间中散布的相位和幅度图,其坐标是频率值。 通过叠加幅度(或幅度)和相位图,我们得到一个复数矩阵(见图4)。
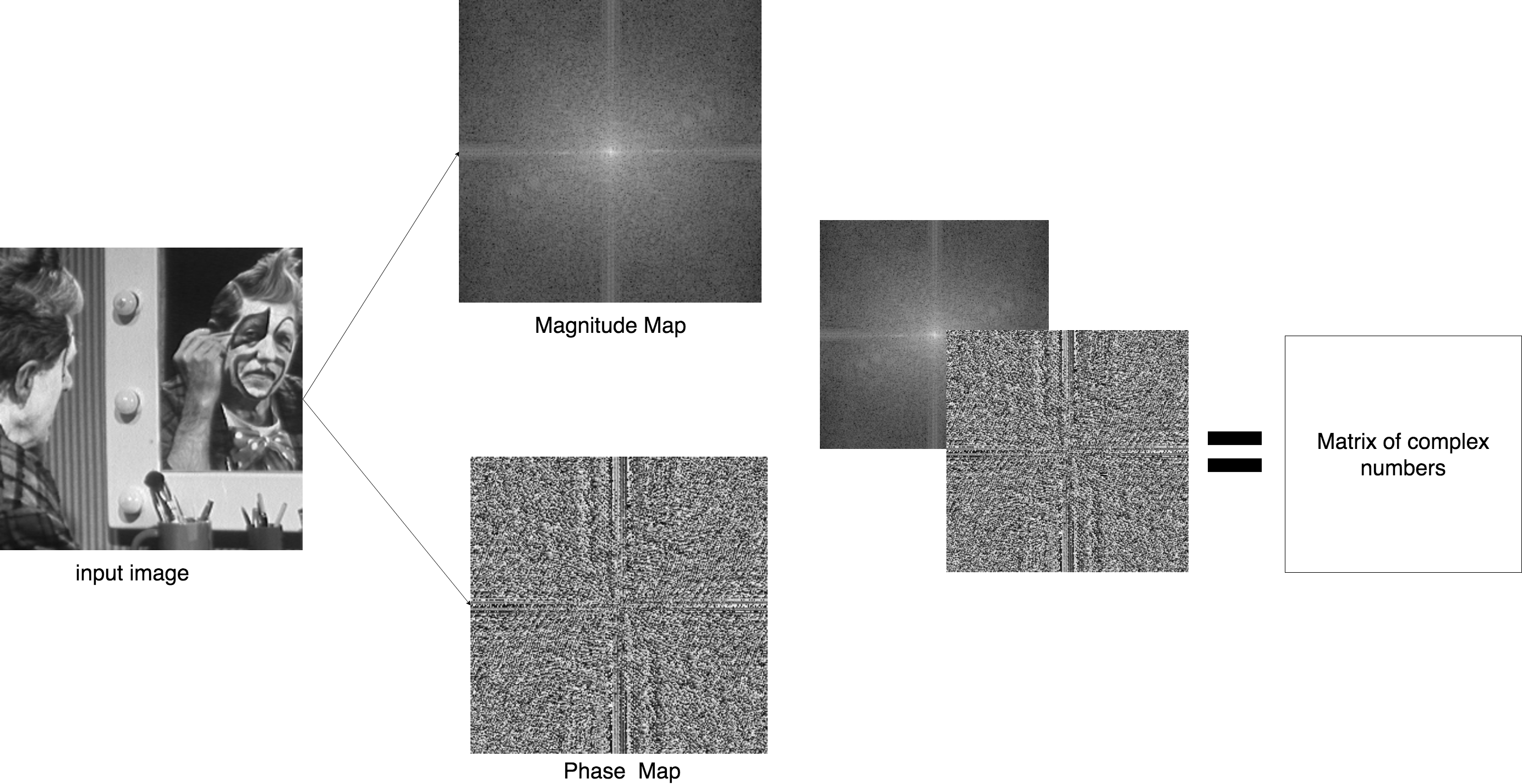
Here, the coordinate of a point [f1,f2] denotes the frequency and the direction of a 2-D pure wave (see Figure 5). The further away the point is from the center, the higher the frequency of the pure wave the point represents. The original image can be reconstructed from amplitude and phase information. There is an ongoing discussion on under what conditions the phase map contains more information about data or vice versa ([1],[2],[3]). This discussion reminds us of the problems that Hinton’s capsule networks aim to solve.
在这里,点[f1,f2]的坐标表示二维纯波的频率和方向(请参见图5)。 该点离中心越远,该点表示的纯波频率越高。 原始图像可以从幅度和相位信息中重建。 关于在什么条件下相图包含更多有关数据的信息,反之亦然([1],[2],[3])。 讨论使我们想起了欣顿胶囊网络旨在解决的问题。
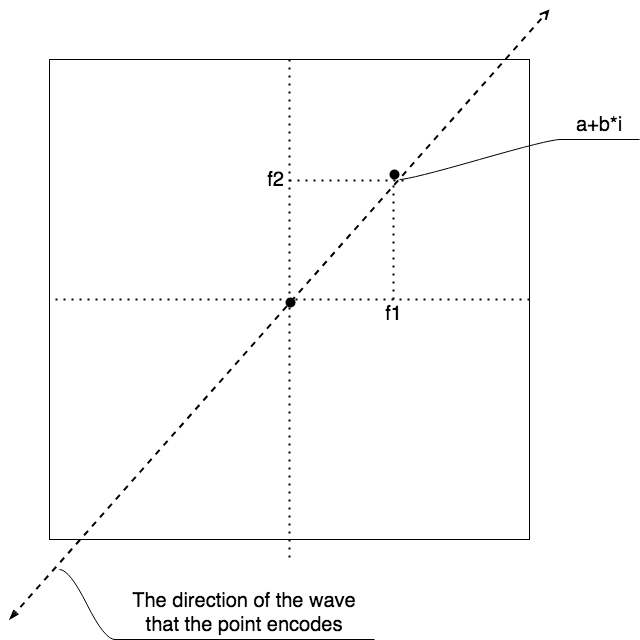
The Fourier transform also solves our problems with temporal data. Instead of appealing to ad-hoc methods of padding, or feeding sparse sequential data to encode relative time distances between points, the Fourier gives us a compact way of encoding these relative timings in the form of phase maps. The Fourier saves us from the trouble of deciding on a sequence length for RNN because it transforms data in the time domain to the data in the frequency domain. The higher frequencies generally correspond to noise, and most information is concentrated in low frequencies. Now, instead of deciding on some arbitrary sequence length, we can decide on a frequency treshold or a spectrum that has an intuitive meaning. One might argue that this way of encoding sequential data might diminish the need for RNNs altogether. Coincidentally, RNN is giving way to transformer networks already.
傅里叶变换也解决了我们的时间数据问题。 傅立叶不是使用临时的填充方法或馈送稀疏的顺序数据来编码点之间的相对时间距离,而是以紧凑的方式以相位图的形式对这些相对时间进行编码。 傅里叶使我们免于为RNN确定序列长度的麻烦,因为它可以将时域中的数据转换为频域中的数据。 较高的频率通常对应于噪声,并且大多数信息集中在低频中。 现在,我们可以决定一个具有直观意义的频率阈值或频谱,而不是决定某个任意的序列长度。 可能有人争辩说,这种对顺序数据进行编码的方法可能会完全消除对RNN的需求。 巧合的是,RNN已经让位于变压器网络。
There is another implication of feeding a neural network a matrix of complex numbers defined as above. Since the coordinate of a point in the matrix denotes frequency, neurons in the first layer will receive data on specific frequency ranges, thereby implicitly encoding frequency information. Frequency (equivalent to firing rate in neuroscience terms), as well as relative timings of the spikes, are believed to be the basis for information coding in biological brains. SNN mimicking natural networks more accurately makes explicit use of frequency in order to code information. A complex-valued neural network, on the other hand, uses frequency only implicitly and makes up for it by using amplitudes explicitly. Here, an analogy to wave-particle duality in physics comes to the surface. The duality in AI is also along the similar lines of being frequency versus amplitude-based computing.
向神经网络提供如上所述定义的复数矩阵还有另一个含义。 由于矩阵中点的坐标表示频率,因此第一层中的神经元将在特定频率范围内接收数据,从而隐式编码频率信息。 频率(相当于神经科学术语的发射速率)以及峰值的相对定时被认为是生物大脑中信息编码的基础。 模仿自然网络的SNN更精确地利用频率来编码信息。 另一方面,复值神经网络仅隐式使用频率,并通过显式使用振幅来弥补频率。 在这里,出现了类似于物理学中的波粒对偶的类比。 AI的对偶性也遵循类似的思路,即基于频率与基于幅度的计算。
结论 (Conclusion)
Spiking Neural Networks is an attempt to address real gaps in the current deep learning paradigm. Proponents of SNN are correct in arguing that behind the rich representational capacity of natural networks lies its wave-based dynamics. However, the application of complex numbers in deep learning can cure the imbalance. There are few papers by well-known figures in AI exploring the use of complex numbers in deep learning, though they have not gained enough attention. I think it is because they mostly focus on practical aspects of it rather than theoretical justifications. In this article, I put forward a theoretical case for complex numbers as an alternative to SNN.
尖刺神经网络(Spiking Neural Networks)试图解决当前深度学习范例中的实际空白。 SNN的支持者认为自然网络的丰富表示能力背后是基于波的动力学,这是正确的。 但是,在深度学习中应用复数可以解决不平衡问题。 尽管没有引起足够的重视,但人工智能领域的知名人物很少有论文探讨深度学习中复数的使用。 我认为这是因为他们大多侧重于实践方面,而不是理论上的依据。 在本文中,我提出了复数作为SNN替代方法的理论案例。
翻译自: https://medium.com/@nariman.mammadli/its-time-for-ai-to-perceive-time-9ffdecd2ce91