深度学习决策支持 时空预测
Digital transformation is one of the top priorities for industrial companies. The largest players are already moving in this direction, for many years continuously working to improve production efficiency and launching large-scale optimisation programs. The adoption of new analytical systems for industrial enterprises ships under a lot of different names. They’re called advanced analytics or digital innovation, and at their core, the technology could be summarised under artificial intelligence. In all cases, the efforts to utilise AI models or data analytics systems are part of a bigger digital transformation effort of the progressing companies.
d igital转型是工业企业的首要任务之一。 最大的参与者已经朝着这个方向前进,多年来不断致力于提高生产效率并启动大规模的优化程序。 工业企业采用新的分析系统的船名很多不同。 它们被称为高级分析或数字创新,其核心是可以在人工智能下总结的技术。 在所有情况下,利用AI模型或数据分析系统的努力都是不断发展的公司进行更大的数字化转型的一部分。
In an industrial context, such strategies for cost-saving and process optimisation often start from pilot projects, or top management directives for digital change guide them. In general, changes in processes or investments in capital-intensive and competitive industries require large sums of money. Traditional capital expenditures usually stretch over a long period, so a current financial standing may not allow for a complete physical overhaul of the plants or facilities. These high costs lead to the search for cheaper alternatives. Investments in digital and artificial intelligence solutions could be a magnitude smaller.
在工业环境中,这种节省成本和流程优化的策略通常从试点项目开始,或者由数字化变更的最高管理指令指导它们。 一般而言,资本密集型和竞争性行业的流程或投资变化需要大量资金。 传统的资本支出通常会持续很长时间,因此,当前的财务状况可能无法对工厂或设施进行全面的物理检查。 这些高成本导致寻找更便宜的替代品。 在数字和人工智能解决方案上的投资可能要小得多。
When creating a transformation strategy, you can find a place to use AI in all operations. Some of the scenarios provide the opportunity for a quick return on investment, such as decision support systems that accompany technological processes, while others require lengthy testing. In this article, we will look at popular and promising use cases of machine learning applications in industry.
创建转换策略时,您可以找到在所有操作中都使用AI的地方。 一些方案提供了快速获得投资回报的机会,例如伴随技术流程的决策支持系统,而其他方案则需要冗长的测试。 在本文中,我们将研究行业中机器学习应用程序的流行和有前途的用例。
Independent of the specific industry, a trend for more cutting-edge artificial intelligence is showing in the last three years, especially in the field of deep learning. Collaborations with technology providers and machine learning startups are more often found. The focus of researchers has shifted from big data analysis to an edge computing. However, industrial companies are still reluctant to adopt advanced data analysis methods.
在过去三年中,尤其是在深度学习领域,独立于特定行业的趋势是,越来越先进的人工智能出现了趋势。 与技术提供商和机器学习初创公司的合作更为频繁。 研究人员的重点已经从大数据分析转移到边缘计算。 但是,工业公司仍然不愿意采用高级数据分析方法。
Benefits from AI implementation can be found in general market trends. In a new report, Meticulous Research notes that the AI in manufacturing market is expected to grow at a CAGR of 39.7% from 2019 to 2027 to reach $27 billion by 2027. The AI in Oil&Gas market was valued by Mordor Intelligence at $2 billion in 2019 and is expected to reach $3.81 billion by 2025, at a CAGR of 10.96% over the forecast period 2020–2025.
人工智能实施的好处可以从总体市场趋势中找到。 Meticulous Research在一份新报告中指出,从2019年到2027年,制造业市场的AI预计将以39.7%的复合年增长率增长,到2027年将达到270亿美元.Mordor Intelligence在2019年对石油和天然气市场的AI估值为20亿美元预计到2025年将达到38.1亿美元,在2020-2025年的预测期内复合年增长率为10.96%。
需求预测 (Demand prediction)
We start with a look at Big River Steel from Arkansas, USA. This steelmaking startup teamed up with an AI consulting firm to improve its profit margins and processes. They have chosen a data-centric approach that utilises historical demand for steel, macroeconomic data, and activities of their customers. They developed a demand signalling application which enhances traditional big data application with machine learning algorithms. This system consists of three parts. Demand sensing, statistical demand, and consensus demand lead to better supply management and a decrease in safety stock. Due to wide fluctuations of demand in the steel industry, new analytics offer great potential.
我们首先看一下美国阿肯色州的Big River Steel 。 这家炼钢初创公司与一家AI咨询公司合作,以提高其利润率和流程。 他们选择了以数据为中心的方法,该方法利用了对钢铁的历史需求,宏观经济数据以及客户的活动。 他们开发了一种需求信号应用程序,通过机器学习算法增强了传统的大数据应用程序。 该系统包括三个部分。 需求感知,统计需求和共识需求导致更好的供应管理和安全库存的减少。 由于钢铁行业需求的广泛波动,新的分析技术提供了巨大的潜力。
More insights are given by the automotive industry. A case study of the automotive industry explains soft-computing approaches for demand prediction. The used models are fuzzy logic and Delphi method, plus time-series ANN (artificial neural network). This structure combines expert opinions with artificial intelligence.
汽车行业给出了更多的见解。 汽车行业的案例研究说明了用于需求预测的软计算方法。 使用的模型是模糊逻辑和Delphi方法,以及时间序列ANN (人工神经网络)。 这种结构将专家意见与人工智能相结合。
Automotive is strong in demand prediction, as the Volkswagen AG Data:Lab Munich shows. They initiated over 100 dedicated projects to forecast sales for different products and regions. The development of the necessary tools that utilise context-based data like growth forecasts, economic sanctions, and weather conditions is one of their key efforts. According to the estimates of the consulting company Capgemini, large manufacturers of structurally complex auto components can increase profits up to 16% due to the large-scale implementation of artificial intelligence.
大众汽车股份公司数据:慕尼黑实验室显示,汽车行业对需求的预测很强。 他们发起了100多个专门项目,以预测不同产品和地区的销售额。 利用基于上下文的数据(例如增长预测,经济制裁和天气状况)开发必要工具是他们的主要工作之一。 根据咨询公司Capgemini的估计,由于人工智能的大规模实施,结构复杂的汽车零部件的大型制造商可以将利润提高多达16%。
Another vendor, Predictive Layer offers a solution for power consumption prediction. They developed a dynamic pricing engine that includes demand and supply elasticity analysis of the client company. According to the company, it was possible to form a fairly accurate forecast of consumption for the next day. The declared savings of the annual electricity purchases in the national electricity markets of the EU is more than $45 million.
另一个供应商,预测层提供了功耗预测解决方案。 他们开发了一个动态的定价引擎,其中包括对客户公司的需求和供应弹性的分析。 据该公司称,有可能对第二天的消费量做出一个相当准确的预测。 在欧盟国家电力市场中,宣布的年度购电节省了超过4500万美元。
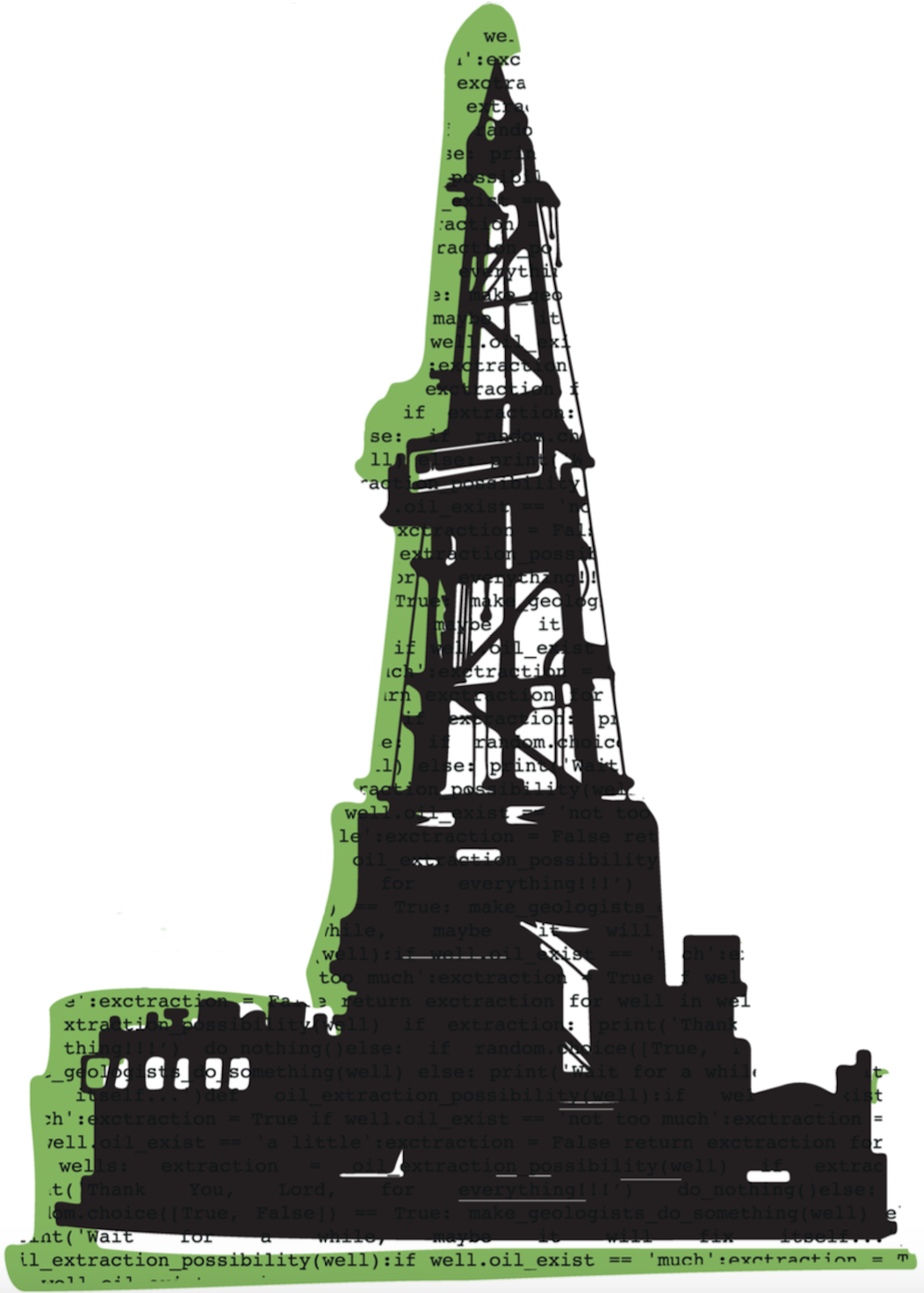
Oil & gas industry is using conventional and artificial intelligence-based models for energy consumption forecasting. And they use a broad spectrum of models for forecasting different horizons. A review from 2019 showed that conventional models are preferred for the yearly energy consumption forecasting at the national level. AI-based models could be applied in full-scale in all forecasting horizons and areas. However, in the short-term forecast regression models and time series models can compete in forecast accuracy with AI-based models. As these are white-box models, they are superior in explainability of the relationship between consumption data and influencing factors. The review also showed deep learning models were only used sparsely so far, and their performance and robustness need to be further validated. This lack of use is that even though some researchers are indicating the superiority of deep learning models.
石油和天然气行业正在使用基于常规和人工智能的模型进行能耗预测。 他们使用广泛的模型来预测不同的前景。 从2019年开始的回顾显示,传统模型更适合用于国家一级的年度能耗预测。 基于AI的模型可以在所有预测范围和区域中全面应用。 但是,在短期预测回归模型和时间序列模型中,预测模型可以与基于AI的模型竞争。 由于这些是白盒模型,因此在解释消费数据与影响因素之间的关系方面具有优越性。 审查还显示,到目前为止,深度学习模型仅被稀疏使用,它们的性能和鲁棒性需要进一步验证。 缺乏使用的是,即使一些研究人员指出了深度学习模型的优越性。
风险管理和预测性维护 (Risk management and predictive maintenance)
The review shows a steep increase in publication on risk management AI in the last ten years with doubling it from 2016 to 2018. The leading industries for the application of AI for risk management are automotive and construction. The Oil&Gas, Mining, and Energy industries are on par. A safety science review from 2020 gives a lot of insights into the use of artificial neural networks in the risk identification phase during risk assessments.
吨他回顾表明出版物的急剧增加对风险管理的AI在过去十年与AI的风险管理中的应用的主导产业倍增它从2016年至2018年是汽车和建筑。 石油,天然气,采矿和能源行业持平。 2020年的安全科学评论对风险评估期间的风险识别阶段中人工神经网络的使用提供了很多见解。
Risk assessment is defined here as “the overall process of risk identification, risk analysis and risk evaluation.” The two main data sources for the application of artificial intelligence are learning from textual data and numerical data. The textual data for risk management consists of written reports of safety incidents or accidents. Large structured questionnaires or unstructured free text are the two forms in which the data are available. So, this kind of data source is ideal for enterprise AI analytics. The numerical data comes in the form of accident frequencies or other time-series data. Here also a wide variety of models are applied. An emphasis lies on classification and regression trees (CART), decision trees and SVM.
风险评估在此定义为“风险识别,风险分析和风险评估的整个过程。” 人工智能应用的两个主要数据源是从文本数据和数字数据中学习。 风险管理的文本数据包括安全事件或事故的书面报告。 大型结构化问卷或非结构化自由文本是可获得数据的两种形式。 因此,这种数据源非常适合企业AI分析。 数字数据以事故频率或其他时间序列数据的形式出现。 在这里也应用了各种各样的模型。 重点在于分类和回归树(CART),决策树和SVM。
A practitioners perspective shows a Petroleum System Risk Assessment as an available service from a technology company that leverages exploration workflows. They help robust decision making with artificial neural networks. They focus on enhancing existing numerical modelling with the determination of the uncertainties, and they create maps of risks at exploration scale and in time frames compatible with operations. They use expert knowledge in combination with continuously updated regression and clustering models.
从业人员的观点将石油系统风险评估显示为利用勘探工作流程的技术公司提供的服务。 它们有助于通过人工神经网络做出可靠的决策。 他们专注于通过确定不确定性来增强现有的数值模型,并在勘探规模和与操作兼容的时间范围内创建风险图。 他们将专业知识与不断更新的回归和聚类模型结合使用。
Predictive maintenance is a hot topic now, but development of this area requires high quality data and a systemic restructuring of maintenance and repair processes. In the Oil&Gas industry, these anomaly detection systems are significant applications of machine learning. The widespread sensors control hardware fosters the use of this kind of analytics. Defect on turbo machines, pumps, and motors could be recognised early, and so it’s possible to prevent further losses by converting unplanned repairs to scheduled ones.
预测性维护现在是一个热门话题,但是该领域的发展需要高质量的数据以及维护和维修过程的系统重组。 在石油和天然气工业中,这些异常检测系统是机器学习的重要应用。 广泛使用的传感器控制硬件促进了这种分析的使用。 涡轮机,泵和电动机上的缺陷可以及早发现,因此可以通过将计划外的维修转换为计划内的维修来防止进一步的损失。
Benefits applying data analysis were clearly demonstrated in creation of oil and gas platform management system by BCG. Obtained operational data helped to prevent shutdowns of dynamic equipment, and assess the achievable potential of its operation. In addition, process control opens up new possibilities for dealing with production bottlenecks.
BCG创建的石油和天然气平台管理系统清楚地证明了应用数据分析的好处。 获得的运行数据有助于防止动态设备停机,并评估其可实现的运行潜力。 此外,过程控制为处理生产瓶颈开辟了新的可能性。
在技术过程中及早发现异常 (Early anomaly detection in a technological process)
Undoubtedly, one of the key priorities for all industries is safety, exclusion of humans from hazardous environment, online operation monitoring and tracking violations of equipment operating modes, as well as prompt response to key risks.
ündoubtedly,为所有行业的主要重点之一是安全,从危险的环境中,在线运行监控和跟踪侵权行为设备工作模式的人的排斥,以及对关键风险Swift作出React。
One of an artificial intelligence system’s inherent capability is anomaly detection. It could be seen as one of the main objectives of the Industrial IoT. The identification of abnormal behaviour within the pool of collected data. Known process patterns could be interrupted by rare events that are usually undetectable by a human expert. As an example for the industrial application of such an anomaly, a leaking connection pipe could be representative. In an unfortunate case, this defect could lead to the shutdown of an entire production line. With the huge data streams inside production plants, the hunt for anomalies by manual inspection seems unreasonable.
人工智能系统的固有能力之一就是异常检测。 可以将其视为工业物联网的主要目标之一。 在收集的数据池中识别异常行为。 已知的过程模式可能会被人类专家通常无法检测到的罕见事件打断。 作为这种异常的工业应用的示例,可以使用泄漏的连接管。 在不幸的情况下,此缺陷可能导致整个生产线关闭。 由于生产工厂内部存在巨大的数据流,因此通过人工检查来寻找异常现象似乎是不合理的。
Ukrainian technology provider Sciforce shares a case study of their manufacturing client who wanted to speed up regular processing algorithms and to increase the system stability. They set up a commercial anomaly detection process which includes the anomaly detection itself and the prediction of future anomalies. As a model for detection, they used autoencoders. These encoders map given data into a hidden representation. Then they attempt to restore the original input from this internal representation. For the prediction part, a recurrent neural network (RNNs) was in service. This model could make accurate predictions for up to 10 minutes ahead.
乌克兰技术提供商Sciforce分享了他们的制造客户的案例研究,他们希望加快常规处理算法的速度并提高系统稳定性。 他们建立了一个商业异常检测过程,其中包括异常检测本身以及对未来异常的预测。 作为检测的模型,他们使用了自动编码器。 这些编码器将给定的数据映射为隐藏的表示形式。 然后,他们尝试从此内部表示形式恢复原始输入。 对于预测部分,使用了递归神经网络(RNN)。 该模型可以在长达10分钟的时间内做出准确的预测。
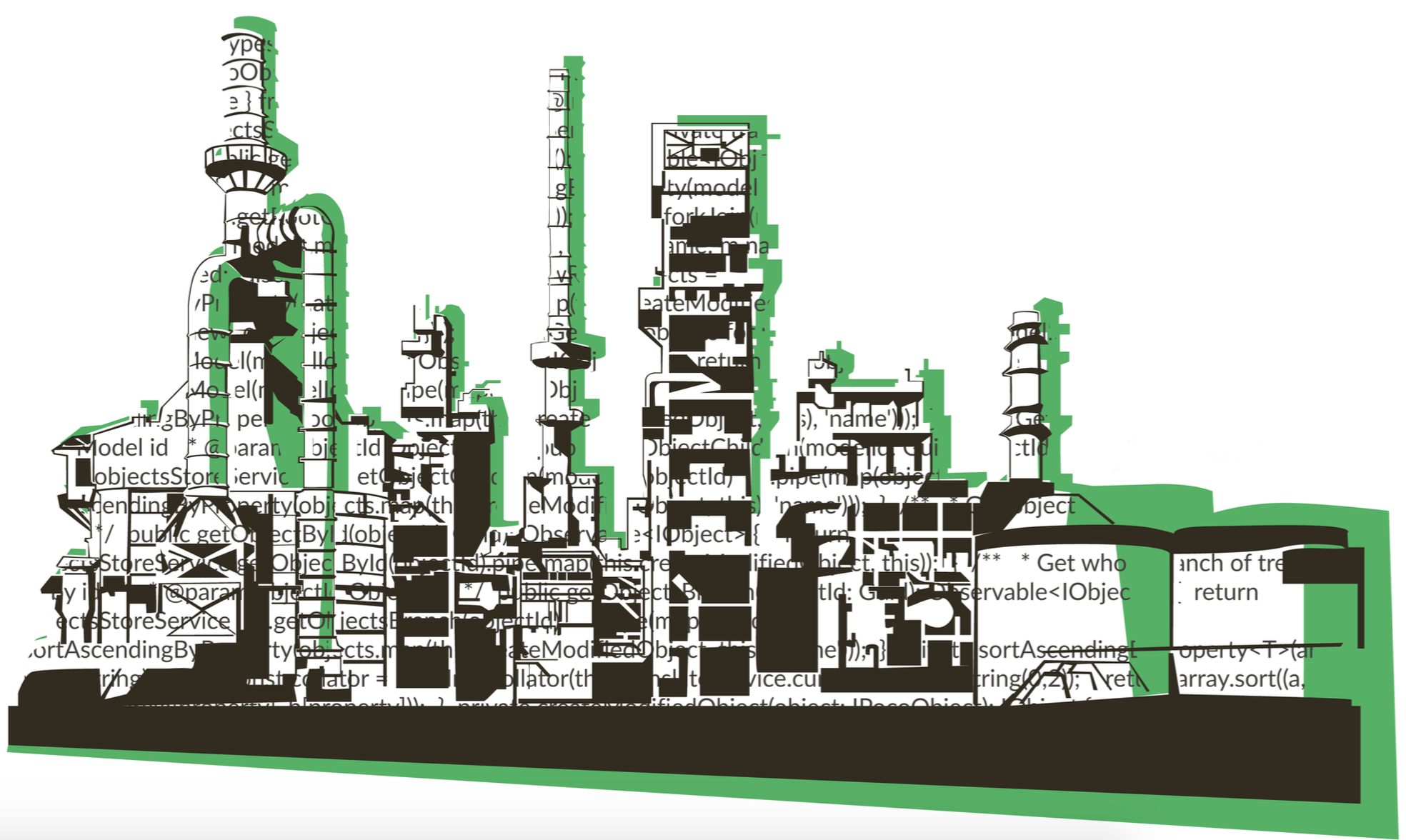
价钱 (Pricing)
The epidemic-related crisis has further exacerbated the long-overdue need for industrial digitalisation. Due to negative oil prices, oil companies found themselves in an extremely unstable environment, forcing them to rethink their strategies and operations. When we look at the supply chain management of Oil&Gas industry, there are several points where artificial intelligence could be applied. AI helps to predict market prices of crude oil and finished products giving more insight to adjust a pricing policy.
吨他流行病有关的危机进一步加剧了对工业数字化的期待已久的需求。 由于油价下跌,石油公司发现自己处于极端不稳定的环境中,迫使他们重新考虑其战略和运营。 当我们查看石油和天然气行业的供应链管理时,可以在几个方面应用人工智能。 人工智能有助于预测原油和成品的市场价格,从而提供更多洞察力来调整定价政策。
The research on AI-based pricing models is an ongoing effort. A review from 2015 shows that all classical artificial intelligence models are used for oil price forecasting, from artificial neural networks, support vector machines, wavelet, genetic algorithms to hybrid systems. The researchers concluded that due to the complexity of the oil price influential factors, the models are very limited. There are no single indicators driving oil prices. And the needed input data is still to discover.
基于AI的定价模型的研究是一项持续的工作。 2015年的评论显示,从人工神经网络,支持向量机,小波,遗传算法到混合系统,所有经典的人工智能模型都可用于油价预测。 研究人员得出结论,由于石油价格影响因素的复杂性,模型非常有限。 没有任何单一指标可以推动油价上涨。 所需的输入数据仍有待发现。
Despite the unpredictability of oil prices, the slightest advantage needs to be used in a fierce competition. The expensive arms race forces traders to keep up without clear benefits of the solutions. An interesting development comes from the commodities market. There, the price risk management could be changed soon through new technology that fundamentally alters how small and mid-size enterprises handle their commodity risk. AI trading systems with autonomous farming and mining operations could minimise price risks.
尽管石油价格难以预测,但在激烈的竞争中仍需利用一点优势。 昂贵的军备竞赛迫使交易者在解决方案没有明显好处的情况下继续前进。 大宗商品市场是一个有趣的发展。 在那里,价格风险管理可能会很快改变 从根本上改变中小企业处理商品风险的新技术。 具有自主农业和采矿业务的AI交易系统可以将价格风险降至最低。
后勤 (Logistics)
The future management of raw materials, logistics, and transportation are closely connected to the concept of the smart factory. When looking at the industries that lead the digital transformation process towards complete digital production, the automotive industry is again the digital master. They are capable of increasing their profits through digitisation efforts at double the margin of beginners. For logistics, the connected plants and factories are key to successful projects. At the scale of global acting companies, the existing communication channels need to be used for a unified digital logistics system.
吨他未来的原材料,物流和运输管理紧密连接到智能工厂的概念。 当考察引导数字化转型过程实现完整数字化生产的行业时,汽车行业再次成为数字化大师。 他们有能力通过数字化努力增加利润,这是初学者的两倍。 对于物流而言,相连的工厂是成功项目的关键。 在全球代理公司的规模上,现有的沟通渠道需要用于统一的数字物流系统。
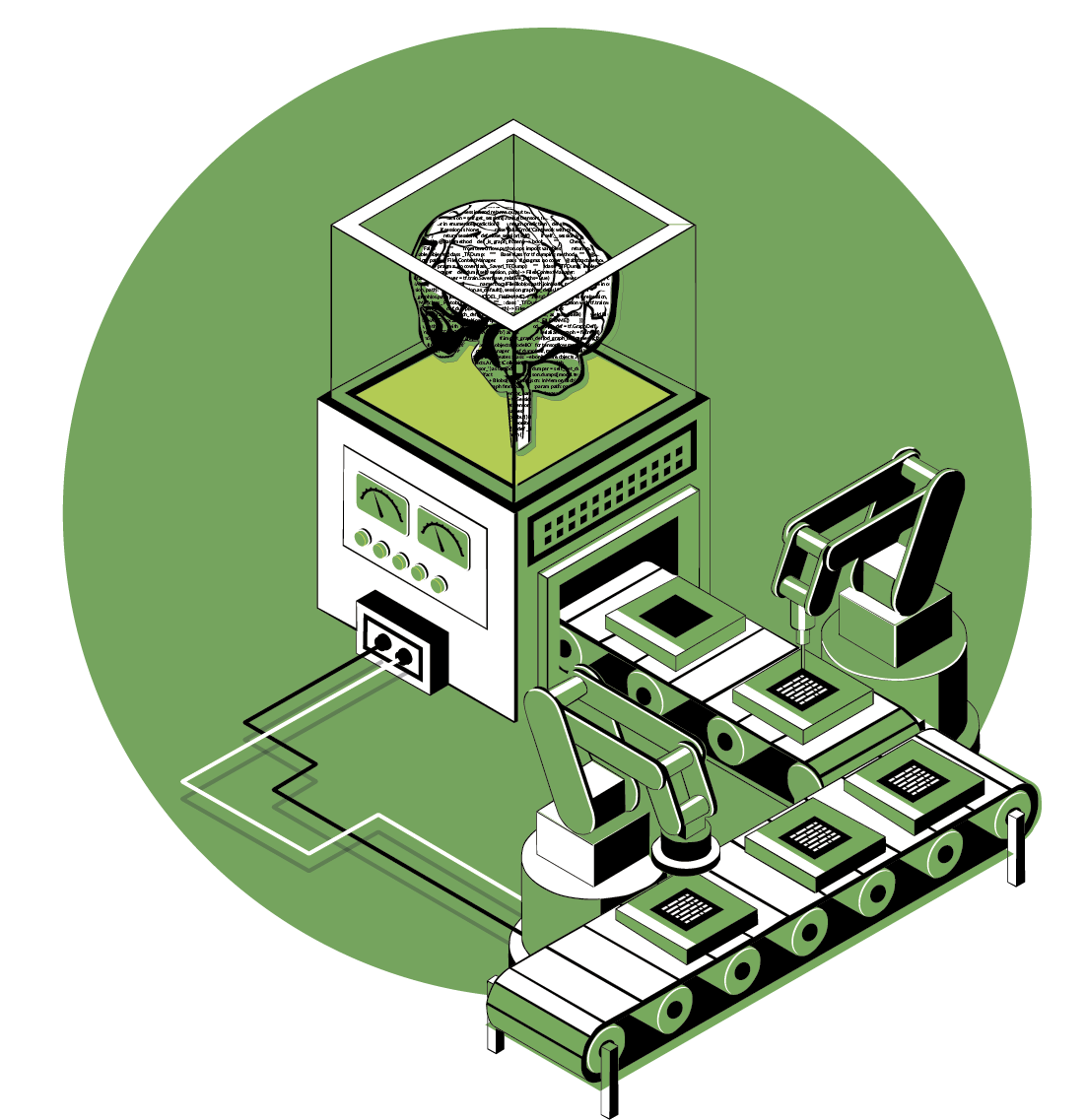
One example of a smart factory solution already in service is general electric’s brilliant factory program. GE powers 20 Brilliant factories worldwide and links real-time data from design, engineering, manufacturing, supply chain, distribution, and service activities. They altogether build one interconnected intelligent system. DHL and IBM describe the function of AI for logistics as an interconnection to robotic process automation (RPA) where AI learns to copy and improve processes based on data provided by RPA. Artificial intelligence systems become the assistant of logistics based on human decisions. They can make fast judgements and interact with humans. Beyond RPA the AI systems help logistics to shift its operating model from reactive behaviour for forecasting and proactive operations with predictive analytics.
通用电气出色的工厂计划就是一个已经投入使用的智能工厂解决方案的例子。 GE为全球20个出色的工厂提供动力,并链接来自设计,工程,制造,供应链,分销和服务活动的实时数据。 他们总共建立了一个互连的智能系统。 DHL和IBM将AI的物流功能描述为与机器人流程自动化(RPA)的互连,其中AI学会根据RPA提供的数据来复制和改进流程。 人工智能系统成为基于人为决策的物流助手。 他们可以做出快速判断并与人类互动。 除了RPA,AI系统还帮助物流将其操作模型从被动行为转变为预测,并通过预测分析进行主动操作。
生产过程 (Production processes)
From robots to cobots, smarter automation, with artificial-intelligence-driven management and control systems AI, is changing the processes in the factory. An example of more process-driven AI is the optimisation of additives consumption is a lot of industrial processes. Despite the fact that most processes have undergone a year’s long traditional optimisation process, machine learning can push these optimisations even further. Complex processes feature still a degree of uncertainty. So expensive additives were used for a broad safety margin. AI can not inspect deeper initial material composition, quality of raw materials, readings from hundreds of sensors and come up with meaningful reasoning. After training the AI model, it can accurately predict the parameters of the final product.
f光盘机器人cobots ,更智能的自动化,具有人工智能驱动的管理和控制系统的AI,正在改变工厂的过程。 更多由流程驱动的AI的示例是许多工业流程中添加剂消耗的优化。 尽管大多数过程都经历了长达一年的传统优化过程,但机器学习可以将这些优化进一步推进。 复杂的过程仍具有一定程度的不确定性。 如此昂贵的添加剂被用于广泛的安全范围。 AI无法检查更深的初始材料成分,原材料质量,数百个传感器的读数,也无法提出有意义的推理。 训练AI模型后,它可以准确地预测最终产品的参数。
An example of the application of such systems is the optimisation of the additives use in many industrial processes, for example, the use of ferroalloys in metallurgy. The case of NLMK is distinctive, revealing the capabilities of machine learning in predicting the chemical composition, when adding certain materials. People are often reinsured and use expensive additives in excess to ensure certain properties of the product. The use of machine learning methods helps to carry out a deeper analysis of the initial composition of the material, the quality of raw materials, streaming data from hundreds of sensors, to predict the properties of the output product and recommend the necessary actions.
此类系统的应用示例是优化许多工业过程中使用的添加剂,例如,冶金中铁合金的使用。 NLMK的案例很独特,它在添加某些材料时揭示了机器学习预测化学成分的能力。 人们通常会被重新保险,并过量使用昂贵的添加剂以确保产品的某些性能。 机器学习方法的使用有助于对材料的初始成分,原材料的质量,来自数百个传感器的数据流进行更深入的分析,以预测输出产品的性能并建议采取必要的措施。
In 2019, Accenture presented a financial model for a typical chemical plant AI implementation. In their example, a business with $11.3 billion in annual revenue could increase its bottom line by 10%. The technology provider bitrefine offers a general solution for a broad range of processes. With a prepared set of machine learning tools, ranging from classification, cluster analysis, regression analysis, structured prediction to reinforcement learning, they serve whole process industries. They promise to optimise ore beneficiation, gas processing, oil refinery, chemical industry, plastics industry, glass manufacturing, and semiconductor manufacturing processes. When, through digitalisation, we can increase the speed of steel casting or reduce the amount of scrap, this is a direct income increase.
在2019年,埃森哲为典型的化工厂AI实施提供了财务模型。 在他们的例子中,年收入113亿美元的业务可以将其底线提高10%。 技术提供商bitrefine为广泛的流程提供了通用的解决方案。 借助一整套准备就绪的机器学习工具,从分类,聚类分析,回归分析,结构化预测到强化学习,它们为整个流程行业提供服务。 他们承诺优化选矿,气体处理,炼油厂,化学工业,塑料工业,玻璃制造和半导体制造Craft.io。 当通过数字化可以提高铸钢速度或减少废品数量时,这就是直接的收入增加。
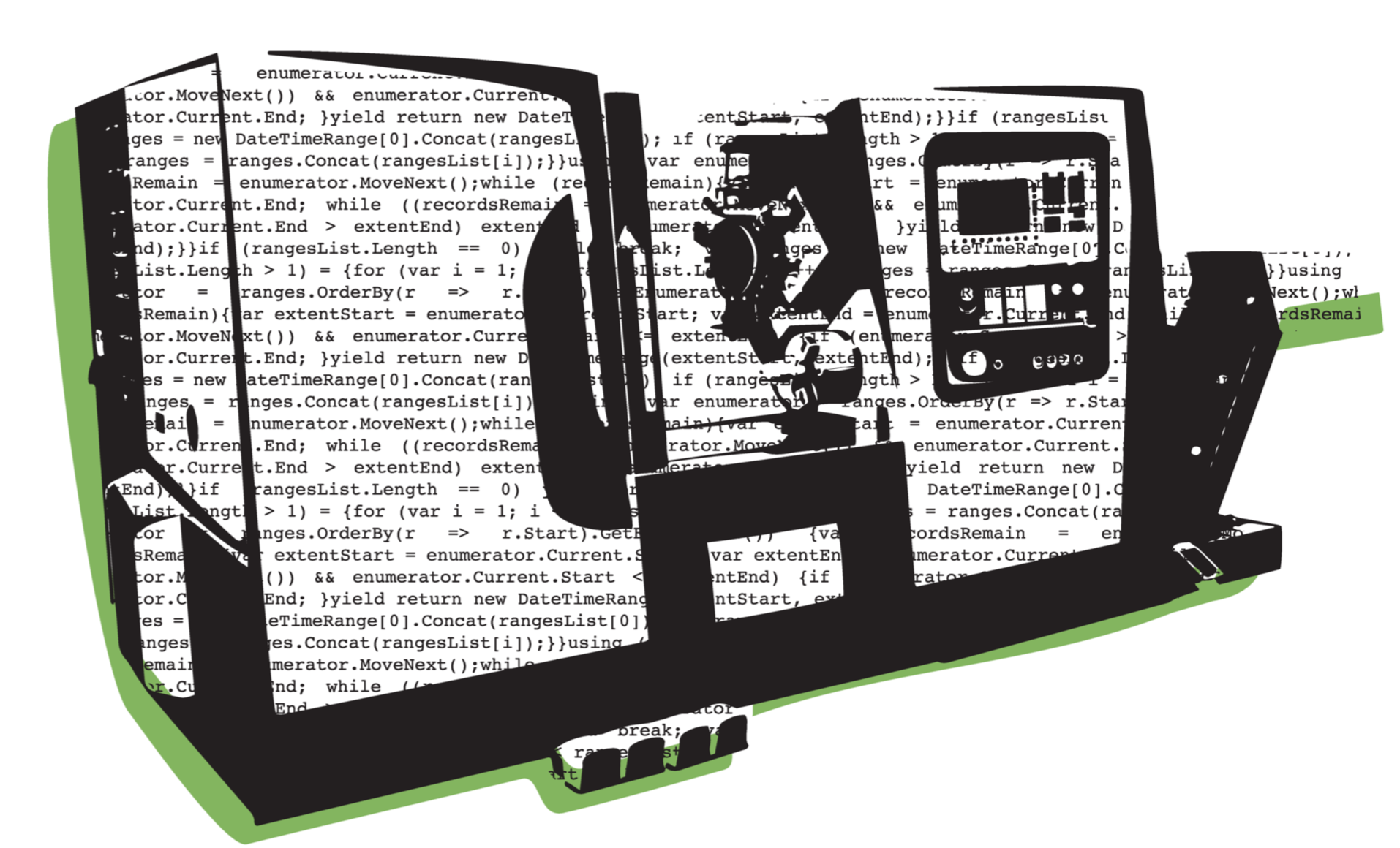
质量控制 (Quality control)
Decision support systems also include quality control, which help to visually control the properties of products and build the logic on working with anomalies after. Here, AI is again the core of such systems. Using deep learning techniques, AI can detect defects better and more accurately than humans. According to McKinsey, AI can be used for automated quality testing. So, productivity could increase up to 50%. Advanced image recognition techniques for visual inspection and fault detection can increase the defect detection rates by up to 90%. This increase is a significant improvement over manual inspection.
d ecision支撑系统还包括质量控制,这有助于在视觉上控制产品的性能,并建立与后异常工作的逻辑。 在这里,人工智能再次成为此类系统的核心。 使用深度学习技术,人工智能可以比人类更好,更准确地检测缺陷。 根据麦肯锡的说法,人工智能可以用于自动化质量测试。 因此,生产率可以提高高达50%。 用于视觉检查和故障检测的高级图像识别技术可以将缺陷检测率提高多达90%。 与手动检查相比,此增加是一项重大改进。
The main approach for visual inspection is a supervised learning algorithm-based system. Here deep neural networks learn to differentiate between good and defective products. The visual system is trained with views from different perspectives, so previously unknown defect types can be identified by employing semi-supervised learning. In this approach, both labelled and unlabelled data is used.
视觉检查的主要方法是基于监督学习算法的系统。 在这里,深度神经网络学会了区分好产品和有缺陷的产品。 视觉系统使用不同角度的视图进行训练,因此可以通过半监督学习来识别以前未知的缺陷类型。 在这种方法中,既使用标记数据也使用未标记数据。
This visual inspection and quality assurance can be used for a large variety of products. The range reaches from machined parts, solar panels, painted car bodies to or textured metal surfaces. Practical examples for automated visual inspection are the approaches by DevisionX. They offer solutions for the automotive industry. Here an industrial camera or sensor is detecting the defects. It can be used to inspect for welding, stamping, assembly, or size conformity. The captured image is analysed through machine learning algorithms, and the system can take action and delivers real-time and customised reports.
这种外观检查和质量保证可用于多种产品。 范围从机械零件,太阳能电池板,涂漆的车身到有纹理的金属表面。 自动视觉检查的实际示例是DevisionX的方法。 他们为汽车行业提供解决方案。 在这里,工业相机或传感器正在检测缺陷。 它可用于检查焊接,冲压,装配或尺寸是否合格。 通过机器学习算法对捕获的图像进行分析,系统可以采取行动,并提供实时和自定义的报告。
新的实际应用胜过传统的人工智能 (New practical applications outperform traditional artificial intelligence)
The use of new deep neural networks and other new machine learning concepts have emerged in recent years, and their application is thriving. But they’re widespread in other industries than the heavy industries. Here in Oil&Gas, mining, manufacturing, and metallurgy and metalworking more traditional AI still prevail. And the automotive industry drives the adoption of the newest models.
近年来出现了新的深度神经网络和其他新的机器学习概念的使用,并且它们的应用正在蓬勃发展。 但是它们在重工业以外的其他行业中也很普遍。 在石油,天然气,采矿,制造,冶金和金属加工领域,更传统的AI仍然流行。 汽车行业推动了最新车型的采用。
The different applications show that all existing artificial intelligence models are used over all the industries. However, most of them are still in pilot phases, or they were promoted by technology providers. The vastly available stockpile of data is only slowly becoming available for new artificial intelligence projects.
不同的应用程序表明,所有现有的人工智能模型已在所有行业中使用。 但是,其中大多数仍处于试验阶段,或者它们是由技术提供商推动的。 大量可用的数据储备仅逐渐变得可用于新的人工智能项目。
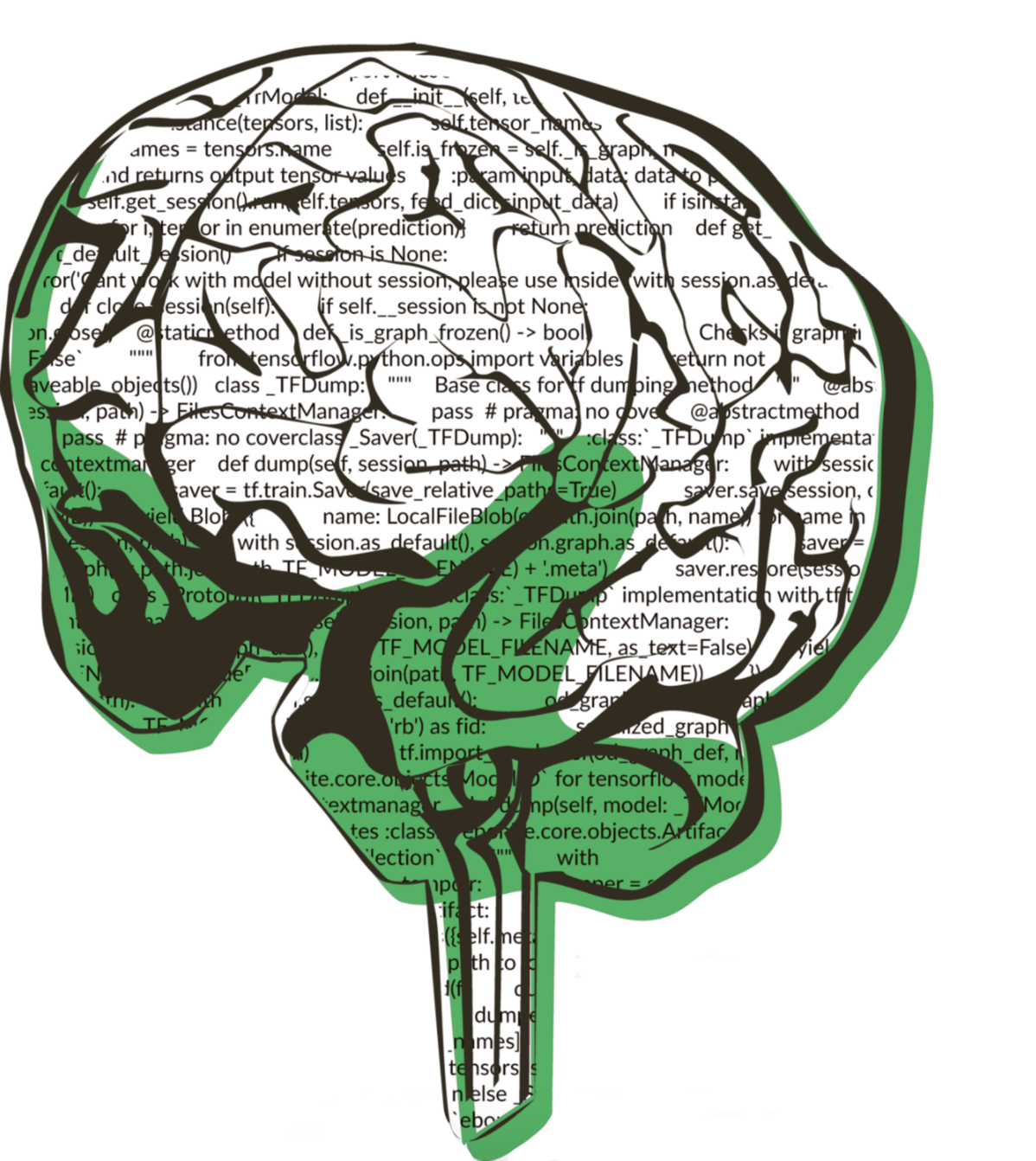
Big companies like Royal Dutch Shell PLC, Tata Steel Europe Ltd., General Electric Company, Volkswagen Group, and Bayerische Motoren Werke AG are promoting huge enterprise-wide digital transformation. These strategies include a lot of smaller AI projects that try to grab the low hanging fruits first.
像荷兰皇家壳牌公司,塔塔钢铁欧洲有限公司,通用电气公司,大众汽车集团和德国巴伐利亚电机公司这样的大公司都在推动整个企业范围的数字化转型。 这些策略包括许多较小的AI项目,这些项目试图首先抢占先机。
Academic research overviews show the maturity of the new AI technologies and the reluctance of the industry for adoption. Some startups are showing the successful implementations of cutting-edge technology and are so leading the way.
学术研究概述表明,新的AI技术已经成熟,并且业界不愿意采用。 一些初创公司展示了尖端技术的成功实施,因此处于领先地位。
_____
_____
This article was originally published in AI almanac.
本文最初发表在AI年鉴中。
深度学习决策支持 时空预测