数据科学数据分析
“According to QuanticMind, 97% of leaders believe that the future of marketing lies in the ways that digital marketers work alongside machine learning-based tools.” — Digital Marketing Institute
“据QuanticMind称,97%的领导者认为营销的未来在于数字营销人员与基于机器学习的工具一起工作的方式。” — 数字营销学院
WORKING WITH CRM (B2B) CAMPAIGNS
使用CRM(B2B)广告系列
In B2B marketing, enterprise companies use Customer Relationship Management (CRM) software such as Salesforce, Oracle, and SugarCRM to keep records of contacts in business partners who maintain key roles in decision-making and purchasing. This data can be used in machine learning environments with marketing analytic software to chart the effectiveness of advertising campaigns to specific markets.
在B2B营销中,企业公司使用诸如Salesforce,Oracle和SugarCRM之类的客户关系管理(CRM)软件来保留在决策和购买中扮演关键角色的业务合作伙伴中的联系记录。 可以将这些数据与营销分析软件一起用于机器学习环境中,以绘制针对特定市场的广告活动的效果图。
- The inclusion of personalized events like outbound calls and email newsletters in the sales channel can be recorded in charts to analyze results in future purchases. 销售渠道中包括个性化事件(如去电和电子邮件新闻通讯),可以记录在图表中,以分析将来的购买结果。
- Attendance at trade shows and promotional events are noted with time-stamps in marketing analytics focusing on sales cycles. 参加营销展览和促销活动时会以注重销售周期的营销分析时间戳记。
A large challenge in B2B marketing is attributing the decision-making within a sale, since there may be several people involved in a single purchase. The personalization of B2B marketing can scale to one million events per contact in the generation of charts and analytics from programmed variables.
B2B营销中的一大挑战是在销售中做出决策,因为单个购买中可能涉及多个人。 B2B营销的个性化可以在通过编程变量生成图表和分析时将每个联系人扩展到一百万个事件。
WORKING WITH E-COMMERCE (B2C) CAMPAIGNS
使用电子商务(B2C)广告系列
In B2C marketing analytics, there is less difficulty in attributing the decision-making for a sale to a single person. In B2C marketing, there is not the same opportunity to track business organizations and companies with specific purchasing agents. The retail consumer market represented by B2C is much broader and defined by e-commerce platform requirements.
在B2C营销分析中,将销售决策归于一个人的难度较小。 在B2C营销中,没有相同的机会来跟踪具有特定采购代理的业务组织和公司。 B2C代表的零售消费市场范围更广,并由电子商务平台要求定义。
- Online stores track consumer sales channels on websites and mobile apps with various forms of cookies, where the largest enterprise sites frequently support 100 to 250 million registered users on their platforms. 在线商店使用各种cookie跟踪网站和移动应用程序上的消费者销售渠道,其中最大的企业站点通常在其平台上支持100至2.5亿注册用户。
- This leads to the potential of personalization of the e-commerce data from automated marketing analytics driven by machine learning and data science in real-time. 这导致了由机器学习和数据科学实时驱动的自动营销分析产生的电子商务数据个性化的潜力。
- Businesses track the variables related to search engine traffic, repeat customers, PPC advertising, and direct email campaigns to determine the effectiveness of advertising in sales cycles. 企业跟踪与搜索引擎流量,回头客,PPC广告和直接电子邮件活动有关的变量,以确定广告在销售周期中的有效性。
- These variable events can also chart the effectiveness of limited-time sales and holiday promotions through marketing analytic software. 这些可变事件还可以通过市场分析软件绘制限时销售和假日促销的效果图。
CALCULATING ROMI ANALYTICS FOR CAMPAIGNS
CAMPAIGNS的ROMI分析计算
Return On Marketing Investment (ROMI) is an OPEX model that compares non-CAPEX spend to yield. The term was coined by Guy Powell in 2002 to be used in the evaluation of marketing campaigns with the investment in expensive commercial advertising designed to build long-term brand identity, customer loyalty, and goodwill. ROMI is calculated on the simple formula related to change in revenue, margins, and program spend.
营销投资回报(ROMI)是一种OPEX模型,用于比较非CAPEX支出与收益。 该术语是由盖伊·鲍威尔 ( Guy Powell)于2002年创造的,用于评估营销活动,并投资于昂贵的商业广告,以建立长期的品牌形象,客户忠诚度和商誉。 ROMI是根据与收入,利润和计划支出的变化有关的简单公式计算的。
- Powell recognized two kinds of ROMI, “Fuzzy” and “Sharp”. 鲍威尔认识到两种ROMI,即“模糊”和“锐利”。
- Fuzzy ROMI attempts to estimate brand loyalty and goodwill through the statistical measurement of qualitative values in customer feedback. Fuzzy ROMI试图通过对客户反馈中的定性值进行统计测量来估计品牌忠诚度和商誉。
- Sharp ROMI is used in 90% of marketing analytics to track the total program spend vs.new revenue generated in a business cycle. 在90%的营销分析中使用Sharp ROMI来跟踪计划总支出与业务周期中产生的新收入之间的关系。
- Sharp ROMI metrics are used to evaluate the effectiveness of B2B & B2C advertising with machine learning and data science. 清晰的ROMI指标用于通过机器学习和数据科学评估B2B和B2C广告的有效性。
“Big data” repositories are filtered by machine learning algorithms to help decision-makers discern patterns through chart analysis to predict similar success. The ideal is to create a live data science model trained to deploy custom content and displays to customers based on their historical likes and preferences. There are benefits to both prescriptive and descriptive models.
机器学习算法对“大数据”存储库进行了过滤,以帮助决策者通过图表分析来识别模式,以预测类似的成功。 理想的做法是创建一个实时数据科学模型,该模型经过培训可以根据客户的历史喜好和喜好向其部署自定义内容和显示。 说明性和描述性模型都有好处。
WORKING WITH DESCRIPTIVE MODELS
使用描述性模型
Descriptive models filter “big data” from e-commerce and CRM resources to evaluate ROMI in the rear view mirror, i.e. through an analysis of past activity.
描述性模型从电子商务和CRM资源中过滤“大数据”,以通过后视镜评估ROMI,即通过对过去的活动进行分析。
- Marketing analytic software prioritizes recent activity in sales cycles and should be retrained daily in a data science notebook. 营销分析软件会优先考虑销售周期中的最新活动,并且应该每天在数据科学笔记本中进行培训。
- Comparative information includes campaigns, results, and costs as variants of success across sales channels. 比较信息包括营销活动,结果和成本,作为跨销售渠道成功的变体。
WORKING WITH PREDICTIVE MODELS
使用预测模型
Predictive models in marketing analytics are based on live feedback and depend on live data. This information from clients and customers must be stored in a solid, secure, and fast database.
预测模型 市场营销分析中的信息基于实时反馈并依赖实时数据。 来自客户和客户的信息必须存储在可靠,安全且快速的数据库中。
- Predictive models of marketing analytics assist decision-makers to discern how to change advertising campaign content to match consumer tastes and trends. 营销分析的预测模型可帮助决策者识别如何更改广告活动的内容以匹配消费者的品味和趋势。
- Predictive models are developed in the ROMI phase to optimize advertising campaigns through both product-side and customer-side recommendation engines. 在ROMI阶段开发了预测模型,以通过产品端和客户端推荐引擎优化广告活动。
These methods can help e-commerce platforms and stores avoid shopping cart abandonment by customers. In marketing analytics driven by data science, the more that you refresh the models, the smarter the results get over time.
这些方法可以帮助电子商务平台和商店避免顾客放弃购物车。 在由数据科学推动的营销分析中,您刷新模型的次数越多,随着时间的推移结果越聪明。
OPTIMIZING SUCCESS IN MARKETING ANALYTICS
优化营销分析的成功
In order to succeed in marketing analytics for advertising campaigns with machine learning, businesses must separate existing customers, new business, and renewals.
为了通过机器学习成功地进行广告活动的营销分析,企业必须将现有客户,新业务和续订分开。
- In e-commerce, most platform revenue is earned from repeat business, which can be optimized through the creation of personalized displays to the customer through product recommendations. 在电子商务中,大多数平台收入是从重复业务中获得的,可以通过创建通过向客户推荐产品的个性化展示来优化收入。
- In preparing data for metrics and analytics in data science, businesses need to collect customer event variables into a single stream, then tag each event, and aggregate the information at scale for the platform. 在为数据科学中的指标和分析准备数据时,企业需要将客户事件变量收集到单个流中,然后标记每个事件,并按平台规模地汇总信息。
Champagne charts can be used to determine when path behavior emerges from data analysis.
香槟图表可用于确定何时从数据分析中得出路径行为。
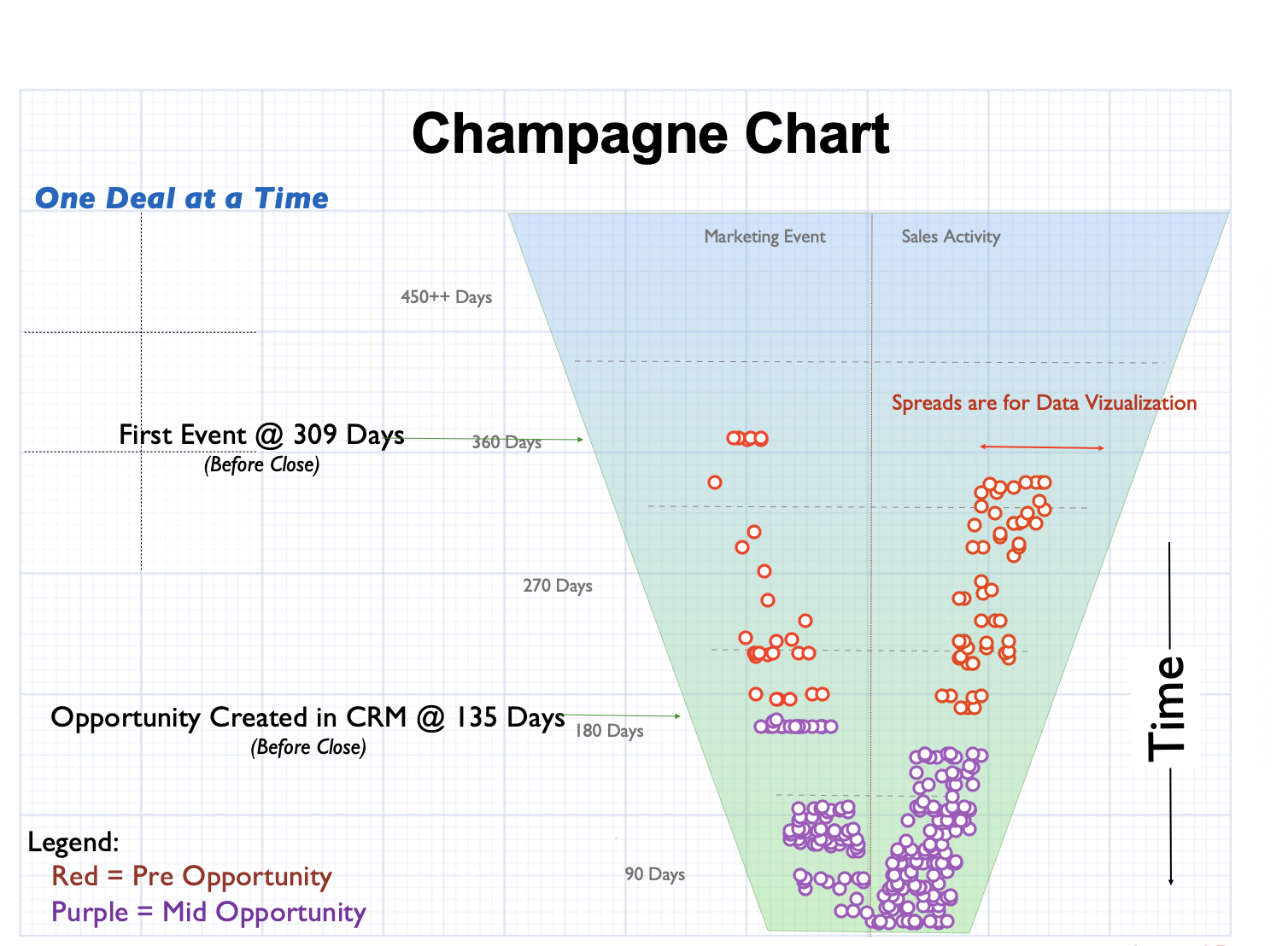
Pattern fitting shows how people consume product evaluations before they buy a product.
模式拟合显示人们在购买产品之前如何使用产品评估。
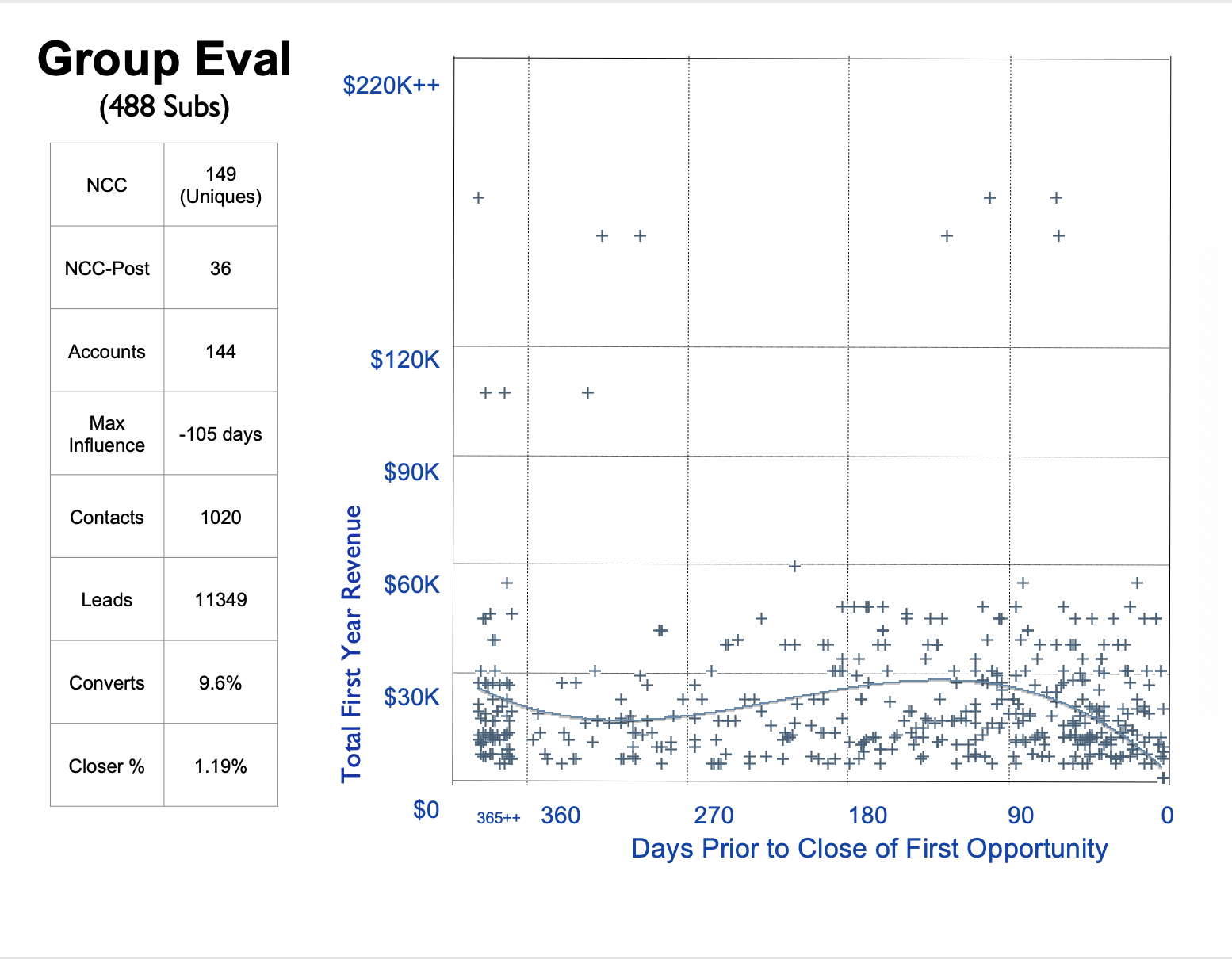
CONCLUSION
结论
Get the data. Be patient; this will take weeks. It takes a lot of time to get all permission to find out where all the systems are and go from there.
获取数据。 耐心一点; 这将需要数周时间。 获得所有许可才能找出所有系统的位置并从那里去,这需要花费大量时间。
- Get access to all systems 访问所有系统
- Agree on a single timestamp method 同意单个时间戳方法
- Agree on what “success” means for a sale (when, what, where, entity) 同意“成功”对销售的意义(何时,何地,何地,实体)
- Find all mid-point systems 查找所有中点系统
- Agree on an organizing campaign model 同意组织运动模型
- Single stream data per person/organization/sale, product family/sales motion 每人/组织/销售,产品系列/销售动向的单流数据
2. Use a cloud database: Do not store your data in flat files or on a laptop. Plan for scale, even if you’re just kicking the tires. I really like Snowflake because it’s fast and it’s cloud-based. So if you want to transfer a project from Europe to the United States for analysis it’s available to you. If it’s in your data center, it may be 50–100 times slower to get it across the ocean.
2. 使用云数据库:不要将数据存储在平面文件或便携式计算机中。 即使您只是踢轮胎,也要计划规模。 我非常喜欢Snowflake,因为它速度快且基于云。 因此,如果您要将项目从欧洲转移到美国进行分析,则可以使用该项目。 如果在您的数据中心中,则跨海传输可能要慢50-100倍。
- Reorganize and normalize data 重组和规范化数据
- Anonymize it on input, but leave a decoder system (as people drill into “proof) 对输入进行匿名处理,但保留解码器系统(因为人们钻研“证明”)
- Plan on scale and long-term global inputs/outputs — data is a movable feast 计划规模和长期全球投入/产出-数据是一场盛宴
- Enable for future why/what/when queries — add a few helper columns and tables 启用将来查询的原因/内容/时间-添加一些帮助程序列和表
- Hook it up to a fast, scaleable data science notebook 将其连接到快速,可扩展的数据科学笔记本上
ADDITIONAL RESOURCES
额外资源
This blog is an excerpt from Grover Righter’s webinar, “Using Data Science for Marketing Analytics. Watch the entire webinar here.
该博客摘自格罗弗·赖特(Grover Righter)的网络研讨会“使用数据科学进行市场营销分析”。 在此处观看整个网络研讨会 。
Zepl’s data science platform lets data scientists and analysts rapidly prototype models in Python, R, Scala and SQL to create rich visuals for their marketing analytics use cases. Try it for free at www.zepl.com
Zepl的数据科学平台使数据科学家和分析师可以使用Python,R,Scala和SQL快速创建模型原型,从而为其营销分析用例创建丰富的视觉效果。 在www.zepl.com免费试用
Snowflake helps marketing analytics teams drive more business value from their data by offering a scalable, elastic, and secure cloud data platform for all of their data workloads. Try it for free at www.snowflake.com
Snowflake通过为所有数据工作负载提供可扩展,灵活且安全的云数据平台 ,帮助营销分析团队从数据中获取更多业务价值。 在www.snowflake.com上免费试用
AUTHOR
作者
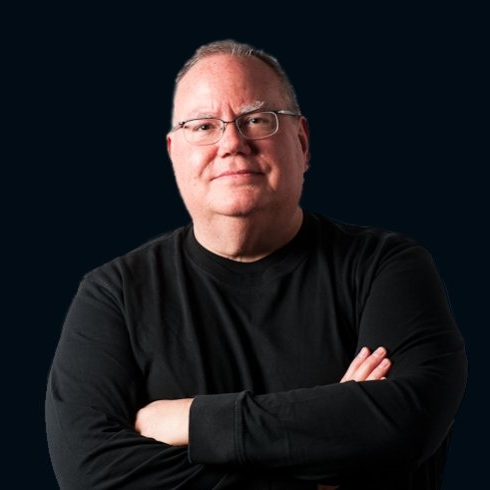
Grover Righter is the Chief Data Scientist at Zepl. He is focused on science-based marketing programs and runs a backplane of analytic engines measuring email, SEO and social media effectiveness. He has worked on every major know marketing automation platforms including Eloqua, Marketo, Pardot, HubSpot and Leadformix.
Grover Righter是Zepl的首席数据科学家。 他专注于基于科学的营销计划,并运行分析引擎的背板,以测量电子邮件,SEO和社交媒体的有效性。 他曾在每个知名的市场营销自动化平台上工作,包括Eloqua,Marketo,Pardot,HubSpot和Leadformix。
Grover has been working in the high technology sector since 1981. He began as a mathematician and design engineer and has been instrumental in the design and development of major technologies at RMS, Inc., AT&T, Unisys and Novell. But every time Grover built a product, he was immediately recruited to ‘sell it’, irrespective of the presence of another sales team within the organization. Eventually, Grover used up his lifetime limit of sales meetings as an engineer and was moved into marketing.
Grover自1981年以来一直从事高科技领域的工作。他最初是一名数学家和设计工程师,并在RMS,Inc.,AT&T,Unisys和Novell的主要技术的设计和开发中发挥了重要作用。 但是,每次Grover生产产品时,无论组织内是否存在其他销售团队,他都会立即被招募以“出售”。 最终,格罗弗(Grover)作为一名工程师用尽了他毕生的销售会议上的极限,并进入市场营销。
翻译自: https://towardsdatascience.com/using-data-science-for-marketing-analytics-8fc066c44238
数据科学数据分析