lime 深度学习
It’s needless to say: machine learning is powerful.
不用说:机器学习功能强大。
At the most basic level, machine learning algorithms can be used to classify things. Given a collection of cute animal pictures, a classifier can separate the pictures into buckets of ‘dog’ and ‘not a dog’. Given data about customer restaurant preferences, a classifier can predict what restaurant a user goes to next.
在最基本的层次上,机器学习算法可用于对事物进行分类 。 给定一组可爱的动物图片,分类器可以将图片分成“狗”和“不是狗”的桶。 给定有关客户餐厅偏好的数据,分类器可以预测用户将前往哪个餐厅。
However, the role of humans is overlooked in the technology. It does not matter how powerful a machine learning model is if one does not use it. With so little explanation or reasoning as to how these algorithms made their predictions, if users do not trust a model or a prediction, they will not use it.
但是, 人类的作用在技术中被忽略了。 如果不使用机器学习模型,它的功能有多强大也没关系。 关于这些算法如何做出预测的解释或推理很少,如果用户不信任模型或预测,他们将不会使用它。
“If the users do not trust a model or a prediction, they will not use it.”
“如果用户不信任模型或预测,他们将不会使用它。”
As machine learning becomes deployed in even more domains, such as medical diagnosis and recidivism, the decisions these models make can have incredible consequences. Thus, it is of utmost importance to understand and explain how their predictions came to be, which then builds trust.
随着机器学习在医疗诊断和累犯等更多领域中的应用,这些模型做出的决策可能会产生令人难以置信的后果。 因此,最重要的是理解和解释他们的预测是如何形成的,然后建立信任。
In their paper “‘Why Should I Trust You?’ Explaining the Predictions of Any Classifier”, Ribeiro, Singh, and Guestrin present a new technique to do so: LIME (Local Interpretable Model-agnostic Explanations). This post will summarize their findings and introduce LIME.
在他们的论文“为什么我应该信任你?” Ribeiro,Singh和Guestrin 解释了“任何分类器的预测” ,提出了一种新的方法:LIME(与局部可解释模型无关的解释)。 这篇文章将总结他们的发现并介绍LIME。
一行摘要 (One line summary)
LIME is a new technique that explains predictions of any machine learning classifier and has been shown to increase human trust and understanding.
LIME是一种新技术,可以解释任何机器学习分类器的预测,并已显示出它可以增加人们的信任和理解。
解释预测 (Explaining predictions)
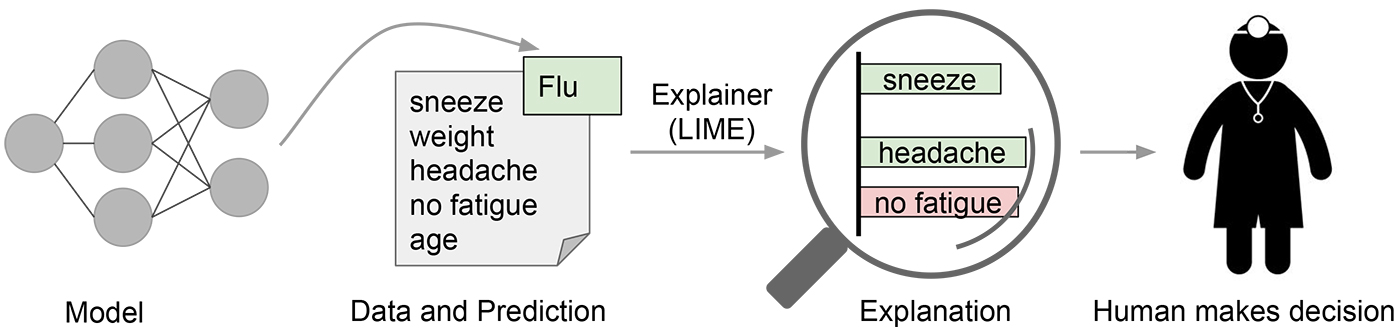
为什么解释预测有用? (Why is explaining predictions useful?)
Let’s look at the example use case of medical diagnosis. Given the patient’s symptoms and measurements, a doctor must make their best judgment as to what the patient’s diagnosis is.
让我们看一下医学诊断的示例用例。 鉴于患者的症状和测量结果,医生必须对患者的诊断做出最佳判断。
Humans (both the doctor and the patient) are more willing to accept (trust) a diagnosis when they have more prior knowledge.
当人类(医生和患者)拥有更多的先验知识时,他们更愿意接受(信任)诊断。
A model has the potential to help a doctor even more with greater data and scalability. Adding an explanation into the process, like in the above figure, would then help humans to trust and use machine learning more effectively.
模型有潜力通过更大的数据和可扩展性来帮助医生。 如上图所示,在流程中添加说明将帮助人们更有效地信任和使用机器学习。
需要什么解释? (What do explanations need?)
1) The explanation needs to be interpretable.
1)说明必须是可解释的 。
An interpretable model provides qualitative understanding between the inputs and the output.
可解释的模型提供了输入和输出之间的定性理解。
Interpretability must also take into account user limitations and target audience. It is not reasonable to expect a user to understand why a prediction was made if thousands of features contribute to that prediction.
可解释性还必须考虑用户限制和目标受众。 如果成千上万的特征对用户的预测有所贡献,那么期望用户理解为什么进行预测是不合理的。
2) The explanation needs to be locally faithful.
2)说明必须是本地忠实的 。
Fidelity measures how well the explanation approximates the model’s prediction. High fidelity is good, low fidelity is useless. Local fidelity means the explanation needs to approximate well to the model’s prediction for a subset of the data.
保真度衡量解释与模型预测的近似程度。 高保真度好,低保真度无用。 局部保真度意味着解释需要很好地近似于模型对数据子集的预测。
3) The explanation needs to be model agnostic.
3)解释需要与模型无关 。
We should always treat the original machine learning model as a black box. This helps equalize non-interpretable and interpretable models + adds flexibility for future classifiers.
我们应该始终将原始的机器学习模型视为黑匣子。 这有助于均衡不可解释和可解释的模型,并增加了将来分类器的灵活性。
4) The explanation needs to provide a global perspective.
4)解释需要提供全局视角 。
Rather than only explaining one prediction, we should select a few explanations to present to users such that they represent the whole model.
不仅要解释一个预测,我们还应该选择一些解释以呈现给用户,以便他们代表整个模型。
LIME如何工作? (How does LIME work?)
“The overall goal of LIME is to identify an interpretable model over the interpretable representation that is locally faithful to the classifier.”
“ LIME的总体目标是在可解释的表示形式上确定一个可忠实于分类器的可解释模型。”
LIME boils down to one central idea: we can learn a model’s local behavior by varying the input and seeing how the outputs (predictions) change.
LIME可以归结为一个中心思想: 我们可以通过更改输入并查看输出(预测)如何变化来学习模型的局部行为 。
This is really useful for interpretability, because we can change the input to make sense for humans (words, images, etc.), while the model itself might use more complicated data representations. We call this input changing process perturbation. Some examples of perturbation include adding/removing words and hiding a part of an image.
这对于可解释性非常有用,因为我们可以更改输入以使人(单词,图像等)有意义,而模型本身可能使用更复杂的数据表示形式。 我们称这种输入改变过程为扰动 。 摄动的一些示例包括添加/删除单词并隐藏图像的一部分。
Rather than trying to approximate a model globally, which is a daunting task, it is easier to approximate a model locally (close to the prediction we want to explain). We can do so by approximating a model by an interpretable one learned from perturbations of the original data, and the perturbed data samples are weighted by how similar they are to the original data.
与其尝试在全球范围内逼近模型(这是一项艰巨的任务),不如在本地范围内逼近模型(接近我们要解释的预测)。 我们可以通过从原始数据的扰动中学到的可解释模型来近似模型,然后通过与原始数据的相似程度对扰动的数据样本进行加权。
Examples were shown in the paper with both text classification and image classification. Here is an image classification example:
本文显示了带有文本分类和图像分类的示例。 这是一个图像分类示例:
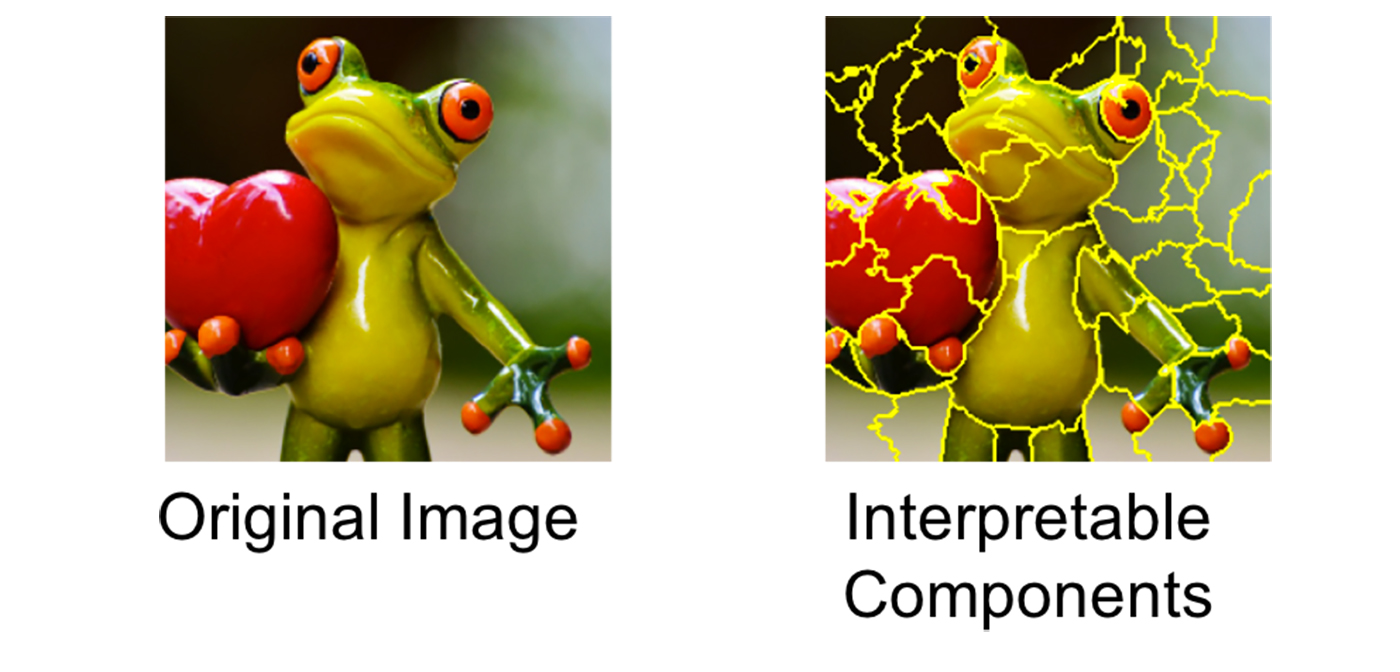
Say we want to explain a classification model that predicts whether an image contains a frog. Given the original image (left), we carve up the photo into different interpretable elements (right).
假设我们要解释一个分类模型,该模型可以预测图像是否包含青蛙。 给定原始图像(左),我们将照片分割成不同的可解释元素(右)。
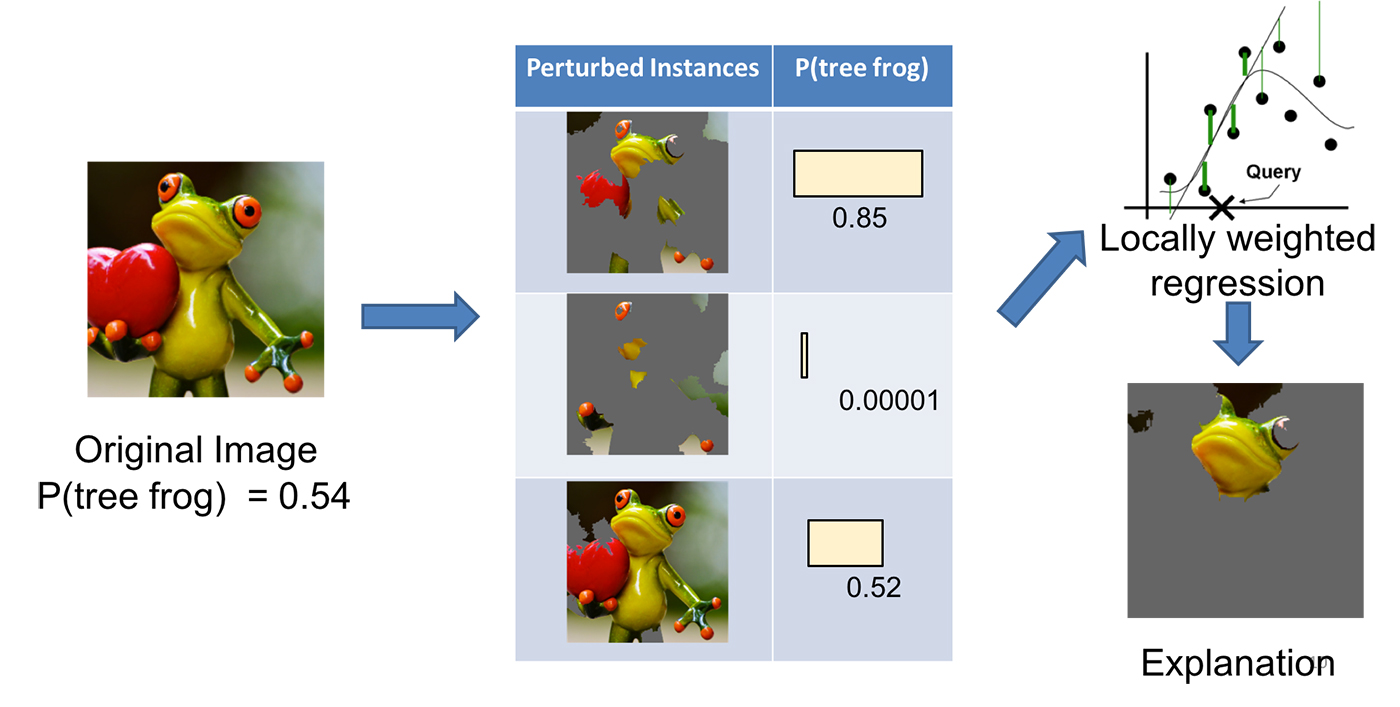
Then, we generate a data set of perturbed samples by hiding some of the interpretable elements (the parts colored gray). For each sample, as we see in the middle table above, we derive the probability of whether the frog is in the image. We learn a locally-weighted model from this dataset (perturbed samples more similar to the original image are more important).
然后,我们通过隐藏一些可解释的元素(颜色为灰色的部分)来生成一个扰动样本的数据集。 对于每个样本,如我们在上面的中间表中所见,我们得出青蛙是否在图像中的概率。 我们从该数据集中学习了局部加权模型(与原始图像更相似的扰动样本更为重要)。
Finally, we return the parts of the image with the highest weights as the explanation.
最后,我们返回图像中权重最高的部分作为说明。
与真实人类的用户研究 (User studies with real humans)
To evaluate the effectiveness of LIME, a few experiments (with both simulated users and human subjects) were conducted with these 3 questions in mind:
为了评估LIME的有效性,针对以下三个问题进行了一些实验(针对模拟用户和人类受试者):
- Are the explanations faithful to the model? 解释是否忠实于模型?
- Can the explanations help users increase trust in predictions? 说明可以帮助用户增加对预测的信任吗?
- Are the explanations useful for evaluating the model as a whole? 这些说明对评估整个模型有用吗?
解释是否忠实于模型? (Are the explanations faithful to the model?)
For each classifier, the researchers kept note of a gold set of features — the most important features. Then, they computed the fraction of the gold features recovered by LIME’s explanations. In the simulated user experiments, LIME consistently provided > 90% recall on all datasets.
对于每个分类器,研究人员都记录了一组金色的功能-最重要的功能。 然后,他们计算了LIME解释中回收的黄金特征的比例。 在模拟的用户实验中,LIME在所有数据集中始终提供> 90%的召回率。
说明可以帮助用户增加对预测的信任吗? (Can the explanations help users increase trust in predictions?)
In the simulated user experiments, the results showed that LIME outperformed other explainability methods. With real human subjects (Amazon Mechanical Turk users), they showed high agreement in choosing the best classifier and improving them.
在模拟的用户实验中,结果表明LIME优于其他可解释性方法。 对于真实的人类受试者(Amazon Mechanical Turk用户),他们在选择最佳分类器和改进分类方面表现出很高的共识。
“Before observing the explanations, more than a third trusted the classifier… After examining the explanations, however, almost all of the subjects identified the correct insight, with much more certainty that it was a determining factor.”
“在观察解释之前,超过三分之一的人信任分类器……但是,在研究了解释之后,几乎所有的受试者都确定了正确的见解,并且更加确定地认为这是决定因素。”
这些说明对评估整个模型有用吗? (Are the explanations useful for evaluating the model as a whole?)
From both simulated user and human subject experiments, yes, it does seem so. Explanations are useful for models in the text and image domains especially, in deciding which model is best to use, assessing trust, improving untrustworthy classifiers, and getting more insight about models’ predictions.
从模拟的用户实验和人类受试者实验来看,是的,确实如此。 解释对于文本和图像域中的模型很有用,尤其是在确定哪种模型最适合使用,评估信任度,改进不可信分类器以及获得有关模型预测的更多见解方面。
我的最后想法 (My final thoughts)
LIME presents a new method to explain predictions of machine learning classifiers. It’s certainly a necessary step in achieving greater explainability and trust in AI, but not perfect — recent work has demonstrated flaws in LIME; for example, this paper from 2019 showed that adversarial attacks on LIME and SHAP (another interpretability technique) could successfully fool their systems. I am excited to continue seeing more research and improvements on LIME and other similar interpretability techniques.
LIME提供了一种新方法来解释机器学习分类器的预测。 当然,这是提高对AI的可解释性和信任度的必要步骤,但不是完美的方法-最近的工作证明LIME中存在缺陷。 例如,2019年的这篇论文表明,对LIME和SHAP(另一种可解释性技术)的对抗攻击可能成功使他们的系统蒙蔽。 我很高兴继续看到有关LIME和其他类似可解释性技术的更多研究和改进。
For more information, check out the original paper on arXiv here and their code repo here.
欲了解更多信息,请查看原文件上的arXiv 这里和他们的代码回购这里 。
Marco Tulio Ribeiro, Sameer Singh, and Carlos Guestrin. “‘Why Should I Trust You?’ Explaining the Predictions of Any Classifier.” ACM Conference on Knowledge Discovery and Data Mining (KDD) 2016.
Marco Tulio Ribeiro,Sameer Singh和Carlos Guestrin。 “'我为什么要相信你?' 解释任何分类器的预测。” 2016年ACM知识发现和数据挖掘(KDD)会议。
Thank you for reading! Subscribe to read more about research, resources, and issues related to fair and ethical AI.
感谢您的阅读! 订阅以了解有关公平,合乎道德的AI的研究,资源和问题的更多信息。
Catherine Yeo is a CS undergraduate at Harvard interested in AI/ML/NLP, fairness and ethics, and everything related. Feel free to suggest ideas or say hi to her on Twitter.
Catherine Yeo是哈佛大学的CS本科生,对AI / ML / NLP,公平与道德以及所有相关方面感兴趣。 随时在Twitter上提出想法或向她打招呼。
lime 深度学习