检测对抗样本
Neural Networks are exceptionally good at recognizing objects shown in an image and in many cases, they have shown superhuman levels of accuracy(E.g.-Traffic sign recognition).
神经网络非常擅长识别图像中显示的物体,并且在许多情况下,它们已经显示出超人的准确度(例如,交通标志识别)。
But they are also known to have an interesting property where we can introduce some small changes to the input photo and have the Neural Network wrongly classify it into something completely different. Such attacks are known as adversarial attacks on a Neural Network. One important variant is known as the Fast Gradient sign method, by Ian GoodFellow et al, as seen in the paper Explaining and Harnessing Adversarial Examples. If properly implemented, such methods can add noise to the image barely perceptible to the human eye but it fools the Neural Network classifier. One classic example is shown below.
但是也众所周知,它们具有有趣的属性,我们可以在输入的照片上进行一些细微的更改,并使神经网络将其错误地分类为完全不同的东西。 这种攻击被称为对神经网络的对抗攻击。 一个重要的变体被Ian GoodFellow等人称为快速梯度符号法,如论文“ 解释和利用对抗性示例”中所见。 如果实施得当,此类方法可能会给人眼几乎看不到的图像增加噪点,但却使神经网络分类器蒙昧。 下面显示了一个经典示例。
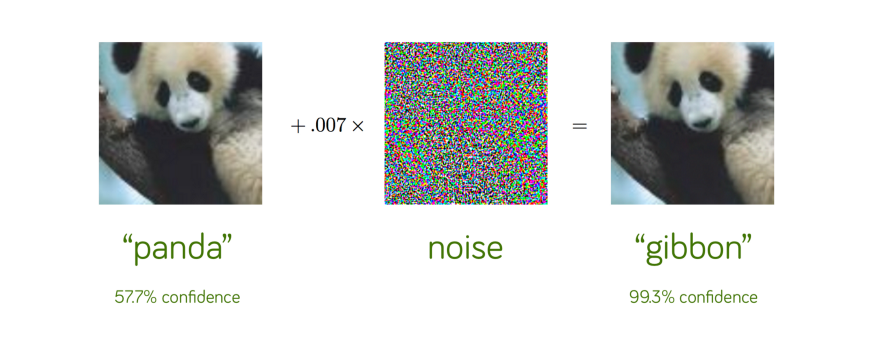
实时物体检测器-自动化监控的基础: (Real time Object detectors-The backbone of automated surveillance:)
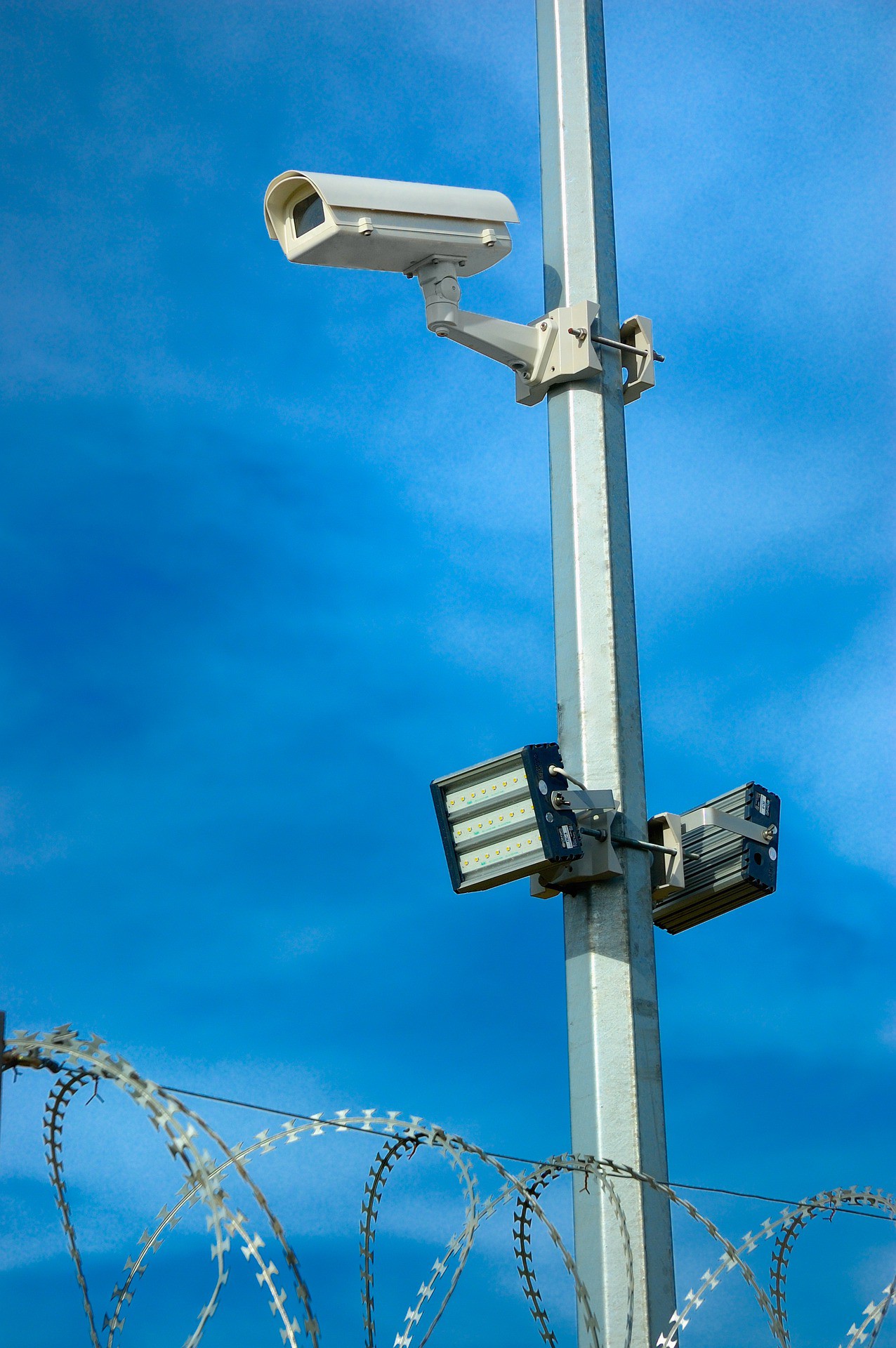
Object detection is a classic problem in Computer Vision where given an image you have to localize an object present in the image as well as classify the category that an object belongs to. Usually this is done by training a neural network on a dataset containing sufficient number of images in each category we want to address and the output being the location of the object as well as the probability of the object belonging to the different categories.Some of the most popular Object detector models are YOLO and Faster RCNN. The detection is real-time and can be embedded in a video feed to detect objects instantaneously.
对象检测是Computer Vision中的经典问题,在给定图像的情况下,您必须定位图像中存在的对象以及对对象所属的类别进行分类。 通常,这是通过在数据集上训练神经网络来完成的,该数据集包含我们要处理的每个类别中足够数量的图像,输出是对象的位置以及对象属于不同类别的概率。最受欢迎的对象检测器模型是YOLO和Faster RCNN。 该检测是实时的,可以嵌入视频源中以即时检测对象。
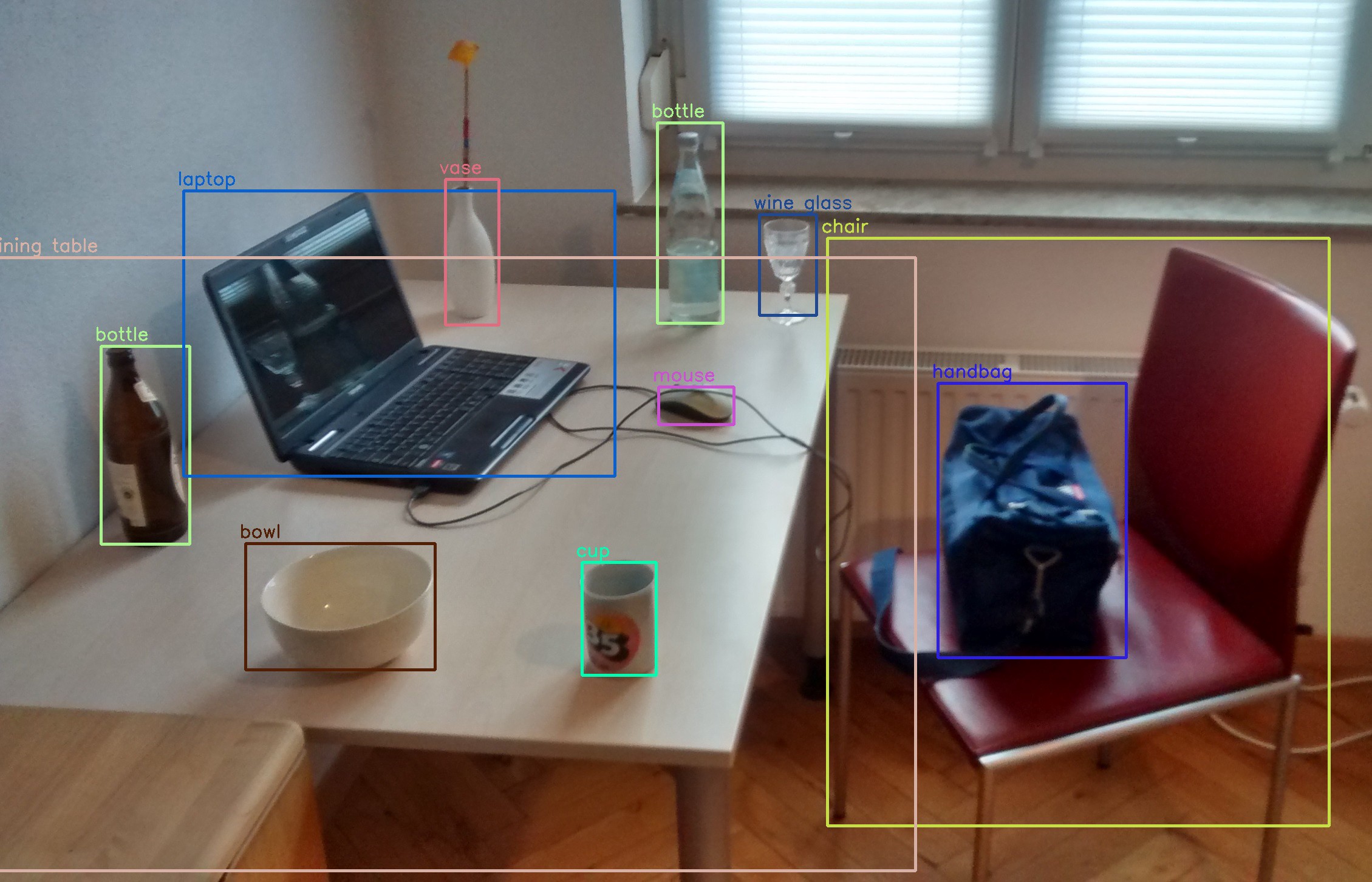
欺骗对象检测器: (Fooling the Object detectors:)
Using the adversarial attacks discussed above researchers have successfully been able to create images or patches that can baffle an object detector. This can be used to evade detection of glass frames, stop signs and images attached to cardboard. But Surveillance systems often have to view a person from a wide variety of angles so creating just a patch is not sufficient for the problem at hand. To do this the authors tracked the deformations of the t-shirts and mapped them onto adversarial images until it was able to fool the classifier. The result was the creation of a checkerboard pattern whose every pattern of deformation in video frame was measured. Then finally the interpolation technique Thin Plate Spline Technique(TPS) was used to replace the checkerboard patch with other images.Finally, a t-shirt was designed by Kaidi Xu and colleagues at Northeastern, MIT-IBM Watson AI Lab, and MIT that was able to baffle variety of object detection methods including YOLO v2 and Faster RCNN which was unsuccessful at detection of people wearing this shirt.
使用上面讨论的对抗攻击,研究人员已经成功地能够创建图像或补丁,使目标检测器感到困惑。 这可用于逃避对玻璃框的检测,停止粘贴在硬纸板上的标志和图像。 但是监视系统通常必须从多种角度观察一个人,因此仅创建补丁不足以解决当前的问题。 为此,作者跟踪了T恤的变形,并将其映射到对抗图像上,直到它能够使分类器蒙骗为止。 结果就是创建了一个棋盘格图案,其视频帧中的每个变形图案都得到了测量。 最后,使用插值技术Thin Plate Spline Technique(TPS)将棋盘格贴图替换为其他图像。能够挡住包括YOLO v2和Faster RCNN在内的多种对象检测方法,这些方法在检测穿这件衬衫的人方面均不成功。
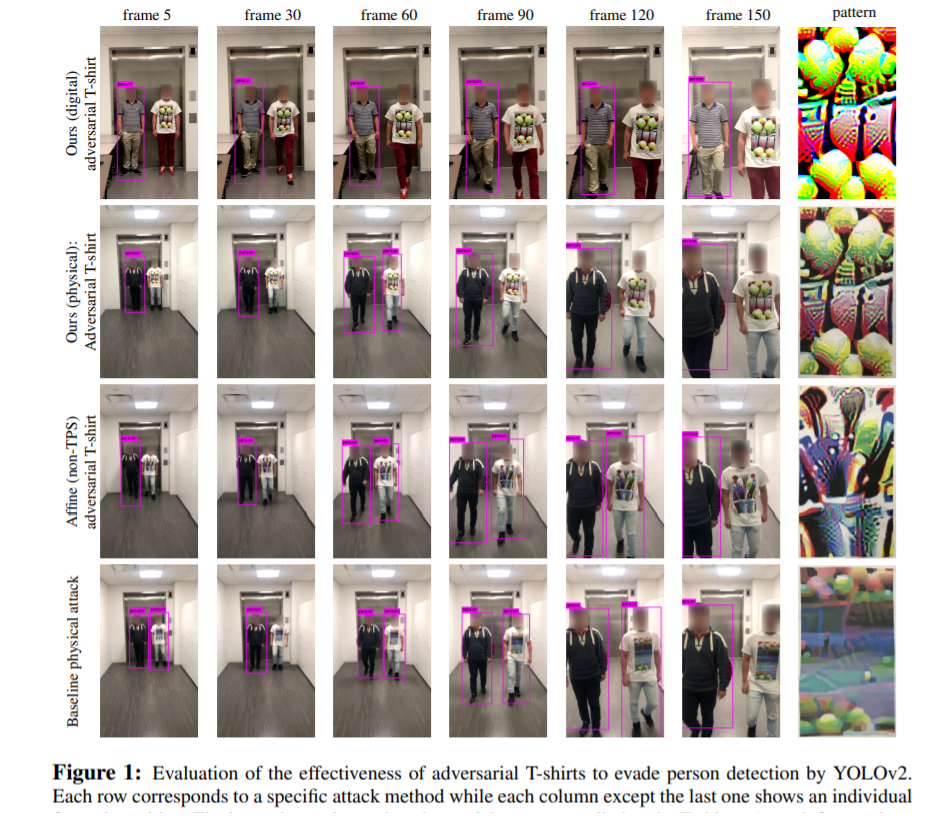
结果: (Results:)
Finally after printing these kinds of shirts they were put into action in front of surveillance cameras and videos of the result was recorded. It turns out YOLO v2 model was fooled in 57 percent of frames in the physical world and 74 percent in the digital world, a substantial improvement over the previous state of the art’s 18 percent and 24 percent.
最终,在将此类衬衫打印后,将它们投入监控摄像机的前面,并记录了结果的视频。 原来YOLO V2模型是在现实世界中的帧的57%和在数字世界74%上当,比上的显着改进现有技术的状态的18%和24%。
结论: (Conclusion:)
This paper provides useful information on how adversarial perturbations and noises can be implemented in real life scenarios. Images generated by this method can be used to train the different classifiers and in turn produce more robust classifiers. Such classifiers may prevent object detectors from getting tricked in the future.
本文提供了有关如何在现实生活中实现对抗性扰动和噪音的有用信息。 通过这种方法生成的图像可用于训练不同的分类器,进而产生更强大的分类器。 这样的分类器可以防止对象检测器将来被欺骗。
翻译自: https://towardsdatascience.com/adversarial-t-shirts-to-evade-ml-person-detectors-8f4ee7af9331
检测对抗样本