Caffe在Cifar10上复现ResNet
ResNet在2015年的ImageNet竞赛上的识别率达到了非常高的水平,这里我将使用Caffe在Cifar10上复现论文4.2节的Cifar实验。
- ResNet的基本模块
- Caffe实现
- Cifar10上的实验结果及说明
ResNet的基本模块
本文参照Torch7在Cifar10上复现ResNet的实验,使用Caffe复现之。ResNet的基本模块可以如下Python代码:
@requires_authorizationfrom __future__ import print_function from caffe import layers as L, params as P, to_proto from caffe.proto import caffe_pb2 import caffe # helper function for building ResNet block structures # The function below does computations: bottom--->conv--->BatchNormdef conv_factory(bottom, ks, n_out, stride=1, pad=0): conv = L.Convolution(bottom, kernel_size=ks, stride=stride, num_output=n_out, pad=pad, param = [dict(lr_mult=1, decay_mult=1), dict(lr_mult=2, decay_mult=0)], bias_filler=dict(type='constant', value=0), weight_filler=dict(type='gaussian', std=0.01)) batch_norm = L.BatchNorm(conv, in_place=True, param=[dict(lr_mult=0, decay_mult=0), dict(lr_mult=0, decay_mult=0), dict(lr_mult=0, decay_mult=0)]) scale = L.Scale(batch_norm, bias_term=True, in_place=True) return scale # bottom--->conv--->BatchNorm--->ReLUdef conv_factory_relu(bottom, ks, n_out, stride=1, pad=0): conv = L.Convolution(bottom, kernel_size=ks, stride=stride, num_output=n_out, pad=pad, param=[dict(lr_mult=1, decay_mult=1), dict(lr_mult=2, decay_mult=0)], bias_filler=dict(type='constant', value=0), weight_filler=dict(type='gaussian', std=0.01)) batch_norm = L.BatchNorm(conv, in_place=True, param=[dict(lr_mult=0, decay_mult=0), dict(lr_mult=0, decay_mult=0), dict(lr_mult=0, decay_mult=0)]) scale = L.Scale(batch_norm, bias_term=True, in_place=True) relu = L.ReLU(scale, in_place=True) return relu # Residual building block! Implements option (A) from Section 3.3. The input# is passed through two 3x3 convolution layers. Currently this block only supports # stride == 1 or stride == 2. When stride is 2, the block actually does pooling.# Instead of simply doing pooling which may cause representational bottlneck as# described in inception v3, here we use 2 parallel branches P && C and add them# together. Note pooling branch may has less channels than convolution branch so we# need to do zero-padding along channel dimension. And to the best knowledge of# ours, we haven't found current caffe implementation that supports this operation. # So later I'll give implementation in C++ and CUDA.def residual_block(bottom, num_filters, stride=1):if stride == 1: conv1 = conv_factory_relu(bottom, 3, num_filters, 1, 1) conv2 = conv_factory(conv1, 3, num_filters, 1, 1) add = L.Eltwise(bottom, conv2, operation=P.Eltwise.SUM) return add elif stride == 2: conv1 = conv_factory_relu(bottom, 3, num_filters, 2, 1) conv2 = conv_factory(conv1, 3, num_filters, 1, 1) pool = L.Pooling(bottom, pool=P.Pooling.AVE, kernel_size=2, stride=2) pad = L.PadChannel(pool, num_channels_to_pad=num_filters / 2) add = L.Eltwise(conv2, pad, operation=P.Eltwise.SUM) return add else: raise Exception('Currently, stride must be either 1 or 2.') # Generate resnet cifar10 train && test prototxt. n_size control number of layers.# The total number of layers is 6 * n_size + 2. Here I don't know any of implementation # which can contain simultaneously TRAIN && TEST phase. # ==========================Note here==============================# !!! SO YOU have to include TRAIN && TEST by your own AFTER you use the script to generate the prototxt !!!def resnet_cifar(train_lmdb, test_lmdb, mean_file, batch_size=100, n_size=3): data, label = L.Data(source=test_lmdb, backend=P.Data.LMDB, batch_size=batch_size, ntop=2, transform_param=dict(mean_file=mean_file, crop_size=28), include=dict(phase=getattr(caffe_pb2, 'TEST'))) residual = conv_factory_relu(data, 3, 16, 1, 1) # --------------> 16, 32, 32 1st groupfor i in xrange(n_size): residual = residual_block(residual, 16) # --------------> 32, 16, 16 2nd group residual = residual_block(residual, 32, 2) for i in xrange(n_size - 1): residual = residual_block(residual, 32) # --------------> 64, 8, 8 3rd group residual = residual_block(residual, 64, 2) for i in xrange(n_size - 1): residual = residual_block(residual, 64) # -------------> end of residual global_pool = L.Pooling(residual, pool=P.Pooling.AVE, global_pooling=True) fc = L.InnerProduct(global_pool, param=[dict(lr_mult=1, decay_mult=1), dict(lr_mult=2, decay_mult=1)],num_output=10, bias_filler=dict(type='constant', value=0), weight_filler=dict(type='gaussian', std=0.01)) loss = L.SoftmaxWithLoss(fc, label) acc = L.Accuracy(fc, label, include=dict(phase=getattr(caffe_pb2, 'TEST'))) return to_proto(loss, acc) def make_net(tgt_file):with open(tgt_file, 'w') as f: print('name: "resnet_cifar10"', file=f) print(resnet_cifar('dataset/cifar10_train_lmdb', 'dataset/cifar10_test_lmdb', 'dataset/mean.proto', n_size=9), file=f) if __name__ == '__main__': tgt_file='D:/VSProjects/caffe/models/ucas_resnet_cifar10/res56_cifar_train_test.prototxt' make_net(tgt_file)
Caffe实现
首先所有的卷积核采用的都是3x3大小,当stride=1时在ResNet一个block里面通过padding那么feature map的尺寸不变。这是输入可以和经过两个卷积的分支相加。当stride=2时,通过两个卷积的分支feature map的尺寸减半,输入可以通过pooling减半,变为和经过两个卷积分支的尺寸一样,但是可能会导致pooling分支的channel数目少于卷积分支的数目。有两种方式解决这个问题,如原文所描述的那样:1、通过1x1的卷积线性投影,不接任何激活函数,然后直接将卷积核个数设置到跟卷积分支输出的channels个数相等; 2:通过对channel维度进行加零填充,即zero-padding。这里我采用后者方式,下面给出后者的Caffe实现代码(也就是上面python代码中的L.PadChannel对应的C++代码):
#ifndef CAFFE_PAD_CHANNEL_LAYER_HPP_#define CAFFE_PAD_CHANNEL_LAYER_HPP_#include "caffe/blob.hpp"#include "caffe/layer.hpp"#include "caffe/proto/caffe.pb.h"namespace caffe { /* * @brief zero-padding channel to extend number of channels * * Note: Back-propagate just drop the pad derivatives */template <typename Dtype> class PadChannelLayer : public Layer<Dtype> { public: explicit PadChannelLayer(const LayerParameter& param) : Layer<Dtype>(param) {} virtualvoid LayerSetUp(constvector<Blob<Dtype>*>& bottom, constvector<Blob<Dtype>*>& top); virtualvoid Reshape(constvector<Blob<Dtype>*>& bottom, constvector<Blob<Dtype>*>& top); virtualinlineconstchar* type() const { return"PadChannel"; } virtualinlineint ExactNumBottomBlobs() const { return1; } virtualinlineint ExactNumTopBlobs() const { return1; } protected: virtualvoid Forward_cpu(constvector<Blob<Dtype>*>& bottom, constvector<Blob<Dtype>*>& top); virtualvoid Backward_cpu(constvector<Blob<Dtype>*>& top, constvector<bool>& propagate_down, constvector<Blob<Dtype>*>& bottom); virtualvoid Forward_gpu(constvector<Blob<Dtype>*>& bottom, constvector<Blob<Dtype>*>& top); virtualvoid Backward_gpu(constvector<Blob<Dtype>*>& top, constvector<bool>& propagate_down, constvector<Blob<Dtype>*>& bottom); int num_channels_to_pad_; }; } // namespace caffe#endif // CAFFE_PAD_CHANNEL_LAYER_HPP_
pad_channel_layer.cpp
#include "caffe/layers/pad_channel_layer.hpp"namespace caffe { template <typename Dtype> void PadChannelLayer<Dtype>::LayerSetUp(constvector<Blob<Dtype>*>& bottom, constvector<Blob<Dtype>*>& top) { CHECK_NE(top[0], bottom[0]) << this->type() << " Layer does not ""allow in-place computation."; num_channels_to_pad_ = this->layer_param_.pad_channel_param().num_channels_to_pad(); CHECK_GT(num_channels_to_pad_, 0) << "num channels to pad must greater than 0!"; } template <typename Dtype> void PadChannelLayer<Dtype>::Reshape(constvector<Blob<Dtype>*>& bottom, constvector<Blob<Dtype>*>& top) { vector<int> top_shape = bottom[0]->shape(); top_shape[1] += num_channels_to_pad_; top[0]->Reshape(top_shape); } template <typename Dtype> void PadChannelLayer<Dtype>::Forward_cpu(constvector<Blob<Dtype>*>& bottom, constvector<Blob<Dtype>*>& top) { const Dtype* bottom_data = bottom[0]->cpu_data(); Dtype* top_data = top[0]->mutable_cpu_data(); int num = bottom[0]->num(); int channels = bottom[0]->channels(); int dim = bottom[0]->height() * bottom[0]->width(); int channel_by_dim = channels * dim; for (int n = 0; n < num; n++){ caffe_copy(channel_by_dim, bottom_data, top_data); bottom_data += channel_by_dim; top_data += channel_by_dim; caffe_set(num_channels_to_pad_ * dim, Dtype(0), top_data); top_data += num_channels_to_pad_ * dim; } } template <typename Dtype> void PadChannelLayer<Dtype>::Backward_cpu(constvector<Blob<Dtype>*>& bottom, constvector<bool>& propagate_down, constvector<Blob<Dtype>*>& top) { const Dtype* top_diff = top[0]->cpu_diff(); Dtype* bottom_diff = bottom[0]->mutable_cpu_diff(); int num = bottom[0]->num(); int channels = bottom[0]->channels(); int dim = bottom[0]->height() * bottom[0]->width(); int channel_by_dim = channels * dim; for (int n = 0; n < num; n++){ // just drop the padding derivatives part. caffe_copy(channel_by_dim, top_diff, bottom_diff); top_diff += (channels + num_channels_to_pad_) * dim; bottom_diff += channel_by_dim; } } INSTANTIATE_CLASS(PadChannelLayer); REGISTER_LAYER_CLASS(PadChannel); } // namespace caffe
pad_channel_layer.cu
#include "caffe/layers/pad_channel_layer.hpp"namespace caffe { // Copy (one line per thread) from one array to another, with arbitrary// strides in the last two dimensions.template <typename Dtype> __global__ void pad_forward_kernel(constint dst_count, constint src_channels, constint dst_channels, constint dim, const Dtype* src, Dtype* dst) { CUDA_KERNEL_LOOP(index, dst_count) { int num = index / (dim * dst_channels); int dst_c = index / dim % dst_channels; int pixel_pos = index % dim; if (dst_c < src_channels) dst[index] = src[num * src_channels * dim + dst_c * dim + pixel_pos]; else dst[index] = Dtype(0); } } template <typename Dtype> void PadChannelLayer<Dtype>::Forward_gpu(constvector<Blob<Dtype>*>& bottom, constvector<Blob<Dtype>*>& top){ const Dtype* bottom_data = bottom[0]->gpu_data(); Dtype* top_data = top[0]->mutable_gpu_data(); int src_channels = bottom[0]->channels(); int dim = bottom[0]->height() * bottom[0]->width(); int dst_channels = src_channels + num_channels_to_pad_; constint dst_count = top[0]->count(); pad_forward_kernel<Dtype> << <CAFFE_GET_BLOCKS(dst_count), CAFFE_CUDA_NUM_THREADS >> >( dst_count, src_channels, dst_channels, dim, bottom_data, top_data); CUDA_POST_KERNEL_CHECK; } template <typename Dtype> __global__ void pad_backward_kernel(constint bottom_count, constint bottom_channels, constint top_channels, constint dim, const Dtype* top, Dtype* bottom) { CUDA_KERNEL_LOOP(index, bottom_count) { int num = index / (dim * bottom_channels); int bottom_c = index / dim % bottom_channels; int pixel_pos = index % dim; bottom[index] = top[num * top_channels * dim + bottom_c * dim + pixel_pos]; } } template <typename Dtype> void PadChannelLayer<Dtype>::Backward_gpu(constvector<Blob<Dtype>*>& top, constvector<bool>& propagate_down, constvector<Blob<Dtype>*>& bottom) { const Dtype* top_diff = top[0]->gpu_diff(); Dtype* bottom_diff = bottom[0]->mutable_gpu_diff(); int bottom_count = bottom[0]->count(); int bottom_channels = bottom[0]->channels(); int dim = bottom[0]->height() * bottom[0]->width(); int top_channels = bottom_channels + num_channels_to_pad_; pad_backward_kernel<Dtype> << <CAFFE_GET_BLOCKS(bottom_count), CAFFE_CUDA_NUM_THREADS >> >( bottom_count, bottom_channels, top_channels, dim, top_diff, bottom_diff); CUDA_POST_KERNEL_CHECK; } INSTANTIATE_LAYER_GPU_FUNCS(PadChannelLayer); } // namespace caffe
1、 使用上述脚本针对Cifar10生成20层的resnet。网络定义太长,这里就不给出来了,但要注意的是因为原图是32x32大小的,这里随机crop 28x28的,并镜像一下。不同于原论文所述的在图片各边补4个像素再随机裁剪32x32的。网络定义的输入部分以及solver.prototxt:
name: "resnet_cifar10" layer{ name: "input" type: "Data" top: "Data1" top: "label" include { phase: TRAIN } transform_param{ mean_file: "dataset/mean.proto" crop_size: 28 mirror: true } data_param{ source: "dataset/cifar10_train_lmdb" batch_size: 100 backend: LMDB } } layer{ name: "input" type: "Data" top: "Data1" top: "label" include { phase: TEST } transform_param{ mean_file: "dataset/mean.proto" crop_size: 28 } data_param{ source: "dataset/cifar10_test_lmdb" batch_size: 100 backend: LMDB } }
net: "res20_cifar_train_test.prototxt"test_iter: 100 # conver the whole test set. 100 * 100 = 10000 images.test_interval: 500 # Each 500 is one epoch, test after each epochbase_lr: 0.1momentum: 0.9weight_decay: 0.0001average_loss: 100lr_policy: "multistep"stepvalue: 40000stepvalue: 80000gamma: 0.1display: 100max_iter: 100000 # 100000 iteration is 200 epochssnapshot: 500snapshot_prefix: "trained_model/cifar"solver_mode: GPU
cigar 10 20层的训练和测试曲线
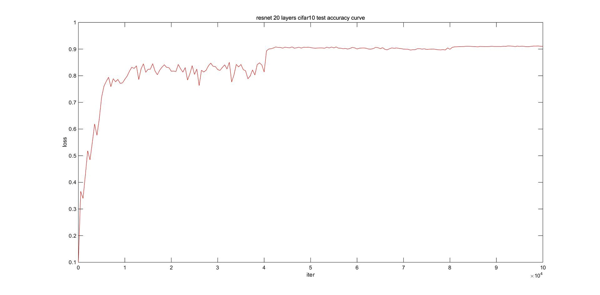