Predicting Materials Properties with Little Data Using Shotgun Transfer Learning
基于迁移学习的少量数据预测材料性能
2019年-ACS central science
DOI: 10.1021/acscentsci.9b00804
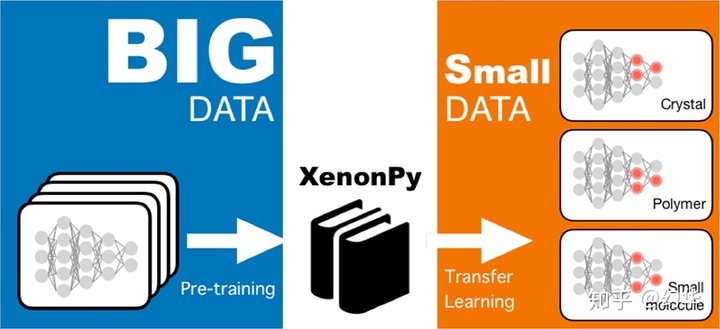
l 本文的逻辑是什么?
机器学习能够快速评估材料性能, 然而目前材料的相关数据在数量和广度的缺乏限制了机器学习的作用, 且这种局势短时间内无法改变. 所以将迁移学习引入, 可以在克服这种情况. 使用少量数据便可预测材料性能
l 文章要解决的问题是什么?
the insufficient volume and diversity of materials data, 但是短时间内不能够解决
transfer learning to overcome the problem of limited amounts of materials data
l 与目标问题相关的研究背景和研究现状是?现有解决方案的缺点是什么?
transfer learning relies on the concept that various property types are physically interrelated
l 文章主要的出发点和思路是什么?论文工作的新颖性体现在?
- 出发点: 是解决现有的少量数据形成模型的困难
- 思路: 使用迁移学习,在大量数据集上学习到共同特征,运用于少量数据集网络的训练
- 目的: to facilitate widespread use of transfer learning, we develop a pretrained model library called XenonPy.MDL.
- 迁移学习可以跨越材料科学不同的学课的不同性质之间使用
l 作者解决目标问题采用的核心方法?如何进行测试,是否有说服力?
XenonPy.MDL
140000 pretrained neural networks
1000 gradient boosting models
16000 pretrained random forests
material types
- small molecules, 15 properties
- polymers, 18 properties
- inorganic crystalline materials, 12 properties
classification models to discriminate 226 space group or 32 point group of crystalline materials
The library also incorporates models success-fully transferred from some of the source models.
For each source task, generated ~1000 neural networks with randomly constructed network structures
使用bootstrap抽样来增加模型多样性
TL
迁移学习的两种策略
- frozen featurizer
- fine-tuning
The prediction performance of a transferred model depends onther choice of source properties,sourcedata,and architectures of the neural networks.
迁移学习的performance不仅仅关乎替代训练的目标和原目标之间的关联程度,而且与网络架构有关, need candidate pool of source moderls to identify a best model
验证
- Prediction of Polymeric Heat Capacity
- prediction model
- Target: the specific heat capacity at constant pressure ($C_P$),
- input: molecular fingerprint
- source: $C_V$ for small organic molecules
- 验证:5折交叉验证, 通过专家知识分类的六折交叉验证
- Thermal Conductivity of Organic Polymers
- target: Thermal Conductivity of Organic Polymers
- source: $C_V$
- Thermal Conductivity of Inorganic Crystals
- target:
- source: SPS
- Transferability across Organic and Inorganic Materials
- target: n(refractive index)
**注意**
源任务中最佳预测的预训练模型在目标任务中的可迁移性并不最佳
迁移的另一种简单的替代方法是将source与target进行回归