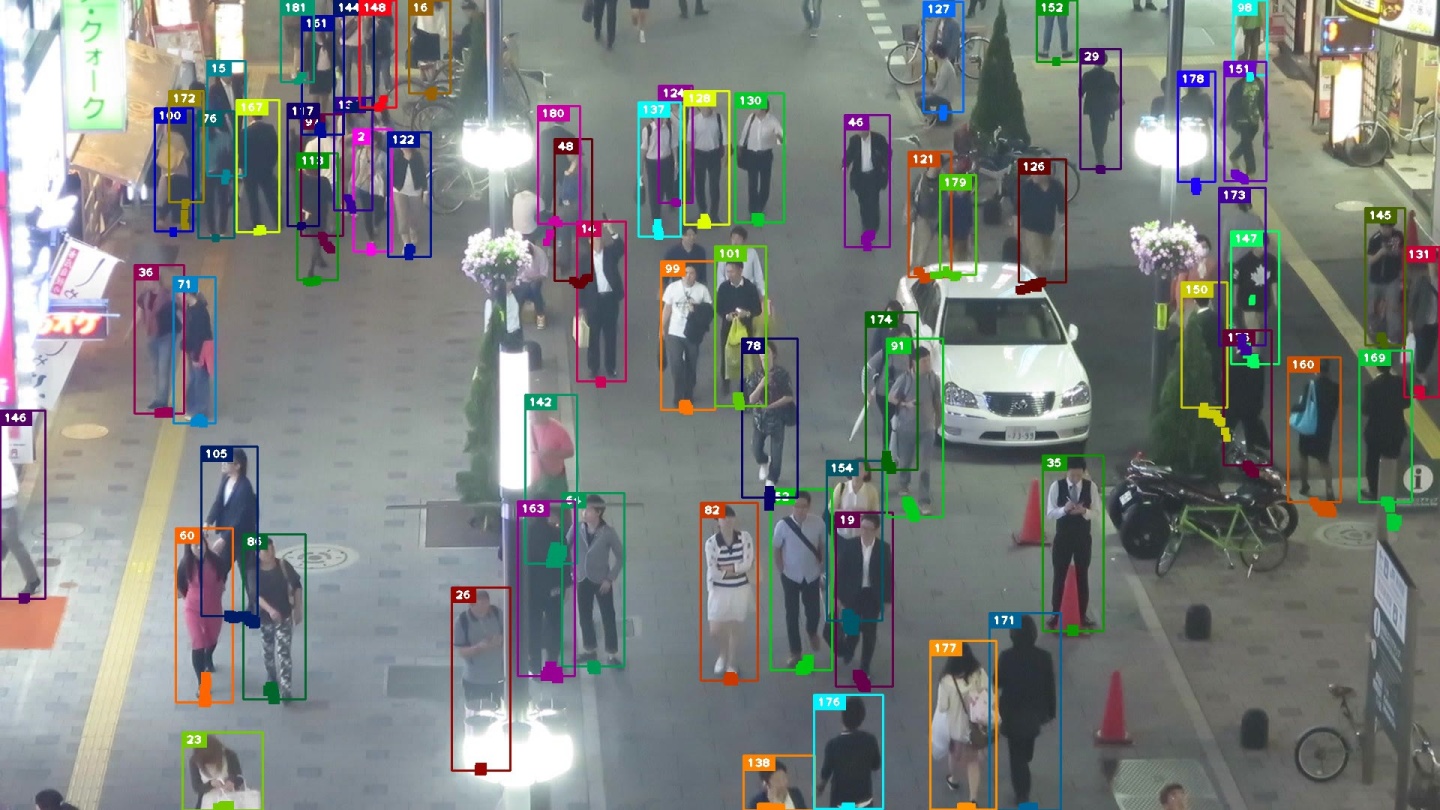
这段时间在整理毕设,所以这里结合了SpyderXu 分享的内容把多目标跟踪相关的文献资源共享一下,由于文章很多,所以我这里只整理3年以内的,对于年限久远的,这里只取提供了代码的和比较经典的。各自算法的性能比较可以看论文以及MOT官网。由于知乎不支持表格,所以具体见我的博客或github(提供了算法在MOT数据集上的简称以及期刊会议名称)。关于最新的论文,我会优先在github上更新,知乎不提供markdown表格,很麻烦。
以下每栏内容为:文章名、文章和代码链接、文章发布位置(期刊会议)、文章对应简称。当前MOT数据集上排名靠前的都加粗了。(收藏的同时别忘了点赞哦~)
在线跟踪(Online)
- DeepMOT:A Differentiable Framework for Training Multiple Object Trackers |[pdf] [code] | CVPR2020 | DeepMOT
- Online multiple pedestrian tracking using deep temporal appearance matching association | [pdf] [code] | arXiv(2019) | DD_TAMA19
- Spatial-temporal relation networks for multi-object tracking | [pdf] | ICCV2019 | STRN
- Towards Real-Time Multi-Object Tracking | [pdf] [code] | arXiv(2019) | JDE(private)
- Multi-object tracking with multiple cues and switcher-aware classification | [pdf] | arXiv(2019) | LSST
- FAMNet: Joint learning of feature, affinity and multi-dimensional assignment for online multiple object tracking | [pdf] | ICCV2019 | FAMNet
- Online multi-object tracking with instance-aware tracker and dynamic model refreshment | [pdf] | WACV2019 | KCF
- Tracking without bells and whistles | [pdf] [code] | ICCV2019 | Tracktor
- Deep affinity network for multiple object tracking | [pdf][code] | PAMI(2019) | DAN
- MOTS: Multi-Object Tracking and Segmentation | [pdf] [code] | CVPR2019 | Track R-CNN
- Eliminating Exposure Bias and Metric Mismatch in Multiple Object Tracking | [pdf] | CVPR2019 | SAS_MOT17
- Recurrent autoregressive networks for online multi-object tracking | [pdf] | WACV2018 | RAN
- Real-time multiple people tracking with deeply learned candidate selection and person re-identification | [pdf] [code] | ICME2018 | MOTDT
- Online multi-object tracking with dual matching attention networks | [pdf] [code] | ECCV2018 | DMAN
- Extending IOU Based Multi-Object Tracking by Visual Information | [pdf] [code] | AVSS2018 | V-IOU
- Online Multi-target Tracking using Recurrent Neural Networks | [pdf] [code] | AAAI2017 | MOT-RNN
- Detect to Track and Track to Detect | [pdf] [code] | ICCV2017 | D&T(private)
- Online multi-object tracking using CNN-based single object tracker with spatial-temporal attention mechanism | [pdf] | ICCV2017 | STAM
- Tracking the untrackable: Learning to track multiple cues with long-term dependencies | [pdf] | ICCV2017 | AMIR
- Simple online and realtime tracking with a deep association metric | [pdf] [code] | ICIP2017 | DeepSort
- High-speed tracking-by-detection without using image information | [pdf] [code] | AVSS2017 | IOU Tracker
- Simple online and realtime tracking | [pdf] [code] | ICIP2016 | Sort
- Temporal dynamic appearance modeling for online multi-person tracking | [pdf] | CVIU(2016) | TDAM
- Online multi-object tracking via structural constraint event aggregation | [pdf] | CVPR2016 |SCEA
- Online Multi-Object Tracking Via Robust Collaborative Model and Sample Selection | [pdf] [code] | CVIU2016 | RCMSS
- Learning to Track: Online Multi-Object Tracking by Decision Making | [pdf] [code] | ICCV2015 | MDP
- Learning to Divide and Conquer for Online Multi-Target Tracking | [pdf][code] | ICCV2015 | LDCT
- Robust online multi-object tracking based on tracklet confidence and online discriminative appearance learning | [pdf] [code] | CVPR2014 | CMOT
- The Way They Move: Tracking Targets with Similar Appearance | [pdf] [code] | ICCV2013 | SMOT
- Online Multi-Person Tracking by Tracker Hierarchy | [pdf] [code] | AVSS2012 | OMPTTH
离线跟踪(Batch)
- Learning non-uniform hypergraph for multi-object tracking | [pdf] | AAAI2019 | NT
- Learning a Neural Solver for Multiple Object Tracking | [pdf] [code] | CVPR2020 | MPNTracker
- Deep learning of graph matching | [pdf] | CVPR2018
- muSSP: Efficient Min-cost Flow Algorithm for Multi-object Tracking | [pdf] [code] | NIPS(2019) | muSSP
- Exploit the connectivity: Multi-object tracking with trackletnet | [pdf] [code] | ACM mm 2019 | TNT(eTC)
- Multiple people tracking using body and joint detections | [pdf] | CVPRW2019 | JBNOT
- Aggregate Tracklet Appearance Features for Multi-Object Tracking | [pdf] | SPL(2019) | NOTA
- Customized multi-person tracker | [pdf] | ACCV2018 | HCC
- Multi-object tracking with neural gating using bilinear lstm |[pdf] | ECCV2018 | MHT_bLSTM
- Trajectory Factory: Tracklet Cleaving and Re-connection by Deep Siamese Bi-GRU for Multiple Object Tracking | [pdf] | ICME2018 |GCRE
- Multiple People Tracking with Lifted Multicut and Person Re-identification | [pdf] | CVPR2017 | LMP
- Deep network flow for multi-object tracking | [pdf] | CVPR2017
- Non-markovian globally consistent multi-object tracking | [pdf] [code] | ICCV2017
- Multi-Object Tracking with Quadruplet Convolutional Neural Networks | [pdf] | CVPR2017 | Quad-CNN
- Enhancing detection model for multiple hypothesis tracking | [pdf] | CVPRW2017 | EDMT
- POI: Multiple Object Tracking with High Performance Detection and Appearance Feature | [pdf] | ECCV2016 | KNDT
- Multiple hypothesis tracking revisited | [pdf] [code] | ICCV2015 | MHT-DAM
- Near-Online Multi-target Tracking with Aggregated Local Flow Descriptor | [pdf] | ICCV2015 | NOMT
- On Pairwise Costs for Network Flow Multi-Object Tracking | [pdf] [code]| CVPR2015
- Multiple Target Tracking Based on Undirected Hierarchical Relation Hypergraph | [pdf] [code] | CVPR2014 | H2T
- Continuous Energy Minimization for Multi-Target Tracking | [pdf] [code] | CVPR2014 | CEM
- GMCP-Tracker: Global Multi-object Tracking Using Generalized Minimum Clique Graphs | [pdf] [code] | ECCV2012 | GMCP
- Multiple Object Tracking using K-Shortest Paths Optimization | [pdf] [code] | PAMI2011 | KSP
- Global data association for multi-object tracking using network flows | [pdf] [code] | CVPR2008
跨摄像头跟踪(MTMC)
- CityFlow: A City-Scale Benchmark for Multi-Target Multi-Camera Vehicle Tracking and Re-Identification | [pdf] | CVPR2019 | CityFlow
- Features for multi-target multi-camera tracking and re-identification | [pdf] [code] | CVPR2018 | DeepCC(MTMC)
- Rolling Shutter and Radial Distortion Are Features for High Frame Rate Multi-Camera Tracking | [pdf] | CVPR2018
- Towards a Principled Integration of Multi-Camera Re-Identification andTracking through Optimal Bayes Filters | [pdf] [code] | CVPR2017 | towards-reid-tracking
3D&多模态跟踪
- Robust Multi-Modality Multi-Object Tracking | [pdf] [code] | ICCV2019 | mmMOT
- A baseline for 3D Multi-Object Tracking | [pdf] [code]
- MOTSFusion: Track to Reconstruct and Reconstruct to Track | [pdf] [code] |ICRA2020 & RA-L
综述
Multiple Object Tracking: A Literature Review
Machine Learning Methods for Solving Assignment Problems in Multi-Target Tracking
Deep Learning in Video Multi-Object Tracking_ A Survey
Globally-Optimal Greedy Algorithms for Tracking a Variable Number of Objects
数据集
MOT:包含2D MOT2015、3D MOT2015、MOT16、MOT17和MOT17Det等多个子数据集,提供了ACF、DPM、Faster RCNN、SDP等多个检测器输入。包含不同的相机视角、相机运动、场景和时间变化以及密集场景。
KITTI:提供了汽车和行人的标注,场景较稀疏。
TUD Stadtmitte:包含3D人体姿态识别、多视角行人检测和朝向检测、以及行人跟踪的标注,相机视角很低,数据集不大。
ETHZ:由手机拍摄的多人跟踪数据集,包含三个场景。
EPFL:多摄像头采集的行人检测和跟踪数据集,每隔摄像头离地2米,实验人员就是一个实验室的,分为实验室、校园、平台、通道、篮球场这5个场景,每个场景下都有多个摄像头,每个摄像头拍摄2分钟左右。
KIT AIS:空中拍摄的,只有行人的头
PETS:比较早期的视频,有各式各样的行人运动。
DukeMTMC:多摄像头多行人跟踪。
MOTS:多目标跟踪与分割。
评价体系
ClearMOT
IDF1
Code: python、matlab
2020.4.3更新
上面的DeepMOT和MPN Tracker都被CVPR2020录用了,跟Tracktor++都是同一个作者团队,有新增了一些MOT榜前的论文和代码:
Tracking Objects as Points | [pdf][code] | arXiv(2019) | CenterTrack
Refinements in Motion and Appearance for Online Multi-Object Tracking| [pdf][code] | arXiv(2019) | MIFT
Multiple Object Tracking by Flowing and Fusing |[pdf] |arXiv(2019) |FFT
A Unified Object Motion and Affinity Model for Online Multi-Object Tracking |[pdf][code] |CVPR2020 |UMA
完整的在github上:
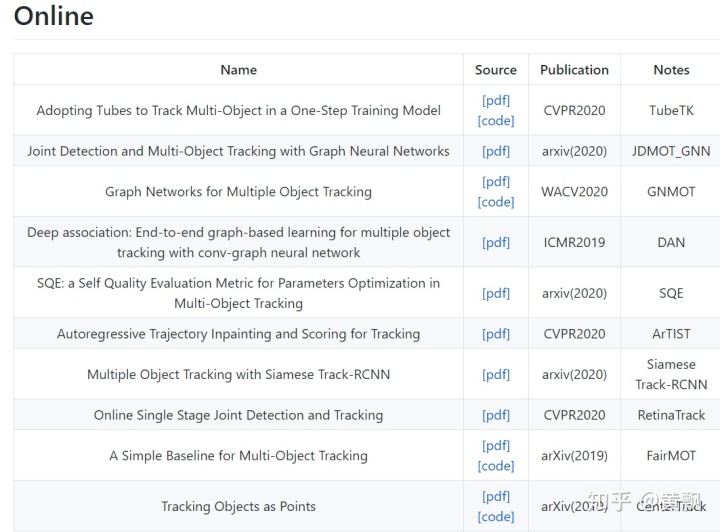