本笔记将会对下面3点进行解释
1 torch.data tensor.detach()
2 nn.ModuleList nn.Sequential
3 tensor.view tensor.reshape
1 torch.data tensor.detach()
detach()保证求导结果是正确的
import torch
a = torch.tensor([1,2,3.], requires_grad = True)
out = a.sigmoid()
c = out.detach()
print(c.zero_()) # modified by c.zero_(),out已经被修改了,求导的结果肯定不正确,所以应该报错
out.sum().backward()
报错:RuntimeError: one of the variables needed for gradient computation has been modified by an inplace operation
import torch
a = torch.tensor([1,2,3.], requires_grad = True)
out = a.sigmoid()
c = out.data
print(c.zero_()) # modified by c.zero_(),out已经被修改了,求导的结果肯定不正确,但没有报错
out.sum().backward() # # The result is very, very wrong because `out` changed!
不会报错,但结果是错的
2 nn.ModuleList nn.Sequential
When should I use nn.ModuleList and when should I use nn.Sequential?discuss.pytorch.org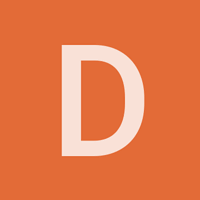
nn.ModuleList类似python的list,只不过在pytorch能感知nn.ModuleList中的参数而感知不了list中的参数。即如果我们想在pytorch中使用list,则可以考虑使用nn.ModuleList。
nn.Sequential中的module则是级联的,也就是这一层的输出必须与下一层的输入相对应,而nn.ModuleList只是用来存储module。
- nn.ModuleList 和 nn.Sequential
# nn.Sequential
import torch
import torch.nn as nn
import numpy as np
x_np = np.arange(1, 244).reshape(-1, 3, 9, 9)
x = torch.tensor(x_np).float()
sequential = nn.Sequential(
nn.Conv2d(3, 32, 3, 1, 0),
nn.Conv2d(32, 64, 3, 1, 0)
)
print(module_list)
能正常运行
import torch
import torch.nn as nn
import numpy as np
x_np = np.arange(1, 244).reshape(-1, 3, 9, 9)
x = torch.tensor(x_np).float()
module_list = nn.ModuleList()
module_list.append(nn.Conv2d(3, 32, 3, 1, 0))
module_list.append(nn.Conv2d(32, 64, 3, 1, 0))
print(module_list)
z = module_list(x)
print(z)
会报错NotImplementedError,因为module_list没有forward(),如果像下面这样就不会报错了
import torch
import torch.nn as nn
import numpy as np
x_np = np.arange(1, 244).reshape(-1, 3, 9, 9)
x = torch.tensor(x_np).float()
module_list = nn.ModuleList()
module_list.append(nn.Conv2d(3, 32, 3, 1, 0))
module_list.append(nn.Conv2d(32, 64, 3, 1, 0))
print(module_list)
z1 = module_list[0](x) # 相当于自己组织module
z2 = module_list[1](z1)
print(z2)
3 tensor.view tensor.reshape
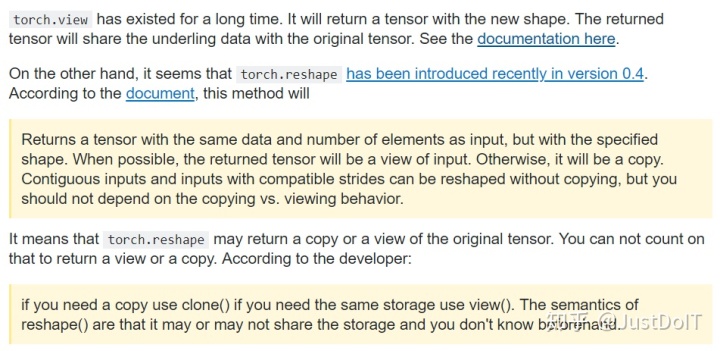
- 是否共享内存
tensor.view()生成一个新的tensor,并且这个新的tensor会和原来的tensor共享内存,改变一个tensor,另一个tensor也会改变。
tesor.reshape()生成一个新的tensor,但这个tensor可能会和原来的tensor共享内存,也可能不会。
import torch
# view
x = torch.tensor([[1, 2, 3], [4, 5, 6], [7, 8, 9]])
y = x.view(-1, 1)
y[0][0] = 5
print(x)
print(y)
可以看到改变了y的值,x的值也改变了
- 是否要求数据连续
tensor.view
tensor.transpose之后数据不连续了,而tensor.view要求数据是连续的(contiguous),如果不连续则会报错
import
可见,transpose之后数据不连续了,如果要使用view,则必须调用contiguous()
import torch
x = torch.tensor([[1, 2, 3], [4, 5, 6], [7, 8, 9]])
# 数据是否连续
print(x.is_contiguous()) # True
# transpose
x = x.transpose(1, 0)
print(x.is_contiguous()) # False
# contiguous
x = x.contiguous()
print(x.is_contiguous()) # True
# view
x = x.view(-1, 1)
tensor.reshape
而在tanspose之后,使用tensor.reshape则不会报错
import torch
x = torch.tensor([[1, 2, 3], [4, 5, 6], [7, 8, 9]])
# 数据是否连续
print(x.is_contiguous())
# transpose
x = x.transpose(1, 0)
print(x.is_contiguous())
# reshape
x = x.reshape(-1, 1)