注意:单击此处https://urlify.cn/3iAzUr下载完整的示例代码,或通过Binder在浏览器中运行此示例
下 图 说明了聚类数量和样本数量对各种聚类性能评估度量指标的影响。 未调整的度量指标(例如V度量)显示了聚类的数量与样本数之间的依赖关系:随机标记的平均V度量随着聚类的数量越接近用于计算的样本总数而显着增加。 针对ARI等偶然性度量指标进行调整后,对于任意数量的样本和聚类,一些随机方差(variations)均以0.0的平均得分为中心。 因此,只有调整后的度量指标才能安全地用作共识指数(consensus index),才能用来评估数据集中在各种重叠子样本上给定k值时,聚类算法的平均稳定性。Computing adjusted_rand_score for 10 values of n_clusters and n_samples=100
done in 0.050s
Computing v_measure_score for 10 values of n_clusters and n_samples=100
done in 0.068s
Computing ami_score for 10 values of n_clusters and n_samples=100
done in 0.356s
Computing mutual_info_score for 10 values of n_clusters and n_samples=100
done in 0.044s
Computing adjusted_rand_score for 10 values of n_clusters and n_samples=1000
done in 0.051s
Computing v_measure_score for 10 values of n_clusters and n_samples=1000
done in 0.064s
Computing ami_score for 10 values of n_clusters and n_samples=1000
done in 0.208s
Computing mutual_info_score for 10 values of n_clusters and n_samples=1000
done in 0.048s
print(__doc__)
# 作者: Olivier Grisel
# 许可证: BSD 3 clause
import numpy as np
import matplotlib.pyplot as plt
from time import time
from sklearn import metrics
def uniform_labelings_scores(score_func, n_samples, n_clusters_range,
fixed_n_classes=None, n_runs=5, seed=42):
"""计算2个随机均一聚类标签的得分。
两个随机标签中每个在n_clusters_range中的可能值都具有相同数量的聚类。
当fixed_n_classes不为None时,第一个标签被认为是具有固定类数量的真实类(ground truth class)。
"""
random_labels = np.random.RandomState(seed).randint
scores = np.zeros((len(n_clusters_range), n_runs))
if fixed_n_classes is not None:
labels_a = random_labels(low=0, high=fixed_n_classes, size=n_samples)
for i, k in enumerate(n_clusters_range):
for j in range(n_runs):
if fixed_n_classes is None:
labels_a = random_labels(low=0, high=k, size=n_samples)
labels_b = random_labels(low=0, high=k, size=n_samples)
scores[i, j] = score_func(labels_a, labels_b)
return scores
def ami_score(U, V):
return metrics.adjusted_mutual_info_score(U, V)
score_funcs = [
metrics.adjusted_rand_score,
metrics.v_measure_score,
ami_score,
metrics.mutual_info_score,
]
# 2个独立的随机聚类,具有相同的聚类数
n_samples = 100
n_clusters_range = np.linspace(2, n_samples, 10).astype(np.int)
plt.figure(1)
plots = []
names = []
for score_func in score_funcs:
print("Computing %s for %d values of n_clusters and n_samples=%d"
% (score_func.__name__, len(n_clusters_range), n_samples))
t0 = time()
scores = uniform_labelings_scores(score_func, n_samples, n_clusters_range)
print("done in %0.3fs" % (time() - t0))
plots.append(plt.errorbar(
n_clusters_range, np.median(scores, axis=1), scores.std(axis=1))[0])
names.append(score_func.__name__)
plt.title("Clustering measures for 2 random uniform labelings\n"
"with equal number of clusters")
plt.xlabel('Number of clusters (Number of samples is fixed to %d)' % n_samples)
plt.ylabel('Score value')
plt.legend(plots, names)
plt.ylim(bottom=-0.05, top=1.05)
# 根据真实类标签使用不同的n_clusters随机标签
# 聚类数量固定
n_samples = 1000
n_clusters_range = np.linspace(2, 100, 10).astype(np.int)
n_classes = 10
plt.figure(2)
plots = []
names = []
for score_func in score_funcs:
print("Computing %s for %d values of n_clusters and n_samples=%d"
% (score_func.__name__, len(n_clusters_range), n_samples))
t0 = time()
scores = uniform_labelings_scores(score_func, n_samples, n_clusters_range,
fixed_n_classes=n_classes)
print("done in %0.3fs" % (time() - t0))
plots.append(plt.errorbar(
n_clusters_range, scores.mean(axis=1), scores.std(axis=1))[0])
names.append(score_func.__name__)
plt.title("Clustering measures for random uniform labeling\n"
"against reference assignment with %d classes" % n_classes)
plt.xlabel('Number of clusters (Number of samples is fixed to %d)' % n_samples)
plt.ylabel('Score value')
plt.ylim(bottom=-0.05, top=1.05)
plt.legend(plots, names)
plt.show()
脚本的总运行时间:(0分钟1.225秒)
估计的内存使用量: 8 MB
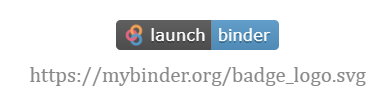
下载Python源代码: plot_adjusted_for_chance_measures.py
下载Jupyter notebook源代码: plot_adjusted_for_chance_measures.ipynb
由Sphinx-Gallery生成的画廊
文壹由“伴编辑器”提供技术支持
☆☆☆为方便大家查阅,小编已将scikit-learn学习路线专栏 文章统一整理到公众号底部菜单栏,同步更新中,关注公众号,点击左下方“系列文章”,如图:欢迎大家和我一起沿着scikit-learn文档这条路线,一起巩固机器学习算法基础。(添加微信:mthler,备注:sklearn学习,一起进【sklearn机器学习进步群】开启打怪升级的学习之旅。)