注意:单击此处https://urlify.cn/NV3Erq下载完整的示例代码,或通过Binder在浏览器中运行此示例
此示例显示了在二维模式下的数据集上层次聚类(hierarchical clustering)的不同链接方法的特征。 主要观察到的有:- 单链接的速度很快,并且可以在非球形数据上表现良好,但是在存在噪声的情况下,效果表现较差。
- 平均链接和完全链接在分离干净的球状上表现良好,但在其他方面却有不同的结果。
- Ward是处理有噪声数据的最有效方法。
print(__doc__)
import time
import warnings
import numpy as np
import matplotlib.pyplot as plt
from sklearn import cluster, datasets
from sklearn.preprocessing import StandardScaler
from itertools import cycle, islice
np.random.seed(0)
生成数据集:我们选择足够大的样本数量以查看算法的可扩展性,但又不能设置太大,以避免运行时间太长。
n_samples = 1500
noisy_circles = datasets.make_circles(n_samples=n_samples, factor=.5,
noise=.05)
noisy_moons = datasets.make_moons(n_samples=n_samples, noise=.05)
blobs = datasets.make_blobs(n_samples=n_samples, random_state=8)
no_structure = np.random.rand(n_samples, 2), None
# 各向异性(Anisotropicly)分布的数据
random_state = 170
X, y = datasets.make_blobs(n_samples=n_samples, random_state=random_state)
transformation = [[0.6, -0.6], [-0.4, 0.8]]
X_aniso = np.dot(X, transformation)
aniso = (X_aniso, y)
# 具有变化的斑点(blobs)
varied = datasets.make_blobs(n_samples=n_samples,
cluster_std=[1.0, 2.5, 0.5],
random_state=random_state)
运行聚类并绘图
# 设置聚类参数
plt.figure(figsize=(9 * 1.3 + 2, 14.5))
plt.subplots_adjust(left=.02, right=.98, bottom=.001, top=.96, wspace=.05,
hspace=.01)
plot_num = 1
default_base = {'n_neighbors': 10,
'n_clusters': 3}
datasets = [
(noisy_circles, {'n_clusters': 2}),
(noisy_moons, {'n_clusters': 2}),
(varied, {'n_neighbors': 2}),
(aniso, {'n_neighbors': 2}),
(blobs, {}),
(no_structure, {})]
for i_dataset, (dataset, algo_params) in enumerate(datasets):
# 使用数据集特定的值更新参数
params = default_base.copy()
params.update(algo_params)
X, y = dataset
# 标准化数据集,以便更轻松地选择参数
X = StandardScaler().fit_transform(X)
# ============
# 创建聚类对象
# ============
ward = cluster.AgglomerativeClustering(
n_clusters=params['n_clusters'], linkage='ward')
complete = cluster.AgglomerativeClustering(
n_clusters=params['n_clusters'], linkage='complete')
average = cluster.AgglomerativeClustering(
n_clusters=params['n_clusters'], linkage='average')
single = cluster.AgglomerativeClustering(
n_clusters=params['n_clusters'], linkage='single')
clustering_algorithms = (
('Single Linkage', single),
('Average Linkage', average),
('Complete Linkage', complete),
('Ward Linkage', ward),
)
for name, algorithm in clustering_algorithms:
t0 = time.time()
# 捕获与kneighbors_graph有关的警告
with warnings.catch_warnings():
warnings.filterwarnings(
"ignore",
message="the number of connected components of the " +
"connectivity matrix is [0-9]{1,2}" +
" > 1. Completing it to avoid stopping the tree early.",
category=UserWarning)
algorithm.fit(X)
t1 = time.time()
if hasattr(algorithm, 'labels_'):
y_pred = algorithm.labels_.astype(np.int)
else:
y_pred = algorithm.predict(X)
plt.subplot(len(datasets), len(clustering_algorithms), plot_num)
if i_dataset == 0:
plt.title(name, size=18)
colors = np.array(list(islice(cycle(['#377eb8', '#ff7f00', '#4daf4a',
'#f781bf', '#a65628', '#984ea3',
'#999999', '#e41a1c', '#dede00']),
int(max(y_pred) + 1))))
plt.scatter(X[:, 0], X[:, 1], s=10, color=colors[y_pred])
plt.xlim(-2.5, 2.5)
plt.ylim(-2.5, 2.5)
plt.xticks(())
plt.yticks(())
plt.text(.99, .01, ('%.2fs' % (t1 - t0)).lstrip('0'),
transform=plt.gca().transAxes, size=15,
horizontalalignment='right')
plot_num += 1
plt.show()
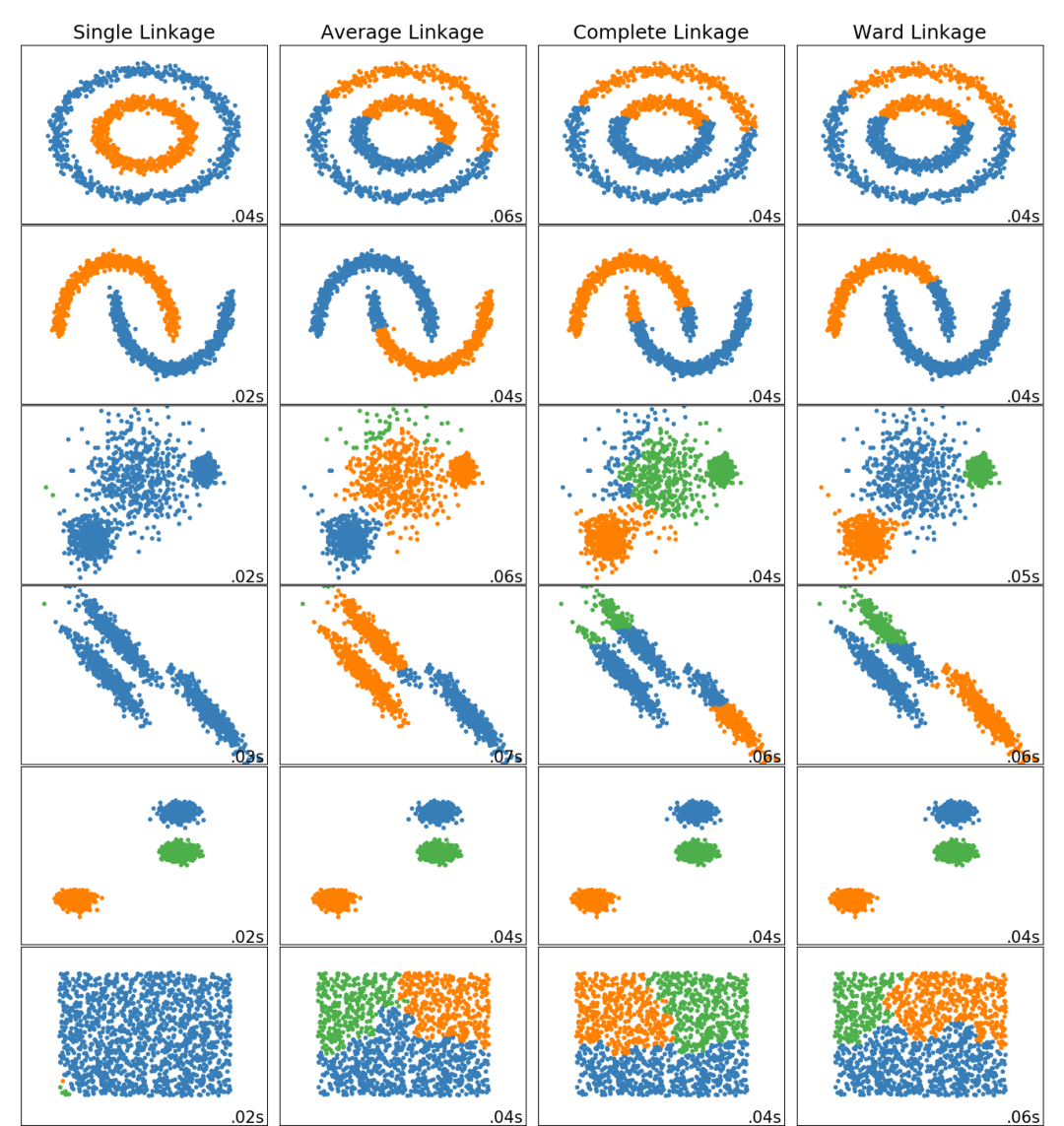
下载Python源代码: plot_linkage_comparison.py
下载Jupyter notebook源代码: plot_linkage_comparison.ipynb
由Sphinx-Gallery生成的画廊
文壹由“伴编辑器”提供技术支持
☆☆☆为方便大家查阅,小编已将scikit-learn学习路线专栏 文章统一整理到公众号底部菜单栏,同步更新中,关注公众号,点击左下方“系列文章”,如图:欢迎大家和我一起沿着scikit-learn文档这条路线,一起巩固机器学习算法基础。(添加微信:mthler,备注:sklearn学习,一起进【sklearn机器学习进步群】开启打怪升级的学习之旅。)