概率PCA和因子分析都是概率模型,新数据的似然性(likelihood)可用于模型选择和协方差估计。在这里,我们将在均等噪声(每个特征的噪声方差相同)或异方差噪声(每个特征的噪声方差均不同)损坏的低秩(low rank)数据上进行交叉验证比较PCA和FA,然后我们将模型似然性与从收缩协方差估计器中获得的似然性进行比较。
可以观察到,在均等噪声的情况下,FA和PCA都成功地恢复了低秩子空间的大小,但PCA的似然性高于FA;而当存在异方差噪声时,PCA会高估了秩(rank)。在适当情况下,低秩模型比收缩模型的性能更高。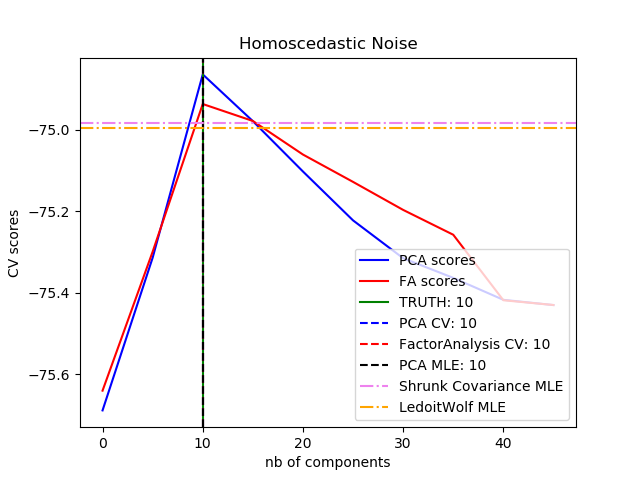
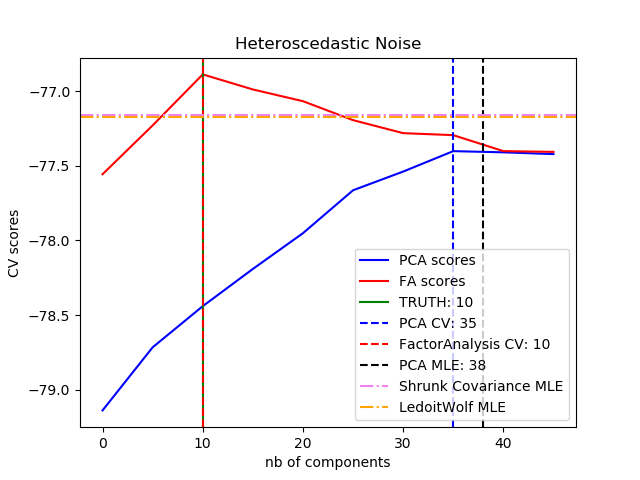
best n_components by PCA CV = 10
best n_components by FactorAnalysis CV = 10
best n_components by PCA MLE = 10
best n_components by PCA CV = 35
best n_components by FactorAnalysis CV = 10
best n_components by PCA MLE = 38
# 作者:Alexandre Gramfort# Denis A. Engemann# 许可证:BSD 3 clauseimport numpy as npimport matplotlib.pyplot as pltfrom scipy import linalgfrom sklearn.decomposition import PCA, FactorAnalysisfrom sklearn.covariance import ShrunkCovariance, LedoitWolffrom sklearn.model_selection import cross_val_scorefrom sklearn.model_selection import GridSearchCV
print(__doc__)# ############################################################################## 创建数据
n_samples, n_features, rank = 1000, 50, 10
sigma = 1.
rng = np.random.RandomState(42)
U, _, _ = linalg.svd(rng.randn(n_features, n_features))
X = np.dot(rng.randn(n_samples, rank), U[:, :rank].T)# 添加均等噪声
X_homo = X + sigma * rng.randn(n_samples, n_features)# 添加异方差噪声
sigmas = sigma * rng.rand(n_features) + sigma / 2.
X_hetero = X + rng.randn(n_samples, n_features) * sigmas# ############################################################################## 拟合模型
n_components = np.arange(0, n_features, 5) # options for n_componentsdef compute_scores(X):
pca = PCA(svd_solver='full')
fa = FactorAnalysis()
pca_scores, fa_scores = [], []for n in n_components:
pca.n_components = n
fa.n_components = n
pca_scores.append(np.mean(cross_val_score(pca, X)))
fa_scores.append(np.mean(cross_val_score(fa, X)))return pca_scores, fa_scoresdef shrunk_cov_score(X):
shrinkages = np.logspace(-2, 0, 30)
cv = GridSearchCV(ShrunkCovariance(), {'shrinkage': shrinkages})return np.mean(cross_val_score(cv.fit(X).best_estimator_, X))def lw_score(X):return np.mean(cross_val_score(LedoitWolf(), X))for X, title in [(X_homo, 'Homoscedastic Noise'),
(X_hetero, 'Heteroscedastic Noise')]:
pca_scores, fa_scores = compute_scores(X)
n_components_pca = n_components[np.argmax(pca_scores)]
n_components_fa = n_components[np.argmax(fa_scores)]
pca = PCA(svd_solver='full', n_components='mle')
pca.fit(X)
n_components_pca_mle = pca.n_components_
print("best n_components by PCA CV = %d" % n_components_pca)
print("best n_components by FactorAnalysis CV = %d" % n_components_fa)
print("best n_components by PCA MLE = %d" % n_components_pca_mle)
plt.figure()
plt.plot(n_components, pca_scores, 'b', label='PCA scores')
plt.plot(n_components, fa_scores, 'r', label='FA scores')
plt.axvline(rank, color='g', label='TRUTH: %d' % rank, linestyle='-')
plt.axvline(n_components_pca, color='b',
label='PCA CV: %d' % n_components_pca, linestyle='--')
plt.axvline(n_components_fa, color='r',
label='FactorAnalysis CV: %d' % n_components_fa,
linestyle='--')
plt.axvline(n_components_pca_mle, color='k',
label='PCA MLE: %d' % n_components_pca_mle, linestyle='--')# 与其他协方差估计器进行比较
plt.axhline(shrunk_cov_score(X), color='violet',
label='Shrunk Covariance MLE', linestyle='-.')
plt.axhline(lw_score(X), color='orange',
label='LedoitWolf MLE' % n_components_pca_mle, linestyle='-.')
plt.xlabel('nb of components')
plt.ylabel('CV scores')
plt.legend(loc='lower right')
plt.title(title)
plt.show()
脚本的总运行时间:( 0 分 8.397 秒)
估计的内存使用量:8 MB
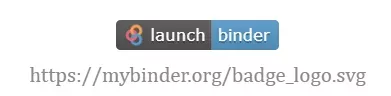
由Sphinx-Gallery生成的画廊
下载python源代码:plot_faces_decomposition.py
下载Jupyter notebook源代码:plot_faces_decomposition.ipynb

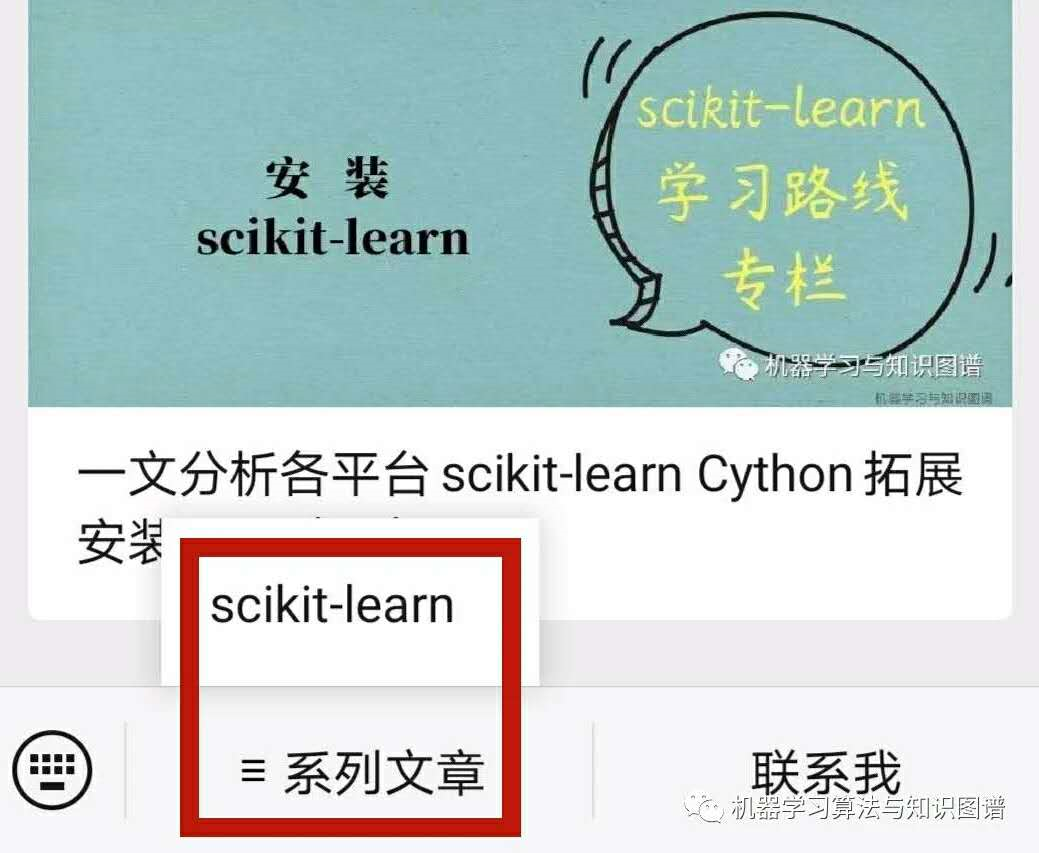
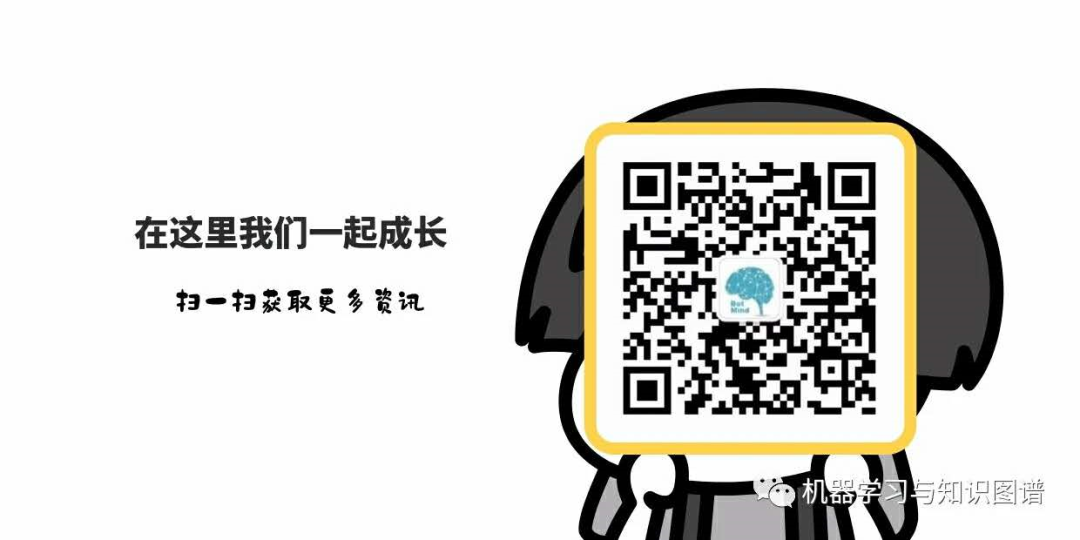