卷积
- 在torch.nn.function当中,能够看到跟视频一样的结果,所以,要对torch官网很熟悉,经常去查:
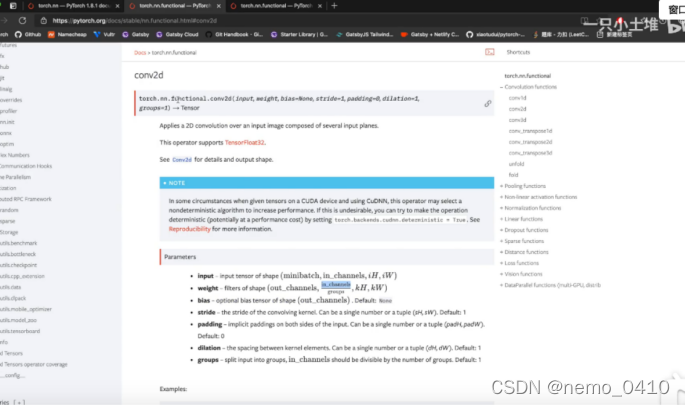
- 代码中说的,kernel和input的四个参数,含义不一样了,指的是这个:
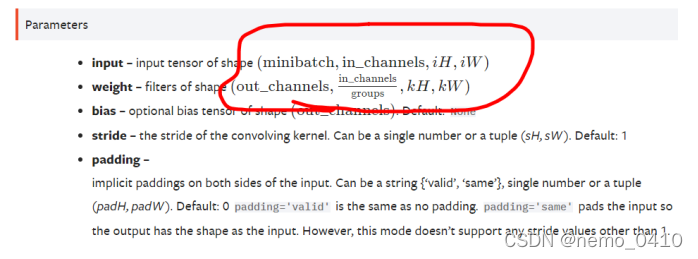
可以运行的代码
"""
author :24nemo
date :2021年07月07日
"""
'''
torch.nn 和 torch.nn.functional 是包含关系,functional 指的是具体的函数源代码,而 torch.nn 是封装好的,可以直接使用
他们的区别,从conv的大小写(Conv和conv),也能看出来。而且,torch.nn.functional中,带着 functional,torch.nn 是不用加的
本节的内容,都是结合torch.nn.functional 实现的,具体的查看 :
https://pytorch.org/docs/stable/generated/torch.nn.functional.conv2d.html#torch.nn.functional.conv2d
具体的函数,以及参数,都有讲到
torch.nn.functional.conv2d(input, weight, bias=None, stride=1, padding=0, dilation=1, groups=1) → Tensor
'''
import torch
import torch.nn.functional as F
input = torch.tensor([[1, 2, 0, 3, 1],
[0, 1, 2, 3, 1],
[1, 2, 1, 0, 0],
[5, 2, 3, 1, 1],
[2, 1, 0, 1, 1]])
kernel = torch.tensor([[1, 2, 1],
[0, 1, 0],
[2, 1, 0]])
print("input:", input)
print("kernel:", kernel)
print("input.shape:", input.shape)
print("kernel.shape:", kernel.shape)
input = torch.reshape(input, (1, 1, 5, 5))
kernel = torch.reshape(kernel, (1, 1, 3, 3))
print("input.shape:", input.shape)
print("kernel.shape:", kernel.shape)
output = F.conv2d(input, kernel, stride=1)
print(output)
output2 = F.conv2d(input, kernel, stride=2)
print(output2)
output3 = F.conv2d(input, kernel, stride=1, padding=1)
print(output3)
output4 = F.conv2d(input, kernel, stride=1, padding=0)
print(output4)
完整目录