import numpy as np # linear algebra
import pandas as pd # data processing, CSV file I/O (e.g. pd.read_csv)
%matplotlib inline
import matplotlib.pyplot as plt # Matlab-style plotting
import seaborn as sns
color = sns.color_palette()
sns.set_style('darkgrid')
import warnings
warnings.filterwarnings("ignore")
from scipy import stats
from scipy.stats import norm, skew #for some statistics
train = pd.read_csv('../input/house-prices-advanced-regression-techniques/train.csv')
test = pd.read_csv('../input/house-prices-advanced-regression-techniques/test.csv')
##display the first five rows of the train/test dataset.
train.head(5)
test.head(5)
#check the numbers of samples and features
print("The train data size before dropping Id feature is : {} ".format(train.shape))
print("The test data size before dropping Id feature is : {} ".format(test.shape))
# 【ID列处理参考模版】
#Save the 'Id' column
train_ID = train['Id']
test_ID = test['Id']
#Now drop the 'Id' colum since it's unnecessary for the prediction process.
train.drop("Id", axis = 1, inplace = True)
test.drop("Id", axis = 1, inplace = True)
#check again the data size after dropping the 'Id' variable
print("\nThe train data size after dropping Id feature is : {} ".format(train.shape))
print("The test data size after dropping Id feature is : {} ".format(test.shape))
# 【ID列处理参考模版】
# data processing
fig, ax = plt.subplots()
ax.scatter(x = train['GrLivArea'], y = train['SalePrice']) # GrLivArea: 地上居住面积平方英尺
plt.ylabel('SalePrice', fontsize=13)
plt.xlabel('GrLivArea', fontsize=13)
plt.show()
# We can see at the bottom right two with extremely large GrLivArea that are of a low price. These values are huge oultliers. Therefore, we can safely delete them.
#Deleting outliers
train = train.drop(train[(train['GrLivArea']>4000) & (train['SalePrice']<300000)].index)
#Check the graphic again
fig, ax = plt.subplots()
ax.scatter(train['GrLivArea'], train['SalePrice'])
plt.ylabel('SalePrice', fontsize=13)
plt.xlabel('GrLivArea', fontsize=13)
plt.show()
# 【回归问题目标值偏态可视化及处理参考模版】
# Target Variable
# SalePrice is the variable we need to predict. So let's do some analysis on this variable first.
sns.distplot(train['SalePrice'] , fit=norm);
# Get the fitted parameters used by the function
(mu, sigma) = norm.fit(train['SalePrice'])
print( '\n mu = {:.2f} and sigma = {:.2f}\n'.format(mu, sigma))
#Now plot the distribution
plt.legend(['Normal dist. ($\mu=$ {:.2f} and $\sigma=$ {:.2f} )'.format(mu, sigma)],
loc='best')
plt.ylabel('Frequency')
plt.title('SalePrice distribution')
#Get also the QQ-plot
fig = plt.figure()
res = stats.probplot(train['SalePrice'], plot=plt)
plt.show()
# The target variable is right skewed. As (linear) models love normally distributed data , we need to transform this variable and make it more normally distributed.
# Log-transformation of the target variable
#We use the numpy fuction log1p which applies log(1+x) to all elements of the column
train["SalePrice"] = np.log1p(train["SalePrice"])
#Check the new distribution
sns.distplot(train['SalePrice'] , fit=norm);
# Get the fitted parameters used by the function
(mu, sigma) = norm.fit(train['SalePrice'])
print( '\n mu = {:.2f} and sigma = {:.2f}\n'.format(mu, sigma))
#Now plot the distribution
plt.legend(['Normal dist. ($\mu=$ {:.2f} and $\sigma=$ {:.2f} )'.format(mu, sigma)],
loc='best')
plt.ylabel('Frequency')
plt.title('SalePrice distribution')
#Get also the QQ-plot
fig = plt.figure()
res = stats.probplot(train['SalePrice'], plot=plt)
plt.show()
# 【回归问题目标值偏态可视化及处理参考模版】
# Features engineering
ntrain = train.shape[0]
ntest = test.shape[0]
y_train = train.SalePrice.values
all_data = pd.concat((train, test)).reset_index(drop=True)
all_data.drop(['SalePrice'], axis=1
【Kaggle-House Price Prediction】代码参考
最新推荐文章于 2024-03-29 09:39:49 发布
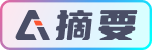