目录
10 多层感知机 + 代码实现
1.感知机
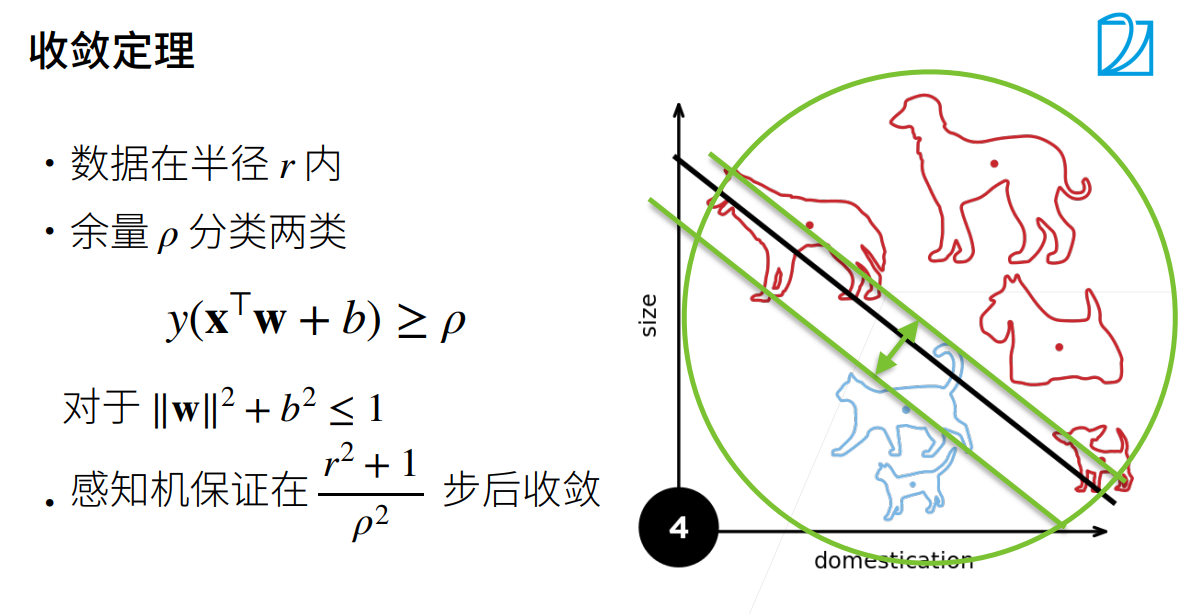
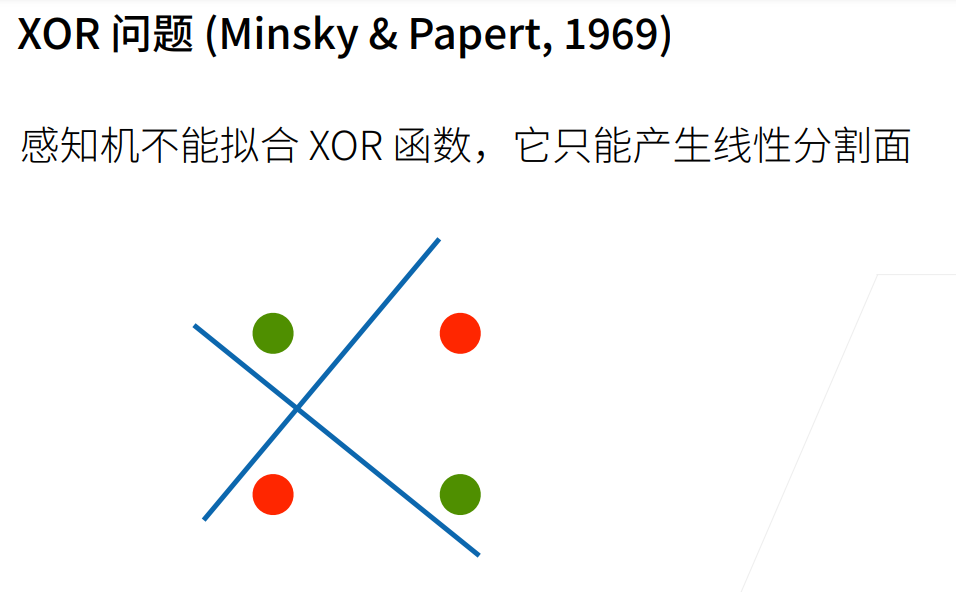
2.多层感知机
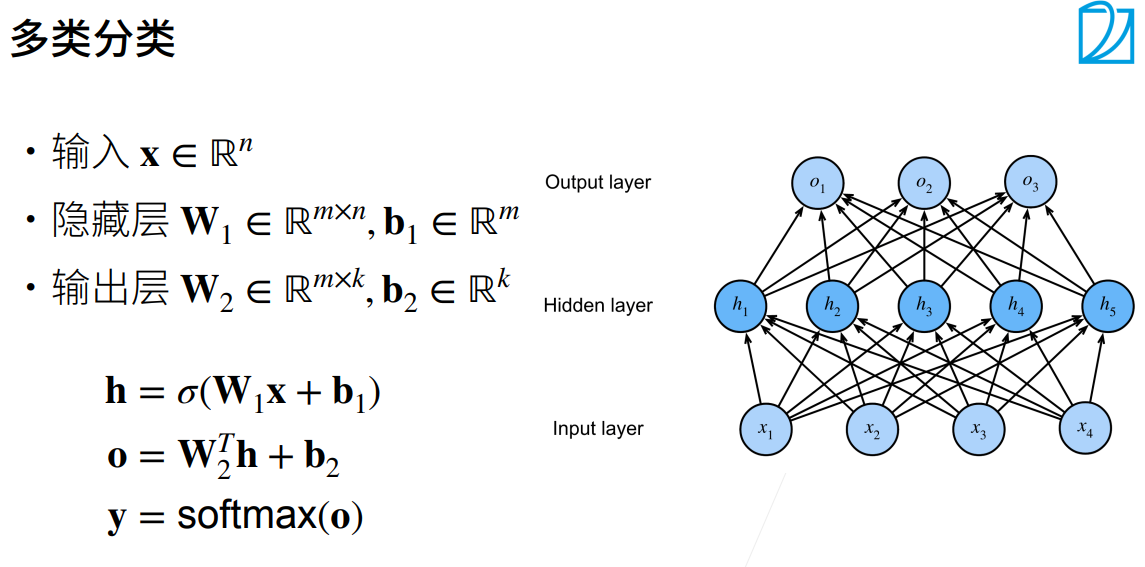
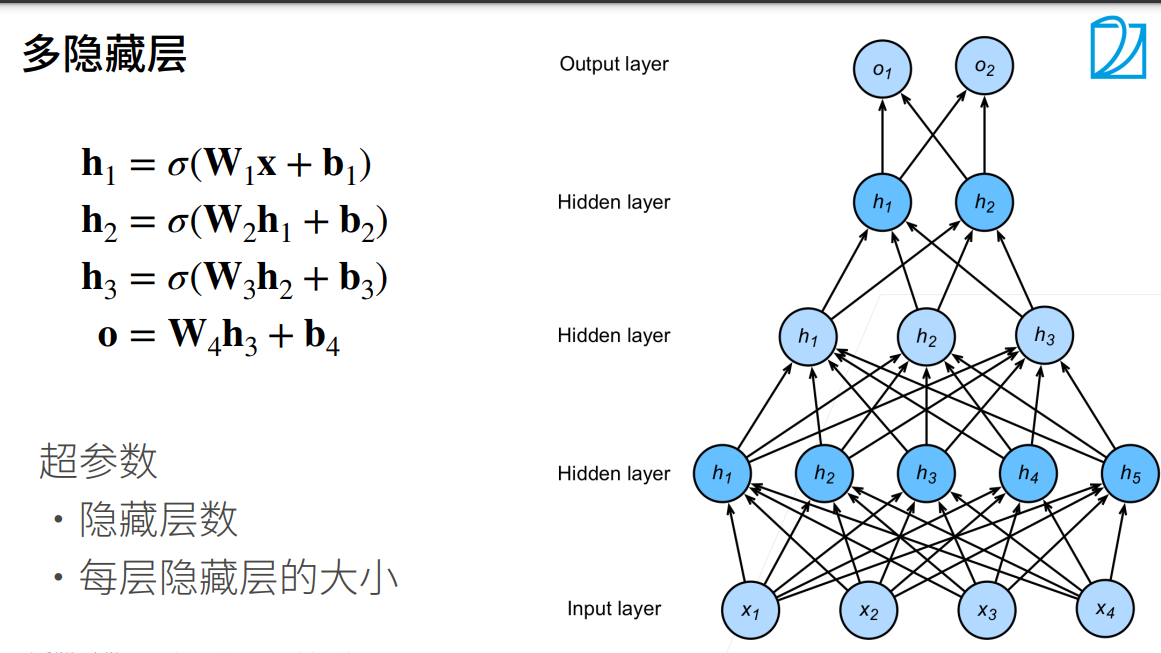
3.多层感知机的从零开始实现
# 多层感知机的从零开始实现
import torch
from torch import nn
from d2l import torch as d2l
import os
import matplotlib.pyplot as plt
os.environ["KMP_DUPLICATE_LIB_OK"] = "TRUE"
batch_size = 256
train_iter, test_iter = d2l.load_data_fashion_mnist(batch_size)
# 实现一个具有单隐藏层的多层感知机,它包含256个隐藏单元
num_inputs, num_outputs, num_hiddens = 784, 10, 256
W1 = nn.Parameter(torch.randn(num_inputs, num_hiddens, requires_grad=True) * 0.01)
b1 = nn.Parameter(torch.zeros(num_hiddens, requires_grad=True))
W2 = nn.Parameter(torch.randn(num_hiddens, num_outputs, requires_grad=True) * 0.01)
b2 = nn.Parameter(torch.zeros(num_outputs, requires_grad=True))
params = [W1, b1, W2, b2]
# 实现ReLU激活函数
def relu(X):
a = torch.zeros_like(X)
return torch.max(X, a)
# 实现我们的模型
def net(X):
X = X.reshape((-1, num_inputs))
H = relu(X @ W1 + b1)
return H @ W2 + b2
loss = nn.CrossEntropyLoss()
# 多层感知机的训练过程与softmax回归的训练过程完全相同
num_epochs, lr = 10, 0.1
updater = torch.optim.SGD(params, lr=lr)
d2l.train_ch3(net, train_iter, test_iter, loss, num_epochs, updater)
plt.show()
<Figure size 350x250 with 1 Axes>
<Figure size 350x250 with 1 Axes>
<Figure size 350x250 with 1 Axes>
<Figure size 350x250 with 1 Axes>
<Figure size 350x250 with 1 Axes>
<Figure size 350x250 with 1 Axes>
<Figure size 350x250 with 1 Axes>
<Figure size 350x250 with 1 Axes>
<Figure size 350x250 with 1 Axes>
<Figure size 350x250 with 1 Axes>
4.多层感知机的简洁实现
# 我们可以通过高级API更简洁地实现多层感知机
import torch
from torch import nn
from d2l import torch as d2l
import os
import matplotlib.pyplot as plt
os.environ["KMP_DUPLICATE_LIB_OK"] = "TRUE"
# 与softmax回归的简洁实现相比,唯一的区别是我们添加了2个全连接层(之前我们只添加了1个全连接层)。第一层是隐藏层,它包含256个隐藏单元,并使用了ReLU激活函数。第二层是输出层。
net = nn.Sequential(nn.Flatten(),
nn.Linear(784, 256),
nn.ReLU(),
nn.Linear(256, 10))
def init_weights(m):
if type(m) == nn.Linear:
nn.init.normal_(m.weight, std=0.01)
net.apply(init_weights)
# 训练过程的实现与我们实现softmax回归时完全相同,这种模块化设计使我们能够将和模型架构有关的内容独立出来。
batch_size, lr, num_epochs = 256, 0.1, 10
loss = nn.CrossEntropyLoss()
trainer = torch.optim.SGD(net.parameters(), lr=lr)
train_iter, test_iter = d2l.load_data_fashion_mnist(batch_size)
d2l.train_ch3(net, train_iter, test_iter, loss, num_epochs, trainer)
plt.show()
<Figure size 350x250 with 1 Axes>
<Figure size 350x250 with 1 Axes>
<Figure size 350x250 with 1 Axes>
<Figure size 350x250 with 1 Axes>
<Figure size 350x250 with 1 Axes>
<Figure size 350x250 with 1 Axes>
<Figure size 350x250 with 1 Axes>
<Figure size 350x250 with 1 Axes>
<Figure size 350x250 with 1 Axes>
<Figure size 350x250 with 1 Axes>