18~20 参考https://blog.csdn.net/weixin_46773169/article/details/108833993
目录
NTIRE 2021 Learning the Super-Resolution Space Challenge
一、任务
二、评价标准
三、结果
NTIRE 2021 Challenge on Burst Super-Resolution: Methods and Results
一、任务
二、评价标准
三、结果
NTIRE 2021 Challenge on Video Super-Resolution
一、任务
二、评价标准
三、结果
NTIRE 2020 Challenge on Real-World Image Super-Resolution
一、任务
二、评价标准
三、结果
NTIRE 2020 Challenge on Perceptual Extreme Super-Resolution
一、任务
二、评价标准
三、结果
NTIRE 2021 Learning the Super-Resolution Space Challenge
一、任务
在大尺寸的超分辨率中存在多个可能解,要求模型可以提供在4x和8x上同一个LR对应的任意多个HR结果。
二、评价标准
数据集为DIV2K bicubic downsample
1、Photo-realism: LPIPS metric,Mean Opinion Rank (MOR) for the user study
2、The spanning of the SR Space
3、Low Resolution Consistency
三、结果
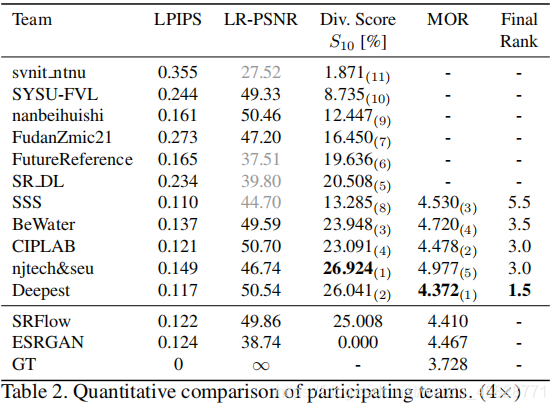
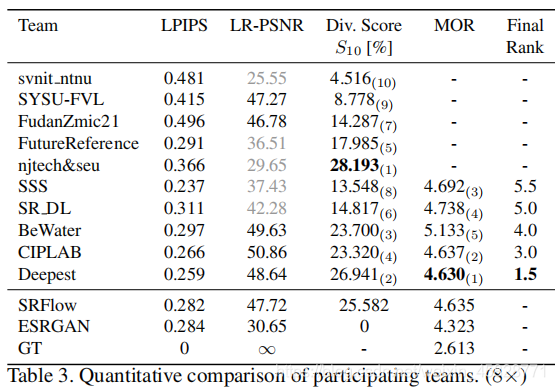
NTIRE 2021 Challenge on Burst Super-Resolution: Methods and Results
一、任务
Given a RAW noisy burst as input, the
task in the challenge was to generate a clean RGB image
with 4 times higher resolution.
连拍的raw→4x的RGB
The challenge contained two
tracks; Track 1 evaluating on synthetically generated data,
and Track 2 using real-world bursts from mobile camera.
Track1是根据GT人为退化生成的数据 Track 2 是不同的设备拍摄对应的数据
二、评价标准
Track 1:PSNR SSIM LPIPS
数据集为Goutam Bhat, Martin Danelljan, L. Gool, and R. Timofte. Deep burst super-resolution. In CVPR, 2021
论文中所给出的数据集(训练集、测试集)
Track 2:Deep burst super-resolution中的处理方法进行处理,standard image quality metrics(PSNR, SSIM, and LPIPS)和MOR
数据集:BurstSR dataset consists of bursts captured using a hand held camera, along with a corresponding HR image captured using a DSLR.
三、结果
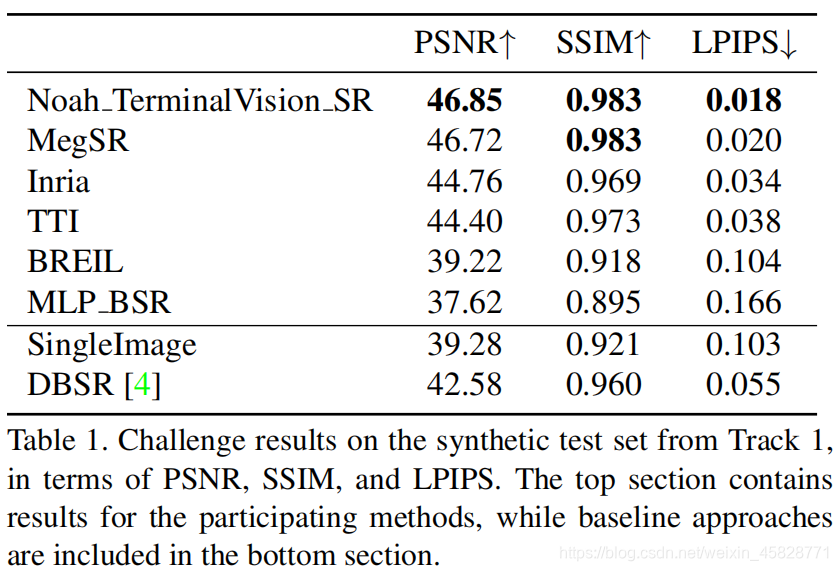
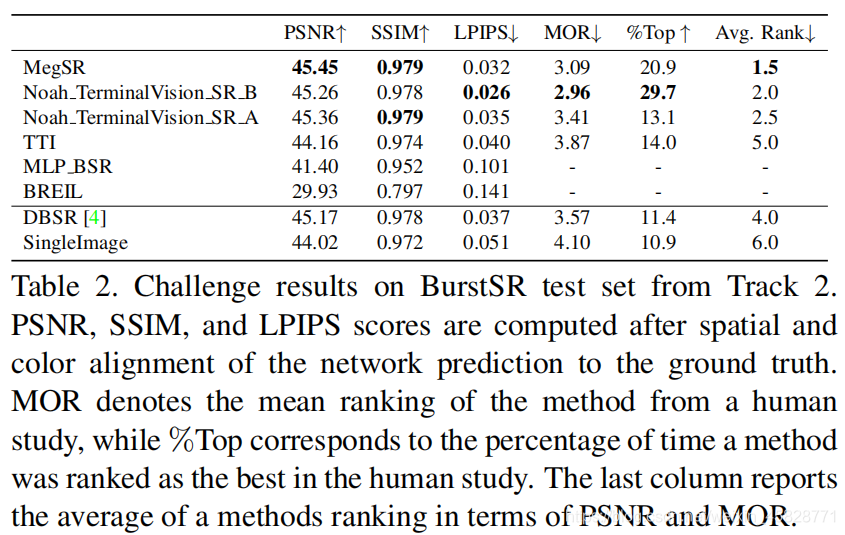
NTIRE 2021 Challenge on Video Super-Resolution
一、任务
Track 1: 传统
reconstruct 30 HR sequences from given
×
4 downsampled videos from the REDS [
37
] dataset, similar to the conventional VSR algorithms.
Track 2:
spatio-temporal super-resolution (STSR) 12fps视频,一边插帧一边超分
二、评价标准
数据集REDS
Track 1:PSNR and SSIM LPIPS
Track 2:PSNR and SSIM LPIPS
三、结果
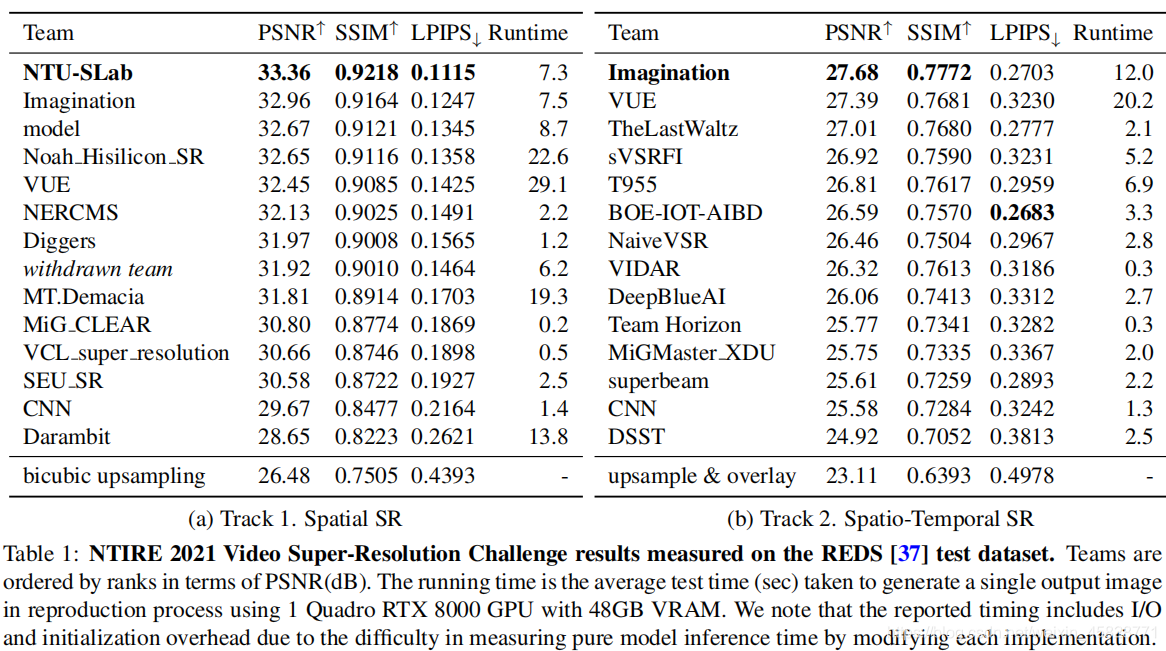
冠军方案解读:https://blog.csdn.net/weixin_47196664/article/details/115922761
NTIRE 2020 Challenge on Real-World Image Super-Resolution
一、任务
真实世界图像的超分
only one set of source
input images is therefore provided along with a set of un
paired high-quality target images
Track 1: Image Processing artifacts
, the aim is to super-resolve images with
synthetically generated image processing artifacts
Track 2: Smartphone Images
, real
low-quality smart phone images have to be super-resolved.
In both tracks, the ultimate goal is to achieve the best per
ceptual quality, evaluated using a human study.
由于真实情景下,LR-HR对 难以获得,所以 In this challenge, the aim is instead to learn super-resolution from unpaired data and without any restricting assumptions on the input image formation.
二、评价标准
No paired reference HR images are available for training.只提供HR
Track1 : the input is generated with a common image signal processing operation to simulate the real-world SR case where we can measure against a undisclosed ground truth 输入人为模拟的退化图像
PSNR SSIM
训练集来自 Unsupervised learning for real-world super-resolution ICCVW2019
Track 2 we the input are untouched iPhone3 images. 数据集是DPED
MOR
三、结果
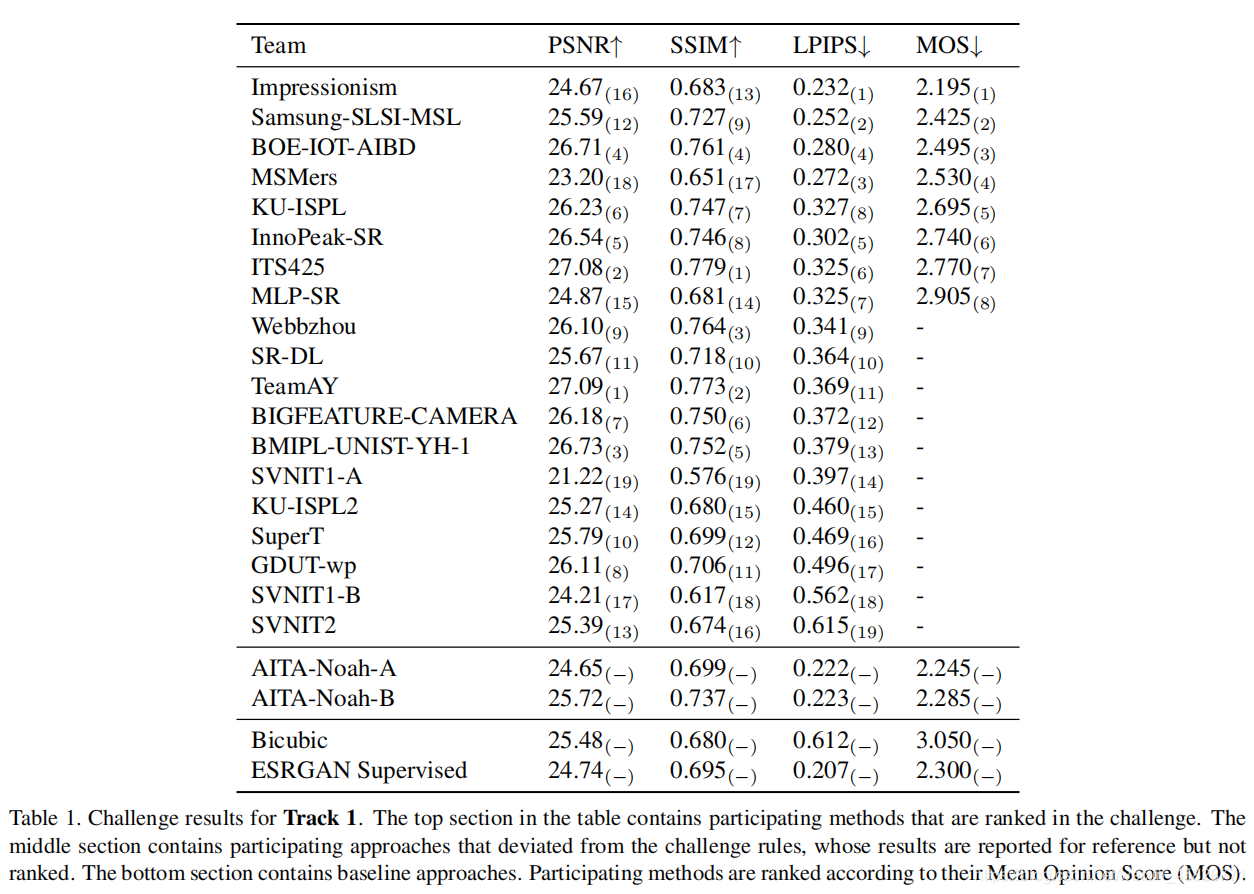
Top解读:https://mp.weixin.qq.com/s/LwMLjjUr4wnzRqsgbanrrQ
NTIRE 2020 Challenge on Perceptual Extreme Super-Resolution
一、任务
x16 且能够生成具有最佳感知质量并与真实情况相似的高分辨率结果
Extreme:倍数很大 x16
Perceptual: 感知质量要求高
二、评价标准
DIV8K数据集
LPIPS (Learned Perceptual Image Patch Similarity) and no-reference PI(Perceptual Index)
三、结果
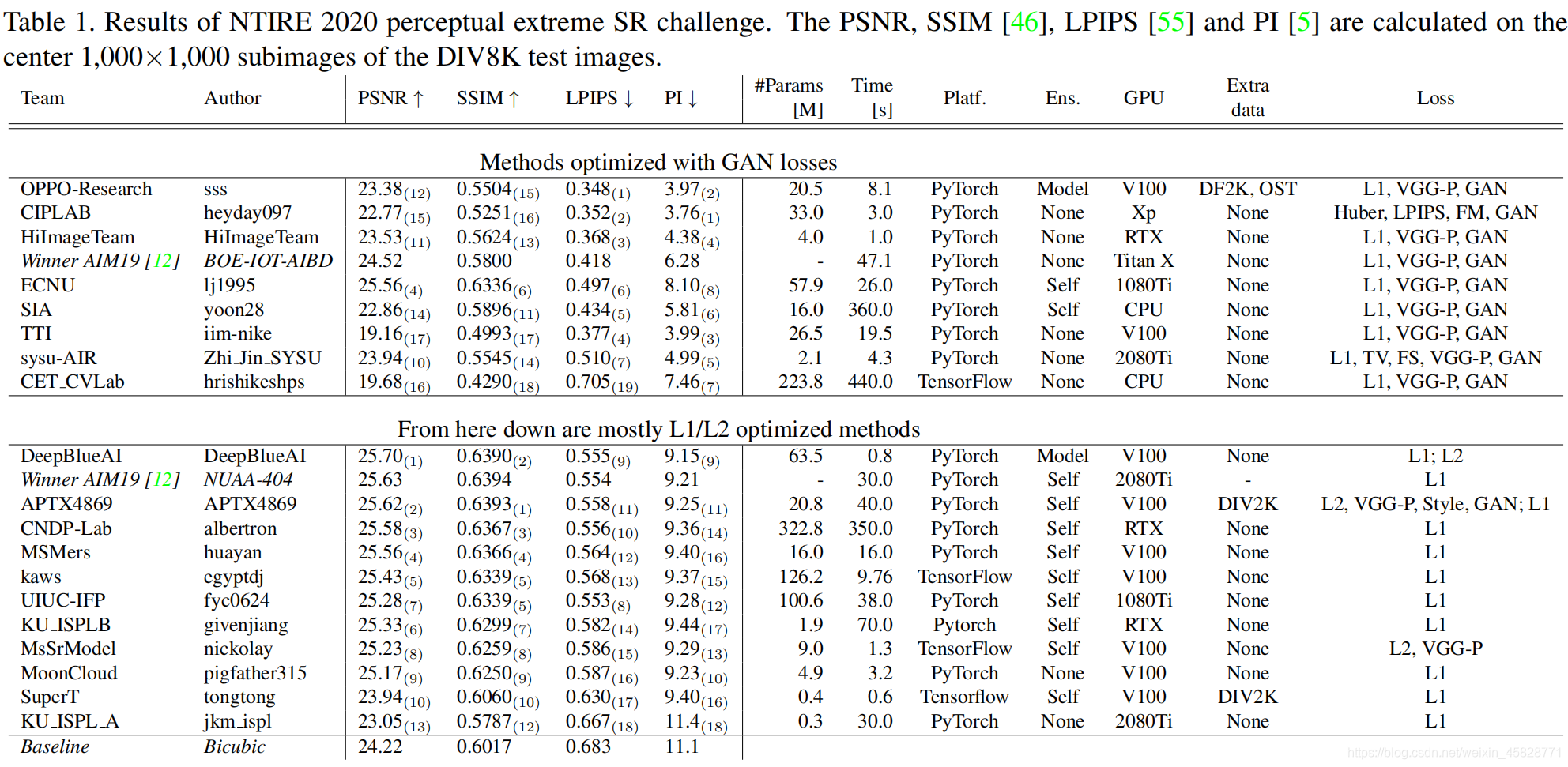
Top解读:https://mp.weixin.qq.com/s/nj_C_LXFpWQZASc4ITJmmA