从磁盘恢复字符级序列到序列模型,并用它生成预测。
代码注释
'''Restore a character-level sequence to sequence model from disk and use it
to generate predictions.
从磁盘恢复字符级序列到序列模型,并用它生成预测。
This script loads the s2s.h5 model saved by lstm_seq2seq.py and generates
sequences from it. It assumes that no changes have been made (for example:
latent_dim is unchanged, and the input data and model architecture are unchanged).
脚本加载lstm_seq2seq.py 保存的s2s.h5模型并从中生成序列。假定没有进行任何更改(例如:latent_dim是
不变的,并且输入数据和模型架构不变)。
See lstm_seq2seq.py for more details on the model architecture and how
it is trained.
有关模型架构的更多细节和如何训练(模型),请参考lstm_seq2seq.py
'''
from __future__ import print_function
from keras.models import Model, load_model
from keras.layers import Input
import numpy as np
batch_size = 64 # Batch size for training. 训练批次大小(每个批次包含样本数)
epochs = 100 # Number of epochs to train for.训练周期数
latent_dim = 256 # Latent dimensionality of the encoding space.编码空间的潜在维数
num_samples = 10000 # Number of samples to train on.训练集样本数
# Path to the data txt file on disk.
# 存储器数据文件路径(先下载,然后存放到和本脚本同级目录)
data_path = 'fra-eng/fra.txt'
# Vectorize the data. We use the same approach as the training script.
# 数据向量化。使用与训练脚本相同的方法。
# NOTE: the data must be identical, in order for the character -> integer
# mappings to be consistent.
# 注意:为了使字符->整数映射一致,数据必须是相同的
# We omit encoding target_texts since they are not needed.
# 省略了target_texts的编码,因为不需要。
input_texts = []
target_texts = []
input_characters = set()
target_characters = set()
with open(data_path, 'r', encoding='utf-8') as f:
lines = f.read().split('\n')
for line in lines[: min(num_samples, len(lines) - 1)]:
input_text, target_text = line.split('\t')
# We use "tab" as the "start sequence" character
# 使用"tab"为开始序列字符
# for the targets, and "\n" as "end sequence" character.
# 使用“tab”作为目标的“开始序列”字符,而“\n”作为“结束序列”字符。
target_text = '\t' + target_text + '\n'
input_texts.append(input_text)
target_texts.append(target_text)
for char in input_text:
if char not in input_characters:
input_characters.add(char)
for char in target_text:
if char not in target_characters:
target_characters.add(char)
input_characters = sorted(list(input_characters))
target_characters = sorted(list(target_characters))
num_encoder_tokens = len(input_characters)
num_decoder_tokens = len(target_characters)
max_encoder_seq_length = max([len(txt) for txt in input_texts])
max_decoder_seq_length = max([len(txt) for txt in target_texts])
print('Number of samples:', len(input_texts))
print('Number of unique input tokens:', num_encoder_tokens)
print('Number of unique output tokens:', num_decoder_tokens)
print('Max sequence length for inputs:', max_encoder_seq_length)
print('Max sequence length for outputs:', max_decoder_seq_length)
input_token_index = dict(
[(char, i) for i, char in enumerate(input_characters)])
target_token_index = dict(
[(char, i) for i, char in enumerate(target_characters)])
encoder_input_data = np.zeros(
(len(input_texts), max_encoder_seq_length, num_encoder_tokens),
dtype='float32')
for i, input_text in enumerate(input_texts):
for t, char in enumerate(input_text):
encoder_input_data[i, t, input_token_index[char]] = 1.
# Restore the model and construct the encoder and decoder.
# 恢复模型并构造编码器和解码器。
model = load_model('s2s.h5')
encoder_inputs = model.input[0] # input_1
encoder_outputs, state_h_enc, state_c_enc = model.layers[2].output # lstm_1
encoder_states = [state_h_enc, state_c_enc]
encoder_model = Model(encoder_inputs, encoder_states)
decoder_inputs = model.input[1] # input_2
decoder_state_input_h = Input(shape=(latent_dim,), name='input_3')
decoder_state_input_c = Input(shape=(latent_dim,), name='input_4')
decoder_states_inputs = [decoder_state_input_h, decoder_state_input_c]
decoder_lstm = model.layers[3]
decoder_outputs, state_h_dec, state_c_dec = decoder_lstm(
decoder_inputs, initial_state=decoder_states_inputs)
decoder_states = [state_h_dec, state_c_dec]
decoder_dense = model.layers[4]
decoder_outputs = decoder_dense(decoder_outputs)
decoder_model = Model(
[decoder_inputs] + decoder_states_inputs,
[decoder_outputs] + decoder_states)
# Reverse-lookup token index to decode sequences back to
# something readable.
# 反向查找分词索引(词的编号)将序列解码为可读的。
reverse_input_char_index = dict(
(i, char) for char, i in input_token_index.items())
reverse_target_char_index = dict(
(i, char) for char, i in target_token_index.items())
# Decodes an input sequence. Future work should support beam search.
# 解码输入序列。未来的工作应该支持波束搜索。
def decode_sequence(input_seq):
# Encode the input as state vectors.
# 将输入(数据)编码为状态向量。
states_value = encoder_model.predict(input_seq)
# Generate empty target sequence of length 1.
# 生成长度为1的空目标序列。
target_seq = np.zeros((1, 1, num_decoder_tokens))
# Populate the first character of target sequence with the start character.
# 用开始字符填充目标序列的第一个字符。
target_seq[0, 0, target_token_index['\t']] = 1.
# Sampling loop for a batch of sequences
# (to simplify, here we assume a batch of size 1).
# 一批序列的采样循环(为了简化,这里假设一批次大小为1)。
stop_condition = False
decoded_sentence = ''
while not stop_condition:
output_tokens, h, c = decoder_model.predict(
[target_seq] + states_value)
# Sample a token
# 采样
sampled_token_index = np.argmax(output_tokens[0, -1, :])
sampled_char = reverse_target_char_index[sampled_token_index]
decoded_sentence += sampled_char
# Exit condition: either hit max length
# or find stop character.
# 退出条件:到达最大长度或找到停止字符。
if (sampled_char == '\n' or
len(decoded_sentence) > max_decoder_seq_length):
stop_condition = True
# Update the target sequence (of length 1).
# 更新目标序列(长度1)。
target_seq = np.zeros((1, 1, num_decoder_tokens))
target_seq[0, 0, sampled_token_index] = 1.
# Update states
# 更新状态
states_value = [h, c]
return decoded_sentence
for seq_index in range(100):
# Take one sequence (part of the training set)
# for trying out decoding.
# 用一个序列(训练集的一部分)进行解码。
input_seq = encoder_input_data[seq_index: seq_index + 1]
decoded_sentence = decode_sequence(input_seq)
print('-')
print('Input sentence:', input_texts[seq_index])
print('Decoded sentence:', decoded_sentence)
代码执行
C:\ProgramData\Anaconda3\python.exe E:/keras-master/examples/lstm_seq2seq_restore.py
Using TensorFlow backend.
Number of samples: 10000
Number of unique input tokens: 71
Number of unique output tokens: 94
Max sequence length for inputs: 16
Max sequence length for outputs: 59
-
Input sentence: Go.
Decoded sentence: Va !
-
Input sentence: Run!
Decoded sentence: Cours !
-
Input sentence: Run!
Decoded sentence: Cours !
-
Input sentence: Fire!
Decoded sentence: Au feu !
-
Input sentence: Help!
Decoded sentence: À l'aide !
-
Input sentence: Jump.
Decoded sentence: Saute.
-
Input sentence: Stop!
Decoded sentence: Arrête-toi !
-
Input sentence: Stop!
Decoded sentence: Arrête-toi !
-
Input sentence: Stop!
Decoded sentence: Arrête-toi !
-
Input sentence: Wait!
Decoded sentence: Attends !
-
Input sentence: Wait!
Decoded sentence: Attends !
-
Input sentence: Go on.
Decoded sentence: Continuez.
-
Input sentence: Go on.
Decoded sentence: Continuez.
-
Input sentence: Go on.
Decoded sentence: Continuez.
-
Input sentence: I see.
Decoded sentence: Je vois une lumière.
-
Input sentence: I try.
Decoded sentence: J'essaye.
-
Input sentence: I won!
Decoded sentence: J'ai demandé à dore.
-
Input sentence: I won!
Decoded sentence: J'ai demandé à dore.
-
Input sentence: Oh no!
Decoded sentence: Oh non !
-
Input sentence: Attack!
Decoded sentence: Attaquez !
-
Input sentence: Attack!
Decoded sentence: Attaquez !
-
Input sentence: Cheers!
Decoded sentence: À votre santé !
-
Input sentence: Cheers!
Decoded sentence: À votre santé !
-
Input sentence: Cheers!
Decoded sentence: À votre santé !
-
Input sentence: Cheers!
Decoded sentence: À votre santé !
-
Input sentence: Get up.
Decoded sentence: Lève-toi.
-
Input sentence: Go now.
Decoded sentence: Va doucement !
-
Input sentence: Go now.
Decoded sentence: Va doucement !
-
Input sentence: Go now.
Decoded sentence: Va doucement !
-
Input sentence: Got it!
Decoded sentence: Compris !
-
Input sentence: Got it!
Decoded sentence: Compris !
-
Input sentence: Got it?
Decoded sentence: Compris ?
-
Input sentence: Got it?
Decoded sentence: Compris ?
-
Input sentence: Got it?
Decoded sentence: Compris ?
-
Input sentence: Hop in.
Decoded sentence: Montez.
-
Input sentence: Hop in.
Decoded sentence: Montez.
-
Input sentence: Hug me.
Decoded sentence: Serre-moi dans tes bras !
-
Input sentence: Hug me.
Decoded sentence: Serre-moi dans tes bras !
-
Input sentence: I fell.
Decoded sentence: Je suis tombée.
-
Input sentence: I fell.
Decoded sentence: Je suis tombée.
-
Input sentence: I know.
Decoded sentence: Je sais.
-
Input sentence: I left.
Decoded sentence: Je suis parti.
-
Input sentence: I left.
Decoded sentence: Je suis parti.
-
Input sentence: I lost.
Decoded sentence: J'ai perdu.
-
Input sentence: I'm 19.
Decoded sentence: J'ai les chocontes.
-
Input sentence: I'm OK.
Decoded sentence: Je vais bien.
-
Input sentence: I'm OK.
Decoded sentence: Je vais bien.
-
Input sentence: Listen.
Decoded sentence: Écoutez !
-
Input sentence: No way!
Decoded sentence: C'est exclu !
-
Input sentence: No way!
Decoded sentence: C'est exclu !
-
Input sentence: No way!
Decoded sentence: C'est exclu !
-
Input sentence: No way!
Decoded sentence: C'est exclu !
-
Input sentence: No way!
Decoded sentence: C'est exclu !
-
Input sentence: No way!
Decoded sentence: C'est exclu !
-
Input sentence: No way!
Decoded sentence: C'est exclu !
-
Input sentence: No way!
Decoded sentence: C'est exclu !
-
Input sentence: No way!
Decoded sentence: C'est exclu !
-
Input sentence: Really?
Decoded sentence: Vrai ?
-
Input sentence: Really?
Decoded sentence: Vrai ?
-
Input sentence: Really?
Decoded sentence: Vrai ?
-
Input sentence: Thanks.
Decoded sentence: Merci !
-
Input sentence: We try.
Decoded sentence: On essaye.
-
Input sentence: We won.
Decoded sentence: Nous avons réveillé.
-
Input sentence: We won.
Decoded sentence: Nous avons réveillé.
-
Input sentence: We won.
Decoded sentence: Nous avons réveillé.
-
Input sentence: We won.
Decoded sentence: Nous avons réveillé.
-
Input sentence: Ask Tom.
Decoded sentence: Demande-leur.
-
Input sentence: Awesome!
Decoded sentence: Faisalez-moi !
-
Input sentence: Be calm.
Decoded sentence: Sois calme !
-
Input sentence: Be calm.
Decoded sentence: Sois calme !
-
Input sentence: Be calm.
Decoded sentence: Sois calme !
-
Input sentence: Be cool.
Decoded sentence: Sois détendu !
-
Input sentence: Be fair.
Decoded sentence: Soyez équitables !
-
Input sentence: Be fair.
Decoded sentence: Soyez équitables !
-
Input sentence: Be fair.
Decoded sentence: Soyez équitables !
-
Input sentence: Be fair.
Decoded sentence: Soyez équitables !
-
Input sentence: Be fair.
Decoded sentence: Soyez équitables !
-
Input sentence: Be fair.
Decoded sentence: Soyez équitables !
-
Input sentence: Be kind.
Decoded sentence: Sois gentil.
-
Input sentence: Be nice.
Decoded sentence: Sois gentille !
-
Input sentence: Be nice.
Decoded sentence: Sois gentille !
-
Input sentence: Be nice.
Decoded sentence: Sois gentille !
-
Input sentence: Be nice.
Decoded sentence: Sois gentille !
-
Input sentence: Be nice.
Decoded sentence: Sois gentille !
-
Input sentence: Be nice.
Decoded sentence: Sois gentille !
-
Input sentence: Beat it.
Decoded sentence: Dégage !
-
Input sentence: Call me.
Decoded sentence: Appellez-moi !
-
Input sentence: Call me.
Decoded sentence: Appellez-moi !
-
Input sentence: Call us.
Decoded sentence: Appelle-nous !
-
Input sentence: Call us.
Decoded sentence: Appelle-nous !
-
Input sentence: Come in.
Decoded sentence: Entrez !
-
Input sentence: Come in.
Decoded sentence: Entrez !
-
Input sentence: Come in.
Decoded sentence: Entrez !
-
Input sentence: Come in.
Decoded sentence: Entrez !
-
Input sentence: Come on!
Decoded sentence: Allez !
-
Input sentence: Come on.
Decoded sentence: Viens !
-
Input sentence: Come on.
Decoded sentence: Viens !
-
Input sentence: Come on.
Decoded sentence: Viens !
-
Input sentence: Drop it!
Decoded sentence: Laissez-le tomber !
-
Input sentence: Drop it!
Decoded sentence: Laissez-le tomber !
Process finished with exit code 0
Keras详细介绍
中文:http://keras-cn.readthedocs.io/en/latest/
实例下载
https://github.com/keras-team/keras
https://github.com/keras-team/keras/tree/master/examples
完整项目下载
方便没积分童鞋,请加企鹅452205574,共享文件夹。
包括:代码、数据集合(图片)、已生成model、安装库文件等。
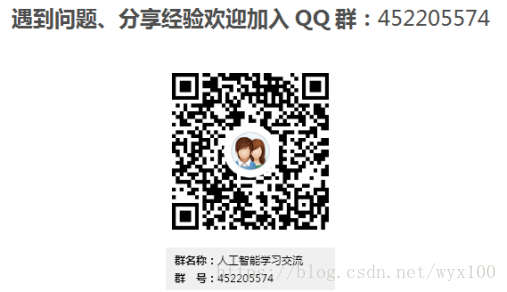