部署过程主要遇到的坑是签名的问题这里做下记录参考文档地址
https://www.tensorflow.org/tfx/serving/serving_basic
https://www.tensorflow.org/api_docs/python/tf/saved_model/signature_constants
这里的部署方式以windows docker为例子,linux系统也是一样的操作,路径相应改变下就行
github demo地址https://github.com/Caigengliang/exportKerasForTensorflowServer
拉取镜像
安装完docker后,可以用一下命令直接拉取最新的tensorflow server镜像
docker pull tensorflow/serving
导出keras模型保存为tensorflow-server指定格式
先创建一个方法,改方法用于创建指定目录的文件夹,存在则不动作,不存在则创建。如果改目录下存在文件应该删除它,以方便模型更新。
def mkdir(path):
import os
import shutil
path=path.strip()
path=path.rstrip("\\")
isExists=os.path.exists(path)
if not isExists:
os.makedirs(path)
return True
else:
filelist=os.listdir(path)
for f in filelist:
filepath = os.path.join( path, f ) #将文件名映射成绝对路劲
if os.path.isfile(filepath): #判断该文件是否为文件或者文件夹
os.remove(filepath) #若为文件,则直接删除
print(str(filepath)+" removed!")
elif os.path.isdir(filepath):
shutil.rmtree(filepath,True) #若为文件夹,则删除该文件夹及文件夹内所有文件
print("dir "+str(filepath)+" removed!")
模型导出
在prediction_signature定义了一下参数
inputs={'images': tensor_info_x}
指定输入张量信息。outputs={'scores': tensor_info_y}
指定分数张量信息。method_name
是用于推理的方法。对于预测请求,应将其设置为tensorflow/serving/predict
。有关其他方法名称,请参阅signature_constants.py 和相关的 TensorFlow 1.0 API文档。
其中method_name映射为api的路径方法名
import tensorflow as tf
import os
import tensorflow.keras.backend as K
from tensorflow.keras.losses import categorical_crossentropy
from tensorflow.keras.optimizers import Adadelta
def export_model(model,
export_model_dir,
model_version
):
"""
:param export_model_dir: type string, save dir for exported model url
:param model_version: type int best
:return:no return
"""
with tf.get_default_graph().as_default():
# prediction_signature
tensor_info_input = tf.saved_model.utils.build_tensor_info(model.input)
tensor_info_output = tf.saved_model.utils.build_tensor_info(model.output)
print(model.output.shape, '**', tensor_info_output)
prediction_signature = (
tf.saved_model.signature_def_utils.build_signature_def(
inputs={'images': tensor_info_input}, # Tensorflow.TensorInfo
outputs={'result': tensor_info_output},
#method_name=tf.saved_model.signature_constants.PREDICT_METHOD_NAME)
method_name= "tensorflow/serving/predict")
)
print('step1 => prediction_signature created successfully')
# set-up a builder
mkdir(export_model_dir)
export_path_base = export_model_dir
export_path = os.path.join(
tf.compat.as_bytes(export_path_base),
tf.compat.as_bytes(str(model_version)))
builder = tf.saved_model.builder.SavedModelBuilder(export_path)
builder.add_meta_graph_and_variables(
# tags:SERVING,TRAINING,EVAL,GPU,TPU
sess=K.get_session(),
tags=[tf.saved_model.tag_constants.SERVING],
signature_def_map={
'predict':
prediction_signature,
tf.saved_model.signature_constants.DEFAULT_SERVING_SIGNATURE_DEF_KEY:
prediction_signature,
},
)
print('step2 => Export path(%s) ready to export trained model' % export_path, '\n starting to export model...')
#builder.save(as_text=True)
builder.save()
print('Done exporting!')
调用导出方法导出,这里假设已经把模型保存为.h5文件了
model = tf.keras.models.load_model('./12308nenghao.h5')
export_model(
model,
'C:/tmp/tfserving/pow',
1
)
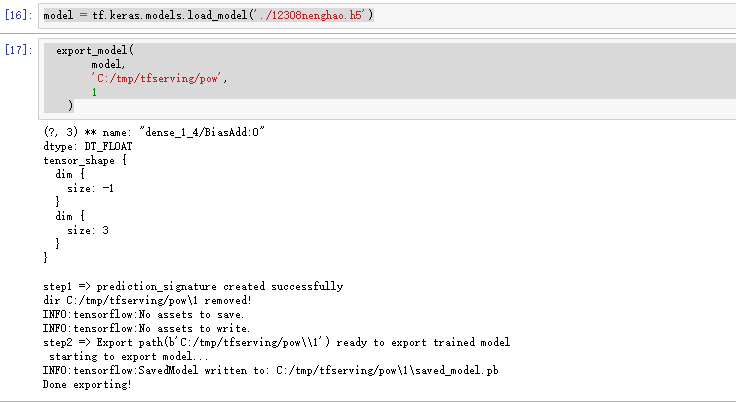
可以看到对应目录下生成了如下文件:
其中1这个父目录是版本好,tensorflow-server会识别这个版本号
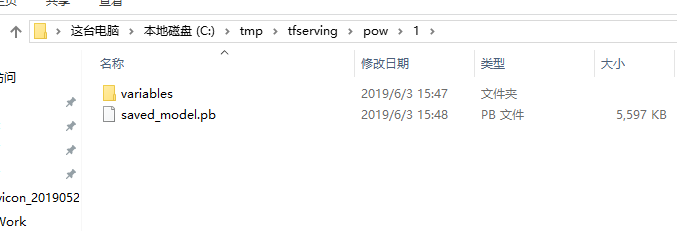
在docker中运行
指定好路径
docker run -p 8501:8501 --mount type=bind,source=C:/tmp/tfserving/pow,target=/models/pow -e MODEL_NAME=pow -t tensorflow/serving '&'
server生成两个接口一个restful一个gprc接口

验证
直接用postman测试restful
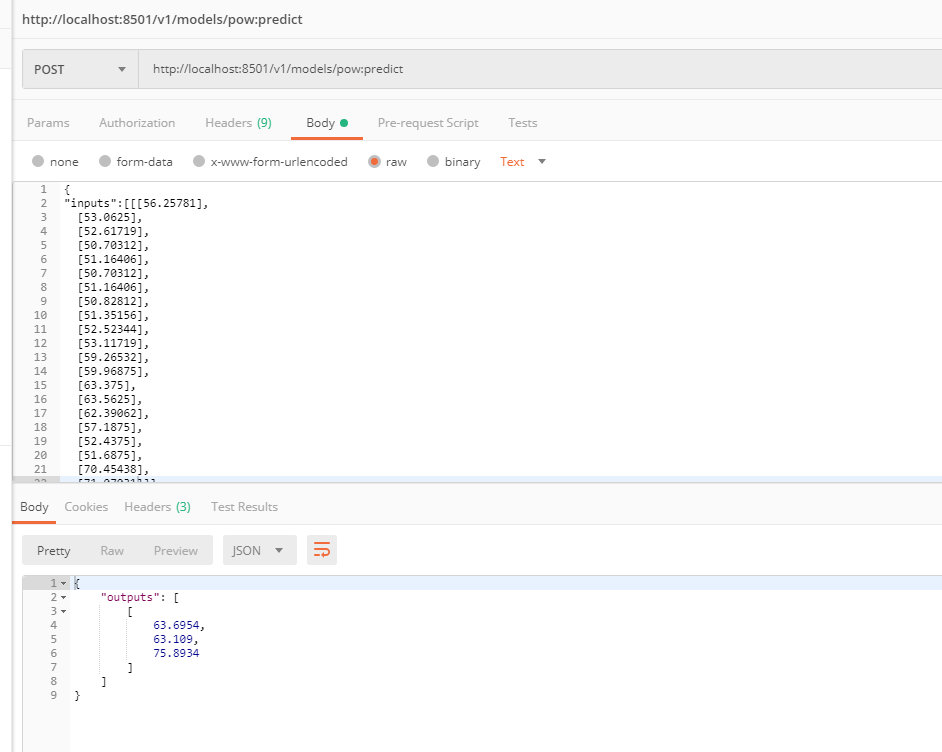
遇到问题
在测试的时候要特别注意输入的张量的形状,在导出模型的时候查看下。
{ "error": "Serving signature name: "serving_default" not found in signature def" }
该问题是由签名没有设置默认serving_default
检查下signature_def_map是否缺少设置,在里面添加 tf.saved_model.signature_constants.DEFAULT_SERVING_SIGNATURE_DEF_KEY:
prediction_signature,
其中prediction_signature是tf.saved_model.signature_def_utils.build_signature_def的定义。