# -*- coding: utf-8 -*-
import pandas as pd
import numpy as np
from datetime import date
import warnings
warnings.filterwarnings("ignore")
"""
dataset split:
(date_received)
dateset3: 20160701~20160731 (113640),features3 from 20160315~20160630 (off_test)
dateset2: 20160515~20160615 (258446),features2 from 20160201~20160514
dateset1: 20160414~20160514 (138303),features1 from 20160101~20160413
1.merchant related:
sales_use_coupon. total_coupon
transfer_rate = sales_use_coupon/total_coupon.
merchant_avg_distance,merchant_min_distance,merchant_max_distance of those use coupon
total_sales. coupon_rate = sales_use_coupon/total_sales.
2.coupon related:
discount_rate. discount_man. discount_jian. is_man_jian
day_of_week,day_of_month. (date_received)
3.user related:
distance.
user_avg_distance, user_min_distance,user_max_distance.
buy_use_coupon. buy_total. coupon_received.
buy_use_coupon/coupon_received.
avg_diff_date_datereceived. min_diff_date_datereceived. max_diff_date_datereceived.
count_merchant.
4.user_merchant:
times_user_buy_merchant_before.
5. other feature:
this_month_user_receive_all_coupon_count
this_month_user_receive_same_coupon_count
this_month_user_receive_same_coupon_lastone
this_month_user_receive_same_coupon_firstone
this_day_user_receive_all_coupon_count
this_day_user_receive_same_coupon_count
day_gap_before, day_gap_after (receive the same coupon)
"""
#,header=0,表明第0行代表列名
#off_train = pd.read_csv('data/ccf_offline_stage1_train.csv',header=0)#如果都这样写下面省不少事
#1754884 record,1053282 with coupon_id,9738 coupon. date_received:20160101~20160615,date:20160101~20160630, 539438 users, 8415 merchants
off_train = pd.read_csv('data/ccf_offline_stage1_train.csv',header=None)
off_train.columns = ['user_id','merchant_id','coupon_id','discount_rate','distance','date_received','date']
#2050 coupon_id. date_received:20160701~20160731, 76309 users(76307 in trainset, 35965 in online_trainset), 1559 merchants(1558 in trainset)
off_test = pd.read_csv('data/ccf_offline_stage1_test_revised.csv',header=None)
off_test.columns = ['user_id','merchant_id','coupon_id','discount_rate','distance','date_received']
#11429826 record(872357 with coupon_id),762858 user(267448 in off_train)
on_train = pd.read_csv('data/ccf_online_stage1_train.csv',header=None)
on_train.columns = ['user_id','merchant_id','action','coupon_id','discount_rate','date_received','date']
dataset3 = off_test
feature3 = off_train[((off_train.date>='20160315')&(off_train.date<='20160630'))|((off_train.date=='null')&(off_train.date_received>='20160315')&(off_train.date_received<='20160630'))]
dataset2 = off_train[(off_train.date_received>='20160515')&(off_train.date_received<='20160615')]
feature2 = off_train[(off_train.date>='20160201')&(off_train.date<='20160514')|((off_train.date=='null')&(off_train.date_received>='20160201')&(off_train.date_received<='20160514'))]
dataset1 = off_train[(off_train.date_received>='20160414')&(off_train.date_received<='20160514')]
feature1 = off_train[(off_train.date>='20160101')&(off_train.date<='20160413')|((off_train.date=='null')&(off_train.date_received>='20160101')&(off_train.date_received<='20160413'))]
############# other feature ##################3
"""
5. other feature:
this_month_user_receive_all_coupon_count
this_month_user_receive_same_coupon_count
this_month_user_receive_same_coupon_lastone
this_month_user_receive_same_coupon_firstone
this_day_user_receive_all_coupon_count
this_day_user_receive_same_coupon_count
day_gap_before, day_gap_after (receive the same coupon)
"""
#for dataset3
t = dataset3[['user_id']]
t['this_month_user_receive_all_coupon_count'] = 1
#将t按照用户id进行分组,然后统计所有用户收取的优惠券数目,并初始化一个索引值
t = t.groupby('user_id').agg('sum').reset_index()
t1 = dataset3[['user_id','coupon_id']]
#提取这个月用户收到的相同的优惠券的数量
t1['this_month_user_receive_same_coupon_count'] = 1
t1 = t1.groupby(['user_id','coupon_id']).agg('sum').reset_index()
t2 = dataset3[['user_id','coupon_id','date_received']]
#将数据转换为str类型
t2.date_received = t2.date_received.astype('str')
#如果出现相同的用户接收相同的优惠券在接收时间上用‘:’连接上第n次接受优惠券的时间 20160716:20160719
t2 = t2.groupby(['user_id','coupon_id'])['date_received'].agg(lambda x:':'.join(x)).reset_index()
#将接收时间的一组按着':'分开,这样就可以计算接受了优惠券的数量,apply是合并
t2['receive_number'] = t2.date_received.apply(lambda s:len(s.split(':')))
#保留领取优惠券大于1
t2 = t2[t2.receive_number>1]
t2['max_date_received'] = t2.date_received.apply(lambda s:max([int(d) for d in s.split(':')]))
t2['min_date_received'] = t2.date_received.apply(lambda s:min([int(d) for d in s.split(':')]))
#去除receive_number这列
#t2 = t2['user_id','coupon_id','max_date_received','min_date_received']不行
#等价#t2 = t2[['user_id','coupon_id','max_date_received','min_date_received']]
t2.drop(['receive_number','date_received'],axis
天池大赛o2o优惠券第一名代码笔记之_extral_feature(1)
最新推荐文章于 2024-07-18 22:21:42 发布
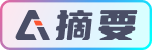